DOI:
10.1039/D1BM00400J
(Paper)
Biomater. Sci., 2021,
9, 5175-5191
Enhanced efficiency of nonviral direct neuronal reprogramming on topographical patterns†
Received
16th March 2021
, Accepted 7th June 2021
First published on 7th June 2021
Abstract
Nonviral direct neuronal reprogramming holds significant potential in the fields of tissue engineering and regenerative medicine. However, the issue of low reprogramming efficiency poses a major barrier to its application. We propose that topographical cues, which have been applied successfully to enhance lineage-directed differentiation and multipotent stem cell transdifferentiation, could improve nonviral direct neuronal reprogramming efficiency. To investigate, we used a polymer-BAM (Brn2, Ascl1, Myt1l) factor transfection polypex to reprogram primary mouse embryonic fibroblasts. Using a multiarchitecture chip, we screened for patterns that may improve transfection and/or subsequent induced neuron reprogramming efficiency. Selected patterns were then investigated further by analyzing β-tubulin III (TUJ1) and microtubule-associated protein 2 (MAP2) protein expression, cell morphology and electrophysiological function of induced neurons. Certain hierarchical topographies, with nanopatterns imprinted on micropatterns, significantly improved the percentage of TUJ1+ and MAP2+ cells. It is postulated that the microscale base pattern enhances initial BAM expression while the nanoscale sub-pattern promotes subsequent maturation. This is because the base pattern alone increased expression of TUJ1 and MAP2, while the nanoscale pattern was the only pattern yielding induced neurons capable of firing multiple action potentials. Nanoscale patterns also produced the highest fraction of cells showing spontaneous synaptic activity. Overall, reprogramming efficiency with one dose of polyplex on hierarchical patterns was comparable to that of five doses without topography. Thus, topography can enhance nonviral direct reprogramming of fibroblasts into induced neurons.
1. Introduction
Direct neuronal reprogramming is the reprogramming of adult somatic cells to adult neurons, without the introduction of an intermediary pluripotent state. This method of cellular reprogramming offers exciting new opportunities in the field of regenerative medicine, and disease modelling.1–3 Using this method, autologous cell sources for cell-based therapies can be generated in situ without a proliferative stem cell-like stage. Because cells do not have to transit a pluripotent state, expansion and subsequent differentiation, this technique is significantly faster as well.2 Further, it is speculated that the induced neuronal (iN) cells generated by direct neuronal reprogramming, can maintain aging and epigenetic signatures of the patient.3–7 It has also been shown that iN can be specified into motor neurons, cholinergic neurons, dopaminergic neurons, and serotoninergic neurons, amongst other types of neurons.8 Thus, iN cells also have potential as a cell source for in vitro disease modelling.
Induced neurons have been generated from a wide variety of cells including mouse fibroblasts,7,9–18 human fibroblasts,11,18–26 marmoset fibroblasts,27 mouse hepatocytes,28 mouse retinal ganglion cells,29 human nasal olfactory stem cells,30 mouse olfactory ensheathing cells,31 human adult peripheral T cells,32 mouse Müller glia,33 and human astrocytes.20,34–37 Reprogramming of these cells has been realized by various methods including combinations of transcription factors such as Brn2, Ascl1, Myt1l, and Neurod1, Lmx1a, and/or microRNAs such as miR-124, miR-9 and/or small molecules that regulate developmental pathways such as CHIR99021, DMH1, forskolin, Y-27632, Repsox and SP600125. The earliest and most investigated method is the use of three transcription factors Brn2, Ascl1, and Mytl1 (BAM factors) developed by Vierbuchen et al., that have been shown to reprogram both mouse and human fibroblasts.9,26 A multitude of different gene delivery methods have been employed to deliver BAM factors for direct neuronal reprogramming. So far, the most efficient method has been the use of viral gene delivery (2–20% reprogramming).9,38,39 However, the use of viruses poses challenges to clinical translation regarding safety. Nonviral methods, both physical and chemical, have been developed as alternatives. Physical methods such as electroporation or ultrasound rely on membrane disruption and in vitro have shown efficiency comparable to that of viral transfection (9–12%). However, in vivo translation remains a challenge and these methods can cause excessive cell damage.40–42 Chemical methods, such as lipids, polymers, inorganic and hybrid carriers, are more amenable to in vivo translation. However, chemical nonviral direct neuronal reprogramming systems tend to have significantly lower reprogramming efficiency (0.05–2%, with single doses).43
Studies have been able to increase the efficiency of these systems by combining them with physical methods or adding in small molecules, but this increases the overall cost and complexity. Additionally, the same barriers to in vivo translation posed by physical systems alone persist. A reprogramming method developed by Adler et al. that uses a nonviral polymer gene vector developed by Lin et al.44 called poly(N,N-cystaminebisacrylamide-4-amino-1-butanol) (poly(CBA-ABOL)), was able to increase neuronal reprogramming efficiency to almost 8% using five doses, without the incorporation of physical methods.43 While this is a significant improvement, this efficiency is still much lower than that of viral reprogramming. The issue of low reprogramming efficiency poses a significant problem as large volumes of iN cells would be required for both in vitro and in vivo applications.2,5,45
One way to improve efficiency, could be the incorporation of topography. Inspired by the phenomenon of contact guidance and the physical microenvironment of the native stem cell niche, nano- and microtopograhical substrates have been shown to enhance lineage-directed differentiation and transdifferentiation of multipotent stem cells. In these systems, topographical cues significantly increased the differentiation rate and neuronal yield. In general, anisotropic patterns have provided the most significant improvement due to their ability to alter focal adhesions and promote cytoskeletal reorganization which in turn affects gene expression.46–53 Topography has also been shown to promote nonviral gene delivery. Both anisotropic and isotropic patterns have been shown to “prime” cells before nonviral transfection by modulating their integrin binding, focal adhesion formation, cytoskeletal organization, endocytic mechanisms and intracellular trafficking.54–56 A study by Kulangara et al. even demonstrated that topography can be used to enhance viral neuronal reprogramming with BAM factors.57
Thus, we hypothesized, the incorporation of biophysical cues in the form of topography, may improve the efficiency of nonviral neuronal reprogramming. To investigate this, we used a high-throughput screening tool, the Multiarchitecture Chip (MARC), to screen fifteen patterns of varying dimensions and geometries to see which, if any, could enhance nonviral transfection and nonviral direct neuronal reprogramming. Patterns that stood out during screening were used in subsequent single pattern studies to further investigate their effect on nonviral direct neuronal reprogramming. The maturation and functionality of the iN cells produced on patterns were then characterized by expression of neuronal markers, morphology, and electrophysiological analysis.
2. Methods and materials
2.1. Molecular cloning and plasmid purification
The pUNO1-mAscl1 (3892 bp) and pUNO1-mMyt1lb (6744 bp) were purchased from InvivoGen (San Diego, CA, USA). pUNO1-mBrn2 (4513 bp) was generated by first amplifying the Brn2 cassette from pmax-Brn243 by PCR (left primer: CAAATGACCGGTCACCATGGCGACCGC, right primer: CTCCCCCTGAACCTGAAAC), creating an AgeI restriction enzyme site near the 5′ end of the amplicon. To improve cloning efficiency, the PCR product was sub-cloned into a TOPO-ligase conjugated vector. The intermediate plasmid and pUNO-mAscl1 were digested AgeI and HpaI restriction enzymes, then separated by agarose gel electrophoresis, extracted, and purified by ethanol precipitation. The Brn2 insert was ligated into the pUNO-mAscl1 backbone, transformed into DH5α E. coli (Invitrogen, Carlsbad, CA, USA), selected by colony PCR, expanded and purified (Plasmid Mini Kit, Qiagen, Hilden, Germany), and validated by sequencing, immunocytochemistry, and western blot. The reporter vector pmax-GFP (3486 bp, Amaxa, Cologne, Germany) which expresses GFP under control of CMV promoter, was used for measurement of transfection efficiency. To generate the plasmid pLV-hSyn1-GFP, pLV-hSyn-RFP (Addgene #22909) was digested with restriction enzymes AgeI and PmeI, and pmaxFP-Green-N (Amaxa, Cologne, Germany) was digested with AgeI and HpaI. Following agarose gel electrophoresis and gel extraction, the GFP fragment was ligated into the pLV-hSyn backbone and transformed into Stbl3 E. coli (Invitrogen). A sequence-validated clone was expanded, purified (EndoFree Plasmid Maxi Kit, Qiagen), and used for viral packaging.
2.2. Poly(CBA-ABOL) synthesis and bulk polyplex formation
The protocol used by Adler et al., for poly(CBA-ABOL) synthesis and bulk polyplex formation was used in this study.43 Briefly, N,N-cystaminebisacrylamide (CBA) (Polysciences, Warrington, PA) and 4-amino-1-butanol (ABOL) (Sigma-Aldrich, Saint Louis, MO) were combined via Michael polyaddition as described by Lin et al. (Fig. S1†).58 The reaction product was purified by dialysis, in acidic deionized water and then lyophilized. The polymer was collected in its HCl-salt form and its structure validated by 1H NMR (in D2O) on a Varian Mercury 300 MHz NMR Spectrometer. Lyophilized p(CBA-ABOL) was dissolved in molecular grade water at a final concentration of 50 μg μL−1. p(CBA-ABOL)/DNA nanocomplexes (polyplexes) were synthesized at a polymer
:
DNA mass ratio of 45
:
1, which was selected based on a preliminary optimization.43 Polyplexes were prepared by adding a HEPES buffer solution (20 mM HEPES, 5 wt% glucose, pH 7.4) of p(CBA-ABOL) (844 μg p(CBA-ABOL) mL−1) to a HEPES buffer solution (20 mM HEPES, 5 wt% glucose, pH 7.4) of plasmid DNA (75 μg mL−1), followed immediately by vortexing for 20 seconds. A dose of 0.25 μg DNA cm−2 was used for all experiments.
2.3. Cytotoxicity characterization and dose determination
Passage-three PMEF cells were seeded into 96-well TCPS culture plates at a density of 20
000 cells per cm2 and grown in complete PMEF media. After 24 hours, the cells were transfected with pABOL-pmaxGFP polyplexes in pre-warmed OptiMEM media for four hours, after which the media was removed and replaced with fresh PMEF media. Twenty-four hours after transfection, the culture media was replaced with fresh PMEF media also containing 10% Alamar Blue (Invitrogen). After 90 minutes incubation at 37 °C and 5% CO2, metabolic reduction of resazurin was measured at 560 nm/590 nm fluorescence excitation/emission with a Varioskan LUX plate reader (Thermo Scientific). Background signal was subtracted, and cell viability was determined by normalization to untransfected controls.
2.4. Lentiviral production
HEK293FT cells (Invitrogen) were seeded in a 75 cm2 flask, cultured in DMEM (Gibco, Carlsbad, CA, USA) containing 10% FBS (Gibco) and 25 μg mL−1 gentamicin (Gibco), and were transfected using Lipofectamine 2000 (Invitrogen) with 5.14 μg pMD2.G (Addgene #12259), 9.73 μg psPAX2 (Addgene #12260), and 15 μg of pLV-hSyn1-GFP according to the manufacturer's protocol. Culture media was discarded and replaced after one day. Three days after transfection, the medium was collected and passed through a 0.45 μm syringe filter to remove cell debris. The supernatant was then concentrated to 30× in Amicon Ultra centrifugal filter tubes (MWCO 100 kDa, Millipore, Billerica, MA, USA), and the concentrated virus was aliquoted and stored at −80 °C.
2.5. Preparation of multi-architecture chip (MARC) arrays and single patterns
Both MARC arrays and single patterns were fabricated via soft lithography on polydimethylsiloxane (PDMS) (Sylgard™184, Elastomer Kit, Dow Corning). An elastomer to curing agent ratio of 10
:
1 was prepared, degassed and then poured on to silanized PDMS master molds. Both the circular and rectangle MARC designs were used in this study (Fig. 1B). A description of MARC master mold fabrication can be found in Moe et al.59 and Ankam et al.60 A list of single patterns used in this study, a description of the pattern features, and the shortened name used to refer to the pattern can be found in Table 1. Topographical features were characterized by scanning electron microscopy (SEM) and the Olympus LEXT OLS4100 laser confocal microscope (Fig. 1C). A schematic of the MARC used for screening can be seen in Fig. 1B.
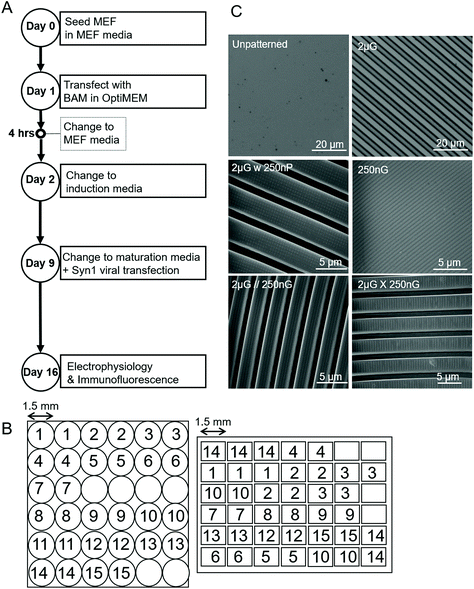 |
| Fig. 1 Parameters of experiment and materials used. (A) Experimental timeline outlining key steps over the 19-day timespan. (B) Schematic of the Multi-Architecture Array Chips (MARC) used in this study, indicating pattern distribution of pattern regions and area of pattern regions. Left, circular chip and right, rectangular chip. Numerical pattern key can be found in Table 1. (C) Images of the unpatterened control (unp), and 2 × 2 × 2 μm gratings (2 μG), taken with the LEXT Olympus5000 microscope. Images of 250 × 250 × 250 nm gratings (250 nG), 2 × 2 × 2 μG with hierarchical 250 nm pillars (2 μG w 250 nP), 2 × 2 × 2 μG with hierarchical parallel 250 × 250 × 250 nm gratings (2 μG//250 nG) and 2 × 2 × 2 μG with hierarchical with perpendicular 250 × 250 × 250 nm gratings (2 μG ∟ 250 nG) taken with scanning electron microscopy (SEM). | |
Table 1 Pattern descriptions, pattern shorthand, and numerical key
No. in Fig. 1B |
Pattern |
Description |
Unp |
Unpatterned control |
1 |
2 μG |
2 μm width, 2 μm space, 2 μm height gratings |
2 |
1 × 2 μG |
1 μm width, 2 μm space, 120 nm depth gratings |
3 |
2 × 1 μG |
2 μm width, 1 μm space, 80 nm depth gratings |
4 |
250 × 250 × 150 nG |
250 nm width, 250 nm space, 150 nm depth gratings |
5 |
1 μH |
1 μm holes, 6.5 μm pitch, 1 μm depth |
6 |
2 μP |
2 μm diameter, 12 μm pitch, 2 μm height pillars |
7 |
2 μG ∟ 250 nG |
2 × 2 × 2 μm gratings with perpendicular 250 × 250 × 250 nm hierarchical gratings |
8 |
2 μG//250 nG |
2 × 2 × 2 μm gratings with parallel 250 × 250 × 250 nm hierarchical gratings |
9 |
2 μG w 250 nP |
2 × 2 × 2 μm gratings with 250 × 250 × 250 nm pillars |
10 |
250 nP |
250 nm diameter, 400 μm pitch, 250 nm height pillars |
11 |
250 nG |
250 nm width, 250 nm space, 250 nm depth gratings |
12 |
1 μL convex |
1 μm pitch, 300 nm sag microlens, convex |
13 |
1 μL concave |
1 μm pitch, 300 nm sag microlens, concave |
14 |
1.8 μL convex |
1.8 μm diameter, 2 μm pitch, 700 nm sag microlens, convex |
15 |
1.8 μL concave |
1.8 μm diameter, 2 μm pitch, 700 nm sag microlens, concave |
Samples were placed under vacuum for at least 1 h to remove any air bubbles and then moved to a 60 °C oven to cure overnight. Once cured, the PDMS samples were left in the oven for an additional seven days. Samples were then stored at room temperature until use. Samples were air-plasma treated for two minutes (Harrick Expanded Plasma Cleaner). Prior to seeding cells, samples were sterilized for one hour with 75% ethanol and ultraviolet irradiation. They were then placed in a six-well tissue culture plate and coated with 100 μL of fibronectin (Sigma) in sterile water (50 μg mL−1) in a 37 °C incubator for one hour. Chips were next washed with PBS then seeded with cells.
2.6. Cell culture and transfection
Primary mouse embryonic fibroblasts (PMEF) (ATCC) between passage two and four were used for all experiments. Cells were expanded from a seeding density of 20
000 cells per cm2 in a TCPS T25 flask. Cells were incubated at 37 °C and 5% CO2 in complete PMEF medium: Dulbecco's Modified Eagle's Medium, high glucose with L-glutamine and phenol red (GIBCO 11965092) (Invitrogen), 15% (v/v) fetal bovine serum (Invitrogen), and 1% (v/v) penicillin streptomycin (Invitrogen). Half of the media was changed every other day. After expansion, PMEFs were seeded at a density of 20
000 cells per cm2 onto substrates. After 24 h, PMEFs were transfected with p(CBA-ABOL)/DNA nanocomplexes containing either pUNO-BAM cocktails or pmax-GFP plasmid. All transfections were carried out in serum- and antibiotic-free OptiMEM (Invitrogen) media. OptiMEM was replaced with complete PMEF medium four hours after the onset of transfection. After 24 hours, PMEF medium was replaced with neuronal induction medium containing: DMEM/F-12 (Invitrogen), 1% Glutamax 100× (Invitrogen), 1% penicillin streptomycin 100× (Invitrogen), 1% N2 Supplement 100× (Invitrogen), 2% B27 Supplement 50× (Invitrogen). A supplement of bFGF (Invitrogen) at final concentration of 5 ng ml−1 was added daily. Two-thirds of media was changed every other day. After seven days in induction media, on day 9, the media was changed to maturation media containing: a 1
:
1 ratio of DMEM/F-12 (Invitrogen) and Neurobasal medium (Invitrogen), 1% Glutamax 100× (Invitrogen), 1% penicillin streptomycin 100× (Invitrogen), 0.25% N2 Supplement 100× (Invitrogen), 2% B27 Supplement 50× (Invitrogen). Growth factors were discontinued from this point onwards. Also on day 9, cells were transduced with the synpasin1-GFP reporter lentivirus, pLV-hSyn1-eGFP, at a concentration of 0.5 μL virus concentrate per cm2. Two-thirds of media was changed every other day until day 16, when cells were fixed and stained, or electrophysiology was done. An overview of the experimental timeline can be seen in Fig. 1A.
2.7. Immunofluorescence and image analysis
Cells were fixed with 4% paraformaldehyde, permeabilized with 0.25% Triton X-100 and blocked with 1% (w/v) bovine serum albumin and 10% (v/v) goat serum in 1× tris-buffered saline (TBS). Next cells were immunostained with β-tubulin III (TUJ1) (rabbit anti-TUJ1 at 1
:
1000, polyclonal, Sigma Aldrich), microtubule associated protein (MAP2) (mouse anti-microtubule associated protein at 1
:
600, polyclonal, Abcam) overnight at 4 °C. Samples were then washed with washing buffer composed of 0.05% Triton X-100 and 1% goat serum in 1× TBS. Secondary staining was done with Alexa Fluor 488 goat anti-rabbit IgG at 1
:
1000 (Invitrogen) for TUJ1 and Alexa Fluor 546 goat anti-mouse IgG at 1
:
1000 (Invitrogen) for MAP2, overnight at 4 °C. Samples were counterstained with DAPI at 1
:
2200 to label nuclei for one hour at room temperature. Imaging was done using a Zeiss fluorescence microscope (Axio Observer Z1) and analyzed using ImageJ software.
For the first round of screening which aimed to determine the transfection efficiency on various patterns, transfection efficiency for the ith pattern, Ti, was calculated as,
and then averaged across biological replicates. For the second round of screening which aimed to determine the reprogramming efficiency on various patterns after BAM transfection, induced neurons were considered to be any cell that expressed TUJ1. The fraction of induced neurons for the
ith pattern,
Ni, was calculated as,
These values were then converted to reprogramming efficiency for each pattern, Ri, as follows,
The reprogramming efficiency for each pattern was then normalized to the reprogramming efficiency of the control. In single pattern studies, to determine the percentage of marker positive iN cells, the criteria outlined in Yang et al. was used. The criteria states that for a cell to be considered an iN it needs to express a neuronal marker and have distinct neuronal morphology. Distinct neuronal morphology is defined as a round soma with at least one thin protruding neurite.61 Cells that expressed a marker but had fibroblastic morphology were considered failed iN cells and thus were not included in the count of marker positive iN cells in single pattern studies. An example of a failed iN compared to a successful iN can be seen in Fig. S2† The ImageJ plugin NeuriteJ was used for measuring neurite length.62
2.8. Electrophysiology
Cells were identified for patch clamp analysis by synapsin-1 promoter-driven GFP expression after 16 days in culture as described in section 2.5. Cells were visualized and patched using the Axon Multiclamp 700B Microelectrode Amplifier (Molecular Devices, Sunnyvale, CA). The micropipettes used for clamping in this system had a resistance of 4–8 MΩ. The cells were perfused with 50 mM HEPES buffer. Giga-Ohm membrane seals were formed under voltage-clamp conditions. Whole-cell current-clamp was done using step-wise increments of 20 pA, starting at −20 pA up to 160 pA for 1 second. Whole-cell voltage-clamp was done using step-wise increments of 5 mV starting at −60 mV up to 40 mV. Spontaneous synaptic currents were recorded in the interim between voltage steps at the resting membrane potential of the neurons. Analysis was done using Clampfit (Molecular Devices).
2.9. Statistical analysis
Data were presented as the mean and standard error of mean (SEM). Data analysis was performed using one-way analysis of variance (ANOVA), followed by post-hoc Fisher's LSD test. The Student's t-test was used to calculate p-values. p values of <0.05 were considered statistically significant. For the screening experiments, a biological replica is one MARC chip. Each MARC chip contains two to three pattern regions (technical replica) per pattern per sample. Two images were taken of each of the two to three pattern regions per pattern. For the single pattern experiments, a biological replica was a single PDMS sample (approximately 1 cm2). Four to seven images were captured per sample per replica. In total approximately 700 to 1200 cells were analyzed per sample per replica. For neurite length analysis on single patterns, approximately 25–100 cells were measured for each group. Counting varied depending on the number of cells available. For neurite length statistical analysis, n was taken to be the number of cells analyzed.
3. Results
3.1. Effect of substrate topography on nonviral transfection efficiency
To screen patterns that may affect the nonviral delivery aspect of neuronal reprogramming, we used GFP as a reporter of transfection. Using p(ABOL) polyplexes, 0.25 μg cm−2 doses of pmax-GFP were delivered to PMEF cells seeded on PDMS MARC chips. A dosage of 0.25 μg DNA cm−2 was selected based on cytotoxicity. We aimed to have the highest dose possible without reducing viability. A dose of 0.25 μg DNA cm−2 had approximately 100% viability whereas the 0.5 μg DNA cm−2 dose had only 58% viability (Fig. S3†).
Using image cytometry, it was found that there were no statistically significant differences in the percentage of GFP+ cells on the different patterns. Most patterns seemed to perform comparatively well to each other and the unpatterned blank (Fig. 2). Patterns were classified by feature size and orientation to determine if any correlations exist between feature types and transfection efficiency. Moderate positive correlations between %-GFP+ cells and anisotropic patterns (R = 0.28), and %-GFP+ cells and hierarchical patterns (R = 0.31) were observed. A moderate negative correlation between %-GFP+ cells and isotropic patterns (R = −0.34) was also observed. There was no notable correlation between %-GFP+ cells and nano- or microscale features.
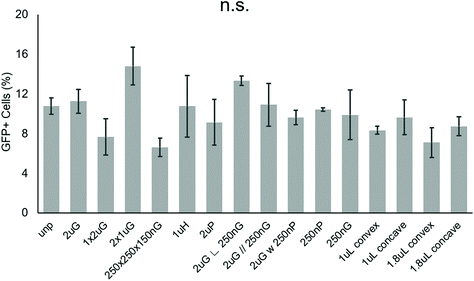 |
| Fig. 2 Effect of topography on pABOL polyplex GFP transfection efficiency. Data shown as average of N = 2 biological replica (each with 2–3 technical replicas) with standard error mean (SEM). | |
3.2. Effect of substrate topography on nonviral neuronal cellular reprogramming
3.2.1. Effect on neuronal cellular reprogramming efficiency.
Next, we screened the patterns for those that may affect the reprogramming efficiency, which would be quantified by the percentage of cells that become iN after one dose of BAM factors (0.25 μg DNA cm−2). After transfection, cells were allowed to mature for two weeks and were then stained for TUJ1, a pan neuronal cytoskeletal marker. The percentage of TUJ1-postive (TUJ1+) cells on each pattern was determined using image cytometry as isolation of cells from patterns for qPCR was not feasible. PMEFs cultured on the unpatterned controls were converted to TUJ1+ cells at a rate of 0.8% ± 0.4%. Fig. 3A shows morphology and fraction of TUJ1+ cells on each of the 15 patterns screened and the unpatterned control.
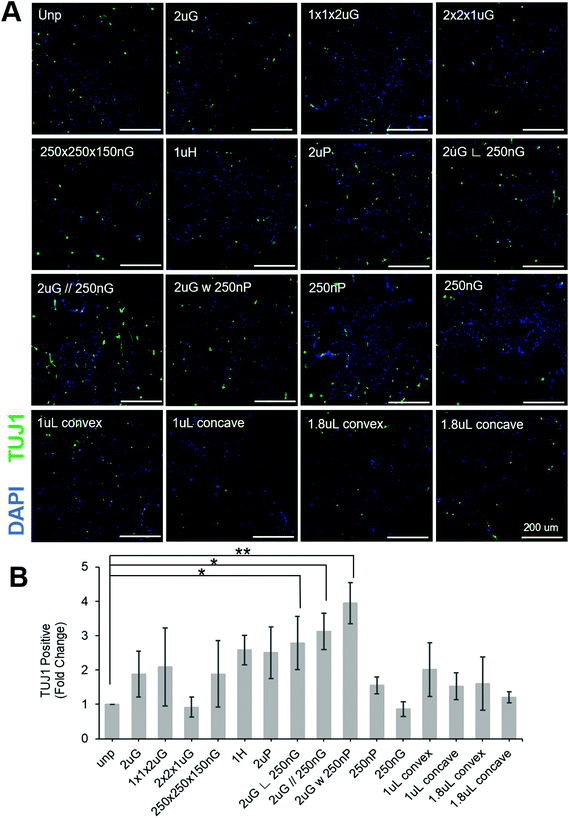 |
| Fig. 3 Screening of topographies on the MARC to determine if any can enhance neuronal reprogramming efficiency using pABOL polyplex. (A) Representative images of induced neuronal (iN) cells stained with beta tubulin III (TUJ1) and DAPI on MARC topographies. All scale bars are 200 μm. (B) Quantification of the generation of TUJ1+ cells on distinct MARC patterns following normalization to the transfection efficiency measured for each pattern. Data are shown as average of N = 5 biological replica (each with 2–3 technical replicas) with SEM. (*, p ≤ 0.05, **, p ≤ 0.01). | |
To separate the effects of patterns on transfection efficiency and reprogramming efficiency, patterns were compared using a fold change normalized to GFP transfection efficiency (see section 2.6 for explanation of calculations). Most patterns did not affect neuronal reprogramming efficiency. However, we were able to identify three patterns that significantly improved neuronal reprogramming efficiency (Fig. 3B). These patterns included 2 μm lines with hierarchical perpendicular 250 nm lines (2 μG ∟ 250 nG), 2 μm lines with hierarchical parallel 250 nm lines (2 μG//250 nG), and 2 μm lines with hierarchical 250 nm pillars (2 μG w 250 nP) (p = 0.038, p = 0.016, and p = 0.002, respectively). All these patterns yielded at least a twofold improvement compared to the reprogramming efficiency of the control. We were also able to see cells with distinct neuronal morphology on these patterns, further confirming iN reprogramming (Fig. 3A). Interestingly, isolation of the components of the hierarchical patterns (2 μG, 250 nG, and 250 nP) did not yield notable changes in reprogramming efficiency.
3.2.2. Effect on resulting iN cell maturity and morphology.
Paracrine signaling effects can occur when using a MARC chip for pattern screening cells as all patterns share the same pool of soluble factors. Thus, to further validate the effects of patterns of interest, they should be tested individually. Based on the results of pattern screening in section 3.2.1, three patterns were selected for further investigation: 2 μG, 250 nG and 250 μG ∟ 250 nG. The hierarchical pattern 250 μG ∟ 250 nG was selected as it performed significantly better than the control. The patterns 2 μG and 250 nG were selected as they are components of the chosen hierarchical pattern. We delivered a single 0.25 μg cm−2 dose of pUNO-BAM factors in p(ABOL) polyplexes to PMEFs on MARC chips. After transfection, cells were allowed to mature for two weeks and were then stained for TUJ1 and MAP2.
ANOVA analysis indicated that patterns had a significant effect on both the percentage of TUJ1+ and MAP2+ iN cells (p = 0.004 and p = 0.013, respectively). In general, all patterns and the control had a higher amount of MAP2+ iN cells than TUJ1+ iN cells (Fig. 4A and B). Only the hierarchical pattern, 2 μG ∟ 250 nG, significantly increased the percentage of TUJ1+ and MAP2+ iN cells compared to the unpatterned control (p = 0.027 and p = 0.007, respectively). On the 2 μG ∟ 250 nG pattern, 6% ± 0.3% of cells were TUJ1+ and 9% ± 0.7% of cells were MAP2+. Similar to what was observed in MARC chip screening, the isolated components of the hierarchical patterns, 2 μG and 250 nG, did not significantly increase the percentage of TUJ1+ and MAP2+ iN. However, while not statistically significant, the pattern 2 μG tended to perform comparatively well to the hierarchical pattern. On the 2 μG sample, 4% ± 0.8% of cells were TUJ1+ and 8% ± 1.5% of cells were MAP2+. The percentage of TUJ1+ and MAP2+ of 250 nG was consistent with that of the control and had the most variation of all the groups. On the 250 nG pattern, 2% ± 1% of cells were TUJ1+ and 3% ± 2.2% of cells were MAP2 positive. On the unpatterened control, 1% ± 0.01% of cells were TUJ1+ and 2% ± 0.5% of cells were MAP2+. Despite the hierarchical substrate having the highest percentage of TUJ1+ and MAP2+ iN cells, the highest yield of TUJ1+ and MAP2+ iN cells was found on the 2 μG substrate (Fig. 4C). The 2 μG substrate on average had 9 ± 3 TUJ1+ iN cells per mm2 and 17 ± 6 MAP2+ iN cells per mm2. The hierarchical pattern only had 4 ± 3 TUJ1+ iN cells per mm2 and 6 ± 5 MAP2+ iN cells per mm2. The 250 nG and unpatterned substrates had notably lower yields. The 250 nG substrate had a yield of 3 ± 1 TUJ1+ iN cells per mm2 and 3 ± 3 MAP2+ iN cells per mm2. The unpatterned substrate had a yield of 1 ± 0 TUJ1+ iN cells per mm2 and 2 ± 1 MAP2+ iN cells per mm2. However, ANOVA analysis indicated there was no statistically significant difference in the yield of marker-positive iN cells between the different substrates (p = 0.08 and p = 0.06, for TUJ1 and MAP2, respectively).
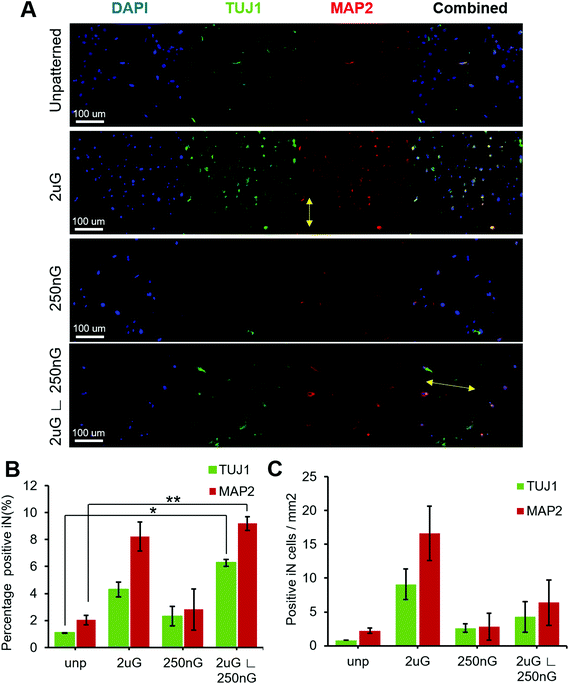 |
| Fig. 4 Single pattern analysis of the effect of topography on neuronal reprogramming efficiency using pABOL polyplex. (A) Representative images of beta tubulin III (TUJ1), microtubule-associated protein 2 (MAP2) expression on the unpatterened control (unp), 2 × 2 × 2 μm gratings (2 μG), 250 × 250 × 250 nm gratings (250 nG), and 2 × 2 × 2 μG with hierarchical with perpendicular 250 × 250 × 250 nm gratings (2 μG ∟ 250 nG). Yellow arrow on 2 μG image indicates direction of pattern. Yellow arrow on 2 μG ∟ 250 nG image indicates direction of base pattern. (B) Percentage of iN cells that express TUJ1, and MAP2, and (C) number of cells per mm2 of substrate that express TUJ1, and MAP2. Data shown as the average and SEM (*, p < 0.05, **, p < 0.01). N = 2 biological replicates, with approximately 700 to 1200 cells were analyzed per sample per replica; 4–7 images were captured at random location per sample. | |
Next, we considered whether topography influenced iN morphology. ANOVA analysis indicated that patterns had a significant effect on the average neurite length (p = 2 × 10−8). On 2 μG samples, cells elongated parallel to the grooves (Fig. 4A and 5A) and were highly aligned (Fig. S4†). The average neurite length was 44 μm ± 30 μm. On the 250 nG samples, orientation of cells relative to the grooves could not be determined (Fig. 4A and 5A) but cells did not align themselves in any particular direction (Fig. S4†). Despite many cells on the 250 nG pattern retaining fibroblastic morphology, those that were successfully reprogrammed had a longer average neurite length, 54 μm ± 29 μm, compared to the 2 μG samples. Though, this difference was not statistically significant. A similar behavior was noted on the unpatterned control. The average neurite length on the unpatterned control was 47 μm ± 26. On the hierarchical pattern, iN cells had an average neurite length, 78 ± 49 μm, that was significantly longer than the 2 μG and 250 nG patterns, and the unpatterned control (p = 3 × 10−7, p = 0.0007 and p = 9 × 10−5, respectively). On the hierarchical pattern, cells elongated parallel to the base grooves (2 μG) (Fig. 4A and 5A) however looking at their alignment it can be seen they also tended to cross the base grooves more often than cells on the 2 μG pattern (Fig. S4†). As it can be seen in Fig. S4,† there is a notable subset of cells that align themselves perpendicular to the main alignment group, which would be the cells aligned to the base grooves. In other words, neurites aligned with both the microgrooves and hierarchical (∟ 250 nG) grooves (Fig. 4A and 5A). The length of neurite outgrowth was quantified by measuring approximately 25–100 MAP2+ outgrowths per pattern. The broad range of the number of outgrowths measured was due to the low reprogramming efficiency of some patterns. Quantifying the length of the iN cell neurite outgrowth, it was found that iN cells on the hierarchical pattern, 2 μG ∟ 250 nG, had statistically significantly longer extensions (78 ± 49 μm) than both the individual 2 μG (44 ± 30 μm) and 250 nG (54 ± 29 μm) patterns, and the unpatterned control (47 ± 26 μm) (Fig. 5B). However, despite the consistent alignment of outgrowth with pattern grooves, iN cells on the 2 μG pattern had the shortest average neurite length of all groups.
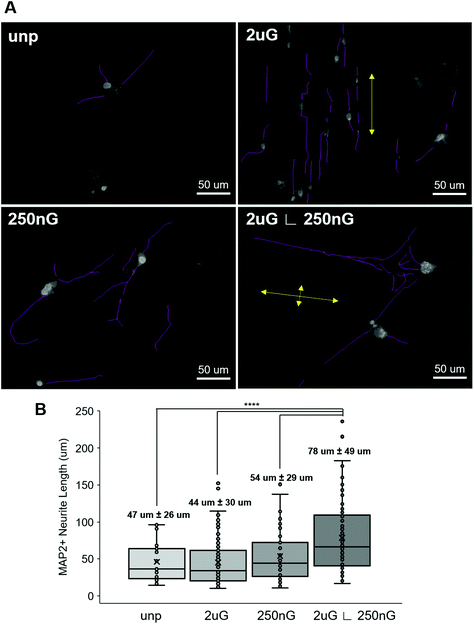 |
| Fig. 5 Neurite length and orientation relative to patterns. (A) Representative images of iN cell morphology, neurites and orientation relative to pattern expression on the unpatterened control (unp), 2 × 2 × 2 μm gratings (2 μG), 250 × 250 × 250 nm gratings (250 nG), and 2 × 2 × 2 μG with hierarchical with perpendicular 250 × 250 × 250 nm gratings (2 μG ∟ 250 nG). Large yellow arrows indicate the direction of the microscale pattern and small yellow arrows indicate direction of nanoscale hierarchical pattern. (B) Distribution and average length of microtubule-associate protein 2 (MAP2) positive neurites on patterns compared to the unpatterened control. Average with 95% confidence interval labelled for each group, where n = the number of cells analyzed. Data are obtained 25–100 cells were for each group. (****, p < 0.0001). | |
3.2.3. Effect on resulting iN cell functionality.
To further determine the reprogramming success on single patterns and subsequent maturity of the iN cells, both voltage- and current-clamp patch clamping was performed. In addition to BAM transfection, cells on single patterns were transduced via lentivirus with a GFP driven by a Syn1 promoter to identify which cells should be patched. Expression of synapsin usually indicates electrophysiological capabilities. This promoter was used so that Syn1 positive cells would be easier to verify as it allows for whole-cell fluorescence when Syn1 is expressed. Direct synapsin staining results in very small fluorescent puncta which are more difficult to visualize while patch clamping. Two independent patch-clamp experiments, each measuring 3–12 cells per pattern, were performed.
All patterned samples had cells capable of firing at least one action potential in response to current injection but only the 250 nG pattern had cells capable of firing multiple action potentials (Fig. 6D). ANOVA analysis indicated there was no statistically significant change in the percentage of cells capable of firing an action potential on patterns. The 2 μG pattern, 4/11 iN cells fired at least one action potential but none fired multiple action potentials. On the 250 nG pattern, 7/15 cells fired at least one action potential and of those cells, 5/7 fired repetitive action potentials. However, these repeated spikes had much lower amplitude than the initial spike. On the hierarchical pattern, 5/15 cells fired at least one action potential, but none fired repetitive action potentials. On the unpatterned control, no action potentials could be recorded. Representative voltage plots for each substrate can be seen in Fig. 6B.
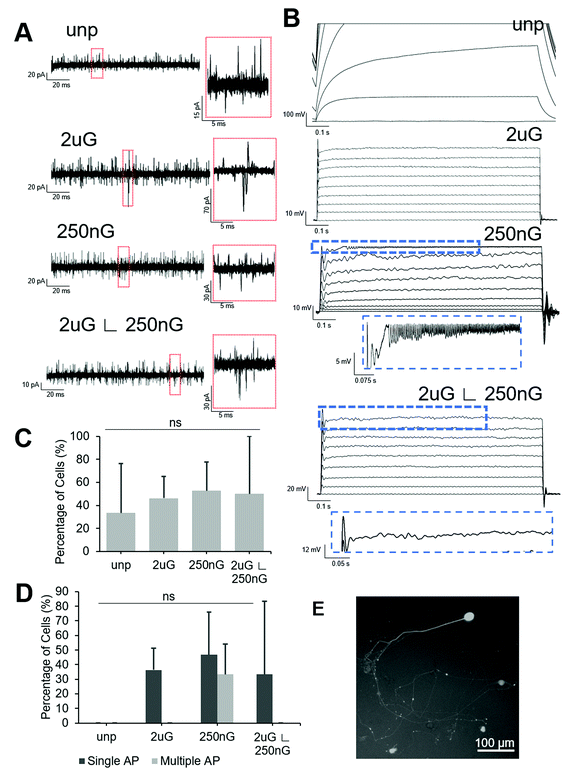 |
| Fig. 6 Electrophysiological properties of induced neuronal (iN) cells on patterns compared to the unpatterened control. Representative image of (A) current profiles from voltage-clamping demonstrating evoked and spontaneous postsynaptic currents (magnified in red box) and (B) action potentials from current-clamping. Scales shown next to each plot. From top to bottom: unpatterened control (unp), 2 × 2 × 2 μm gratings (2 μG), 250 × 250 × 250 nm gratings (250 nG), and 2 × 2 × 2 μG with hierarchical with perpendicular 250 × 250 × 250 nm gratings (2 μG ∟ 250 nG). (C) Percentage of iN cells demonstrating spontaneous synaptic activity. (D) Percentage of iN cells firing a single action potential (AP) or multiple AP. Data were collected in two independent experiments from 3–12 cells per experiment. (E) Representative image of induced neuron expressing GFP after transfection with lentiviral Syn1-GFP promoter. | |
Square voltage test pulses were delivered to cells, and spontaneous synaptic activity was recorded in intervals between stimuli. To differentiate between spontaneous synaptic currents and noise, a threshold of 15 pA, and a peak showing sharp rise and slow decay were used as selection criteria. Representative current plots for each substrate can be found in Fig. 6A. ANOVA analysis there was no statistically significant change in the percentage of cells capable of spontaneous synaptic activity on patterns. About half of the cells tested on patterns showed spontaneous synaptic currents (Fig. 6C). On the 2 μG pattern 6/12 iN cells had spontaneous synaptic activity. On the 250 nG pattern, 10/19 cells had spontaneous synaptic activity. On the hierarchical pattern 5/10 cells had spontaneous synaptic activity. On the unpatterned control only 4/12 cells had spontaneous synaptic activity.
4. Discussion
This study aimed to identify patterns that may enhance the direct nonviral neuronal reprogramming of PMEF via transfection with BAM factors using a bioreducible linear poly(amido amine) carrier. We hypothesized topographical patterns would enhance the efficiency of direct nonviral neuronal reprogramming. A differentiation period of 14 days was selected based on the timing and kinetics of reprogramming observed in early direct neuronal reprogramming studies. Treutlein et al. showed that using primary mouse embryonic fibroblasts the neuronal identity is maintained and matures after 22 days in cells transduced with BAM factors.63 Further, Vierbuchen et al., found functional synapses are only formed 2 to 3 weeks into the neuronal reprogramming process using BAM factors.9 Hence a 14-day differentiation period was selected for this study. However, as the culture period extends there is a risk that fibroblasts may begin to express neuronal markers and grow neurites, which would be classified as failed iN. Thus, in single pattern studies to prevent the inclusion of failed iN in cell counts, the criteria outline by Yang et al. regarding identifying induced neurons based on morphology were utilized.61 Fig. S2,† illustrates the difference between a successful iN and a failed iN.
To determine which patterns, if any, affected the nonviral neuronal reprogramming, we used the multi-architecture chip (MARC) as a template on PDMS as a screening tool.59 We then performed single pattern studies. PDMS was selected to as the MARC chip substrate as it is simple and inexpensive to fabricate, offers high resolution in the nanoscale and is easy to coat with extracellular matrix such as fibronectin. PDMS has also been shown to be a useful platform for in vitro screening64–67 and cell expansion for cell-based therapy.68–70 Single pattern studies were also performed using PDMS to avoid issues with variability and other potential confounding effects. Additionally, for patch clamping very thin (μm), optically transparent samples are required which can be easily replicated in bulk using PDMS. While this study aimed to screen patterns and determine if any could enhance topography, future in-depth studies could consider using electrospinning and 3D printing. By translating the findings of this study to biocompatible materials such as hydrogels and fibrous meshes, the effects of topography on nonviral direct neuronal reprogramming in a 3D environment and in vivo could be investigated.
Using the MARC chip, we were able to screen 16 distinct patterns that contained anisotropic and/or isotropic features in the nanoscale and/or microscale. Two rounds of screening were done to better discern how topography affects neuronal reprogramming. We considered the overall process of nonviral transdifferentiation of fibroblasts to neurons to be, broadly, comprised of two main processes: (1) the delivery and uptake of BAM plasmids, and (2) neuronal reprogramming, comprised of expression of BAM genes with subsequent iN emergence and maturation. The first round of screening focused on the first process and investigated the effect of topography on transfection efficiency using a GFP reporter. The second round of screening focused on the second process and investigated the effect of topography on neuronal reprogramming efficiency after transfection with BAM factors. No patterns had a statistically significant effect on transfection efficiency though three patterns were shown to significantly improve neuronal reprogramming. It is important to note, however, that the first round of screening involved the uptake of only one gene (GFP). During BAM reprogramming cells must take up three plasmids and express each at sufficiently high levels for successful reprogramming.9 The effects of topographies on transfection efficiency with the GFP reporter were minimal, but the incremental improvement seen with a one plasmid transfection could be amplified when three plasmids are used.
The significant difference in the fold change of TUJ1+ positive cells on certain patterns in the second round of screening implies that topographies affect the second step of neuronal reprogramming, more than the first. A fold change and normalization to transfection efficiency was used in the second round of screening to decouple the reprogramming effect from any transfection effects. It should be noted though, on occasion TUJ1 can be expressed in cells that do not fully convert into iNs and still have fibroblastic morphology.57 In this round of screening only the hierarchical patterns were shown to have a significant effect on neuronal reprogramming efficiency, 2 μG ∟ 250 nG, 2 μG//250 nG, and 2 μG w 250 nP. Similar to the findings of Kulangara et al. and most lineage-directed neuronal differentiation studies, these are anisotropic patterns. Interestingly, while the hierarchical patterns performed the best overall, their microscale base pattern and their respective nanoscale hierarchical patterns alone did not have a significant effect on iN development. This implied a cooperative or synergistic effect between the base pattern and the hierarchical pattern.
To further investigate the effect of topography on nonviral neuronal reprogramming, and the synergistic effect of hierarchical patterns, single pattern studies were performed. The 2 μG ∟ 250 nG hierarchical pattern was selected as it performed better than the unpatterned control in the first round of screening and resulted in a significant fold change in TUJ1+ cells in the second round of screening. The patterns of which this hierarchical pattern is comprised, 2 μG and 250 nG, were selected so we could better understand the synergistic effect observed in the screening. An unpatterned substrate was used as the control. In general, all substrates had a higher percentage of TUJ1+ cells in single pattern studies, compared to that observed during screening, perhaps due to paracrine signaling depressing pattern effects. In general, compared to the study from Adler et al. which used the same nonviral reprogramming system on blank substrates with various doses, patterns greatly improved neuronal reprogramming efficiency with only one dose. The unpatterned substrate with one dose from this study and the sample with one dose from Adler et al. performed comparatively well (0.67% TUJ1+ and 0.47% TUJ1+, respectively). The 2 μG ∟ 250 nG substrate with one dose in this study performed better than the five-dose sample from Adler et al. The 2 μG sample with one dose performed comparatively well to the five-dose sample. The 250 nG sample with one dose, which showed a low percentage of TUJ1+ cells compared to other patterns in this study, still performed better than the three-dose sample from Adler et al.43
In general, all substrates showed a trend of higher yield and percentage of MAP2+ cells, compared to TUJ1+ cells on the same substrate. Though this difference was not statistically significant, it implies mature cells have been derived. During neuronal development, the expression window of TUJ1 has considerable overlap with MAP2 expression, however, the MAP2 expression window extends further.71 The hierarchical pattern, 2 μG ∟ 250 nG, was the only pattern to have a significantly higher percentage of TUJ1+ and MAP2+ iN cells compared to the unpatterned substrate. However, the 2 μG sample also had a high percentage of TUJ1+ and MAP2+ iN cells and had the highest average yield of TUJ1+ and MAP2+ cells per area. A high yield but non-significant change in the percentage of TUJ1+ and MAP2+ iN cells indicates that the total number of cells on this substrate was greater than that of the other substrates. On the other substrates, this phenomenon was not observed. This implies that the 2 μG pattern improves cell adhesion or cell survival, but it is less efficient in reprogramming the attached cells as the 2 μG ∟ 250 nG pattern. Overall, this implies the base pattern of 2 μG helps with induction to the neuronal phenotype after transfection with BAM factors. It also suggests that this base pattern may help promote initial BAM expression. Since the BAM transcription factors are what give rise to the induced neuronal phenotype, it follows that cells must be expressing BAM factors if they are expressing neuronal markers and become iN. Further, if certain patterns increase the percentage of cells that express neuronal markers, they must therefore also be affecting the fraction of cells expressing BAM factors. While it could be argued that there will be some cells that express BAM factors but to do not become induced neurons, as we are interested in the fraction of cells that become induced neurons, we elected to look at the effect of topography on marker expression in cells with neuronal morphology rather than BAM expression. Future studies could investigate BAM expression as well to further elucidate the effects of topography on direct neuronal reprogramming.
Looking at neurite length, the synergistic effect of the components of the hierarchical patterns becomes clearer. On the hierarchical pattern, cells had a significantly longer average neurite length and neurites were able to extend parallel to both the microscale base pattern and the hierarchical nanoscale pattern. In both transdifferentiation and lineage-directed differentiation, it has been shown that microscale gratings can improve the rate of neuronal marker expression, as they help cells to elongate. By providing contact guidance cues to cells, gratings promote the elongated neuronal morphology which in turn alters focal adhesions, cytoskeletal arrangement, and subsequently gene expression.46,51,72–76 However, the effect of contact guidance on direct neuronal reprogramming and the mechanisms involved have been less explored. In this study, once reprogrammed, as the iN cells mature, the iN neurites could either continue to follow the base grooves or move in other directions by following the hierarchical grooves. Previous studies have shown that the effects of topography can be additive, and the optimal topography may vary during different phase of differentiation.46,77 Tan et al. showed that induced pluripotent stem cells undergoing neuronal differentiation, matured fastest when first induced on microgratings then transferred to micropillars. The secondary isotropic pattern allowed committed neurons to have more neuronal complexity by not restricting their direction of extension.77 Studying lineage-directed neuronal differentiation, Chua et al. proposed shallower gratings were easier for neurites to extend which increased the number of directions in which the neurite could travel.78 Similarly, a study by Yang et al., investigating neuronal transdifferentiation of mesenchymal stem cells, showed hierarchical patterns help to reduce cell contractility and subsequently decrease nuclear localization of Yes-associated protein (YAP/TAZ), increasing neurogenesis.79 A study by Abadi et al. studying the effect of hierarchical topography on cardiomyocyte differentiation of induce pluripotent stem cells, found that the microscale patterns enhance maturation and the sub-micrometer patterns further accelerated maturation. By analyzing in vitro gene expression and functioning, combined with a virtual cell model, they showed these synergistic effects were due to reorganization of the cytoskeletal network and regulation of chromatin formation.80
The results of electrophysiology analysis also support this proposed synergistic effect. Despite showing the lowest percentage and yield of neuronal marker positive cells amongst the patterns, the 250 nG substrate had iN cells with the most mature electrophysiological functionalities. In addition to neuronal marker expression, the successful neuronal cellular reprogramming of PMEFs requires that the resulting iN cells display neuronal functionality.61 Features such as the ability to generate action potentials upon depolarization and synaptic functioning indicate successful neuronal reprogramming. More mature features, such as repetitive action potentials and spontaneous synaptic currents with high amplitudes, can be used to indicate the extent of reprogramming. The 250 nG substrate had the highest percentage of cells showing at least one action potential and was the only pattern to have iN cells that were capable for firing action potential trains. The 250 nG pattern also had the highest percentage of cells with spontaneous synaptic activity. Thus, like what was seen in the analysis of neurite length, the 250 nG pattern helped to promote cell maturation once iN cells had committed to the neuronal lineage. The 2 μG substrate and the 2 μG ∟ 250 nG substrate, had similar percentages of cells capable of firing action potentials. The unpatterned substrate did not have any iN cells capable of firing an action potential. This is consistent with previous studies using this nonviral carrier for neuronal reprogramming of PMEF with BAM factors, wherein three doses were required to see iN cells capable of firing action potentials.43
Improving neuronal reprogramming efficiency has been a major goal for nonviral neuronal reprogramming techniques, as a large quantity of cells are required for potential cell therapies. Our findings that hierarchical patterns can significantly increase the percentage of TUJ1+ and MAP2+ cells and produce mature cells capable of firing an action potential and showing spontaneous synaptic activity, shows that patterns can enhance neuronal reprogramming efficiency in vitro. Further, as previously stated, this significant improvement in efficiency was seen using only one dose. Adler et al. showed that with five doses, efficiency could be improved to 8% reprogramming,43 whereas we showed with a single dose on optimal topography, efficiency could be improved to 9% reprogramming. Thus, it is speculated that with multiple doses on optimal topography, efficiency could be comparable to that of virus-based protocols (2–20%).9,38,39 Further studies should investigate the combination of topography with multiple doses.
Topography is low-cost, and a variety of well-developed techniques exist for imparting detailed topographies in both the micro- and nano-range on wide variety of different materials. Importantly, many techniques for controlling topography of biocompatible materials such as hydrogels and injectable electro-spun fibrous meshes with modifiable fiber orientation have been developed.81–84 These materials have also widely been used in vivo. Topographical cues and fibrous meshes are very commonly used to enhance artificial nerve guides in both the central and peripheral nervous systems.112–114 Injectable fibrous biomaterials have also been used in vivo for central nervous system (CNS) repair. Biomaterial-based nerve conduits and injectable gels enhanced with neural or mesenchymal stem cells, or stem cell derived neurons have also been investigated.85–100 Nonviral gene delivery vectors have also been combined with biomaterial scaffolds for in vivo transfection. Raferty et al. used a polypeptide-based gene carrier in a porous collagen scaffold in a Wistar rat model to repair bone defects and found host cell infiltration, localized transfection and sustained changes in gene expression could all be observed.101 Walsh et al. used a collagen-based scaffold and polypeptide-base gene carrier to successfully transfect autologous host cells after infiltration in vivo.102 This is particularly interesting as it has been shown that BAM transcription factors can be used to directly reprogram astrocytes into neurons.20,34–37 Astrocytes are present in CNS trauma and are abundant throughout the brain.103,104 Other genetic engineering techniques such as CRISPR/Cas9 have been combined with biomaterials to create substrates that can mediate localized delivery of CRISPR/Cas9 systems for neuronal regeneration.105,106 This demonstrates the potential to translate the use of topographical cues to enhance direct neuronal reprogramming from in vitro to in vivo.
Further, for nervous system repair topography to enhance direct neuronal reprogramming presents itself as an interesting system for ex vivo genetic engineering, wherein patient cells are modified in vitro and then re-transplanted.107 Therapeutics of this nature have been developed using CRISPR/Cas9107 suggesting they could also be developed for BAM nonviral direct neuronal reprogramming of fibroblasts. It has been shown that induced neuronal cells do not revert to their original cell type.3,108 To the best of the authors’ knowledge, whether induced neurons can be detached from substrates and remain functional is currently unknown. Studies have shown that cells, including neurons, can maintain their phenotypes after being detached from topographies due to their mechanical memory, so there is a possibility this could also be the case with iN cells.109–111 However, this may be cell dependent and thus whether iN can also be detached and retain their phenotype warrants further investigation. Beyond in vivo use for cell therapies, in vitro study platforms using iN are particularly promising for disease modelling as it is believed that iN can maintain patient age.3–7
While the exact mechanisms behind the observed enhancement in this study are not clear, previous studies have shown topography can influence gene expression via nuclear deformation caused by cytoskeletal contraction. For example, chromatin remodeling and epigenetic regulation following elongation due to topography have been observed by various groups.51,72,115,116 For example, using human mesenchymal stem cells and human embryonic stem cells, Ankam et al. shows that nanotopography can directly modify nucleus morphology. This change in morphology resulted in modified laminin A/C expression, and histone methylation.73 Seeing as cellular reprogramming involves large scale changes in the epigenome it is expected that these changes to chromatin and epigenetic regulation caused by topography are the likely cause of reprogramming enhancement.117,118 Yoo et al. used nanotopography to enhance direct lineage reprogramming of fibroblasts to induced cardiomyocytes and found that this enhancement was due to changes in the activation of focal adhesion kinase and histone modification.118 Yoo et al. also investigated the effect of nanotopography on direct neuronal reprogramming and found that there was an amplification of histone H3 tri-methylation at lysine4 (H3K4me3) on nanopatterned substrates. This epigenetic change is closely associated with transcription activation at early stages of cellular reprogramming.22 By investigating changes in the epigenome and localization of transcriptional regulators like YAP/TAZ, in conjunction with mechanotransduction pathway components such as focal adhesions, Rho GTPase signaling and cell contractility, the roles of the microscale and nanoscale patterns comprising hierarchical patterns can be better understood.
5. Conclusion
The findings of this study indicate that topography can be used to enhance nonviral BAM factor direct neuronal reprogramming of embryonic mouse fibroblasts using a polyplex carrier. By performing screening on MARC chips, we were able to select patterns that affected transfection and/or neuronal reprogramming efficiency. We found that anisotropic patterns may be able to enhance transfection efficiency but the effect was not significant. Meanwhile, hierarchical patterns showed a significant effect on increasing neuronal reprogramming efficiency. Further investigating using single pattern studies, we speculated that the base pattern of microscale gratings could be responsible for improving induction to the neuronal phenotype after BAM transfection. The secondary pattern on the hierarchical pattern, the 250 nG perpendicular to the base gratings, was speculated to be responsible for promoting subsequent maturation and development of iN cells. Using hierarchical patterns, we were able to increase efficiency with one dose to a level was similar to previous studies using five doses. The combination of multiple doses and topography may have the potential to produce an even more efficient system. Future optimization of patterns and dosing may be able to provide efficiencies that bring direct neuronal reprogramming closer to being clinically feasible.
Author contributions
Conceptualization: EKFY, KWL, CLG. Data curation: EKFY, KWL, SM, MR, CLG. Formal analysis: SM, MR, CLG. Funding acquisition: EKFY, KWL. Investigation: SM, MR, CLG, AMZ. Methodology: EKFY, KWL, SM, MR, CLG, AMZ. Project administration: EKFY. Resources: EKFY, KWL, CLG. Software: n/a. Supervision: EKFY, KWL. Validation: SM, MR. Visualization: SM. Writing – original draft: SM, EKFY. Writing – review & editing: EKFY, KWL, SM, MR, CLG.
Conflicts of interest
The authors declare no conflicts of interest.
Acknowledgements
The authors would like to thank Dr Seok Hong Goh, Mr Jack Moffat, Dr David Spafford, Dr Mark Bruder for technical assistance. This research was funded by Natural Science and Engineering Research Council (NSERC) Discovery (2016040), the Center for Biotechnology and Bioengineering Seed Fund from the University of Waterloo and the University of Waterloo Startup Fund, and partially supported by Canada Foundation for Innovation (CFI)-John R. Evans Leaders Fund (JELF) (35573) and NSERC Research Tools and Instruments (RTI-2018-00220). SM was supported by the NSERC Canada through the Alexander Graham Bell Canada Graduate Scholarships – Master's program, NSERC CREATE (401207296) and the University of Waterloo Engineering Excellence Fellowship.
References
- D. Srivastava and N. DeWitt, Cell, 2016, 166, 1386–1396 Search PubMed.
- H. Wang, Y. Yang, J. Liu and L. Qian, Nat. Rev. Mol. Cell Biol., 2021,(22), 410–424 Search PubMed.
- S. Gascón, G. Masserdotti, G. L. Russo and M. Götz, Cell Stem Cell, 2017, 21, 18–34 Search PubMed.
- J. Mertens, J. R. Herdy, L. Traxler, S. T. Schafer, J. C. M. Schlachetzki, L. Böhnke, D. A. Reid, H. Lee, D. Zangwill, D. P. Fernandes, R. K. Agarwal, R. Lucciola, L. Zhou-Yang, L. Karbacher, F. Edenhofer, S. Stern, S. Horvath, A. C. M. Paquola, C. K. Glass, S. H. Yuan, M. Ku, A. Szücs, L. S. B. Goldstein, D. Galasko and F. H. Gage, Cell Stem Cell, 2021,(28), 1–16 Search PubMed.
- J. Drouin-Ouellet, K. Pircs, R. A. Barker, J. Jakobsson and M. Parmar, Front. Neurosci., 2017, 11, 530 Search PubMed.
- N. N. Tran, I. G. Ladran and K. J. Brennand, Schizophr. Bull., 2013, 39, 4–10 Search PubMed.
- C. Y. Luo, Q. Y. Lee, O. Wapinski, R. Castanon, J. R. Nery, M. Malin, M. S. Kareta, S. M. Cullen, M. A. Goodell, H. Y. Chang, M. Wernig and J. R. Ecker, eLife, 2019, 8, e40197 Search PubMed.
- Z. Xu, S. Su, S. Zhou, W. Yang, X. Deng, Y. Sun, L. Li and Y. Li, Cell Biosci., 2020, 10, 116 Search PubMed.
- T. Vierbuchen, A. Ostermeier, Z. P. Pang, Y. Kokubu, T. C. Südhof and M. Wernig, Nature, 2010, 463, 1035–1041 Search PubMed.
- H. Ahlenius, S. Chanda, A. E. Webb, I. Yousif, J. Karmazin, S. B. Prusiner, A. Brunet, T. C. Sudhof and M. Wernig, Proc. Natl. Acad. Sci. U. S. A., 2016, 113, 8514–8519 Search PubMed.
- S. Chanda, C. E. Ang, J. Davila, C. Pak, M. Mall, Q. Y. Lee, H. Ahlenius, S. W. Jung, T. C. Sudhof and M. Wernig, Stem Cell Rep., 2014, 3, 282–296 Search PubMed.
- Y. Jin, J. S. Lee, J. Kim, S. Min, S. Wi, J. H. Yu, G. E. Chang, A. N. Cho, Y. Choi, D. H. Ahns, S. R. Cho, E. Cheong, Y. G. Kim, H. P. Kim, Y. Kim, D. S. Kim, H. W. Kim, Z. Quan, H. C. Kang and S. W. Cho, Nat. Biomed. Eng., 2018, 2, 522–539 Search PubMed.
- Y. Jin, J. Seo, J. S. Lee, S. Shin, H. J. Park, S. Min, E. Cheong, T. Lee and S. W. Cho, Adv. Mater., 2016, 28, 7365–7374 Search PubMed.
- A. Grande, K. Sumiyoshi, A. Lopez-Juarez, J. Howard, B. Sakthivel, B. Aronow, K. Campbell and M. Nakafuku, Nat. Commun., 2013, 4, 12 Search PubMed.
- C. Sheng, Q. Zheng, J. Wu, Z. Xu, L. Sang, L. Wang, C. Guo, W. Zhu, M. Tong, L. Liu, W. Li, Z.-H. Liu, X.-Y. Zhao, L. Wang, Z. Chen and Q. Zhou, Cell Res., 2012, 22, 769–772 Search PubMed.
- B. J. Wainger, E. D. Buttermore, J. T. Oliveira, C. Mellin, S. Lee, W. A. Saber, A. J. Wang, J. K. Ichida, I. M. Chiu, L. Barrett, E. A. Huebner, C. Bilgin, N. Tsujimoto, C. Brenneis, K. Kapur, L. L. Rubin, K. Eggan and C. J. Woolf, Nat. Neurosci., 2015, 18, 17–24 Search PubMed.
- D. W. Han, N. Tapia, A. Hermann, K. Hemmer, S. Höing, M. J. Araúzo-Bravo, H. Zaehres, G. Wu, S. Frank and S. Moritz, Cell Stem Cell, 2012, 10, 465–472 Search PubMed.
- K. L. Ring, L. M. Tong, M. E. Balestra, R. Javier, Y. Andrews-Zwilling, G. Li, D. Walker, W. R. Zhang, A. C. Kreitzer and Y. Huang, Cell Stem Cell, 2012, 11, 100–109 Search PubMed.
- P. Wang, H. L. Zhang, W. G. Li, H. Y. Sha, C. S. Xu, L. Yao, Q. S. Tang, H. L. Tang, L. P. Chen and J. H. Zhu, Stem Cells Dev., 2014, 23, 16–23 Search PubMed.
- O. Torper, U. Pfisterer, D. A. Wolf, M. Pereira, S. Lau, J. Jakobsson, A. Björklund, S. Grealish and M. Parmar, Proc. Natl. Acad. Sci. U. S. A., 2013, 110, 7038 Search PubMed.
- R. Ambasudhan, M. Talantova, R. Coleman, X. Yuan, S. Zhu, S. A. Lipton and S. Ding, Cell Stem Cell, 2011, 9, 113–118 Search PubMed.
- J. Yoo, M. Noh, H. Kim, N. L. Jeon, B. S. Kim and J. Kim, Biomaterials, 2015, 45, 36–45 Search PubMed.
- M. Caiazzo, M. T. Dell'Anno, E. Dvoretskova, D. Lazarevic, S. Taverna, D. Leo, T. D. Sotnikova, A. Menegon, P. Roncaglia, G. Colciago, G. Russo, P. Carninci, G. Pezzoli, R. R. Gainetdinov, S. Gustincich, A. Dityatev and V. Broccoli, Nature, 2011, 476, 224–227 Search PubMed.
- X. Y. Wan, L. Y. Xu, B. Li, Q. H. Sun, Q. L. Ji, D. D. Huang, L. Zhao and Y. T. Xiao, Int. J. Mol. Med., 2018, 41, 1463–1468 Search PubMed.
- W. Hu, B. Qiu, W. Guan, Q. Wang, M. Wang, W. Li, L. Gao, L. Shen, Y. Huang, G. Xie, H. Zhao, Y. Jin, B. Tang, Y. Yu, J. Zhao and G. Pei, Cell Stem Cell, 2015, 17, 204–212 Search PubMed.
- Z. P. Pang, N. Yang, T. Vierbuchen, A. Ostermeier, D. R. Fuentes, T. Q. Yang, A. Citri, V. Sebastiano, S. Marro and T. C. Südhof, Nature, 2011, 476, 220–223 Search PubMed.
- A. Nemoto, R. Kobayashi, S. Yoshimatsu, Y. Sato, T. Kondo, A. S. Yoo, S. Shiozawa and H. Okano, Cells, 2021, 10, 6 Search PubMed.
- S. Marro, Z. P. P. Pang, N. Yang, M. C. Tsai, K. Qu, H. Y. Chang, T. C. Sudhof and M. Wernig, Cell Stem Cell, 2011, 9, 374–382 Search PubMed.
- Y. Lu, B. Brommer, X. Tian, A. Krishnan, M. Meer, C. Wang, D. L. Vera, Q. Zeng, D. Yu and M. S. Bonkowski, Nature, 2020, 588, 124–129 Search PubMed.
- A. Chabrat, E. Lacassagne, R. Billiras, S. Landron, A. Pontisso-Mahout, H. Darville, A. Dupront, F. Coge, E. Schenker, D. Piwnica, E. Nivet, F. Féron and C. Mannoury La Cour, Stem Cells Int., 2019, 2019, 1–15 Search PubMed.
- X. Sun, Z. Tan, X. Huang, X. Cheng, Y. Yuan, S. Qin, D. Wang, X. Hu, Y. Gu and W.-J. Qian, Cell Death Dis., 2019, 10, 1–17 Search PubMed.
- K. Tanabe, C. E. Ang, S. Chanda, V. H. Olmos, D. Haag, D. F. Levinson, T. C. Südhof and M. Wernig, Proc. Natl. Acad. Sci. U. S. A., 2018, 115, 6470–6475 Search PubMed.
- M. Lahne, M. Nagashima, D. R. Hyde and P. F. Hitchcock, Annu. Rev. Vis. Sci., 2020, 6, 171–193 Search PubMed.
- M.-H. Liu, W. Li, J.-J. Zheng, Y.-G. Xu, Q. He and G. Chen, Neural Regen. Res., 2020, 15, 342–351 Search PubMed.
- J.-C. Yin, L. Zhang, N.-X. Ma, Y. Wang, G. Lee, X.-Y. Hou, Z.-F. Lei, F.-Y. Zhang, F.-P. Dong, G.-Y. Wu and G. Chen, Stem Cell Rep., 2019, 12, 488–501 Search PubMed.
- L. Gao, W. Guan, M. Wang, H. Wang, J. Yu, Q. Liu, B. Qiu, Y. Yu, Y. Ping, X. Bian, L. Shen and G. Pei, Stem Cell Rep., 2017, 8, 538–547 Search PubMed.
-
K. Aravantinou-Fatorou and D. Thomaidou, in Stem Cells and Tissue Repair, Springer US, 2020, pp. 41–61 Search PubMed.
- A. McCaughey-Chapman and B. Connor, Stem Cells Dev., 2018, 27, 1674–1692 Search PubMed.
- C. E. Ang and M. Wernig, J. Comp. Neurol., 2014, 522, 2877–2886 Search PubMed.
- J. Long, H. Kim, D. Kim, J. B. Lee and D.-H. Kim, J. Mater. Chem. B, 2017, 5, 2375–2389 Search PubMed.
- J. Fang, Y.-Y. Hsueh, J. Soto, W. Sun, J. Wang, Z. Gu, A. Khademhosseini and S. Li, ACS Nano, 2020, 14, 1296–1318 Search PubMed.
- D. Gallego-Perez, J. J. Otero, C. Czeisler, J. Ma, C. Ortiz, P. Gygli, F. P. Catacutan, H. N. Gokozan, A. Cowgill, T. Sherwood, S. Ghatak, V. Malkoc, X. Zhao, W.-C. Liao, S. Gnyawali, X. Wang, A. F. Adler, K. Leong, B. Wulff, T. A. Wilgus, C. Askwith, S. Khanna, C. Rink, C. K. Sen and L. J. Lee, Nanomedicine, 2016, 12, 399–409 Search PubMed.
- A. F. Adler, C. L. Grigsby, K. Kulangara, H. Wang, R. Yasuda and K. W. Leong, Mol. Ther. Nucleic Acids, 2012, 1, e32–e32 Search PubMed.
- C. Lin, Z. Zhong, M. C. Lok, X. Jiang, W. E. Hennink, J. Feijen and J. F. J. Engbersen, Bioconjugate Chem., 2007, 18, 138–145 Search PubMed.
- R. H. Liou, T. L. Edwards, K. R. Martin and R. C. Wong, Int. J. Mol. Sci., 2020, 21(12), 4273 Search PubMed.
- D. Jain, S. Mattiassi, E. L. Goh and E. K. F. Yim, Neural Regen. Res., 2020, 15, 573–585 Search PubMed.
- S. Ding, P. Kingshott, H. Thissen, M. Pera and P. Y. Wang, Biotechnol. Bioeng., 2017, 114, 260–280 Search PubMed.
- H. K. Kim, E. Kim, H. Jang, Y.-K. Kim and K. Kang, ChemNanoMat, 2017, 3, 278–287 Search PubMed.
- A. T. Nguyen, S. R. Sathe and E. K. F. Yim, J. Phys.: Condens. Matter, 2016, 28, 183001 Search PubMed.
- C. Simitzi, K. Karali, A. Ranella and E. Stratakis, ChemPhysChem, 2018, 19, 1143–1163 Search PubMed.
- E. K. F. Yim and M. P. Sheetz, Stem Cell Res. Ther., 2012, 3, 41 Search PubMed.
- M. Poudineh, Z. Wang, M. Labib, M. Ahmadi, L. Zhang, J. Das, S. Ahmed, S. Angers and S. O. Kelley, Nano Lett., 2018, 18, 7188–7193 Search PubMed.
- B. S. Eftekhari, M. Eskandari, P. A. Janmey, A. Samadikuchaksaraei and M. Gholipourmalekabadi, Adv. Funct. Mater., 2020, 30, 1907792 Search PubMed.
- R. L. Youngblood, N. F. Truong, T. Segura and L. D. Shea, Mol. Ther., 2018, 26, 2087–2106 Search PubMed.
- R. K. Singh, J. C. Knowles and H.-W. Kim, J. Tissue Eng., 2019, 10, 2041731419877528 Search PubMed.
- L. Larsson, S. P. Pilipchuk, W. V. Giannobile and R. M. Castilho, J. Biomed. Mater. Res., Part B, 2018, 106, 2065–2071 Search PubMed.
- K. Kulangara, A. F. Adler, H. Wang, M. Chellappan, E. Hammett, R. Yasuda and K. W. Leong, Biomaterials, 2014, 35, 5327–5336 Search PubMed.
- C. Lin, Z. Zhong, M. C. Lok, X. Jiang, W. E. Hennink, J. Feijen and J. F. Engbersen, Bioconjugate Chem., 2007, 18, 138–145 Search PubMed.
- A. A. Moe, M. Suryana, G. Marcy, S. K. Lim, S. Ankam, J. Z. Goh, J. Jin, B. K. Teo, J. B. Law, H. Y. Low, E. L. Goh, M. P. Sheetz and E. K. Yim, Small, 2012, 8, 3050–3061 Search PubMed.
- S. Ankam, M. Suryana, L. Y. Chan, A. A. Moe, B. K. Teo, J. B. Law, M. P. Sheetz, H. Y. Low and E. K. Yim, Acta Biomater., 2013, 9, 4535–4545 Search PubMed.
- N. Yang, Y. H. Ng, Z. P. Pang, T. C. Südhof and M. Wernig, Cell Stem Cell, 2011, 9, 517–525 Search PubMed.
- A. Torres-Espín, D. Santos, F. González-Pérez, J. del Valle and X. Navarro, J. Neurosci. Methods, 2014, 236, 26–39 Search PubMed.
- B. Treutlein, Q. Y. Lee, J. G. Camp, M. Mall, W. Koh, S. A. M. Shariati, S. Sim, N. F. Neff, J. M. Skotheim, M. Wernig and S. R. Quake, Nature, 2016, 534, 391–395 Search PubMed.
- T. Takemuro, H. Yamamoto, S. Sato and A. Hirano-Iwata, Jpn. J. Appl. Phys., 2020, 59, 117001 Search PubMed.
- M. Ristola, L. Sukki, M. M. Azevedo, A. I. Seixas, J. B. Relvas, S. Narkilahti and P. Kallio, J. Micromech. Microeng., 2019, 29, 065009 Search PubMed.
- C. Forró, G. Thompson-Steckel, S. Weaver, S. Weydert, S. Ihle, H. Dermutz, M. J. Aebersold, R. Pilz, L. Demkó and J. Vörös, Biosens. Bioelectron., 2018, 122, 75–87 Search PubMed.
- C. Chen, X. Dong, K.-H. Fang, F. Yuan, Y. Hu, M. Xu, Y. Huang, X. Zhang, D. Fang and Y. Liu, Acta Pharm. Sin. B, 2019, 9, 557–564 Search PubMed.
- F. Sarvi, Z. Yue, K. Hourigan, M. C. Thompson and P. P. Y. Chan, J. Mater. Chem. B, 2013, 1, 987–996 Search PubMed.
- L. H. Lambert, G. K. E. Goebrecht, S. E. De Leo, R. S. O'Connor, S. Nunez-Cruz, T.-D. Li, J. Yuan, M. C. Milone and L. C. Kam, Nano Lett., 2017, 17, 821–826 Search PubMed.
- K. Futrega, K. Atkinson, W. B. Lott and M. R. Doran, Tissue Eng., Part C, 2017, 23, 200–218 Search PubMed.
- A. Caceres, G. Banker and L. Binder, J. Neurosci., 1986, 6, 714–722 Search PubMed.
- S. Ankam, C. K. Lim and E. K. Yim, Biomaterials, 2015, 47, 20–28 Search PubMed.
- S. Ankam, B. K. K. Teo, G. Pohan, S. W. L. Ho, C. K. Lim and E. K. F. Yim, Front. Bioeng. Biotechnol., 2018, 6, 69 Search PubMed.
- K. Wang, A. Bruce, R. Mezan, A. Kadiyala, L. Wang, J. Dawson, Y. Rojanasakul and Y. Yang, ACS Appl. Mater. Interfaces, 2016, 8, 5082–5092 Search PubMed.
- K. Yang, K. Jung, E. Ko, J. Kim, K. I. Park, J. Kim and S. W. Cho, ACS Appl. Mater. Interfaces, 2013, 5, 10529–10540 Search PubMed.
- J. M. Stukel and R. K. Willits, Tissue Eng., Part B, 2016, 22, 173–182 Search PubMed.
- K. K. B. Tan, W. W. M. Lim, C. Chai, M. Kukumberg, K. L. Lim, E. L. K. Goh and E. K. F. Yim, Sci. Rep., 2018, 8, 9567 Search PubMed.
- J. S. Chua, C. P. Chng, A. A. Moe, J. Y. Tann, E. L. Goh, K. H. Chiam and E. K. Yim, Biomaterials, 2014, 35, 7750–7761 Search PubMed.
- L. Yang, K. M. Jurczak, L. Ge and P. Rijn, Adv. Healthcare Mater., 2020, 9, 2000117 Search PubMed.
- P. P. S. S. Abadi, J. C. Garbern, S. Behzadi, M. J. Hill, J. S. Tresback, T. Heydari, M. R. Ejtehadi, N. Ahmed, E. Copley, H. Aghaverdi, R. T. Lee, O. C. Farokhzad and M. Mahmoudi, Adv. Funct. Mater., 2018, 28, 1707378 Search PubMed.
- J. J. Norman and T. A. Desai, Ann. Biomed. Eng., 2006, 34, 89–101 Search PubMed.
- H. Jeon, C. G. Simon Jr. and G. Kim, J. Biomed. Mater. Res., Part B, 2014, 102, 1580–1594 Search PubMed.
- K. T. M. Tran and T. D. Nguyen, J. Sci.: Adv. Mater. Devices, 2017, 2, 1–14 Search PubMed.
- M. Rizwan, G. S. L. Peh, H.-P. Ang, N. C. Lwin, K. Adnan, J. S. Mehta, W. S. Tan and E. K. F. Yim, Biomaterials, 2017, 120, 139–154 Search PubMed.
- A. R. Nectow, K. G. Marra and D. L. Kaplan, Tissue Eng., Part B, 2012, 18, 40–50 Search PubMed.
- S. Yao, X. Liu, S. Yu, X. Wang, S. Zhang, Q. Wu, X. Sun and H. Mao, Nanoscale, 2016, 8, 10252–10265 Search PubMed.
- S. E. Thomson, C. Charalambous, C.-A. Smith, P. M. Tsimbouri, T. Déjardin, P. J. Kingham, A. M. Hart and M. O. Riehle, Acta Biomater., 2017, 60, 220–231 Search PubMed.
- E. C. Spivey, Z. Z. Khaing, J. B. Shear and C. E. Schmidt, Biomaterials, 2012, 33, 4264–4276 Search PubMed.
- E. Biazar, M. T. Khorasani, N. Montazeri, K. Pourshamsian, M. Daliri, M. Rezaei, M. Jabarvand, A. Khoshzaban, S. Heidari, M. Jafarpour and Z. Roviemiab, Int. J. Nanomed., 2010, 5, 839–852 Search PubMed.
- Y.-A. Huang, C. T. Ho, Y.-H. Lin, C.-J. Lee, S.-M. Ho, M.-C. Li and E. Hwang, Macromol. Biosci., 2018, 18, 1800335 Search PubMed.
- G. Li, S. Li, L. Zhang, S. Chen, Z. Sun, S. Li, L. Zhang and Y. Yang, ACS Appl. Mater. Interfaces, 2019, 11, 37397–37410 Search PubMed.
- C.-Y. Yang, W.-Y. Huang, L.-H. Chen, N.-W. Liang, H.-C. Wang, J. Lu, X. Wang and T.-W. Wang, J. Mater. Chem. B, 2020, 567–584 Search PubMed.
- W. Liu, B. Xu, W. Xue, B. Yang, Y. Fan, B. Chen, Z. Xiao, X. Xue, Z. Sun, M. Shu, Q. Zhang, Y. Shi, Y. Zhao and J. Dai, Biomaterials, 2020, 243, 119941 Search PubMed.
- A. J. Ciciriello, D. R. Smith, M. K. Munsell, S. J. Boyd, L. D. Shea and C. M. Dumont, ACS Biomater. Sci. Eng., 2020, 6, 5771–5784 Search PubMed.
- Y. Zou, Y. Zhao, Z. Xiao, B. Chen, D. Ma, H. Shen, R. Gu and J. Dai, ACS Biomater. Sci. Eng., 2020, 6, 1671–1680 Search PubMed.
- A. L. Carlson, N. K. Bennett, N. L. Francis, A. Halikere, S. Clarke, J. C. Moore, R. P. Hart, K. Paradiso, M. Wernig, J. Kohn, Z. P. Pang and P. V. Moghe, Nat. Commun., 2016, 7, 10862 Search PubMed.
- A. Higuchi, Q.-D. Ling, Y. Chang, S.-T. Hsu and A. Umezawa, Chem. Rev., 2013, 113, 3297–3328 Search PubMed.
- S. W. P. Kemp, S. K. Walsh and R. Midha, Neurol. Res., 2008, 30, 1030–1038 Search PubMed.
- E. Onode, T. Uemura, K. Takamatsu, T. Yokoi, K. Shintani, S. Hama, Y. Miyashima, M. Okada and H. Nakamura, Sci. Rep., 2021, 11, 4204 Search PubMed.
- M. Ikeda, T. Uemura, K. Takamatsu, M. Okada, K. Kazuki, Y. Tabata, Y. Ikada and H. Nakamura, J. Biomed. Mater. Res., Part A, 2014, 102, 1370–1378 Search PubMed.
- R. M. Raftery, D. P. Walsh, L. Blokpoel Ferreras, I. Mencía Castaño, G. Chen, M. Lemoine, G. Osman, K. M. Shakesheff, J. E. Dixon and F. J. O'Brien, Biomaterials, 2019, 216, 119277 Search PubMed.
- D. P. Walsh, R. M. Raftery, I. M. Castaño, R. Murphy, B. Cavanagh, A. Heise, F. J. O'Brien and S.-A. Cryan, J. Controlled Release, 2019, 304, 191–203 Search PubMed.
- M. D. Laird, J. R. Vender and K. M. Dhandapani, Neurosignals, 2008, 16, 154–164 Search PubMed.
- J. E. Burda, A. M. Bernstein and M. V. Sofroniew, Exp. Neurol., 2016, 275, 305–315 Search PubMed.
- K. Zhang, W. H. Chooi, S. Liu, J. S. Chin, A. Murray, D. Nizetic, D. Cheng and S. Y. Chew, Biomaterials, 2020, 256, 120225 Search PubMed.
- J. S. Chin, W. H. Chooi, H. Wang, W. Ong, K. W. Leong and S. Y. Chew, Acta Biomater., 2019, 90, 60–70 Search PubMed.
- Y. Li, Z. Glass, M. Huang, Z.-Y. Chen and Q. Xu, Biomaterials, 2020, 234, 119711 Search PubMed.
- G. Masserdotti, S. Gascón and M. Götz, Development, 2016, 143, 2494–2510 Search PubMed.
- C. Yang, M. W. Tibbitt, L. Basta and K. S. Anseth, Nat. Mater., 2014, 13, 645–652 Search PubMed.
- R. Muhammad, G. S. L. Peh, K. Adnan, J. B. K. Law, J. S. Mehta and E. K. F. Yim, Acta Biomater., 2015, 19, 138–148 Search PubMed.
- L. Y. Chan, W. R. Birch, E. K. F. Yim and A. B. H. Choo, Biomaterials, 2013, 34, 382–392 Search PubMed.
- D. Hoffman-Kim, J. A. Mitchel and R. V. Bellamkonda, Annu. Rev. Biomed. Eng., 2010, 12, 203–231 Search PubMed.
-
J. I. Kim, C. S. Kim and C. H. Park, in Cutting-Edge Enabling Technologies for Regenerative Medicine, ed. H. J. Chun, C. H. Park, I. K. Kwon and G. Khang, Springer Singapore, Singapore, 2018, pp. 395–408 Search PubMed.
- S. Vijayavenkataraman, Acta Biomater., 2020, 106, 54–69 Search PubMed.
- E. K. F. Yim, E. M. Darling, K. Kulangara, F. Guilak and K. W. Leong, Biomaterials, 2010, 31, 1299–1306 Search PubMed.
- A. K. Yip, N. Anh Tuan, M. Rizwan, S. T. Wong, K.-H. Chiam and E. K. F. Yim, Biomaterials, 2018, 181, 103–112 Search PubMed.
- J. Yoo, M. Noh, H. Kim, N. L. Jeon, B.-S. Kim and J. Kim, Biomaterials, 2015, 45, 36–45 Search PubMed.
- J. Yoo, Y. Chang, H. Kim, S. Baek, H. Choi, G.-J. Jeong, J. Shin, H. Kim, B.-S. Kim and J. Kim, J. Biomed. Nanotechnol., 2017, 13, 269–279 Search PubMed.
Footnotes |
† Electronic supplementary information (ESI) available. See DOI: 10.1039/d1bm00400j |
‡ These authors contributed equally to this work. |
|
This journal is © The Royal Society of Chemistry 2021 |
Click here to see how this site uses Cookies. View our privacy policy here.