DOI:
10.1039/D0BM01943G
(Review Article)
Biomater. Sci., 2021,
9, 2362-2383
Hydrogels to engineer tumor microenvironments in vitro
Received
15th November 2020
, Accepted 28th December 2020
First published on 13th January 2021
Abstract
In recent years, hydrogel-based three-dimensional tumor models have become increasingly mainstream for cancer research. Hydrogels enable recapitulation of biochemical and biophysical cues in the tumor microenvironment (TME) for the culture of cancer and stromal cells. While there is increasing insight into how cancer–stromal interactions support tumor progression and drug resistance, much remains to be understood for the successful development of therapeutic targets that are capable of controlling tumors in patients. This review aims to first describe both acellular and cellular characteristics of the TME, focusing on cancer cell interactions with the extracellular matrix, fibroblasts, endothelial cells and immune cells. We will then discuss hydrogel systems that have been developed in the past four years to mimic these interactions in the TME and finally propose future directions in the field of in vitro tumor modeling.
1. Introduction
Despite advances in therapeutic strategies and diagnostic capabilities in the last few decades, cancer is still the second leading cause of death worldwide.1 Treating cancer has always been a major challenge due to the extensive heterogeneity, complexity, and dynamism of the tumor microenvironment (TME).2 Within the TME of many cancers, there is a plethora of different stromal cell populations and sub-populations3 which engage in heterotypic and homotypic crosstalk with cancer cells and each other, collectively contributing to tumor progression and drug resistance.4,5 These complex interactions occur through different mechanisms, including paracrine signaling6 and physical contact.7
The recapitulation of patient tumors in vitro and in vivo as tumor models enables mechanistic studies into cancer biology as well as drug development and personalized drug testing. These models range from simple two-dimensional (2D) monolayer cultures, to more in vivo-like three-dimensional (3D) spheroid and organoid cultures, to complex tumor xenografts and genetically-modified animal models.8 Historically, cancer cells have been cultured as immortalized cell lines on flat tissue culture plastic due to the low cost, ease of handling and robust reproducibility; however it is now well-recognized that these cell lines generally do not maintain the inherent cancer heterogeneity found in patient tumors due to clonal expansion and selection after prolonged passaging on plastic.9 Furthermore, given the limited capacity for manipulation, 2D tumor models poorly recapitulate the tumor extracellular matrix (ECM), spatial distribution of cells within the TME, as well as the crosstalk between cancer and stromal cells. On the other end of the spectrum, murine models have also been widely used in cancer research since the late 1960s.10 With their inherent complexity and recapitulation of the TME at least in part, murine models enable the study of cancers in a more physiologically and pathologically relevant context. However, the use of animal models in preclinical research can be very expensive, and there exists species-specific differences in the TME between humans and animals. For example, there are notable differences between human and mouse immune systems such as the proportion of immune components (e.g. toll-like receptors, leukocytes and antibody subsets).11 As such, cross-species extrapolation of data obtained from murine models may not be valid in certain circumstances.12 Additionally, there could be genetic differences between animals and humans such as the expression levels of drug-metabolizing enzymes.13 Accordingly, 3D in vitro tumor models have been developed to serve as a bridge between simple 2D tumor models and complex in vivo tumor models and they have been shown to not only better recapitulate cancer cell signaling in vivo,14 but also enable the recapitulation of all-human cancer–stromal interactions in vitro.15
In this review, we focus on highlighting various strategies that have been developed in the past four years to reconstruct the TME in vitro with cancer spheroids and organoids. Specifically, we discuss recent developments that aim to recapitulate cancer cell interactions with the ECM, vasculature, fibroblasts, and immune cells using engineered hydrogel systems.
2. 3D tumor models
Supported by a large number of studies16–19 demonstrating the importance of the third dimension for cancer cell culture, the recent paradigm shift from 2D monolayer to 3D cell culture for improved recapitulation of patient tumor characteristics and drug response has made 3D tumor models increasingly mainstream. An ideal 3D tumor model should mimic the in vivo tumor architecture, composition, and the molecular profile of cancer cells.20 While 2D cultures are based on growing cells adherent on rigid materials such as polystyrene and glass, 3D cultures allow cells to interact with their environment in all three dimensions and typically involve the formation of cellular aggregates.21 In this section, we will briefly describe two main categories of 3D tumor models – models based on spheroids and organoids.
2.1 Tumor spheroid models
Spheroids are typically defined as 3D homo-cellular or hetero-cellular aggregates generated from immortalized cancer cell lines. In the 1970s, Sutherland and colleagues first developed multi-cellular spheroids to recapitulate the in vivo cancer phenotype and evaluate the response of spheroids to radiotherapy.22 Broadly categorized into scaffold-based and scaffold-free technologies, several methods have been developed to allow cells to form 3D spheroids.23 We direct the reader to several excellent reviews on techniques that have been developed to generate 3D spheroid cultures.23–26 Spheroids are generally preferred over 2D monolayer cultures as they better mimic cell–cell and cell–matrix interactions in in vivo tumors. In addition, as spheroids have a well-defined architecture comprising a hypoxic necrotic core surrounded by a layer of normoxic and quiescent cells and an exterior layer of proliferating cells due to established gradients in oxygen levels, nutrients, and other signaling molecules, spheroids are thought to well-represent avascular solid tumors.16 Accordingly, numerous studies have demonstrated that 3D spheroid models better recapitulate the in vivo cancer cell phenotype,27,28 such as the molecular profile of cells undergoing epithelial-to-mesenchymal transition and cancer stem cells,29 as well as in vivo drug response.30 As an example, Riedl and colleagues compared differences in activation of the Akt-mTOR signaling pathway between cancer cells cultured as 2D monolayers or as 3D spheroids. Besides a significant decrease in Akt signaling in 3D spheroids, the authors also reported a reduction in phosphorylated S6K towards the spheroid core, closely resembling tumor regions that surround the vasculature in vivo.14 Expectedly, when treated with Akt pathway inhibitors, 3D spheroids also displayed stark differences in drug response. While spheroid cultures possess numerous advantages over 2D monolayer models, there are a few key limitations. In addition to the poor amenability of spheroids for long-term culture, they also start to lose important genetic features, experience hypoxia, and undergo necrosis over time.27,31 Moreover, spheroids are also less able to recapitulate cancer heterogeneity compared to patient-derived organoid-based models.
2.2 Patient-derived tumor organoid models
Organoids were first reported by the Clevers group in 2007
32 and are rapidly becoming the gold standard approach to model both normal and cancerous tissues in vitro. Organoids are 3D clusters of cells typically generated from stem, progenitor and circulating tumor cells.33 When these cells are grown in an appropriate matrix (most commonly based on basement membrane extracts) in the presence of tissue-specific morphogens and biochemical cues, specific signaling pathways that control stem cell expansion and differentiation34 are activated. By providing specific environmental cues, organoids spontaneously self-assemble and comprise differentiated cell types that exhibit phenotypic and functional features of tissue-specific cells in vivo. Compared to spheroid cultures, organoids better recapitulate the structural and compositional complexity of tissues and organs. For example, gut organoids are able to maintain apical-basal polarity, a characteristic feature of cells in the intestinal epithelium,35 as well as recapitulate the spatial organization of heterogenous tissue-specific cells.36 Accordingly, numerous studies have demonstrated the feasibility of leveraging the organoid technology to generate tissues for regenerative medicine. As an example, Nie and colleagues generated liver organoids from induced pluripotent stem cells and showed that they exhibited hepatic functions and enhanced the survival rate of mice with acute liver failure.37 In a similar study, organoids developed from small intestinal epithelial cells were able to recapitulate unique features of the small intestine such as self-renewal, form villi and differentiate into Paneth cells that only reside in the epithelia of the small intestine.38 In addition, intestinal organoids may provide new insights into novel polygenic signatures causing disease.39
Besides serving as potential novel sources for cell therapy that could pave the way for the next wave in regenerative medicine, organoids have also been established for a plethora of different cancer types40 for modeling of interactions between tumor and its milieu, drug development, and personalized drug testing.40,41 To simulate the pathophysiological development of colorectal cancers, the CRISPR-Cas9 genome editing system has been used on organoid models to establish known genetic mutations found in patient tumors.42,43 Targeting the most commonly mutated genes in colorectal cancer (tumor suppressor genes APC, SMAD4 and TP53, and oncogene KRAS), organoids harboring mutations displayed chromosomal instability, aneuploidy, and displayed invasive behavior when implanted in vivo.44 By modeling the genetic aberrations that lead to malignancy, organoid models enable an increased understanding of genetic changes that occur during tumorigenesis and the development of improved targeted therapies.
In a series of recent studies,45,46 the Clevers group has also demonstrated the feasibility of establishing tumor organoids not by engineering mutations into stem cells, but directly from resected patient-derived tumor tissues or biopsies. To date, an immense collection of patient-derived tumor organoids for long-term cultures have been generated and biobanked, including cancers of the lung,47 breast,48 gastrointestinal tissues,49 and pancreas.50 As these tumor organoid models reflect the molecular profile and inherent heterogeneity in cancer cells found in the corresponding patient,48 these organoids present tremendous opportunities for drug development and personalized drug testing. In a landmark study by Vlachogiannis and colleagues, the authors established gastrointestinal organoid cultures, treated them with drugs used in patients, and obtained 93% specificity and 88% positive predictive values, suggesting that these models may be very useful for personalized drug testing.49 Other groups were able to leverage patient-derived prostate cancer organoids to model rare neurocrine prostate cancer as well as generate prostate epithelial cells without any genetic manipulation.51,52 Although tumor organoid models recapitulate the molecular features and drug response of patient tumors, it is well-known that existing models are limited by the lack of stromal components that make up the TME, such as the vasculature, cancer-associated fibroblasts (CAFs) and immune cells, which collectively influence cancer cell behavior and drug response.34,53 As such, current organoid models are poorly suited for evaluating drugs that rely on the stromal compartment to be effective, such as anti-angiogenic drugs and immune checkpoint inhibitors.34,54,55 Therefore, there is an urgent need to develop more complex organoid-based models which incorporate stromal components and better mimic cancer–stromal interactions.
3. The tumor microenvironment
The TME (Fig. 1) comprises cancer cells and the surrounding stroma. Within the tumor stroma, there are cellular components56 such as fibroblasts, endothelial cells and immune cells, as well as non-cellular components. The latter includes the extracellular matrix and soluble signaling molecules.57 In solid tumors, the microenvironment has several distinct prominent characteristics. For example, the regions surrounding the tumor are hypoxic, have higher interstitial fluid pressure due to leaky blood vessels, and undergo ECM remodeling.58,59 In this section, we will describe features and known cancer–stromal interactions in the TME to highlight the importance of recapitulating these interactions in in vitro tumor models.
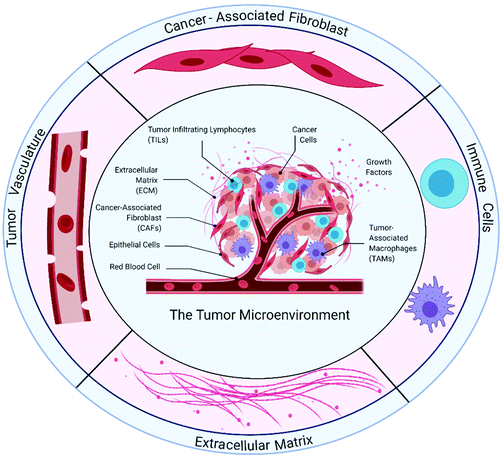 |
| Fig. 1 Components of the tumor microenvironment (TME). The extracellular matrix (ECM) is a 3D dynamic network comprising various macromolecules which provide both structural support and soluble cues (growth factors) to cancer cells. As a result of an imbalance in ECM deposition and degradation, the ECM undergoes remodeling where excessive deposition typically contributes to increased tissue stiffness, leading to tumor progression. Tumor-associated angiogenesis generates dysfunctional and leaky blood vessels. These vessels poorly supply oxygen and nutrients to cells in the TME, creating regions of hypoxia. Leaky blood vessels also contribute to cancer cell metastasis. Cancer-associated fibroblasts are a highly heterogeneous stromal cell population in the TME and contribute to ECM remodeling, epithelial-to-mesenchymal transition and drug resistance. Immune cells such as tumor-infiltrating lymphocytes and tumor-associated macrophages comprise different subtypes that have pro-tumoral or anti-tumoral properties. Immune-targeting approaches in cancer typically involve re-activation of T-cells and manipulation of macrophage polarization. | |
3.1 Tumor extracellular matrix
The tumor ECM acts as a complex and dynamic scaffold consisting of a wide variety of macromolecules, including proteoglycans and fibrous proteins,60–62 adhesion molecules63,64 and stromal cells,65 which collectively modulates cancer cell phenotype and drug response66 through provision of structural support and ligands to cell surface receptors. Unlike normal tissues where there is a tightly regulated balance between ECM deposition and degradation, this fine balance is disrupted in tumors, where the ECM is constantly being remodeled by both cancer and stromal cells.67 ECM dysregulation is commonly seen in fibrotic tissues and cancer, manifested as abnormal ECM deposition and increased tissue stiffness.68,69 While ECM remodeling contributes to normal tissue homeostasis, cancer cells exploit this to form tumor niches and metastasize.70,71 In many cancers, the tumor ECM is highly enriched in certain subsets of collagens, fibronectin, hyaluronan (HA), tenascin, proteoglycans such as perlecan, and others.5,62,72,73 The increased deposition of these ECM components has been shown to support cancer progression and drug resistance by altering cell–cell adhesions, cell–ECM interactions and cell polarity.74–76 For example, in the presence of persistent tissue inflammation, stromal fibroblasts are activated to become myofibroblasts, which become highly synthetic and produce large amounts of collagen type I and other ECM components, resulting in desmoplasia.77 Collagen type I, an abundant ECM component in many cancers, has been shown to promote epithelial-to-mesenchymal transition through activation of ILK and subsequently NF-kB-dependent inactivation of GSK-3β.78 The presence of a dense collagen ECM network has also been shown to inhibit cytotoxic CD8+ T-cell migration into tumors,79 while tumor ECM high in HA facilitates cancer cell invasion and metastasis.80 It has also been shown that the latter is able to shield cancer cells from immunosurveillance as well as hinder the accessibility of antibodies, making cancer cells resistant to monoclonal antibody-derived therapy.81 Additionally, fibronectin has been shown to contribute to poor drug treatment response via cell adhesion-mediated drug resistance82 as well as contribute to the formation of pre-metastatic niches.83 Lastly, the dysregulated degradation of the tumor ECM via matrix metalloproteinases (MMP) and other proteases not only disrupts the function of the ECM as a structural support to maintain cell polarity,84,85 uncontrolled ECM degradation also activates latent secreted growth factors that can support cancer cell survival and invasion.86,87
3.2 Physical properties
As cancer progresses, physical properties of the tumor stroma also evolve in concert with tumor growth and metastasis. Physical properties such as stiffness of the ECM and oxygen levels have an impact on cancer cell signaling pathways,88 cancer heterogeneity,89,90 ECM remodeling,91 the formation of vascular networks,92 as well as the phenotypic state of fibroblasts93 and immune cells.94,95 In breast and colorectal tumors, mechanical stiffness of the tumor itself is often higher than the surrounding normal tissues.96,97 Gradual stiffening of tumor tissues is highly correlated with cancer progression and is caused by the excessive deposition and restructuring of the ECM network. During the early stages of cancer, cancer cells secrete growth factors that inhibit MMPs and reduce ECM degradation resulting in increased tissue stiffness.91 Additionally, growth factors such as transforming growth factor-β (TGF-β) secreted by cancer cells into the TME induces the activation of fibroblasts to CAFs.98,99 In turn, CAFs produces lysyl oxidase which promotes collagen crosslinking95,100 and loss of Caveolin-1 causes structural disorganization of the ECM101 which drives tissue stiffening.
Reciprocally, remodeling of the tumor ECM and alterations in tissue stiffness affect signaling pathways within cancer and stromal cells. Higher tissue stiffness is reported to enhance cancer cell proliferation102–104 and induce phosphatidylinositide 3-kinase activity, promoting cancer cell migration and metastasis.105 The transcription factor YAP/TAZ, a central mediator of the Hippo pathway and sensor of mechanotransduction, is activated by high tissue stiffness and mediates cancer cells to adopt a stem cell-like state, as well as direct normal fibroblasts to adopt and maintain the CAF phenotype.106 Enhanced matrix stiffness also induces CAFs to secrete activin A, activating TGF-β/activin A signaling, and promotes a more invasive and pro-metastatic phenotype in colorectal cancer.107 Besides fibroblasts, stiffening of the tumor stroma also influences immune cells such as macrophages. Macrophages that were cultured on more rigid substrates displayed enhanced migratory behavior and increased production of inflammatory cytokines (e.g. nitric oxide, tumor necrosis factor-α).108 Lastly, tissue stiffness has also been shown to affect drug delivery, where dense ECM decreases the ability of drugs to diffuse through, reducing the access of chemotherapeutics to the tumor core.109
3.3 Tumor-vasculature interactions
When tumor masses increase in size beyond 1–2 mm3, the surrounding blood vessels are typically unable to supply sufficient oxygen and nutrients to support the intensive metabolic demands of the tumor.110,111 To overcome this, the tumor adopts an angiogenic phenotype and promotes the formation of additional blood vessels in the tumor region, contributing to tumor growth and malignancy.112 Characterized by its hierarchical disorganization, aberrant structure and reduced blood flow, the resulting tumor-initiated blood vessels that form are typically structurally and functionally different from the normal vascular network.113,114 Structurally, tumor blood vessels do not have a uniform diameter, exhibit irregular branching and bulge at certain regions.114,115 Due to the aberrant arrangement and inconsistent vessel shape, blood in tumor vessels faces greater resistance, flows through different paths in an uneven manner and may not perfuse all open blood vessels.116,117 Consequently, these poorly functional tumor blood vessels result in poorly perfused tumors and hypoxic regions within the TME.118 Tumor angiogenesis can occur through different mechanisms. While new vascular outgrowth can form from pre-existing ones (sprouting angiogenesis), pre-existing blood vessels can also undergo structural remodeling and split into two finer branches of blood vessels (intussusceptive angiogenesis).112 In these angiogenic processes, various pro-angiogenic factors such as the vascular endothelial growth factor (VEGF), fibroblast growth factor (FGF) and platelet-derived growth factor (PDGF) modulate the development of blood vessels.119 For example, in sprouting angiogenesis, VEGF play a pivotal role in the activation of endothelial cells and induce sprout formation and extension which contributes to vascular formation.120
Besides serving as a conduit to deliver nutrients and oxygen, the tumor vasculature interacts with other components of the TME to create a milieu that supports tumor development. For example, the tumor vasculature mediates the immune landscape of tumors by modulating the migration and activation of immune cells.121,122 Endothelial cells that line blood vessels are known to express E-selectin and P-selectin which enable the adhesion of leukocytes.123 These cell adhesion molecules enable trafficking of leukocytes from the circulating blood flow, facilitating trans-endothelial migration and recruitment of immune cells to the tumor site.121 Dysfunctional vasculature has been shown to impede immune cell infiltration, thereby contributing to immunotherapy resistance in cancer patients.124 On the other hand, immune cells also secrete soluble factors that support angiogenesis and vascular remodeling.125 For instance, in a hypoxic TME, tumor-associated macrophages (TAMs) undergo metabolic changes to exhibit angiogenic characteristics such as the secretion of pro-angiogenic factors, VEGF, epidermal growth factor (EGF) and FGF-2.125 These pro-angiogenic factors promote vascular endothelial cell proliferation, induce angiogenic sprouts, lumen formation and vascular maturation.126 Given the importance of the tumor vasculature in supporting cancer growth and development, anti-angiogenic drugs are now part of standard-of-care treatments for several cancers.127 More recently, combinatorial therapeutic strategies based on vascular normalization has been proposed (such as those aimed at inhibiting Angiopoietin-2 to regain vascular function and enhance the efficacy of immunotherapy.128–130
3.4. Tumor-fibroblast interactions
Tumors are often described as ‘wounds that never heal’131 and numerous studies have shown that fibroblasts play a critical role in regulating this process.132–134 Generally referred to as cancer-associated fibroblasts (CAFs), these stromal cells can make up the majority of the TME in some cancers and are associated with poor prognosis.99,135–137 During normal wound healing, quiescent inactivated fibroblasts differentiate into highly contractile myofibroblasts expressing alpha-smooth muscle actin (α-SMA) to regulate ECM remodeling.138 By modulating Wnt/β-catenin signaling, fibroblasts promote normal tissue renewal, regeneration and homeostasis.139 However, prolonged activation of Wnt signaling can increase the production of stress molecules such as heat shock factor 1 and upregulate pro-inflammatory cytokines (TNF-α and CXCL12) which contribute to tumor progression.140,141 Moreover, systemic autocrine signaling of these factors can convert normal myofibroblasts to CAFs during tumor progression.142
Besides contributing to dysfunctional ECM regulation,143 CAFs have also been shown to support epithelial-to-mesenchymal transition,144 the adoption of cancer stem cell phenotype145,146 and enhance cancer cell survival during drug treatment.144,147,148 Additionally, CAFs are also able to recruit immuno-suppressive cells such as M2 macrophages into the tumor stroma to create an immune-suppressive TME.149,150 For example, Zhang and colleagues151 showed that in colorectal cancer, CAFs are able to polarize monocytes into immune-suppressive M2 macrophages by secreting interleukin (IL)-2/CXCL2, thereby inhibiting natural killer (NK) cell-mediated cancer cell killing. More recently, CAFs were found to recruit programmed cell death protein 1 (PD-1)-expressing TAMs that contribute to immune suppression.152,153 Accordingly, emerging immunotherapies now focus on targeting the CAF-immune cell crosstalk.148
3.5 Tumor-immune interactions
It is now widely recognized that the crosstalk amongst cancer cells, different stromal cell populations and immune cells contribute to tumor growth and progression.149,154,155 Cancer cells are able to subvert the innate anti-tumor immune mechanisms and exploit immune cells to support cancer growth.156–158 Since the 19th century, it was hypothesized that cancer develops due to chronic inflammation159 and it is now well-established that tumor-associated inflammation contributes to cancer progression.160,161 Studies have shown that immune cells such as TAMs are able to infiltrate into the TME and mediate the production of a range of chemo-attractants and cytokines to modulate the progression of cancer.158,162 Broadly categorized into M1-like and M2-like macrophages, TAMs are known to exhibit considerable plasticity in state depending on the cellular environment163,164 and undergo polarization as tumor progresses. Functionally, pro-inflammatory molecules like IL-6 produced by TAMs165 and stromal cells166–168 are able to trigger the JAK/STAT3 signaling pathway in cancer cells through a feed-forward autocrine fashion, thereby promoting tumor progression and metastasis.169,170 However, IL-6 is also able to recruit cytotoxic T cells (CD8+) into the TME.171 As such, TAMs are deemed a double-edge sword in cancer and the increasing number of studies looking to elucidate TAM heterogeneity may shed light into this potentially targetable stromal cell population.172,173
Another important subset of tumor-associated immune cells are the tumor infiltrating lymphocytes (TILs). These cells include CD4+ T helper cells and CD8+ cytotoxic T cells that are critical components of the adaptive immune response and play an important role in tumor progression. However, due to the presence of dysregulated suppressive signals in some TME, T cells often become terminally exhausted.156,174 With the goal of mitigating T cell anergy, immune checkpoint inhibitors were developed and are now widely investigated and used as standard-of-care treatment for some cancers.175,176 PD-1 on myeloid-derived cells and cytotoxic T lymphocyte associated protein-4 (CTLA-4) bind to programmed death-ligand 1 (PD-L1) and CD86 respectively, to dampen immune response. Intriguingly, cancer cells are able to express the ligands PD-L1 and/or CD86 to mount an anti-inflammatory response in the TME.177,178 By introducing neutralizing antibodies against these immune checkpoints, the immune checkpoint ligands are unable to bind to their receptors, thereby eliciting an immune response.179,180 As other stromal cell types can also influence the recruitment of immune cells to the tumor154,181 and express these immune checkpoint molecules,182 understanding the cancer–stromal-immune axis in the TME is now of paramount importance to develop strategies to overcome resistance to immune checkpoint blockade.183
In sum, the complex bi-directional communication between cancer cells and the TME plays a significant role in influencing mechano-biological signaling pathways, collectively contributing to tumor development184,185 and treatment.186,187 Therefore, it is critical to reconstruct models that are able to recapitulate such intricate relationships between various components of the tumor.188,189
4. Hydrogel systems to model cancer interactions with the TME
With the growing consensus that elements of the TME need to be incorporated into in vitro tumor models, some investigators have adopted the approach of generating simple, scaffold-less heterospheroids comprising cancer and stromal cells,190–196 while some have also leveraged the use of biomaterials to model cancer-TME interactions.197–200 The use of scaffolds, including hydrogels, enables the recapitulation of certain biophysical and biochemical aspects of the tumor ECM to support cancer and stromal cell culture. We direct the reader to several excellent review articles on the use of scaffolds for tumor engineering,201–207 and focus specifically on describing the use of hydrogels to model the TME in vitro (Fig. 2) in this section of the review. Hydrogels are highly hydrophilic networks of polymers cross-linked either by physical or chemical means, ideal for mimicking the ECM of tissues and organs. Depending on the polymer chemistry, they can be manipulated or engineered to have a range of biochemical and biophysical properties with adequate porosity for diffusion and nutrient transfer to represent the ECM composition and mechanical properties of specific tissue types.208,209 Hydrogels can be classified into natural, synthetic or hybrid depending on the origin of the polymer used for hydrogel fabrication. Recent developments in materials engineering have resulted in the generation of hydrogels with tunable biophysical, biochemical and biological properties which recapitulate the in vivo tissue microenvironment with fidelity.210
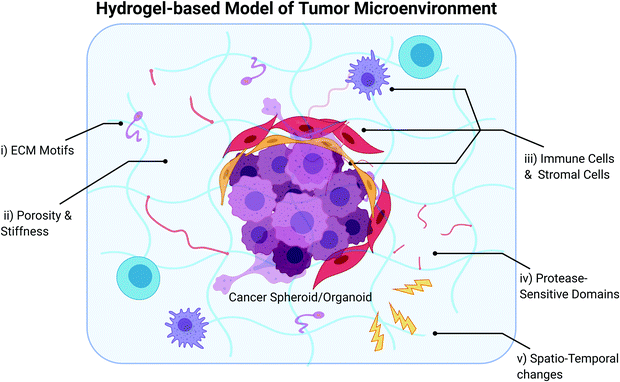 |
| Fig. 2 Hydrogel-based model of the tumor microenvironment (TME). An ideal model should consider the incorporation of (i) ECM motifs present in the tumor ECM, (ii) tumor-matched porosity and stiffness, (iii) various stromal components, (iv) protease-sensitive domains and (v) enable spatio-temporal changes that occur with tumor progression. (i) ECM motifs enable recapitulation of the tumor ECM that can influence drug resistance and metastasis. For example, integrin-binding peptides (RGD) are typically used to mimic the adhesive domains found in ECM macromolecules such as fibronectin and mediate cell attachment in the hydrogel matrix. (ii) Porosity and stiffness of the hydrogel can be fine-tuned to recapitulate biophysical properties of the tumor ECM which influence cell migration and invasion. (iii) Many different stromal cell populations co-exist with cancer cells in the TME. Hydrogel systems with tunable biochemical and biophysical properties may enable expansion of patient-derived stromal cells and maintenance of phenotype. (iv) Protease-sensitive domains such as matrix metalloproteinase-degradable peptide sequences typically used to crosslink polymer networks forming hydrogels enable dynamic cell-mediated matrix remodeling. (v) Hydrogels which enable stimuli-triggered changes in biochemical and biophysical properties can enable the study of spatio-temporal changes that occur in tumors with progression. | |
4.1 Types of hydrogels
Natural hydrogels are generally protein- or polysaccharide-based materials derived from biological origins such as collagen, fibrin, alginate, and basement membrane extract.211–216 Even up till today, they remain popular matrices for growing cancer and stromal cells given their inherent bioactivity, biocompatibility and innately represent components of the in vivo ECM. Indeed, many studies have demonstrated how basement membrane extracts support organoid culture of a wide variety of cancers217–220 while collagen and fibrin gels are typically used to culture CAFs,221,222 endothelial223,224 and immune cells.225 Hydrogels fabricated from basement membrane extracts such as Matrigel® and Geltrex™ are one of the most widely used natural hydrogels in tumor engineering and are derived from Engelbreth-Holm-Swarm (EHS) mouse sarcoma cells.226 These matrices are mainly used to support epithelial cell culture as they contain laminin-1, type IV collagen, heparan sulfate proteoglycan, nidogen, and key growth factors.227 Many recent in vitro studies have shown that basement membrane extract matrices support cell morphogenesis and differentiation of cell lines, primary cells and tissue explants.228–232 Collagen-based hydrogels are fabricated using extracts of animal or human tissue, and are typically used in the form of gels, sponge or hollow fiber tubings for 3D cell culture.233 A wide range of physical (UV or gamma irradiation) and chemical (introduced by functional groups or activation of carboxylic acid groups) crosslinking methods have been developed to modify the biocompatibility and biodegradability of collagen gels.234 As a result of its protein-rich composition and fibrous structure, collagen gels have been shown to promote vascular morphogenesis235 and enable the study of immune cell trafficking within the TME.225,236 Fibrin is another type of biologically-derived matrix that is formed following the polymerization of fibrinogen alpha-chains with thrombin.237 This creates a clot-like network with nano- and micro-scale features and viscoelastic properties that mimic the in vivo ECM.238 Taken together, numerous studies have demonstrated the utility of natural hydrogels to mimic the 3D tissue microenvironment for both tissue and tumor engineering.211,213,217,239 However, these matrices present a few key limitations, including a narrow range of mechanical properties as well as changes in matrix properties, such as collagen gel contraction,240 leading to short-term studies.239 Lastly, there may be limited reproducibility of experiments as a result of batch-to-batch variations as well as undesired immunogenic response as they are typically derived from animal tissues.241–243
To better control the bioactive signals presented to cultured cells as well as reduce the variation in material properties of natural hydrogels, synthetic hydrogels such as those based on poly(ethylene glycol) (PEG) are an attractive alternative for tissue and tumor engineering.244,245 PEG-based hydrogels are highly hydrophilic, mechanically stable over time, and permeable polymeric networks that facilitate the diffusion of gas and nutrients.246–248 Importantly, these hydrogels remain relatively inert with low protein adsorption, hence a wide range of bioactive moieties (proteins, peptides and drugs) or biopolymers (HA, collagen and fibrin) can be conjugated to PEG to mimic the in vivo ECM with controlled and tunable bioactivity.249–251 By modulating the polymer concentration or extent of crosslinking,252,253 synthetic hydrogels also enable tuning of physical properties such as stiffness to match that of the tissue ECM of interest.254–256 More recently, hybrid hydrogels which are composites of natural and synthetic polymers have been developed. Hybrid hydrogels merge the advantages of both polymer types in one system, that is, the presence of ligands for cell adhesion and filamentous nature of natural polymers and user-defined control of synthetic polymers, to exhibit different biochemical and physical properties.257–259 As an example, HA-based hydrogels have been shown to be highly tunable and conducive for cancer cell culture.260–262 HA is a non-sulfated glycosaminoglycan composed of repeating glucuronate disaccharides and N-acetyl glucosamine263 and is an integral part of the in vivo tumor ECM and synovial fluid.264 The different functional groups (acid and hydroxyl groups) on the HA backbone allow different crosslinking chemistries including hydrazide modifications, non-covalent reactions, crosslinking with polyfunctional epoxide, glutaraldehyde (GTA) and carbodiimides.264,265 We have previously shown how HA can be modified with integrin-binding peptides and MMP-degradable domains to support mesenchymal cell attachment and matrix remodeling to recapitulate the prostatic bone metastasis TME.266 In the next few sections, we will discuss how hydrogels have been employed to model the tumor ECM and recapitulate interactions between cancer cells and the tumor ECM, vasculature, fibroblasts, and immune cells.
4.2 Hydrogels mimicking the extracellular matrix
Basement membrane extracts such as Matrigel® are widely used as the gold standard matrix for organoid culture due to its tissue ECM-like biochemical and biophysical properties. Besides basement membrane extracts, hydrogels fabricated from decellularized tissues were recently explored as an alternative to provide more tissue-specific biochemical cues for organoid culture.267,268 Using decellularized mouse and human mammary tissues as biomaterials to fabricate a self-gelling hydrogel system that contains growth factors unique to the mammary gland, Mollica and colleagues bioprinted mammary normal epithelial and tumor organoids and demonstrated that the tissue-specific ECM hydrogels were capable of supporting organoid proliferation, comparable to the use of rat tail collagen or basement membrane extracts. Furthermore, the authors showed that the mammary ECM-based hydrogels elicited unique gene expression profiles in the cultured organoids compared to those grown in basement membrane extracts, likely due to differences in structure and growth factor composition, highlighting the importance of incorporating tissue-specific biochemical cues to support organoid culture.269 Taking a different approach, Broguiere and colleagues developed the first well-defined 3D matrix that allows for long-term expansion of organoids comprising both progenitor and differentiated cells. In seeking to develop a well-defined hydrogel system alternative to hydrogels based on basement membrane extracts, the authors not only identified soft fibrin scaffolds as a suitable physical substitute, they also found that the presence of naturally occurring adhesive Arg-Gly-Asp (RGD) peptide sequences on the scaffold, as well as laminin-111, are critical for robust organoid formation and expansion. Functionally, no significant differences in drug response were observed in pancreatic cancer organoids cultured in basement membrane extracts, fibrin/basement membrane extracts or the fibrin/laminin hydrogel, suggesting that the fibrin/laminin hydrogel system may be amenable for the culture of epithelial cancer organoids for drug screening applications.270
While biologically-derived matrices provide innate cues, these systems typically confer poor tunability in material properties and architecture as well as low reproducibility of experimental results due to batch-to-batch variations.218 As such, synthetic biomaterials are actively being explored to fabricate hydrogel systems for organoid culture. In a landmark study, Gjorevski and colleagues used enzymatically crosslinked PEG hydrogels to determine the critical ECM components that dictate intestinal stem cell expansion and organoid formation. Interestingly, the authors found that different stages of the organoid formation process necessitated different cellular microenvironments. For example, while a non-degradable and stiff matrix that enabled fibronectin-mediated adhesion was required for stem cell expansion, a soft matrix containing laminin-111 was needed for organoid formation.271 Although the authors only showed the amenability of this system for the culture of normal and cancerous colorectal organoids, the modular nature of this system opens up the possibility of identifying and customizing unique organoid-supporting conditions for different tissues using a tunable and minimalistic platform. Indeed, in a subsequent study, these same PEG hydrogels were shown to also support the culture of hepatic progenitors and organoids, where integrin-mediated adhesion was again shown to be critical for the expansion of liver progenitors and organoid growth.272 In another study to create a well-defined substitute to the use of basement membrane extracts for organoid culture, Bergenheim and colleagues used QGel CN99 (a fully defined PEG-based hydrogel) to culture human colonic organoids derived from biopsy tissue. Organoids in QGel CN99 showed similar cell growth, differentiation, organoid forming efficiency and gene expression profiles when compared to those grown in Matrigel® and could be cultured for at least 6 passages.273 Rather than using fully synthetic hydrogels to recapitulate the in vivo ECM to support organoid culture in vitro, Ng and colleagues leveraged enzymatically crosslinked hydrogels consisting of functionalized natural biomaterials, gelatin-phenol and HA-phenol, that allow for independent alterations in mechanical properties and gelation rate. The authors found that gelatin-phenol hydrogels better supported organoid survival of colorectal cancer patient-derived xenograft cells as compared to HA-phenol or composite hydrogels, suggesting the importance of integrin-mediated adhesion for organoid survival. Additionally, the authors identified hypoxia as an important factor for improving organoid viability, and organoids cultured in gelatin-phenol hydrogels maintained the histological and mutational features of the corresponding in vivo tumor.274 Given the prevalence of HA in the colorectal cancer TME275,276 and that different molecular weight HA can interact differently with HA receptors such as CD44 and RHAMM, further work is necessary to determine if HA truly is dispensable for organoid formation and survival.
In recent years, there has been an increased interest in polyisocyanide-based hydrogels for 3D cell culture due its stress-induced stiffening properties, collagen-like helical architecture and thermosensitive properties which allow for facile recovery of encapsulated cells.277–279 Zhang et al. developed a polyisocyanide-based hydrogel decorated with the adhesive RGD peptide to form mammary gland organoids. Not only did the hydrogels enable mammary gland organoid formation, the resulting organoids could be maintained for prolonged durations in vitro and retain their ability to branch. By varying the density of RGD presented in the hydrogel, the authors found that an increased engagement of integrins that bind to RGD may result in downstream intracellular signaling that favors differentiation into the basal cell lineage.280 Although no cancer organoids were cultured in this system, it would be interesting to observe whether similar preferential selection of basal cell progenitors also occurs in the presence of enhanced integrin-mediated signaling via RGD, which may make this system useful for studying the basal subtype of breast cancer.281 In another similar study, Ye and colleagues formed human liver organoids using a polyisocyanopeptide hydrogel with laminin-111. Unlike previous studies, RGD alone was not sufficient to support organoid growth. Rather, the authors found that laminin-111 was necessary to support organoid proliferation and formation similar to Matrigel®.282 The importance of laminin-111 on organoid growth was highlighted again in a study by Brandenberg and colleagues, who engineered PEG-based hydrogel microcavity arrays to improve the heterogeneity of generated organoids. While organoids could be grown in microcavity arrays with laminin-111 on the surface, poor cell survival was observed in cultures with collagen type IV alone. Importantly, this system enabled high content phenotypic screening of 80 anti-cancer drugs in colorectal cancer organoids. However, it remains to be seen whether the cultured organoids recapitulate the molecular features and drug response of the original tumors from which they were derived.283
Taken together, in recent years, efforts have been made to develop in vitro conditions to culture organoids by recapitulating certain aspects of the in vivo ECM. While most of these studies have focused on colorectal cancer, it is expected that this approach of modularly investigating the role of different ECM components on organoid growth would be extrapolated to other cancers. Lastly, rather than just focusing on cancer cells, much more work needs to be placed on understanding the effect of the tumor ECM on stromal cells, especially CAFs and immune cells.
4.3 Mimicking physical properties of the TME
Increased tissue stiffness is characteristic of solid tumors and is typically because of an increase in the amount of tumor ECM. Increased matrix stiffness can enhance cell–ECM interaction and promote cancer cell invasiveness,284 while supporting CAF differentiation.285 Engineered hydrogels with tunable mechanical properties enable recapitulation of in vivo tissue stiffness and elucidation of downstream effects on cellular state in both cancer and stromal cells. Using PEG-diacrylate hydrogels, Jabbari and colleagues showed that stiffness of the cancer stem cell niche may be unique for different cancers as the optimum hydrogel stiffness was found to be 5 kPa for breast cancer cells, 25 kPa for gastrointestinal cancer cells and 50 kPa for osteosarcoma cells,286 suggesting the need to customize matrix stiffness for tumor model development in a tissue-specific manner. To develop a hydrogel system that accommodates the culture of sensitive cells, Macdougall and colleagues developed PEG-based hydrogels which made use of nucleophilic thiol–yne chemistry to enable the formation of hydrogels without the need for any external catalyst or free radicals. These hydrogels can be tuned to exhibit a stiffness range of 4–10 kPa and modified to present ECM components of interest. While the authors did not demonstrate the effect of stiffness, the gentle chemistry behind this hydrogel system makes this system potentially very useful for culturing sensitive cells such as some patient-derived primary cells.287 Rather than investigating the effect of stiffness in a static manner, in recent years there has been an increased interest in developing dynamic enzyme-mediated in situ stiffening hydrogels. In one example, Tirella and colleagues reported the development of PEG-based lysyl oxidase-responsive hydrogels with initial stiffness range of 0.5–4 kPa which can be further tuned by 0.5 kPa using lysyl oxidase.288 In another example, Liu and colleagues developed tyrosinase-mediated PEG-peptide hydrogels with initial stiffnesses of 0.8, 1.8 and 2.8 kPa where tyrosinase treatment increased hydrogel stiffness to 1.44 kPa, 2.34 kPa and 3.08 kPa, respectively.289 Taken together, these dynamic hydrogels may enable the study of pathological tissue stiffening during tumorigenesis. However, in order to better mimic tissue stiffening as observed in vivo, these hydrogel systems may need to be modified to enable greater induced differences in stiffness. Besides focusing on elucidating the effect of tissue stiffness on cancer cells alone, it is also critical to understand how increased tissue stiffness influences cancer–stromal interactions given that cancer cells are only one part of the whole tumor which comprises stromal cells. Indeed, there is increasing evidence to suggest a positive relationship between normal tissue stiffening and cancer occurrence and progression.290,291 In order to understand the relationship between ECM stiffness and breast cancer cell-adipocyte interactions, Yue and colleagues modulated the extent of methacrylated-gelatin crosslinking and showed that adipogenesis was influenced in a stiffness-dependent manner in the presence of tumor spheroids. While cancer cells inhibited adipogenesis at high stiffness levels within the range of breast cancer, the inhibition effect was lower in low stiffness tissue constructs within the range of normal breast tissue.292 It would be interesting to leverage this same approach to investigate whether stiffness also influences the interaction of cancer cells with other stromal cell types like mechano-sensitive macrophages.
Apart from stiffness as an important biophysical parameter, the physical structure of the ECM also plays an important role in cancer progression. It is well-established that cancer cells metastasize to distant sites using the circulatory system, lymphatic system or through the peritoneal cavity.293,294 During this migration and invasion process, cancer cells travel through different tissue ECM by squeezing through micro-pores larger than 3 μm or by inducing protease-mediated matrix degradation which is dependent on the stiffness and architecture of the ECM.295–297 In recent years, much effort has been made to elucidate the effect of matrix stiffness using hydrogels on cancer cell invasiveness.298–300 However, very little attention has been paid on the role of micro-structure and pore features to better mimic the physical parameters governing cancer cell invasion. In a recent study, Liu and colleagues designed collagen- and alginate-based hydrogels with defined micro-structure, tunable mechanical stiffness and permeability. By varying the degree of calcium-mediated crosslinking, stiffness of the collagen-alginate hydrogels could be varied between 1.2 kPa and 10 kPa. Additionally, with increase in the degree of calcium-mediated crosslinking, pore geometry changed from round to flat. Using the collagen-alginate hydrogel formulation with the largest pore micro-structure (median pore size of 95 μm by volume and 93% in porosity), the authors then demonstrated how these hybrid hydrogels with large pore size better supported mammary fibroblast migration as compared to standard collagen gels and that these fibroblasts led the way for cancer cell invasion.301 This study suggests the importance of supporting both protease-independent motility as well as protease-dependent matrix degradation in hydrogel systems for the study of both cancer and stromal cell migration. The authors then introduced polyhedral oligomeric silsesquioxanes (POSS) nano-molecules into the collagen-alginate hydrogel to induce different gelling mechanisms and showed the feasibility of decoupling matrix stiffness from pore architecture. Cultured mammary fibroblasts remodeled the collagen network differently depending on the matrix micro-structure. Interestingly, the remodeled collagen matrix was thicker and aligned perpendicular to spheroids, and facilitated the invasion of cancer cells.302 Collectively, these studies underscore the importance of the hydrogel micro-structure in mediating fibroblast-initiated cancer cell migration and invasion. In another interesting study, Wisdom and colleagues asked if cells could still migrate through a protease-independent manner if the ECM pore size were too small. By designing interpenetrating network hydrogels comprising reconstituted basement and alginate that exhibit different degrees of plasticity while having the same stiffness and ligand density, the authors demonstrated that in hydrogels with high plasticity, cancer cells extend invadopodia protrusions to mechanically pry open micro-scale channels and migrate through them.303 Again, this study highlights another important biophysical aspect of the ECM – plasticity – that engineered hydrogels could be designed with to recapitulate in in vitro tumor models.
4.4 Recapitulating the cancer–vasculature interaction
Leveraging on previous work that showed how starPEG-heparin hydrogels can support the co-culture of liver cancer cells with human umbilical endothelial vein endothelial cells (HUVECs),304 Brey and colleagues developed a complex co-culture system comprising breast or prostate cancer cells, HUVECs and mesenchymal stromal cells. This hydrogel system is decorated with RGD to enable integrin-mediated cell attachment, crosslinked with MMP-sensitive domains to enable cell-mediated matrix remodeling, and contains heparin which pro-angiogenic growth factors have an affinity to and can be delivered to encapsulated cells. The resulting co-cultures established using the starPEG-heparin hydrogels exhibited more ordered development of tumor angiogenesis and vascular recruitment where tumors proliferated while in contact with HUVECs as compared to Matrigel®. Furthermore, treating the tri-culture with angiogenesis inhibitors led to a decrease in tumor angiogenesis.305 Taubenberger and colleagues further modified the above PEG-heparin hydrogels by using collagen type I- and laminin-111-derived peptides to provide additional biochemical cues for the culture of breast (MCF-7) and prostate (PC-3, LNCaP) cancer cells. Besides enhancing the growth of PC-3 prostate cancer cells, the collagen type I- and laminin-111-derived peptides also increased cancer-HUVEC interactions over hydrogels with RGD.306 Using a photo-polymerizable methacrylamide-functionalized gelatin hydrogel system, Ngo and colleagues developed a model of the perivascular niche in glioblastoma. Given the importance of HA in the glioblastoma TME, the authors first investigated the effect of matrix-immobilized HA on endothelial network formation and found that HA reduced the complexity of endothelial cell networks comprising HUVECs and fibroblasts. Interestingly, in the absence of glioblastoma cells, endothelial cell networks could persist over two weeks in culture; however in the presence of glioblastoma cells, network regression occurred over time, potentially mimicking vessel co-option and regression observed in the in vivo glioblastoma TME.307 In a follow-up study, the authors used RNA-sequencing to elucidate temporal transcriptomic changes that accompany the development and dissolution of endothelial cell networks in the presence of glioblastoma cells and showed that the co-culture of glioblastoma cells with endothelial cell network indeed recapitulated vessel co-option and regression in vivo.308 Taking a microfluidics-based approach to engineer organotypic models of glioblastoma, Cui and colleagues showed using an integrin (αvβ3)-specific collagen hydrogel that perivascular macrophage-endothelial interactions mediate in vitro proangiogenic activity through integrin (αvβ3) receptors and Src-PI3K-YAP signaling. Importantly, the authors demonstrated the potential of inhibiting both αvβ3 integrin and TGFβ-R1 for blocking tumor vascularization in the glioblastoma TME as the dual inhibition arrested pro-angiogenic endothelial–macrophage interactions in the in vitro model.309 Lastly, in seeking to spatially control the location of cancer cells with respect to the vasculature, Roudsari and colleagues engineered a PEG-based two-layer hydrogel with cancer and vasculature layers. Interestingly, large invasive cancer clusters were mainly located at the interface region with the adjacent vasculature, supporting the importance of vascular cells in tumor progression.310
4.5 Recapitulating the cancer-fibroblast interaction
CAFs are a critical component of the TME with wide-ranging functions, including ECM remodeling, reciprocal interactions with cancer cells to influence cell survival, invasiveness and drug resistance, as well as immunosuppressive effects on other stromal cells such as infiltrating leukocytes.311 Yet, much remains to be understood about this highly heterogeneous stromal cell population. While normal fibroblasts and some CAF subpopulations can inhibit cancer growth and invasiveness, CAFs generally have the opposite effect of supporting tumor progression in the TME.312 Given that CAF state and associated properties may be a function of environmental cues, it is important to elucidate how changes in the context influence CAF behavior so that cancer–CAF interactions can be accurately recapitulated. To mimic the breast cancer stromal niche, Cao et al. developed a mechanically-tunable collagen and alginate interpenetrating network hydrogel (collagen-alginate-IPN) by modulating the crosslinking of either or both polymers. Interestingly, in the softer hydrogel with only collagen crosslinked, CAFs adopted a spread morphology with activated mechanosensitive YAP and increased expression of α-SMA and fibroblast activation protein as compared to the stiffer matrix with both collagen and alginate crosslinked. As a result of this phenotypic shift, CAFs in the softer matrix also exhibited changes in secretory factors which increased cancer invasiveness over those in the stiffer matrix, suggesting the importance of understanding how matrix mechanical properties influence CAF state both in vivo and in vitro.313 On the contrary, using orthogonally crosslinked PEG-peptide thiol-norbornene hydrogels susceptible to tyrosinase-mediated in situ gel stiffening, Liu and colleagues showed that stiffened matrices enhanced the expression of α-SMA in pancreatic stellate cells, precursors to pancreatic CAFs.289 These opposing findings highlight the need to carefully dissect and understand the biochemical and mechanical cues that influence CAF activation and phenotypes.
To study cancer–CAF interactions, different systems have been developed including spheroids,314 microfluidic systems,315 hydrogel plugs comprising collagen,316 stacked paper-based co-cultures317 and bioprinted models.318 Seeking to elucidate the effect of stromal fibroblast paracrine signaling on cancer cell invasiveness, McLane and colleagues engineered a model of tumor in situ by coating tumor spheroids with basement membrane and further encapsulating the spheroids within a collagen type I matrix of in vivo stiffness with or without fibroblasts. Using PEG-based polymers to crosslink collagen type I, the authors found that while increased stromal stiffness increased cancer invasiveness in the absence of fibroblasts, this effect was diminished when fibroblasts were incorporated. Mechanistically, it was found that stiffness-activated fibroblasts inhibited cancer invasiveness through osteoprotegerin-mediated paracrine signaling.319 While these two studies appear to suggest that increased stiffness promotes the tumor-restraining CAF phenotype, much more work needs to be done to deeply characterize the molecular profile and functions of CAFs under study as these results are contrary to what we understand of the relationship between CAF biology and matrix mechanics.285 Stack paper-based co-culture systems enable easy retrieval of co-cultured cells for downstream analysis and spatially mapping the location of both cancer and CAFs.317,320 In these models, cells are encapsulated in hydrogel matrices such as collagen, and infiltrated into thin cellulose scaffold layers which are then stacked or rolled. Akin to a roll of paper, the McGuigan group developed the tumor roll for analysis of cellular environment and response (TRACER) model317 and showed how the platform could be used to establish robust co-cultures of head and neck cancer cells with CAFs.321 By using non-adhesive agarose as a barrier between the cancer and CAFs to prevent cell–cell contact, the TRACER system can be modified to enable the study of paracrine interactions only. Using this model, CAFs were found to increase the invasiveness of the cancer cells through paracrine signaling. In a subsequent study, the authors also developed another system, gels for live analysis of compartmentalized environments (GLAnCE), that enables easy imaging and characterization of spatio-temporal interactions that occur at the tumor-stroma interface.322 In another approach to model the cancer–CAF interface, Truong and colleagues developed a microfluidic platform that can spatially organize hydrogel matrices with different ECM and cell compositions and enable the study of cancer invasion in real-time at the single-cell level.315 While these engineered platforms greatly enable the improved characterization and manipulation of cancer–CAF interactions, rather than relying on collagen or basement membrane extract gels, further efforts need to be placed on integrating biochemically and mechanically tunable hydrogel systems into these engineered platforms to investigate how different matrix properties influence cancer and CAF behavior. Lastly, given our increasing understanding of CAF heterogeneity and their sensitivity to environmental context, it would be critical to deeply characterize the phenotype of CAFs used in these studies.
Leading the front on CAF heterogeneity, the Tuveson group recently demonstrated the existence of two CAF subpopulations using co-culture models comprising pancreatic cancer organoids with pancreatic stellate cells in Matrigel®. Of notable mention, as reported in this study (and our own study323), the integration of CAFs into organoid-based models requires significant optimization, as several factors present in organoid media, including Noggin, B27 and TGF-β inhibitor, can significantly inhibit fibroblast growth.324 Leveraging this developed co-culture model, the authors showed that two distinct CAF subpopulations exist, including a myofibroblastic-type adjacent to the cancer organoids, and a more inflammatory-type further away. These spatially segregated different CAF subpopulations were validated in many other subsequent studies and highlight the importance of the tumor architecture in tumor modeling. Accordingly, 3D bioprinting may be a very useful tool to recreate the tumor architecture. Using sodium alginate-gelatin hydrogels, Mondal and colleagues demonstrated the feasibility of printing non-small cell lung cancer patient-derived xenograft cells together with lung CAFs.318 While the authors demonstrated printability of the biomaterial and that cell viability was supported in the 3D bioprinted co-culture, subsequent studies are needed to deeply characterize the cancer–CAF crosstalk as well as create spatial architecture in the model. Lastly, efforts are also been made to investigate the effect of CAFs on other stromal cell types in the TME. For example, Koch and colleagues used starPEG-heparin hydrogels to generate tri-cultures comprising mammary epithelial, endothelial and fibroblast cells to investigate the effect of CAFs on endothelial network formation. As compared to normal fibroblasts, CAFs induced the formation of denser endothelial cell networks which had increased number of vessels and branching points.325 While further work could have been done to profile the molecular changes that occur in the cultured cells to validate these findings, this study highlights the importance of recapitulating stromal–stromal interactions especially with CAFs, which have nodal interactions in the TME.
4.6 Recapitulating tumor-immune interactions
TAMs are inherently highly plastic in nature, adopting different states in response to ECM composition, stiffness and topology, cellular interactions, amongst other environmental cues.326 As a result of education within the TME, monocytes polarize into TAMs that typically are M2-like and have a pro-tumoral role in advanced cancers, supporting cancer growth and invasiveness, angiogenesis and immunosuppression.327,328 To understand the effect of integrin engagement on macrophage polarization, Cha and colleagues used gelatin methacryloyl (GelMA) and PEG-diacrylate hydrogels encapsulating human monocytic cells and showed that while the bioactive GelMA hydrogel changed cell shape, the bioinert PEG-diacrylate hydrogel did not. Accordingly, in the presence of IL-4, GelMA induced the generation of M2-like macrophages in contrast to PEG-diacrylate hydrogels, which induced M1-like macrophages. Mechanistically, differences in integrin expression were observed in macrophages cultured in the two hydrogel systems; specifically, GelMA hydrogels induced M2-like macrophage polarization through integrin α2β1 attachment, potentially via STAT6 activation.329 In a more recent study, Court and colleagues made use of 3D collagen type I hydrogels and showed that the hydrogel differentially modulated human macrophage polarization as compared to 2D culture.330 Collectively, these studies highlight the tight interplay between matrix cues and macrophage phenotype, mandating careful attention to how macrophages are cultured in vitro for tumor modeling. In this regard, tunable hydrogel systems enable investigations into how biochemical and mechanical cues in the TME influence macrophage polarization and its interactions with cancer and stromal cells.
Besides matrix cues, cell–cell proximity can also influence macrophage polarization. Tevis and colleagues compared two cancer–TAM spheroid models using collagen hydrogels, where macrophages were incorporated either through diffuse encapsulation within the cancer spheroid-containing collagen hydrogel or within the cancer spheroid itself forming a heterospheroid. In the former, the diffuse encapsulation of TAMs in the surrounding collagen hydrogel mimics TAMs in the stroma while in the latter model, the direct cell–cell contact between TAMs and cancer cells in the heterospheroid mimics TAMs in the tumor core. Interestingly, oxygen consumption and production of EGF was greater in the heterospheroid configuration compared to the model where TAMs were diffusely encapsulated. By measuring IL-10, it was found that the heterospheroid configuration enhanced the polarization of macrophages towards a M2-like state, highlighting the importance of close cancer-macrophage proximity on generation of TAMs.331 Leveraging this finding, Huang developed a hydrogel system which enables facile recovery of encapsulated cells. In this system, hydrogels are fabricated from inducing host–guest interactions between aromatic groups on gelatin and modified photocrosslinkable β-cyclodextrins, and this crosslinking can be disrupted using another guest monomer that competes for β-cyclodextrins. In seeking to understand the behavior of reprogrammed TAMs, endometrial cancer-macrophage heterospheroids were encapsulated in the hydrogel and following the application of interferon-γ, the authors showed that there was a reduction in pro-angiogenic VEGF, cancer cell migration and tumor growth when compared to M2-like macrophages co-cultured with cancer cells.332
Within the TME, the macrophage-CAF crosstalk contributes to tumor progression. For example, it was recently shown that the complement component C3 is specifically expressed by the ‘immune’ CAF subpopulation and the resulting cleaved product (C3a) recruits C3aR+ macrophages, creating an immunosuppressive TME.333 To understand the macrophage-fibroblast crosstalk in prostatic bone metastasis, Costa and colleagues developed a perlecan-modified collagen type I and HA-based hydrogel that supported the tri-culture of prostate cancer cells, macrophages and stromal fibroblasts. Perlecan was used to sequester and present Wnt3a in the engineered model. Using this tri-culture, M2-like macrophages were found to increase levels of SULF1 and HSPG2 in fibroblasts, and that SULF1 can mitigate Wnt3a-driven growth signals.334 In another example, Rebelo and colleagues developed an alginate-based tri-culture of lung cancer cells, CAFs and monocytes and demonstrated that the model creates an immunosuppressive and invasive environment that activated monocytes towards TAMs. It should be noted that the TAM-like phenotype was observed only when all three cell types were in the tri-culture. The authors also demonstrated that the M2-like phenotype could be modulated through the CSF1R inhibitor BLZ945, resulting in a decrease in the M2-like macrophages.335 Lastly, to elucidate the role of macrophages in the glioblastoma TME, Tang and colleagues used a 3D bioprinting system to develop a complex tetra-culture of glioblastoma stem cells, macrophages, astrocytes, and neural stem cells, in a hydrogel comprising GelMA and glycidyl methacrylate-HA (GMHA) hydrogels. In doing so, the model represented aspects of the normal brain, immune components, stromal components, and important biochemical cues from the extracellular matrix (HA). The authors found that the tetra-culture better recapitulated transcriptomic signatures found in glioblastoma tissues as compared to standard sphere cultures, and that macrophages upregulated immune activation signatures, exhibited increased M2 polarization, and promoted glioblastoma stem cell invasion.333 As one of the most complex models established, this 3D bioprinted model enables recapitulation and interrogation of cellular crosstalk in the TME to identify novel functional dependencies and therapeutic targets.
In the past decade, the introduction of T-cell targeting immunomodulators blocking the immune checkpoints CTLA-4 and PD-1 or PD-L1 has revived the use of immunotherapy to treat cancer patients.336 While some patients can respond remarkably well to immune checkpoint blockade, it is still challenging to predict whether an individual patient would be sensitive to immunotherapy.337In vitro models that simulate T cell-mediated tumor recognition on an individual patient level may be very useful for predicting the efficacy of immune checkpoint blockage or understanding underlying mechanisms of resistance. In a landmark study, Dijkstra and colleagues co-cultured peripheral blood lymphocytes with matched cancer organoids and showed that the co-culture yielded tumor-reactive T-cells.338 Of notable mention, as the co-culture system involved the use of animal-derived basement membrane extracts, the authors reported that the material can induce CD4+ T cell reactivity that is not directed against tumor antigens. To circumvent the reactivity of basement membrane extracts, protocols now call for a scaffold-less approach by isolating organoids prior to co-culture with peripheral blood lymphocytes.339 This finding highlights the need for synthetic matrices that would not elicit undesired T cell reactivity, and more broadly, an increased understanding of matrix cues that may alter T cell behavior such as proliferation and activation. As an example, Pérez Del Río and colleagues engineered PEG hydrogels covalently linked with low molecular weight heparin to present the cytokine CCL21, mimicking the lymph nodes to support T cell expansion.340 In another example, Kim and colleagues investigated different hydrogel systems – collagen, fibrin and collagen-fibrin – to support lymphoid stromal network formation and T-cell interaction. While fibrin gel-encapsulated stromal cells enabled T-cell penetration due to stromal remodeling that created voids for T-cell migration, T-cell migration was not observed for the other two gel systems.341 This study highlights again, the importance of considering matrix properties, in this case, mesh size, that can support T-cell motility. T-cell motility would be particularly important in generating complex immune contextures to study T-cell migration within the TME.
In sum, recapitulating cancer-immune interactions in vitro is particularly challenging as immune cells, being innate environmental sensors, exhibit phenotypes and functions that can be modulated by biochemical and biophysical cues.342 As such, increased attention needs to be placed on deeply characterizing these cells when cultured in hydrogel conditions which attempt to recapitulate the TME.
5. Hydrogels for next generation tumor modeling
In recent years, with cancer organoid models becoming the in vitro gold standard for mechanistic studies and drug screening, the use of naturally-derived matrices such as Matrigel® is becoming increasing popular. While a handful of studies have explored the development and characterization of alternative hydrogel systems which enable the identification of critical acellular cues (ECM and matrix stiffness) that support organoid culture, only very few models have considered the incorporation of cellular TME components and the tumor architecture. Given the increasing recognition that targeting the TME may be necessary to successfully control tumors in patients such as in the case of immune checkpoint inhibitors, there is clearly a need to build complex tumor models in vitro that accurately recapitulates the network of relevant TME interactions. For this, several challenges exist. First, how should patient-derived stromal cells be cultured in vitro while preserving function and heterogeneity343? Studies suggest that some stromal cell types, such as macrophages and CAFs, may be particularly contextual in which phenotype changes with the environment.313 Hence, there is an urgent need to develop hydrogels that are not only conducive for maintaining cancer organoids, but also different stromal cell types of interest. As described, very few studies have begun to investigate how the stromal cell phenotype changes with dimensionality, biochemical and biophysical cues. Apart from maintenance, efforts also need to be placed on designing hydrogels that support stromal cell expansion, such as in the case of T-cells, if these stromal cells were to be eventually used to build the final tumor model. Instead of just focusing on RGD to represent an adhesive domain found in many ECM proteins, investigations should also focus on leveraging hydrogels to probe the role of other ECM components344 in the TME, such as the different splice variants of fibronectin, tenascin and proteoglycans, for culturing stromal (and cancer) cells. Additionally, besides stiffness as a biophysical cue, recent studies demonstrating the effect of stress-relaxation345 and stress-stiffening279 on cell spreading and differentiation suggests that these parameters should also be investigated in carefully designed hydrogel systems for stromal cell culture.
The micro- and macro-architecture of tumors have thus far been largely overlooked in in vitro tumor models. Using live-imaging of tumor slices, it was previously shown that T-cell migration in tumors is affected by the density and orientation of the stromal ECM.346 While T-cells were actively motile in loose fibronectin and collagen regions, the reverse was observed for dense matrix areas. Importantly, T-cells preferentially migrated along aligned ECM fibers in perivascular regions or around tumor nests which prevented T-cells from reaching the cancer cells. As most studies on T-cell migration use fibrous collagen gels, hydrogel systems which enable the presentation of fibrous micro-architectures may be very valuable for the reconstruction of the immune contexture in the TME for the study of T-cell migration. Lastly, recent advances in spatial analysis of the TME at the single-cell level347,348 have delineated the localization patterns of different stromal cell types with respect to cancer, highlighting the importance of recapitulating the architecture or spatial distribution of cells in tumor models.347 For example, Ji and colleagues showed that in squamous cell carcinomas, while fibroblasts and macrophages accumulate at the tumor-stroma interface, CD8+ T-cells and neutrophils were largely excluded from the tumor.347 Hydrogel systems which enable spatial patterning and positioning of cells may be useful to reconstruct the tumor architecture.349
In sum, in vitro tumor modeling is on the rapid uphill climb with the advent of organoid technologies. Hydrogels are a versatile tool for recapitulating cellular and acellular components of the TME. With further efforts in enabling the incorporation of heterogeneous stromal cell populations as well as tumor architecture, hydrogel-based tumor models may be a very valuable tool for drug development and personalized medicine.
Conflicts of interest
There are no conflicts to declare.
Acknowledgements
E. L. S. Fong would like to acknowledge funding from the National University of Singapore Start-Up grant and the National Medical Research Council-Young Individual Research Grant (MOH-000136).
References
- World Health Organization, Cancer, https://www.who.int/news-room/fact-sheets/detail/cancer, (accessed 2nd November 2020).
- C. Roma-Rodrigues, R. Mendes, P. V. Baptista and A. R. Fernandes, Int. J. Mol. Sci., 2019, 20, 840 CrossRef CAS.
- F. R. Balkwill, M. Capasso and T. Hagemann, J. Cell Sci., 2012, 125, 5591 CrossRef CAS.
- D. Hanahan and L. M. Coussens, Cancer Cell, 2012, 21, 309–322 CrossRef CAS.
- S. Nallanthighal, J. P. Heiserman and D.-J. Cheon, Front. Cell Dev. Biol., 2019, 7, 86 CrossRef.
- Y.-H. Wang, Y.-Y. Dong, W.-M. Wang, X.-Y. Xie, Z.-M. Wang, R.-X. Chen, J. Chen, D.-M. Gao, J.-F. Cui and Z.-G. Ren, J. Exp. Clin. Cancer Res., 2013, 32, 51–51 CrossRef CAS.
- S. C. Schwager, P. V. Taufalele and C. A. Reinhart-King, Cell. Mol. Bioeng., 2019, 12, 1–14 CrossRef.
- D. M. S. van Marion, U. M. Domanska, H. Timmer-Bosscha and A. M. E. Walenkamp, Crit. Rev. Oncol. Hematol., 2016, 97, 107–117 CrossRef.
- W. Wang, N. G. Iyer, H. T. u. Tay, Y. Wu, T. K. H. Lim, L. Zheng, I. C. Song, C. K. Kwoh, H. Huynh, P. O. B. Tan and P. K. H. Chow, BMC Cancer, 2015, 15, 828–828 CrossRef.
- M. Breitenbach and J. Hoffmann, Front. Oncol., 2018, 8, 401 CrossRef.
- J. Mestas and C. C. W. Hughes, J. Immunol., 2004, 172, 2731–2738 CrossRef CAS.
- T. Denayer, T. Stöhr and M. Van Roy, New Horiz. Transl. Med., 2014, 2, 5–11 Search PubMed.
- M. Mandon, S. Huet, E. Dubreil, V. Fessard and L. Le Hégarat, Sci. Rep., 2019, 9, 10548 CrossRef.
- A. Riedl, M. Schlederer, K. Pudelko, M. Stadler, S. Walter, D. Unterleuthner, C. Unger, N. Kramer, M. Hengstschläger, L. Kenner, D. Pfeiffer, G. Krupitza and H. Dolznig, J. Cell Sci., 2017, 130, 203 CrossRef CAS.
- P. Benien and A. Swami, Future Oncol., 2014, 10, 1311–1327 CrossRef CAS.
- S. Nath and G. R. Devi, Pharmacol. Ther., 2016, 163, 94–108 CrossRef CAS.
- A. Nyga, U. Cheema and M. Loizidou, J. Cell Commun. Signaling, 2011, 5, 239 CrossRef.
- R. Edmondson, J. J. Broglie, A. F. Adcock and L. Yang, Assay Drug Dev. Technol., 2014, 12, 207–218 CrossRef CAS.
- H. Nalluri, S. Subramanian and C. Staley, Future Microbiol., 2020, 15, 16 Search PubMed.
- S. A. Langhans, Front. Pharmacol., 2018, 9, 6 CrossRef.
- J. Hoarau-Véchot, A. Rafii, C. Touboul and J. Pasquier, Int. J. Mol. Sci., 2018, 19, 181 CrossRef.
- R. M. Sutherland, Pharmacol. Ther., 1980, 8, 105–123 CrossRef CAS.
- Y. Fang and R. M. Eglen, SLAS Discovery, 2017, 22, 456–472 CAS.
- D. Lv, Z. Hu, L. Lu, H. Lu and X. Xu, Oncol. Lett., 2017, 14, 6999–7010 Search PubMed.
- N.-E. Ryu, S.-H. Lee and H. Park, Cells, 2019, 8, 1620 CrossRef CAS.
- T.-M. Achilli, J. Meyer and J. R. Morgan, Expert Opin. Biol. Ther., 2012, 12, 1347–1360 CrossRef CAS.
- F. Hirschhaeuser, H. Menne, C. Dittfeld, J. West, W. Mueller-Klieser and L. A. Kunz-Schughart, J. Biotechnol., 2010, 148, 3–15 CrossRef CAS.
- T. Herheliuk, O. Perepelytsina, A. Ugnivenko, L. Ostapchenko and M. Sydorenko, Stem Cell Invest., 2019, 6, 21 CrossRef CAS.
- S. Melissaridou, E. Wiechec, M. Magan, M. V. Jain, M. K. Chung, L. Farnebo and K. Roberg, Cancer Cell Int., 2019, 19, 16 CrossRef.
- A. S. Nunes, A. S. Barros, E. C. Costa, A. F. Moreira and I. J. Correia, Biotechnol. Bioeng., 2019, 116, 206–226 CrossRef CAS.
- R. M. Sutherland, B. Sordat, J. Bamat, H. Gabbert, B. Bourrat and W. Mueller-Klieser, Cancer Res., 1986, 46, 5320–5329 CAS.
- N. Barker, J. H. van Es, J. Kuipers, P. Kujala, M. van den Born, M. Cozijnsen, A. Haegebarth, J. Korving, H. Begthel, P. J. Peters and H. Clevers, Nature, 2007, 449, 1003–1007 CrossRef CAS.
- Q. Zhang, Y. Rong, K. Yi, L. Huang, M. Chen and F. Wang, Theranostics, 2020, 10, 12060–12071 CrossRef CAS.
- J. Kim, B.-K. Koo and J. A. Knoblich, Nat. Rev. Mol. Cell Biol., 2020, 21, 571–584 CrossRef CAS.
- A. Fatehullah, P. L. Appleton and I. S. Näthke, Philos. Trans. R. Soc., B, 2013, 368, 20130014–20130014 CrossRef.
- S. J. Edwards, V. Carannante, K. Kuhnigk, H. Ring, T. Tararuk, F. Hallböök, H. Blom, B. Önfelt and H. Brismar, Front. Mol. Biosci., 2020, 7, 208 CrossRef.
- Y.-Z. Nie, Y.-W. Zheng, M. Ogawa, E. Miyagi and H. Taniguchi, Stem Cell Res. Ther., 2018, 9, 5 CrossRef CAS.
- M. Fukuda, T. Mizutani, W. Mochizuki, T. Matsumoto, K. Nozaki, Y. Sakamaki, S. Ichinose, Y. Okada, T. Tanaka, M. Watanabe and T. Nakamura, Genes Dev., 2014, 28, 1752–1757 CrossRef CAS.
- M. Funata, Y. Nio, D. M. Erion, W. L. Thompson and T. Takebe, Cell Death Differ., 2020 DOI:10.1038/s41418-020-00661-3.
- Y.-H. Lo, K. Karlsson and C. J. Kuo, Nat. Cancer, 2020, 1, 761–773 CrossRef.
- Y. Li, D. Guo, Y. Zhang, L. Wang, T. Sun, Z. Li, X. Zhang, S. Wang, Y. Chen and A. Wu, Transl. Oncol., 2020, 14, 100935–100935 CrossRef.
- M. Matano, S. Date, M. Shimokawa, A. Takano, M. Fujii, Y. Ohta, T. Watanabe, T. Kanai and T. Sato, Nat. Med., 2015, 21, 256–262 CrossRef CAS.
- K. Dzobo, A. Rowe, D. A. Senthebane, M. A. M. AlMazyadi, V. Patten and M. I. Parker, OMICS, 2018, 22, 733–748 CrossRef CAS.
- J. Drost, R. H. van Jaarsveld, B. Ponsioen, C. Zimberlin, R. van Boxtel, A. Buijs, N. Sachs, R. M. Overmeer, G. J. Offerhaus, H. Begthel, J. Korving, M. van de Wetering, G. Schwank, M. Logtenberg, E. Cuppen, H. J. Snippert, J. P. Medema, G. J. P. L. Kops and H. Clevers, Nature, 2015, 521, 43–47 CrossRef CAS.
- E. Driehuis, K. Kretzschmar and H. Clevers, Nat. Protoc., 2020, 15, 3380–3409 CrossRef CAS.
- J. Drost and H. Clevers, Development, 2017, 144, 968 CrossRef CAS.
- K. G. Huo, E. D’Arcangelo and M. S. Tsao, Transl. Lung Cancer Res., 2020, 9(5), 2214–2232 CrossRef CAS.
- J. Sumbal, Z. Budkova, G. Á. Traustadóttir and Z. Koledova, J. Mammary Gland Biol. Neoplasia, 2020 DOI:10.1007/s10911-020-09468-x.
- G. Vlachogiannis, S. Hedayat, A. Vatsiou, Y. Jamin, J. Fernández-Mateos, K. Khan, A. Lampis, K. Eason, I. Huntingford, R. Burke, M. Rata, D. M. Koh, N. Tunariu, D. Collins, S. Hulkki-Wilson, C. Ragulan, I. Spiteri, S. Y. Moorcraft, I. Chau, S. Rao, D. Watkins, N. Fotiadis, M. Bali, M. Darvish-Damavandi, H. Lote, Z. Eltahir, E. C. Smyth, R. Begum, P. A. Clarke, J. C. Hahne, M. Dowsett, J. de Bono, P. Workman, A. Sadanandam, M. Fassan, O. J. Sansom, S. Eccles, N. Starling, C. Braconi, A. Sottoriva, S. P. Robinson, D. Cunningham and N. Valeri, Science, 2018, 359, 920–926 CrossRef CAS.
- O. Grbovic-Huezo, K. L. Pitter, N. Lecomte, J. Saglimbeni, G. Askan, M. Holm, J. P. Melchor, R. Chandwani, S. Joshi, C. Haglund, C. A. Iacobuzio-Donahue, G. Chiosis, T. Tammela and S. D. Leach, Proc. Natl. Acad. Sci. U. S. A., 2020, 117, 30670 CrossRef CAS.
- L. Puca, R. Bareja, D. Prandi, R. Shaw, M. Benelli, W. R. Karthaus, J. Hess, M. Sigouros, A. Donoghue, M. Kossai, D. Gao, J. Cyrta, V. Sailer, A. Vosoughi, C. Pauli, Y. Churakova, C. Cheung, L. D. Deonarine, T. J. McNary, R. Rosati, S. T. Tagawa, D. M. Nanus, J. M. Mosquera, C. L. Sawyers, Y. Chen, G. Inghirami, R. A. Rao, C. Grandori, O. Elemento, A. Sboner, F. Demichelis, M. A. Rubin and H. Beltran, Nat Commun, 2018, 9(1), 2404 CrossRef.
- K. Cheaito, H. F. Bahmad, H. Jalloul, O. Hadadeh, H. Msheik, A. El-Hajj, D. Mukherji, M. Al-Sayegh and W. Abou-Kheir, Front. Cell Dev. Biol., 2020, 8, 571677–571677 CrossRef.
- K. Dzobo, OMICS, 2020, 24, 175–179 CrossRef CAS.
- H. Xu, Y. Jiao, S. Qin, W. Zhao, Q. Chu and K. Wu, Exp. Hematol. Oncol., 2018, 7, 30–30 CrossRef CAS.
- M. Kim, H. Mun, C. O. Sung, E. J. Cho, H.-J. Jeon, S.-M. Chun, D. J. Jung, T. H. Shin, G. S. Jeong, D. K. Kim, E. K. Choi, S.-Y. Jeong, A. M. Taylor, S. Jain, M. Meyerson and S. J. Jang, Nat. Commun., 2019, 10, 3991 CrossRef.
- C. Belli, D. Trapani, G. Viale, P. D’Amico, B. A. Duso, P. D. Vigna, F. Orsi and G. Curigliano, Cancer Treat. Rev., 2018, 65, 22–32 CrossRef CAS.
- V. Vultaggio-Poma, A. C. Sarti and F. Di Virgilio, Cells, 2020, 9(11), 2496 CrossRef.
- C.-H. Heldin, K. Rubin, K. Pietras and A. Östman, Nat. Rev. Cancer, 2004, 4, 806–813 CrossRef CAS.
- B. S. Sørensen and M. R. Horsman, Front. Oncol., 2020, 10, 562 CrossRef.
- C. Frantz, K. M. Stewart and V. M. Weaver, J. Cell Sci., 2010, 123, 4195–4200 CrossRef CAS.
- M. Yamauchi, T. H. Barker, D. L. Gibbons and J. M. Kurie, J. Clin. Invest., 2018, 128, 16–25 CrossRef.
- S. Xu, H. Xu, W. Wang, S. Li, H. Li, T. Li, W. Zhang, X. Yu and L. Liu, J. Transl. Med., 2019, 17, 309 CrossRef.
- G. Bendas and L. Borsig, Int. J. Cell Biol., 2012, 2012, 676731 Search PubMed.
- M. Janiszewska, M. C. Primi and T. Izard, J. Biol. Chem., 2020, 295, 2495–2505 CrossRef CAS.
- N. Kozlova, J. E. Grossman, M. P. Iwanicki and T. Muranen, Trends Pharmacol. Sci., 2020, 41, 183–198 CrossRef CAS.
- D. A. Senthebane, T. Jonker, A. Rowe, N. E. Thomford, D. Munro, C. Dandara, A. Wonkam, D. Govender, B. Calder, N. C. Soares, J. M. Blackburn, M. I. Parker and K. Dzobo, Int. J. Mol. Sci., 2018, 19(10), 2861 CrossRef.
- F. Spill, D. S. Reynolds, R. D. Kamm and M. H. Zaman, Curr. Opin. Biotechnol, 2016, 40, 41–48 CrossRef CAS.
- C. Frantz, K. M. Stewart and V. M. Weaver, J. Cell Sci., 2010, 123, 4195–4200 CrossRef CAS.
- C. Bonnans, J. Chou and Z. Werb, Nat. Rev. Mol. Cell Biol., 2014, 15, 786–801 CrossRef CAS.
- M. W. Pickup, J. K. Mouw and V. M. Weaver, EMBO Rep., 2014, 15, 1243–1253 CrossRef CAS.
- F. Kai, A. P. Drain and V. M. Weaver, Dev. Cell, 2019, 49, 332–346 CrossRef CAS.
- P. Lu, V. M. Weaver and Z. Werb, J. Cell Biol., 2012, 196, 395–406 CrossRef CAS.
- V. B. Lokeshwar, S. Mirza and A. Jordan, Adv. Cancer Res., 2014, 123, 35–65 CrossRef.
- G. Bendas and L. Borsig, Int. J. Cell Biol., 2012, 2012, 676731 CrossRef.
- V. Gkretsi and T. Stylianopoulos, Front. Oncol., 2018, 8, 145 CrossRef.
- M. Egeblad, M. G. Rasch and V. M. Weaver, Curr. Opin. Cell Biol., 2010, 22, 697–706 CrossRef CAS.
- S. Yazdani, R. Bansal and J. Prakash, Adv. Drug Delivery Rev., 2017, 121, 101–116 CrossRef CAS.
- D. Medici and A. Nawshad, Matrix Biol., 2010, 29, 161–165 CrossRef CAS.
- D. E. Kuczek, A. M. H. Larsen, M.-L. Thorseth, M. Carretta, A. Kalvisa, M. S. Siersbæk, A. M. C. Simões, A. Roslind, L. H. Engelholm, E. Noessner, M. Donia, I. M. Svane, P. t. Straten, L. Grøntved and D. H. Madsen, J. ImmunoTher. Cancer, 2019, 7, 68 CrossRef.
- M. Liu, C. Tolg and E. Turley, Front. Immunol., 2019, 10, 947 CrossRef CAS.
- N. C. Singha, T. Nekoroski, C. Zhao, R. Symons, P. Jiang, G. I. Frost, Z. Huang and H. M. Shepard, Mol. Cancer Ther., 2015, 14, 523–532 CrossRef CAS.
- Y. Ye, R. Zhang and H. Feng, Cell Biol. Int., 2020, 44, 1840–1849 CrossRef CAS.
- R. N. Kaplan, R. D. Riba, S. Zacharoulis, A. H. Bramley, L. Vincent, C. Costa, D. D. MacDonald, D. K. Jin, K. Shido, S. A. Kerns, Z. Zhu, D. Hicklin, Y. Wu, J. L. Port, N. Altorki, E. R. Port, D. Ruggero, S. V. Shmelkov, K. K. Jensen, S. Rafii and D. Lyden, Nature, 2005, 438, 820–827 CrossRef CAS.
- A. Das, M. Monteiro, A. Barai, S. Kumar and S. Sen, Sci. Rep., 2017, 7, 14219 CrossRef.
- K. Kessenbrock, V. Plaks and Z. Werb, Cell, 2010, 141, 52–67 CrossRef CAS.
- M. Egeblad and Z. Werb, Nat. Rev. Cancer, 2002, 2, 161–174 CrossRef CAS.
- C. Gialeli, A. D. Theocharis and N. K. Karamanos, FEBS J., 2011, 278, 16–27 CrossRef CAS.
- M. Kalli and T. Stylianopoulos, Front. Oncol., 2018, 8, 55–55 CrossRef.
- J. Qian and E. B. Rankin, Adv. Exp. Med. Biol., 2019, 1136, 43–55 CrossRef CAS.
- A. López-Carrasco, S. Martín-Vañó, R. Burgos-Panadero, E. Monferrer, A. P. Berbegall, B. Fernández-Blanco, S. Navarro and R. Noguera, J. Exp. Clin. Cancer Res., 2020, 39, 226 CrossRef.
- B. Emon, J. Bauer, Y. Jain, B. Jung and T. Saif, Comput. Struct. Biotechnol. J., 2018, 16, 279–287 CrossRef CAS.
- F. Bordeleau, B. N. Mason, E. M. Lollis, M. Mazzola, M. R. Zanotelli, S. Somasegar, J. P. Califano, C. Montague, D. J. LaValley, J. Huynh, N. Mencia-Trinchant, Y. L. Negrón Abril, D. C. Hassane, L. J. Bonassar, J. T. Butcher, R. S. Weiss and C. A. Reinhart-King, Proc. Natl. Acad. Sci. U. S. A., 2017, 114, 492–497 CrossRef CAS.
- N. I. Nissen, M. Karsdal and N. Willumsen, J. Exp. Clin. Cancer Res., 2019, 38, 115 CrossRef.
- I. Acerbi, L. Cassereau, I. Dean, Q. Shi, A. Au, C. Park, Y. Y. Chen, J. Liphardt, E. S. Hwang and V. M. Weaver, Integr. Biol., 2015, 7, 1120–1134 CrossRef CAS.
- V. Petrova, M. Annicchiarico-Petruzzelli, G. Melino and I. Amelio, Oncogenesis, 2018, 7, 10 CrossRef.
- J. I. Lopez, I. Kang, W. K. You, D. M. McDonald and V. M. Weaver, Integr. Biol., 2011, 3, 910–921 CrossRef CAS.
- S. Kawano, M. Kojima, Y. Higuchi, M. Sugimoto, K. Ikeda, N. Sakuyama, S. Takahashi, R. Hayashi, A. Ochiai and N. Saito, Cancer Sci., 2015, 106, 1232–1239 CrossRef CAS.
- M.-T. Melissari, N. Chalkidi, M. E. Sarris and V. Koliaraki, Front. Cell Dev. Biol., 2020, 8, 630 CrossRef.
- R. Kalluri and M. Zeisberg, Nat. Rev. Cancer, 2006, 6, 392–401 CrossRef CAS.
- T. Liu, L. Zhou, D. Li, T. Andl and Y. Zhang, Front. Cell Dev. Biol., 2019, 7, 60–60 CrossRef.
- C. Thompson, S. Rahim, J. Arnold and A. Hielscher, PLoS One, 2017, 12, e0172067 CrossRef.
- L. Hui, J. Zhang, X. Ding, X. Guo and X. Jiang, Int. J. Oncol., 2017, 50, 1439–1447 CrossRef CAS.
- J. Schrader, T. T. Gordon-Walker, R. L. Aucott, M. van Deemter, A. Quaas, S. Walsh, D. Benten, S. J. Forbes, R. G. Wells and J. P. Iredale, Hepatology, 2011, 53, 1192–1205 CrossRef CAS.
- S. E. Reid, E. J. Kay, L. J. Neilson, A.-T. Henze, J. Serneels, E. J. McGhee, S. Dhayade, C. Nixon, J. B. G. Mackey, A. Santi, K. Swaminathan, D. Athineos, V. Papalazarou, F. Patella, Á. Román-Fernández, Y. ElMaghloob, J. R. Hernandez-Fernaud, R. H. Adams, S. Ismail, D. M. Bryant, M. Salmeron-Sanchez, L. M. Machesky, L. M. Carlin, K. Blyth, M. Mazzone and S. Zanivan, EMBO J., 2017, 36, 2373–2389 CrossRef CAS.
- V. Gkretsi and T. Stylianopoulos, Front. Oncol., 2018, 8, 145–145 CrossRef.
- S. Noguchi, A. Saito and T. Nagase, Int. J. Mol. Sci., 2018, 19, 3674 CrossRef.
- J. Bauer, M. A. B. Emon, J. J. Staudacher, A. L. Thomas, J. Zessner-Spitzenberg, G. Mancinelli, N. Krett, M. T. Saif and B. Jung, Sci. Rep., 2020, 10, 50 CrossRef CAS.
- E. J. Hoffmann and S. M. Ponik, Front. Oncol., 2020, 10, 787 CrossRef.
- H. Wang, R. Mislati, R. Ahmed, P. Vincent, S. F. Nwabunwanne, J. R. Gunn, B. W. Pogue and M. M. Doyley, Clin. Cancer Res., 2019, 25, 2136 CrossRef CAS.
- J. Folkman, N. Engl. J. Med., 1971, 285, 1182–1186 CrossRef CAS.
- J. Folkman, JNCI, J. Natl. Cancer Inst., 1990, 82, 4–7 CrossRef CAS.
- F. Hillen and A. W. Griffioen, Cancer Metastasis Rev., 2007, 26, 489–502 CrossRef.
- D. W. Siemann, Cancer Treat. Rev., 2011, 37, 63–74 CrossRef CAS.
-
J. Nagy, A. Husain, S.-C. Jaminet, A. Dvorak and H. Dvorak, Tumor Vasculature, Structure and Function, 2013 Search PubMed.
- M. A. Konerding, E. Fait and A. Gaumann, Br. J. Cancer, 2001, 84, 1354–1362 CrossRef CAS.
- W. Liang, Y. Ni and F. Chen, Oncotarget, 2016, 7, 15444–15459 CrossRef.
- D. J. Chaplin, P. L. Olive and R. E. Durand, Cancer Res., 1987, 47, 597–601 CAS.
- M. B. Schaaf, A. D. Garg and P. Agostinis, Cell Death Dis., 2018, 9, 115 CrossRef.
- I. Marech, C. Leporini, M. Ammendola, M. Porcelli, C. D. Gadaleta, E. Russo, G. De Sarro and G. Ranieri, Cancer Lett., 2016, 380, 216–226 CrossRef CAS.
- R. Lugano, M. Ramachandran and A. Dimberg, Cell. Mol. Life Sci., 2020, 77, 1745–1770 CrossRef CAS.
- S. A. Hendry, R. H. Farnsworth, B. Solomon, M. G. Achen, S. A. Stacker and S. B. Fox, Front. Immunol., 2016, 7, 621–621 Search PubMed.
- J. S. Pober and G. Tellides, Trends Immunol., 2012, 33, 49–57 CrossRef CAS.
- L. Borsig, Glycobiology, 2018, 28, 648–655 CrossRef CAS.
- Y. Huang, B. Y. S. Kim, C. K. Chan, S. M. Hahn, I. L. Weissman and W. Jiang, Nat. Rev. Immunol., 2018, 18, 195–203 CrossRef CAS.
- A. Albini, A. Bruno, D. M. Noonan and L. Mortara, Front. Immunol., 2018, 9, 527 CrossRef.
- C. Zhu, J. M. Kros, C. Cheng and D. Mustafa, Neuro-Oncology, 2017, 19, 1435–1446 CrossRef CAS.
- R. I. Teleanu, C. Chircov, A. M. Grumezescu and D. M. Teleanu, J. Clin. Med., 2019, 9, 84 CrossRef.
- F. Mpekris, C. Voutouri, J. W. Baish, D. G. Duda, L. L. Munn, T. Stylianopoulos and R. K. Jain, Proc. Natl. Acad. Sci. U. S. A., 2020, 117, 3728 CrossRef CAS.
- Z. Liu, Y. Wang, Y. Huang, B. Y. S. Kim, H. Shan, D. Wu and W. Jiang, Trends Pharmacol. Sci., 2019, 40, 613–623 CrossRef CAS.
- M. Schmittnaegel and M. De Palma, Trends Cancer, 2017, 3, 809–812 CrossRef.
- H. F. Dvorak, N. Engl. J. Med., 1986, 315, 1650–1659 CrossRef CAS.
- D. Öhlund, E. Elyada and D. Tuveson, J. Exp. Med., 2014, 211, 1503–1523 CrossRef.
- D. S. Foster, R. E. Jones, R. C. Ransom, M. T. Longaker and J. A. Norton, JCI Insight, 2018, 3, 18 CrossRef.
- B. Rybinski, J. Franco-Barraza and E. Cukierman, Physiol. Genomics, 2014, 46, 223–244 CrossRef CAS.
- L. Liu, L. Liu, H. H. Yao, Z. Q. Zhu, Z. L. Ning and Q. Huang, PLoS One, 2016, 11, e0159947 CrossRef.
- K. Dzobo and C. Dandara, Omics, 2020, 24, 340–351 CrossRef CAS.
- K. Dzobo and C. Dandara, Omics, 2020, 24, 314–339 CrossRef CAS.
- G. Gabbiani, J. Pathol., 2003, 200, 500–503 CrossRef CAS.
- Z. Steinhart and S. Angers, Development, 2018, 145, dev146589 CrossRef.
- N. Ferrari, R. Ranftl, I. Chicherova, N. D. Slaven, E. Moeendarbary, A. J. Farrugia, M. Lam, M. Semiannikova, M. C. W. Westergaard, J. Tchou, L. Magnani and F. Calvo, Nat. Commun., 2019, 10, 130 CrossRef.
- R. Scherz-Shouval, S. Santagata, M. L. Mendillo, L. M. Sholl, I. Ben-Aharon, A. H. Beck, D. Dias-Santagata, M. Koeva, S. M. Stemmer, L. Whitesell and S. Lindquist, Cell, 2014, 158, 564–578 CrossRef CAS.
- Y. Kojima, A. Acar, E. N. Eaton, K. T. Mellody, C. Scheel, I. Ben-Porath, T. T. Onder, Z. C. Wang, A. L. Richardson, R. A. Weinberg and A. Orimo, Proc. Natl. Acad. Sci. U. S. A., 2010, 107, 20009–20014 CrossRef CAS.
- T. Liu, L. Zhou, D. Li, T. Andl and Y. Zhang, Front. Cell Dev. Biol., 2019, 7, 60 CrossRef.
- M. E. Fiori, S. Di Franco, L. Villanova, P. Bianca, G. Stassi and R. De Maria, Mol. Cancer, 2019, 18, 70 CrossRef.
- E. Y. Lau, J. Lo, B. Y. Cheng, M. K. Ma, J. M. Lee, J. K. Ng, S. Chai, C. H. Lin, S. Y. Tsang, S. Ma, I. O. Ng and T. K. Lee, Cell Rep., 2016, 15, 1175–1189 CrossRef CAS.
- W. J. Chen, C. C. Ho, Y. L. Chang, H. Y. Chen, C. A. Lin, T. Y. Ling, S. L. Yu, S. S. Yuan, Y. J. Chen, C. Y. Lin, S. H. Pan, H. Y. Chou, Y. J. Chen, G. C. Chang, W. C. Chu, Y. M. Lee, J. Y. Lee, P. J. Lee, K. C. Li, H. W. Chen and P. C. Yang, Nat. Commun., 2014, 5, 3472 CrossRef.
- X. Long, W. Xiong, X. Zeng, L. Qi, Y. Cai, M. Mo, H. Jiang, B. Zhu, Z. Chen and Y. Li, Cell Death Dis., 2019, 10, 375 CrossRef.
- T. Liu, C. Han, S. Wang, P. Fang, Z. Ma, L. Xu and R. Yin, J. Hematol. Oncol., 2019, 12, 86 CrossRef.
- L. Monteran and N. Erez, Front. Immunol., 2019, 10, 1835 CrossRef CAS.
- H. Takahashi, K. Sakakura, T. Kudo, M. Toyoda, K. Kaira, T. Oyama and K. Chikamatsu, Oncotarget, 2017, 8, 8633–8647 CrossRef.
- R. Zhang, F. Qi, F. Zhao, G. Li, S. Shao, X. Zhang, L. Yuan and Y. Feng, Cell Death Dis., 2019, 10, 273–273 CrossRef.
- Z. Li, J. Zhou, J. Zhang, S. Li, H. Wang and J. Du, Int. J. Cancer, 2019, 145, 1946–1957 CAS.
- B. G. Yavuz, G. Gunaydin, M. E. Gedik, K. Kosemehmetoglu, D. Karakoc, F. Ozgur and D. Guc, Sci. Rep., 2019, 9, 3172 CrossRef.
- R. J. Seager, C. Hajal, F. Spill, R. D. Kamm and M. H. Zaman, Convergent Sci. Phys. Oncol., 2017, 3, 034002 CrossRef.
- Y. An, F. Liu, Y. Chen and Q. Yang, J. Cell. Mol. Med., 2020, 24, 13–24 CrossRef.
- Y. Jiang, Y. Li and B. Zhu, Cell Death Dis., 2015, 6, e1792–e1792 CrossRef CAS.
- T. Kitamura, D. Doughty-Shenton, L. Cassetta, S. Fragkogianni, D. Brownlie, Y. Kato, N. Carragher and J. W. Pollard, Front. Immunol., 2018, 8, 2004 CrossRef.
- H. Gonzalez, C. Hagerling and Z. Werb, Genes Dev., 2018, 32, 1267–1284 CrossRef CAS.
- F. Balkwill and A. Mantovani, Lancet, 2001, 357, 539–545 CrossRef CAS.
- L. M. Coussens and Z. Werb, Nature, 2002, 420, 860–867 CrossRef CAS.
- F. R. Greten and S. I. Grivennikov, Immunity, 2019, 51, 27–41 CrossRef CAS.
- S. I. Grivennikov, F. R. Greten and M. Karin, Cell, 2010, 140, 883–899 CrossRef CAS.
- S. K. Biswas, A. Sica and C. E. Lewis, J. Immunol., 2008, 180, 2011–2017 CrossRef.
- N. Bercovici, M. V. Guérin, A. Trautmann and E. Donnadieu, Front. Immunol., 2019, 10, 1563 CrossRef CAS.
- S. Wan, E. Zhao, I. Kryczek, L. Vatan, A. Sadovskaya, G. Ludema, D. M. Simeone, W. Zou and T. H. Welling, Gastroenterology, 2014, 147, 1393–1404 CrossRef CAS.
- T. Nagasaki, M. Hara, H. Nakanishi, H. Takahashi, M. Sato and H. Takeyama, Br. J. Cancer, 2014, 110, 469–478 CrossRef CAS.
- M. Walter, S. Liang, S. Ghosh, P. J. Hornsby and R. Li, Oncogene, 2009, 28, 2745–2755 CrossRef CAS.
- X. Wu, P. Tao, Q. Zhou, J. Li, Z. Yu, X. Wang, J. Li, C. Li, M. Yan, Z. Zhu, B. Liu and L. Su, Oncotarget, 2017, 8, 20741–20750 CrossRef.
- D. E. Johnson, R. A. O'Keefe and J. R. Grandis, Nat. Rev. Clin. Oncol., 2018, 15, 234–248 CrossRef CAS.
- L. Kong, Y. Zhou, H. Bu, T. Lv, Y. Shi and J. Yang, J. Exp. Clin. Cancer Res., 2016, 35, 131 CrossRef.
- D. T. Fisher, M. M. Appenheimer and S. S. Evans, Semin. Immunol., 2014, 26, 38–47 CrossRef CAS.
- X. Tang, C. Mo, Y. Wang, D. Wei and H. Xiao, Immunology, 2013, 138, 93–104 CrossRef CAS.
- C. Anfray, A. Ummarino, F. T. Andón and P. Allavena, Cells, 2019, 9, 46 CrossRef.
- Z. Zhang, S. Liu, B. Zhang, L. Qiao, Y. Zhang and Y. Zhang, Front. Cell Dev. Biol., 2020, 8, 17 CrossRef.
- S. E. Stanton and M. L. Disis, J. ImmunoTher. Cancer, 2016, 4, 59 CrossRef.
- G. P. Linette and B. M. Carreno, Curr. Hematol. Malig. Rep., 2019, 14, 286–291 CrossRef.
- L. Barrueto, F. Caminero, L. Cash, C. Makris, P. Lamichhane and R. R. Deshmukh, Transl. Oncol., 2020, 13, 100738 CrossRef.
- M. Chapon, C. Randriamampita, E. Maubec, C. Badoual, S. Fouquet, S.-F. Wang, E. Marinho, D. Farhi, M. Garcette, S. Jacobelli, A. Rouquette, A. Carlotti, A. Girod, A. Prévost-Blondel, A. Trautmann, M.-F. Avril and N. Bercovici, J. Invest. Dermatol., 2011, 131, 1300–1307 CrossRef CAS.
- A. J. Korman, K. S. Peggs and J. P. Allison, Adv. Immunol., 2006, 90, 297–339 CAS.
- S. C. Wei, C. R. Duffy and J. P. Allison, Cancer Discovery, 2018, 8, 1069–1086 CrossRef.
- J. Zhang, X. Zhong, H. Jiang, H. Jiang, T. Xie, Y. Tian, R. Li, B. Wang, J. Zhang and Y. Yuan, Aging, 2020, 12, 22509–22526 Search PubMed.
- M. Yu, G. Guo, L. Huang, L. Deng, C. S. Chang, B. R. Achyut, M. Canning, N. Xu, A. S. Arbab, R. J. Bollag, P. C. Rodriguez, A. L. Mellor, H. Shi, D. H. Munn and Y. Cui, Nat. Commun., 2020, 11, 515 CrossRef CAS.
- Y. Li, J. Liu, L. Gao, Y. Liu, F. Meng, X. Li and F. X. Qin, Immunol. Lett., 2020, 220, 88–96 CrossRef CAS.
- L. Laplane, D. Duluc, A. Bikfalvi, N. Larmonier and T. Pradeu, Int. J. Cancer, 2019, 145, 2611–2618 CrossRef CAS.
- D. F. Quail and J. A. Joyce, Nat. Med., 2013, 19, 1423–1437 CrossRef CAS.
- D. A. Senthebane, A. Rowe, N. E. Thomford, H. Shipanga, D. Munro, M. Mazeedi, H. A. M. Almazyadi, K. Kallmeyer, C. Dandara, M. S. Pepper, M. I. Parker and K. Dzobo, Int. J. Mol. Sci., 2017, 18, 1586 CrossRef.
- K. Dzobo, Omics, 2020, 24, 175–179 CrossRef CAS.
- T. Dey, Integr. Biol., 2020 DOI:10.1093/intbio/zyaa023.
- M. Devarasetty, S. D. Forsythe, E. Shelkey and S. Soker, Tissue Eng. Regener. Med., 2020, 17, 759–771 CrossRef.
- H. Jaganathan, J. Gage, F. Leonard, S. Srinivasan, G. R. Souza, B. Dave and B. Godin, Sci. Rep., 2014, 4, 6468 CrossRef CAS.
- I. Yakavets, S. Jenard, A. Francois, Y. Maklygina, V. Loschenov, H. P. Lassalle, G. Dolivet and L. Bezdetnaya, J Clin Med, 2019, 8, 1686 CrossRef CAS.
- M. Durymanov, C. Kroll, A. Permyakova, E. O'Neill, R. Sulaiman, M. Person and J. Reineke, Transl Oncol, 2019, 12, 180–189 CrossRef.
- J. Lee, D. Shin and J. L. Roh, Theranostics, 2018, 8, 3964–3973 CrossRef CAS.
- D. Zboralski and A. Frömming, Methods Mol. Biol., 2019, 1884, 269–281 CrossRef CAS.
- M. J. Lamberti, M. Rettel, J. Krijgsveld, V. A. Rivarola and N. B. Rumie Vittar, Cell. Oncol., 2019, 42, 173–196 CrossRef CAS.
- Q. Gao, Z. Yang, S. Xu, X. Li, X. Yang, P. Jin, Y. Liu, X. Zhou, T. Zhang, C. Gong, X. Wei, D. Liu, C. Sun, G. Chen, J. Hu, L. Meng, J. Zhou, K. Sawada, R. Fruscio, T. W. Grunt, J. Wischhusen, V. M. Vargas-Hernández, B. Pothuri and R. L. Coleman, J. Exp. Med., 2019, 216, 688–703 CrossRef CAS.
- P. Brun, M. Dettin, L. G. Campana, F. Dughiero, P. Sgarbossa, C. Bernardello, A. L. Tosi, A. Zamuner and E. Sieni, Bioelectrochemistry, 2019, 125, 15–24 CrossRef CAS.
- I. M. Adjei and S. Blanka, J. Funct. Biomater., 2015, 6, 81–103 CrossRef CAS.
- M. E. Lombardo, F. Carfì Pavia, I. Vitrano, G. Ghersi, V. Brucato, F. Rosei and V. La Carrubba, Tissue Cell, 2019, 58, 33–41 CrossRef CAS.
- J. Li, Y. Zhou, W. Chen, Z. Yuan, B. You, Y. Liu, S. Yang, F. Li, C. Qu and X. Zhang, ACS Appl. Mater. Interfaces, 2018, 10, 36641–36651 CrossRef CAS.
- T. Rodrigues, B. Kundu, J. Silva-Correia, S. C. Kundu, J. M. Oliveira, R. L. Reis and V. M. Correlo, Pharmacol. Ther., 2018, 184, 201–211 CrossRef CAS.
- H. Ma, C. Feng, J. Chang and C. Wu, Acta Biomater., 2018, 79, 37–59 CrossRef CAS.
- D. Karami, N. Richbourg and V. Sikavitsas, Cancer Lett., 2019, 449, 178–185 CrossRef CAS.
- J. A. Belgodere, C. T. King, J. B. Bursavich, M. E. Burow, E. C. Martin and J. P. Jung, Front. Bioeng. Biotechnol., 2018, 6, 66 CrossRef.
- V. Brancato, J. M. Oliveira, V. M. Correlo, R. L. Reis and S. C. Kundu, Biomaterials, 2020, 232, 119744 CrossRef CAS.
- P. S. Thakuri, C. Liu, G. D. Luker and H. Tavana, Adv. Healthcare Mater., 2018, 7, e1700980 CrossRef.
- G. Rijal and W. Li, Biomaterials, 2016, 81, 135–156 CrossRef CAS.
- M. Guvendiren and J. A. Burdick, Curr. Opin. Biotechnol, 2013, 24, 841–846 CrossRef CAS.
- O. Chaudhuri, Biomater. Sci., 2017, 5, 1480–1490 RSC.
- C. Y. Liaw, S. Ji and M. Guvendiren, Adv. Healthcare Mater., 2018, 7, 4 Search PubMed.
- G. Jose, K. T. Shalumon and J. P. Chen, Curr. Med. Chem., 2020, 27, 2734–2776 CrossRef CAS.
- C. Fuss, J. C. Palmaz and E. A. Sprague, J. Vasc. Interv. Radiol., 2001, 12, 677–682 CrossRef CAS.
- A. D. Augst, H. J. Kong and D. J. Mooney, Macromol. Biosci., 2006, 6, 623–633 CrossRef CAS.
- R. M. Salasznyk, W. A. Williams, A. Boskey, A. Batorsky and G. E. Plopper, J. Biomed. Biotechnol., 2004, 2004, 24–34 CrossRef.
- I. Arnaoutova, J. George, H. K. Kleinman and G. Benton, Stem Cell Rev. Rep., 2012, 8, 163–169 CrossRef CAS.
- R. Tanaka, Y. Saito, Y. Fujiwara, J. I. Jo and Y. Tabata, Acta Biomater., 2019, 89, 152–165 CrossRef CAS.
- G. Benton, I. Arnaoutova, J. George, H. K. Kleinman and J. Koblinski, Adv. Drug Delivery Rev., 2014, 79–80, 3–18 CrossRef CAS.
- G. Rossi, A. Manfrin and M. P. Lutolf, Nat. Rev. Genet., 2018, 19, 671–687 CrossRef CAS.
- S. F. Boj, C. I. Hwang, L. A. Baker, I. I. Chio, D. D. Engle, V. Corbo, M. Jager, M. Ponz-Sarvise, H. Tiriac, M. S. Spector, A. Gracanin, T. Oni, K. H. Yu, R. van Boxtel, M. Huch, K. D. Rivera, J. P. Wilson, M. E. Feigin, D. Ohlund, A. Handly-Santana, C. M. Ardito-Abraham, M. Ludwig, E. Elyada, B. Alagesan, G. Biffi, G. N. Yordanov, B. Delcuze, B. Creighton, K. Wright, Y. Park, F. H. Morsink, I. Q. Molenaar, I. H. Borel Rinkes, E. Cuppen, Y. Hao, Y. Jin, I. J. Nijman, C. Iacobuzio-Donahue, S. D. Leach, D. J. Pappin, M. Hammell, D. S. Klimstra, O. Basturk, R. H. Hruban, G. J. Offerhaus, R. G. Vries, H. Clevers and D. A. Tuveson, Cell, 2015, 160, 324–338 CrossRef CAS.
- O. Kopper, C. J. de Witte, K. Lohmussaar, J. E. Valle-Inclan, N. Hami, L. Kester, A. V. Balgobind, J. Korving, N. Proost, H. Begthel, L. M. van Wijk, S. A. Revilla, R. Theeuwsen, M. van de Ven, M. J. van Roosmalen, B. Ponsioen, V. W. H. Ho, B. G. Neel, T. Bosse, K. N. Gaarenstroom, H. Vrieling, M. P. G. Vreeswijk, P. J. van Diest, P. O. Witteveen, T. Jonges, J. L. Bos, A. van Oudenaarden, R. P. Zweemer, H. J. G. Snippert, W. P. Kloosterman and H. Clevers, Nat. Med., 2019, 25, 838–849 CrossRef CAS.
- H. Dolznig, C. Rupp, C. Puri, C. Haslinger, N. Schweifer, E. Wieser, D. Kerjaschki and P. Garin-Chesa, Am. J. Pathol., 2011, 179, 487–501 CrossRef.
- D. Yip and C. H. Cho, Biochem. Biophys. Res. Commun., 2013, 433, 327–332 CrossRef CAS.
- A. Nyga, M. Loizidou, M. Emberton and U. Cheema, Acta Biomater., 2013, 9, 7917–7926 CrossRef CAS.
- N. Bayat, R. Izadpanah, S. Ebrahimi-Barough, A. Norouzi Javidan, A. Ai, M. M. Mokhtari Ardakan, H. Saberi and J. Ai, Asian Pac. J. Cancer Prev., 2018, 19, 2553–2560 CAS.
- P. Sun, Y. Xu, X. Du, N. Ning, H. Sun, W. Liang and R. Li, Oncol. Lett., 2013, 5, 489–494 CrossRef CAS.
- R. Timpl, G. R. Martin, P. Bruckner, G. Wick and H. Wiedemann, Eur. J. Biochem., 1978, 84, 43–52 CrossRef CAS.
- H. K. Kleinman and G. R. Martin, Semin. Cancer Biol., 2005, 15, 378–386 CrossRef CAS.
- D. J. Carey, M. S. Todd and C. M. Rafferty, J. Cell Biol., 1986, 102, 2254–2263 CrossRef CAS.
- M. A. Hadley, S. W. Byers, C. A. Suàrez-Quian, H. K. Kleinman and M. Dym, J. Cell Biol., 1985, 101, 1511–1522 CrossRef CAS.
- M. C. Kibeey, L. S. Royce, M. Dym, B. J. Baum and H. K. Kleinman, Exp. Cell Res., 1992, 198, 343–351 CrossRef.
- R. F. Nicosia and A. Ottinetti, In Vitro Cell. Dev. Biol., 1990, 26, 119–128 CrossRef CAS.
- C. Oliver, J. F. Waters, C. L. Tolbert and H. K. Kleinman, J. Dent. Res., 1987, 66, 594–595 CrossRef CAS.
- B. Chevallay and D. Herbage, Med. Biol. Eng. Comput., 2000, 38, 211–218 CrossRef CAS.
- M. K. Gordon and R. A. Hahn, Cell Tissue Res., 2010, 339, 247–257 CrossRef CAS.
- M. D. Shoulders and R. T. Raines, Annu. Rev. Biochem., 2009, 78, 929–958 CrossRef CAS.
- D. E. Kuczek, A. M. H. Larsen, M. L. Thorseth, M. Carretta, A. Kalvisa, M. S. Siersbaek, A. M. C. Simoes, A. Roslind, L. H. Engelholm, E. Noessner, M. Donia, I. M. Svane, P. T. Straten, L. Grontved and D. H. Madsen, J. Immunother. Cancer, 2019, 7, 68 CrossRef.
- J. Liu, Y. Tan, H. Zhang, Y. Zhang, P. Xu, J. Chen, Y. C. Poh, K. Tang, N. Wang and B. Huang, Nat. Mater., 2012, 11, 734–741 CrossRef CAS.
- M. W. Mosesson, J. Thromb. Haemostasis., 2005, 3, 1894–1904 CrossRef CAS.
- D. W. Infanger, M. E. Lynch and C. Fischbach, Annu. Rev. Biomed. Eng., 2013, 15, 29–53 CrossRef CAS.
- P. Ngo, P. Ramalingam, J. A. Phillips and G. T. Furuta, Methods Mol. Biol., 2006, 341, 103–109 CAS.
- B. J. Gill and J. L. West, J. Biomech., 2014, 47, 1969–1978 CrossRef.
- S. H. Lang, R. M. Sharrard, M. Stark, J. M. Villette and N. J. Maitland, Br. J. Cancer, 2001, 85, 590–599 CrossRef CAS.
- M. Alemany-Ribes and C. E. Semino, Adv. Drug Delivery Rev., 2014, 79–80, 40–49 CrossRef CAS.
- S. P. Singh, M. P. Schwartz, E. Y. Tokuda, Y. Luo, R. E. Rogers, M. Fujita, N. G. Ahn and K. S. Anseth, Sci. Rep., 2015, 5, 17814 CrossRef CAS.
- C. Wang, X. Tong and F. Yang, Mol. Pharm., 2014, 11, 2115–2125 CrossRef CAS.
- S. Nam, R. Stowers, J. Lou, Y. Xia and O. Chaudhuri, Biomaterials, 2019, 200, 15–24 CrossRef CAS.
- Y. Zhang, D. An, Y. Pardo, A. Chiu, W. Song, Q. Liu, F. Zhou, S. P. McDonough and M. Ma, Acta Biomater., 2017, 53, 100–108 CrossRef CAS.
- V. Hagel, T. Haraszti and H. Boehm, Biointerphases, 2013, 8, 36 CrossRef.
- C. Diaferia, M. Ghosh, T. Sibillano, E. Gallo, M. Stornaiuolo, C. Giannini, G. Morelli, L. Adler-Abramovich and A. Accardo, Soft Matter, 2019, 15, 487–496 RSC.
- Y. Deng, A. X. Sun, K. J. Overholt, G. Z. Yu, M. R. Fritch, P. G. Alexander, H. Shen, R. S. Tuan and H. Lin, Acta Biomater., 2019, 83, 167–176 CrossRef CAS.
- C. C. Lin and K. S. Anseth, Biomacromolecules, 2009, 10, 2460–2467 CrossRef CAS.
- L. Lu, S. Yuan, J. Wang, Y. Shen, S. Deng, L. Xie and Q. Yang, Curr. Stem Cell Res. Ther., 2018, 13, 490–496 CrossRef CAS.
- S. J. Buwalda, K. W. Boere, P. J. Dijkstra, J. Feijen, T. Vermonden and W. E. Hennink, J. Controlled Release, 2014, 190, 254–273 CrossRef CAS.
- K. S. Hof and M. M. C. Bastings, Chimia, 2017, 71, 342–348 CrossRef CAS.
- E. C. González-Díaz and S. Varghese, Gels, 2016, 2, 20 CrossRef.
- K. Vats and D. S. Benoit, Tissue Eng., Part B, 2013, 19, 455–469 CrossRef CAS.
- S. Pradhan, I. Hassani, J. M. Clary and E. A. Lipke, Tissue Eng., Part B, 2016, 22, 470–484 CrossRef CAS.
- S. Pradhan, I. Hassani, W. J. Seeto and E. A. Lipke, J. Biomed. Mater. Res., Part A, 2017, 105, 236–252 CrossRef CAS.
- C. C. Lin and M. Korc, Cancer Lett., 2018, 436, 22–27 CrossRef CAS.
- L. David, V. Dulong, D. Le Cerf, C. Chauzy, V. Norris, B. Delpech, M. Lamacz and J. P. Vannier, Matrix Biol., 2004, 23, 183–193 CrossRef CAS.
- L. David, V. Dulong, D. Le Cerf, L. Cazin, M. Lamacz and J. P. Vannier, Acta Biomater., 2008, 4, 256–263 CrossRef CAS.
- S. Bonnesœur, S. Morin-Grognet, O. Thoumire, D. Le Cerf, O. Boyer, J. P. Vannier and B. Labat, J. Biomed. Mater. Res., Part A, 2020, 108, 1256–1268 CrossRef.
- T. Pouyani, G. S. Harbison and G. D. Prestwich, J. Am. Chem. Soc., 1994, 116, 7515–7522 CrossRef CAS.
- E. Ahmadian, S. M. Dizaj, A. Eftekhari, E. Dalir, P. Vahedi, A. Hasanzadeh and M. Samiei, Drug Res., 2020, 70, 6–11 CrossRef CAS.
- C. Chircov, A. M. Grumezescu and L. E. Bejenaru, Rom. J. Morphol. Embryol., 2018, 59, 71–76 Search PubMed.
- E. L. Fong, X. Wan, J. Yang, M. Morgado, A. G. Mikos, D. A. Harrington, N. M. Navone and M. C. Farach-Carson, Biomaterials, 2016, 77, 164–172 CrossRef CAS.
- G. G. Giobbe, C. Crowley, C. Luni, S. Campinoti, M. Khedr, K. Kretzschmar, M. M. De Santis, E. Zambaiti, F. Michielin, L. Meran, Q. Hu, G. van Son, L. Urbani, A. Manfredi, M. Giomo, S. Eaton, D. Cacchiarelli, V. S. W. Li, H. Clevers, P. Bonfanti, N. Elvassore and P. De Coppi, Nat. Commun., 2019, 10, 5658 CrossRef.
- M. Saheli, M. Sepantafar, B. Pournasr, Z. Farzaneh, M. Vosough, A. Piryaei and H. Baharvand, J. Cell. Biochem., 2018, 119, 4320–4333 CrossRef CAS.
- P. A. Mollica, E. N. Booth-Creech, J. A. Reid, M. Zamponi, S. M. Sullivan, X. L. Palmer, P. C. Sachs and R. D. Bruno, Acta Biomater., 2019, 95, 201–213 CrossRef CAS.
- N. Broguiere, L. Isenmann, C. Hirt, T. Ringel, S. Placzek, E. Cavalli, F. Ringnalda, L. Villiger, R. Züllig, R. Lehmann, G. Rogler, M. H. Heim, J. Schüler, M. Zenobi-Wong and G. Schwank, Adv. Mater., 2018, 30, e1801621 CrossRef.
- N. Gjorevski, N. Sachs, A. Manfrin, S. Giger, M. E. Bragina, P. Ordóñez-Morán, H. Clevers and M. P. Lutolf, Nature, 2016, 539, 560–564 CrossRef CAS.
- G. Sorrentino, S. Rezakhani, E. Yildiz, S. Nuciforo, M. H. Heim, M. P. Lutolf and K. Schoonjans, Nat. Commun., 2020, 11, 3416 CrossRef CAS.
- F. Bergenheim, G. Fregni, C. F. Buchanan, L. B. Riis, M. Heulot, J. Touati, J. B. Seidelin, S. C. Rizzi and O. H. Nielsen, Biomaterials, 2020, 262, 120248 CrossRef CAS.
- S. Ng, W. J. Tan, M. M. X. Pek, M. H. Tan and M. Kurisawa, Biomaterials, 2019, 219, 119400 CrossRef CAS.
- L. Ma, L. Dong and P. Chang, Cell Death Dis., 2019, 10, 30 CrossRef CAS.
- Y. S. Jian, C. W. Chen, C. A. Lin, H. P. Yu, H. Y. Lin, M. Y. Liao, S. H. Wu, Y. F. Lin and P. S. Lai, Int. J. Nanomed., 2017, 12, 2315–2333 CrossRef CAS.
- M. Gavrilov, E. P. Gilbert, A. E. Rowan, J. Lauko and G. E. Yakubov, Macromol. Rapid Commun., 2020, e2000304, DOI:10.1002/marc.202000304.
- P. H. Kouwer, M. Koepf, V. A. Le Sage, M. Jaspers, A. M. van Buul, Z. H. Eksteen-Akeroyd, T. Woltinge, E. Schwartz, H. J. Kitto, R. Hoogenboom, S. J. Picken, R. J. Nolte, E. Mendes and A. E. Rowan, Nature, 2013, 493, 651–655 CrossRef CAS.
- R. K. Das, V. Gocheva, R. Hammink, O. F. Zouani and A. E. Rowan, Nat. Mater., 2016, 15, 318–325 CrossRef CAS.
- Y. Zhang, C. Tang, P. N. Span, A. E. Rowan, T. W. Aalders, J. A. Schalken, G. J. Adema, P. H. J. Kouwer, M. M. P. Zegers and M. Ansems, Adv. Sci., 2020, 7, 2001797 CrossRef.
- F. Bertucci, P. Finetti and D. Birnbaum, Curr. Mol. Med., 2012, 12, 96–110 CrossRef CAS.
- S. Ye, J. W. B. Boeter, M. Mihajlovic, F. G. van Steenbeek, M. E. van Wolferen, L. A. Oosterhoff, A. Marsee, M. Caiazzo, L. J. W. van der Laan, L. C. Penning, T. Vermonden, B. Spee and K. Schneeberger, Adv. Funct. Mater., 2020, 30, 2000893 CrossRef CAS.
- N. Brandenberg, S. Hoehnel, F. Kuttler, K. Homicsko, C. Ceroni, T. Ringel, N. Gjorevski, G. Schwank, G. Coukos, G. Turcatti and M. P. Lutolf, Nat. Biomed. Eng., 2020, 4, 863–874 CrossRef CAS.
- V. Gkretsi and T. Stylianopoulos, Front. Oncol., 2018, 8, 145 CrossRef.
- I. Jang and K. A. Beningo, Cancers, 2019, 11, 721 CrossRef CAS.
- E. Jabbari, S. K. Sarvestani, L. Daneshian and S. Moeinzadeh, PLoS One, 2015, 10, e0132377 CrossRef.
- L. J. Macdougall, K. L. Wiley, A. M. Kloxin and A. P. Dove, Biomaterials, 2018, 178, 435–447 CrossRef CAS.
- A. Tirella, G. Mattei, M. La Marca, A. Ahluwalia and N. Tirelli, Front. Bioeng. Biotechnol., 2020, 8, 208 CrossRef.
- H. Y. Liu, T. Greene, T. Y. Lin, C. S. Dawes, M. Korc and C. C. Lin, Acta Biomater., 2017, 48, 258–269 CrossRef CAS.
- M. J. Paszek, N. Zahir, K. R. Johnson, J. N. Lakins, G. I. Rozenberg, A. Gefen, C. A. Reinhart-King, S. S. Margulies, M. Dembo, D. Boettiger, D. A. Hammer and V. M. Weaver, Cancer Cell, 2005, 8, 241–254 CrossRef CAS.
- K. R. Levental, H. Yu, L. Kass, J. N. Lakins, M. Egeblad, J. T. Erler, S. F. Fong, K. Csiszar, A. Giaccia, W. Weninger, M. Yamauchi, D. L. Gasser and V. M. Weaver, Cell, 2009, 139, 891–906 CrossRef CAS.
- X. Yue, T. D. Nguyen, V. Zellmer, S. Zhang and P. Zorlutuna, Biomaterials, 2018, 170, 37–48 CrossRef CAS.
- J. T. Kaifi, E. F. Yekebas, P. Schurr, D. Obonyo, R. Wachowiak, P. Busch, A. Heinecke, K. Pantel and J. R. Izbicki, J. Natl. Cancer Inst., 2005, 97, 1840–1847 CrossRef CAS.
- L. R. Patel, D. F. Camacho, Y. Shiozawa, K. J. Pienta and R. S. Taichman, Future Oncol., 2011, 7, 1285–1297 CrossRef CAS.
- R. J. Eddy, M. D. Weidmann, V. P. Sharma and J. S. Condeelis, Trends Cell Biol., 2017, 27, 595–607 CrossRef CAS.
- F. Sabeh, R. Shimizu-Hirota and S. J. Weiss, J. Cell Biol., 2009, 185, 11–19 CrossRef CAS.
- K. Wolf, M. Te Lindert, M. Krause, S. Alexander, J. Te Riet, A. L. Willis, R. M. Hoffman, C. G. Figdor, S. J. Weiss and P. Friedl, J. Cell Biol., 2013, 201, 1069–1084 CrossRef CAS.
- M. Wang, Y. Yang, L. Han, S. Han, N. Liu, F. Xu and F. Li, Biochem. Biophys. Res. Commun., 2020, 528, 459–465 CrossRef CAS.
- A. J. Berger, C. M. Renner, I. Hale, X. Yang, S. M. Ponik, P. S. Weisman, K. S. Masters and P. K. Kreeger, Matrix Biol., 2020, 85–86, 80–93 CrossRef CAS.
- R. S. Stowers, S. C. Allen, K. Sanchez, C. L. Davis, N. D. Ebelt, C. Van Den Berg and L. J. Suggs, Cell. Mol. Bioeng., 2017, 10, 114–123 CrossRef CAS.
- C. Liu, D. Lewin Mejia, B. Chiang, K. E. Luker and G. D. Luker, Acta Biomater., 2018, 75, 213–225 CrossRef CAS.
- C. Liu, B. Chiang, D. Lewin Mejia, K. E. Luker, G. D. Luker and A. Lee, Acta Biomater., 2019, 83, 221–232 CrossRef CAS.
- K. M. Wisdom, K. Adebowale, J. Chang, J. Y. Lee, S. Nam, R. Desai, N. S. Rossen, M. Rafat, R. B. West, L. Hodgson and O. Chaudhuri, Nat. Commun., 2018, 9, 4144 CrossRef.
- K. Chwalek, M. V. Tsurkan, U. Freudenberg and C. Werner, Sci. Rep., 2014, 4, 4414 CrossRef.
- L. J. Bray, M. Binner, A. Holzheu, J. Friedrichs, U. Freudenberg, D. W. Hutmacher and C. Werner, Biomaterials, 2015, 53, 609–620 CrossRef CAS.
- A. V. Taubenberger, L. J. Bray, B. Haller, A. Shaposhnykov, M. Binner, U. Freudenberg, J. Guck and C. Werner, Acta Biomater., 2016, 36, 73–85 CrossRef CAS.
- M. T. Ngo and B. A. Harley, Adv. Healthcare Mater., 2017, 6, 1700687 CrossRef.
- M. T. Ngo and B. A. C. Harley, Biomaterials, 2019, 198, 122–134 CrossRef CAS.
- X. Cui, R. T. Morales, W. Qian, H. Wang, J. P. Gagner, I. Dolgalev, D. Placantonakis, D. Zagzag, L. Cimmino, M. Snuderl, R. H. W. Lam and W. Chen, Biomaterials, 2018, 161, 164–178 CrossRef CAS.
- L. C. Roudsari, S. E. Jeffs, A. S. Witt, B. J. Gill and J. L. West, Sci. Rep., 2016, 6, 32726 CrossRef CAS.
- E. Sahai, I. Astsaturov, E. Cukierman, D. G. DeNardo, M. Egeblad, R. M. Evans, D. Fearon, F. R. Greten, S. R. Hingorani, T. Hunter, R. O. Hynes, R. K. Jain, T. Janowitz, C. Jorgensen, A. C. Kimmelman, M. G. Kolonin, R. G. Maki, R. S. Powers, E. Puré, D. C. Ramirez, R. Scherz-Shouval, M. H. Sherman, S. Stewart, T. D. Tlsty, D. A. Tuveson, F. M. Watt, V. Weaver, A. T. Weeraratna and Z. Werb, Nat. Rev. Cancer, 2020, 20, 174–186 CrossRef CAS.
- C. Sazeides and A. Le, Adv. Exp. Med. Biol., 2018, 1063, 149–165 CrossRef CAS.
- H. Cao, M. K. H. Lee, H. Yang, S. K. Sze, N. S. Tan and C. Y. Tay, Langmuir, 2019, 35, 7487–7495 CrossRef CAS.
- H. Neuwirt, J. Bouchal, G. Kharaishvili, C. Ploner, K. Jöhrer, F. Pitterl, A. Weber, H. Klocker and I. E. Eder, Cell Commun. Signaling, 2020, 18, 11 CrossRef CAS.
- D. Truong, J. Puleo, A. Llave, G. Mouneimne, R. D. Kamm and M. Nikkhah, Sci. Rep., 2016, 6, 34094 CrossRef CAS.
- C. Gaggioli, S. Hooper, C. Hidalgo-Carcedo, R. Grosse, J. F. Marshall, K. Harrington and E. Sahai, Nat. Cell Biol., 2007, 9, 1392–1400 CrossRef CAS.
- D. Rodenhizer, E. Gaude, D. Cojocari, R. Mahadevan, C. Frezza, B. G. Wouters and A. P. McGuigan, Nat. Mater., 2016, 15, 227–234 CrossRef CAS.
- A. Mondal, A. Gebeyehu, M. Miranda, D. Bahadur, N. Patel, S. Ramakrishnan, A. K. Rishi and M. Singh, Sci. Rep., 2019, 9, 19914 CrossRef CAS.
- J. S. McLane and L. A. Ligon, Cancer Microenviron., 2016, 9, 127–139 CrossRef CAS.
- G. Camci-Unal, D. Newsome, B. K. Eustace and G. M. Whitesides, Adv. Healthcare Mater., 2016, 5, 641–647 CrossRef CAS , 626.
- M. Young, D. Rodenhizer, T. Dean, E. D'Arcangelo, B. Xu, L. Ailles and A. P. McGuigan, Biomaterials, 2018, 164, 54–69 CrossRef CAS.
- E. D'Arcangelo, N. C. Wu, T. Chen, A. Shahaj, J. L. Cadavid, L. Huang, L. Ailles and A. P. McGuigan, Biomaterials, 2020, 228, 119572 CrossRef.
- X. Luo, E. L. S. Fong, C. Zhu, Q. X. X. Lin, M. Xiong, A. Li, T. Li, T. Benoukraf, H. Yu and S. Liu, Acta Biomater, 2020 DOI:10.1016/j.actbio.2020.12.037 33388439.
- D. Öhlund, A. Handly-Santana, G. Biffi, E. Elyada, A. S. Almeida, M. Ponz-Sarvise, V. Corbo, T. E. Oni, S. A. Hearn, E. J. Lee, I.I Chio, C. I. Hwang, H. Tiriac, L. A. Baker, D. D. Engle, C. Feig, A. Kultti, M. Egeblad, D. T. Fearon, J. M. Crawford, H. Clevers, Y. Park and D. A. Tuveson, J. Exp. Med., 2017, 214, 579–596 CrossRef.
- M. K. Koch, A. Jaeschke, B. Murekatete, A. Ravichandran, M. Tsurkan, C. Werner, P. Soon, D. W. Hutmacher, L. M. Haupt and L. J. Bray, Acta Biomater., 2020, 114, 256–269 CrossRef CAS.
- E. J. Hoffmann and S. M. Ponik, Front. Oncol., 2020, 10, 787 CrossRef.
- N. B. Hao, M. H. Lü, Y. H. Fan, Y. L. Cao, Z. R. Zhang and S. M. Yang, Clin. Dev. Immunol., 2012, 2012, 948098 Search PubMed.
- Q. Yang, N. Guo, Y. Zhou, J. Chen, Q. Wei and M. Han, Acta Pharm. Sin. B, 2020, 10(11), 2156–2170 CrossRef CAS.
- B. H. Cha, S. R. Shin, J. Leijten, Y. C. Li, S. Singh, J. C. Liu, N. Annabi, R. Abdi, M. R. Dokmeci, N. E. Vrana, A. M. Ghaemmaghami and A. Khademhosseini, Adv. Healthcare Mater., 2017, 6, 1700289 CrossRef.
- M. Court, M. Malier and A. Millet, Biomaterials, 2019, 208, 98–109 CrossRef CAS.
- K. M. Tevis, R. J. Cecchi, Y. L. Colson and M. W. Grinstaff, Acta Biomater., 2017, 50, 271–279 CrossRef CAS.
- Y. Huang, Q. Feng, H. Jiang, W. Zhou, J. Chen, J. Gao, K. Wang, X. Wan and Y. Yu, Int. J. Nanomed., 2020, 15, 4625–4637 CrossRef CAS.
- S. Davidson, M. Efremova, A. Riedel, B. Mahata, J. Pramanik, J. Huuhtanen, G. Kar, R. Vento-Tormo, T. Hagai, X. Chen, M. A. Haniffa, J. D. Shields and S. A. Teichmann, Cell Rep., 2020, 31, 107628 CrossRef CAS.
- F. H. Brasil da Costa, M. S. Lewis, A. Truong, D. D. Carson and M. C. Farach-Carson, PLoS One, 2020, 15, e0230354 CrossRef CAS.
- S. P. Rebelo, C. Pinto, T. R. Martins, N. Harrer, M. F. Estrada, P. Loza-Alvarez, J. Cabeçadas, P. M. Alves, E. J. Gualda, W. Sommergruber and C. Brito, Biomaterials, 2018, 163, 185–197 CrossRef CAS.
- D. M. Pardoll, Nat. Rev. Cancer, 2012, 12, 252–264 CrossRef CAS.
- A. Kalbasi and A. Ribas, Nat. Rev. Immunol., 2020, 20, 25–39 CrossRef CAS.
- K. K. Dijkstra, C. M. Cattaneo, F. Weeber, M. Chalabi, J. van de Haar, L. F. Fanchi, M. Slagter, D. L. van der Velden, S. Kaing, S. Kelderman, N. van Rooij, M. E. van Leerdam, A. Depla, E. F. Smit, K. J. Hartemink, R. de Groot, M. C. Wolkers, N. Sachs, P. Snaebjornsson, K. Monkhorst, J. Haanen, H. Clevers, T. N. Schumacher and E. E. Voest, Cell, 2018, 174, 1586–1598 CrossRef CAS.
- C. M. Cattaneo, K. K. Dijkstra, L. F. Fanchi, S. Kelderman, S. Kaing, N. van Rooij, S. van den Brink, T. N. Schumacher and E. E. Voest, Nat. Protoc., 2020, 15, 15–39 CrossRef CAS.
- E. Pérez Del Río, F. Santos, X. Rodriguez Rodriguez, M. Martínez-Miguel, R. Roca-Pinilla, A. Arís, E. Garcia-Fruitós, J. Veciana, J. P. Spatz, I. Ratera and J. Guasch, Biomaterials, 2020, 259, 120313 CrossRef.
- J. Kim, B. Wu, S. M. Niedzielski, M. T. Hill, R. M. Coleman, A. Ono and A. Shikanov, J. Biomed. Mater. Res., Part A, 2015, 103, 2701–2710 CrossRef CAS.
- J. K. Kim, Y. J. Shin, L. J. Ha, D. H. Kim and D. H. Kim, Adv. Healthcare Mater., 2019, 8, e1801332 CrossRef.
- R. E. Vickman, D. V. Faget, P. Beachy, D. Beebe, N. A. Bhowmick, E. Cukierman, W. M. Deng, J. G. Granneman, J. Hildesheim, R. Kalluri, K. S. Lau, E. Lengyel, J. Lundeberg, J. Moscat, P. S. Nelson, K. Pietras, K. Politi, E. Puré, R. Scherz-Shouval, M. H. Sherman, D. Tuveson, A. T. Weeraratna, R. M. White, M. H. Wong, E. C. Woodhouse, Y. Zheng, S. W. Hayward and S. A. Stewart, Oncotarget, 2020, 11, 3621–3632 CrossRef.
- S. Brassart-Pasco, S. Brézillon, B. Brassart, L. Ramont, J. B. Oudart and J. C. Monboisse, Front. Oncol., 2020, 10, 397 CrossRef.
- O. Chaudhuri, L. Gu, M. Darnell, D. Klumpers, S. A. Bencherif, J. C. Weaver, N. Huebsch and D. J. Mooney, Nat. Commun., 2015, 6, 6364 CrossRef.
- H. Salmon, K. Franciszkiewicz, D. Damotte, M. C. Dieu-Nosjean, P. Validire, A. Trautmann, F. Mami-Chouaib and E. Donnadieu, J. Clin. Invest., 2012, 122, 899–910 CrossRef.
- A. L. Ji, A. J. Rubin, K. Thrane, S. Jiang, D. L. Reynolds, R. M. Meyers, M. G. Guo, B. M. George, A. Mollbrink, J. Bergenstråhle, L. Larsson, Y. Bai, B. Zhu, A. Bhaduri, J. M. Meyers, X. Rovira-Clavé, S. T. Hollmig, S. Z. Aasi, G. P. Nolan, J. Lundeberg and P. A. Khavari, Cell, 2020, 182, 497–514 CrossRef CAS.
- H. W. Jackson, J. R. Fischer, V. R. T. Zanotelli, H. R. Ali, R. Mechera, S. D. Soysal, H. Moch, S. Muenst, Z. Varga, W. P. Weber and B. Bodenmiller, Nature, 2020, 578, 615–620 CrossRef CAS.
- S. Khetan and J. A. Burdick, Soft Matter, 2011, 7, 830–838 RSC.
|
This journal is © The Royal Society of Chemistry 2021 |