DOI:
10.1039/C4AN01874E
(Paper)
Analyst, 2015,
140, 2266-2272
Identification of spectral biomarkers for type 1 diabetes mellitus using the combination of chiroptical and vibrational spectroscopy†
Received
17th October 2014
, Accepted 5th January 2015
First published on 5th January 2015
Abstract
The current diagnostic tools are insufficient for the early detection of many diseases, including type 1 diabetes mellitus. The disease is accompanied not only by a permanently elevated level of blood glucose and altered levels of other biomarkers, but also by changes in the conformation of blood plasma proteins and other biomolecules associated with the pathogenesis of diabetes. However, the observation of these structural changes by conventional Raman and infrared spectroscopy is limited. Therefore, we used chiroptical spectroscopy which is inherently sensitive to the 3D structure of chiral molecules and able to detect any possible structural changes. We investigated the blood plasma samples of diabetic patients and healthy controls by Raman optical activity and electronic circular dichroism. The measurements were combined with conventional methods of molecular spectroscopy, i.e. Raman and infrared spectroscopy. The obtained data sets were statistically evaluated using linear discriminant analysis focusing on the spectral ranges that correspond to the structure and conformation of proteins and other plasmatic biomolecules. Our results suggest that chiroptical spectroscopy gives more detailed information about the 3D structure of biomolecules; and therefore, might be a promising complement to conventional diagnostic methods.
Introduction
Raman and infrared (IR) spectroscopy have been widely tested as powerful tools for medical diagnostics offering a great potential for the real-time analysis of large sample number in the clinical setting.1–3 A number of studies have been primarily focused on the quantitation of clinically relevant biomarkers present in blood, plasma and/or urine (glucose, electrolytes, proteins, lipids, hormones etc.),2–5 or tissue/organ imaging.6,7 However, many pathological processes, such as protein-misfolding diseases, do not significantly alter biomarker levels at their very onset; and by the time they do, it is usually too late to prevent severe complications of the disease.8,9 In some cases, significant changes within the structure of several bodily biomolecules are believed to occur long before alterations of biomarker levels can even be detected.1,9,10 These stereochemical changes cannot be easily monitored using conventional methods of molecular spectroscopy; thus, advanced spectroscopic techniques are necessary. Since many biomolecules in the human body are chiral, chiroptical spectroscopy may be a method of choice. Based on the interaction of circularly polarized radiation with chiral molecules, chiroptical spectroscopy is inherently sensitive to the 3D structure of chiral molecules.11,12 In spite of the ability to detect any possible conformational changes, chiroptical methods have never been used to analyze body fluids; except our previous studies.13–15
In this pilot study, we propose the utilization of Raman optical activity (ROA) and electronic circular dichroism (ECD) of human blood plasma for the diagnostics of type 1 diabetes mellitus (T1DM). The hypothesis of T1DM etiopathogenesis assumes a virus-induced autoimmune inflammation of pancreatic β-cells leading to the production of different signaling molecules (antigens) on the surface of the affected cells. The molecular structure of these newly produced antigens differs from “healthy” proteins; and thus, they are not recognized by the immune system, which results in the destruction of β-cells; and subsequently, in insufficient insulin production and an increasing blood glucose level.16,17 The elevated blood glucose level along with the disruption of the acid–base balance of the body is the main indicator of an ongoing diabetic condition. Blood glucose is routinely measured by placing a drop of blood on a diagnostic strip and using an enzymatic reaction.18 Many experiments have also been conducted in search of a reliable non-invasive diagnostic method based mainly on Raman and IR spectroscopy.19,20 However dealing primarily with glucose or glycated hemoglobin, the research focus is narrow and does not affect all pathological changes within plasma biomolecules that might be worth following. Moreover, some of these changes appear before the disease onset.17 For example, the production of altered antigens begins shortly after the virus attacks the β-cells but before the blood glucose level starts to increase and clinical symptoms occur.16 We believe that focusing on these pre-diabetic alterations in the structure of proteins, carbohydrates and other essential biomolecules in blood plasma might lead to a diagnosis early enough to prevent children and adolescents from life-threatening sudden collapses and severe complications that are inevitably connected with the outbreak of T1DM.
Materials and methods
Blood plasma
For this pilot study, 12 T1DM patients were selected at the Department of Children and Adolescents, Faculty Hospital Královské Vinohrady (FNKV), Prague. Eight first-grade students of the Third Faculty of Medicine, Charles University in Prague were involved in the study as healthy controls. The mean age of the patients and controls was 15.1 and 19.4 years, respectively. Several physiological and biochemical markers related to T1DM were determined for the subjects (Table 1). Due to blood glucose levels within the normal range, the levels of glycated hemoglobin (HbA1c), serum albumin (HSA) or other diabetes-related biomarkers were not necessarily measured in healthy controls.
Table 1 Mean values of significant physiological and biochemical indicators for T1DM patients and healthy controls
Indicator |
BMI |
Blood glucose [mmol l−1] |
HbA1c [mmol mol−1] |
HSA [%] |
T1DM patients |
21.2 |
10.9–11.9 |
75.9 |
53.0 |
healthy controls |
23.3 |
4.0–4.5 |
N/A |
N/A |
Whole blood from all subjects was collected by venipuncture using 9 ml sterile Vacuette® blood collection tubes (Greiner Bio-One GmbH, Kremsmünster, Austria) with K3EDTA (tripotassium salt of ethylenediaminetetraacetic acid) as the anticoagulant agent at the Department of Children and Adolescents, FNKV, Prague. The samples were centrifuged at 1500g and 25 °C for 10 minutes at the Department of Biochemistry, Cell and Molecular Biology, Third Faculty of Medicine, Charles University in Prague. The obtained plasma fractions were frozen immediately and stored at −75 °C. Prior to each analysis, the frozen samples were thawed at room temperature and filtered using centrifugal tubes with a PVDF membrane with 0.45 μm sized pores (Grace, Chicago, IL, USA) at 13
000g and 15 °C for 10 minutes.
The study was carried out according to the principles expressed in the Declaration of Helsinki and approved by the Ethics Committee of the Third Faculty of Medicine of the Charles University in Prague. A written informed consent was secured from all subjects.
Raman spectroscopy and Raman optical activity
The Raman spectra and Raman optical activity were acquired simultaneously on the ChiralRAMAN-2X™ spectrometer (BioTools Inc., Jupiter, FL, USA) equipped with a Laser Quantum OPUS 2W/MPC6000 system (Stockport, UK) with an excitation wavelength of 532 nm. The filtered plasma samples (100 μl) were measured in a 4 × 4 × 10 mm optical cell with an antireflective coating (BioTools Inc., Jupiter, FL, USA), which was placed in a homemade Peltier cell holder for sample temperature control (15 °C). To obtain reliable spectra with the resolution of about 7 cm−1 in the 2500–90 cm−1 spectral region, the parameters of the measurements were as follows: the addition of NaI (10 mg per 100 μl of plasma) as a kinetic fluorescence quencher followed by leaving the sample in the laser beam (280 mW real laser power on the sample) for 12 hours to expedite fluorescence quenching and spectra acquisition (250 mW, 24 h). The illumination period for the measurements was set according to the optimal working range of the CCD detector (1–2.5 s depending on individual samples).13 The real laser power on the sample was monitored by an Optical power meter 1916-R with an 818-P sensor (Newport Corporation, Irvine, CA, USA). To correct the raw Raman/ROA spectra for residual baseline distortion, we modified the procedure described in the literature21 and used a fast Fourier transform (FFT) filter. The measured spectra were highly smoothed to create a virtual baseline, which was subtracted from the raw sample spectrum. Finally, the ROA spectra were smoothed by the FFT filter with a period of ∼10 cm−1.13
Infrared spectroscopy
The IR spectra were recorded on the Nicolet 6700 FTIR spectrometer (Thermo Scientific, USA) equipped with ATR accessory (ZnSe crystal). The filtered plasma samples (30 μl) were measured directly without any additional treatment. Five hundred and twelve scans were performed to create each individual spectrum with the resolution of 4 cm−1 in the mid-infrared region (4000–400 cm−1). Water and water vapor spectra were measured under identical conditions and subtracted from all sample spectra. Eventually, linear baseline correction was performed in the OMNIC 32 program, version 8.2 (Thermo Scientific, USA).
Electronic circular dichroism
The ECD measurements were performed using the J-815 spectrometer (Jasco, Japan) with a Peltier unit set to 23 °C. To allow measurements at lower wavelengths, all filtered samples were diluted (1/3 v/v) with a sterile phosphate buffer (pH = 7.4). The diluted samples (25 μl) were placed into a 0.01 mm quartz cell (Hellma, Germany) and measured in the spectral region of 185–280 nm. Six scans with the resolution of 0.1 nm were accumulated for each sample and averaged in the Spectra Analysis module of the Spectra Manager program, version 2.6.0.1 (Jasco, Japan).
All optical cells and the ATR crystal were cleaned before and after the spectral measurements using a Starna CellClean solution (Starna Scientific Ltd., Essex, UK), rinsed repeatedly with demineralized water and methanol, and dried.
Statistical data evaluation
Using linear discriminant analysis (LDA), the data sets obtained from each spectroscopic method were evaluated in the XLSTAT software (Addinsoft, France). Based on the investigation and maximization of the differences between within-class and between-class distances, LDA classifies the data into groups/classes.22,23 A statistical model was created for the selected spectral bands that may carry crucial information about the molecular structure and its possible changes in proteins and other biomolecules in blood plasma. During LDA, band intensities are normalized; and thus, any intensity change provides essential information. The selection of the particular bands was performed using correlation and covariance matrices. Sensitivity and specificity of the statistical model were established. The leave-one-out cross-validation (LOOCV) was performed to test the statistical model quality. LOOCV is a special case of cross-validation where the number of folds equals the number of instances in the data set. Thus, the learning algorithm is applied once for each instance, using all other instances as a training set and using the selected instance as a single-item test set.24
Results
Raman spectroscopy
In the average Raman spectra of T1DM patients and healthy controls (Fig. 1a), we can recognize three intense bands arising primarily from C
C and C–C stretching vibrations of carotenoids (1007, 1157 and 1520 cm−1) that are present in blood plasma at low concentrations. Their high intensity in the Raman spectra is caused by resonance enhancement due to the excitation in the visible spectral region (532 nm).25–27 The spectra show changes in the intensities of bands that are typical for proteins with a high content of α-helix, specifically the bands at 1654 cm−1 in the amide I region (C
O stretch of the peptide bond), 1285 and 1346 cm−1 in the extended amide III region (in-phase combination of in-plane N–H and Cα–H deformations with C–N stretches) and the bands localized at 879 and 958 cm−1 in the Cα–C, Cα–Cβ and C–N stretching region (skeletal vibrations of the protein/peptide backbone).14,28 In the amide I region of the patient spectra, the less pronounced band at 1654 cm−1 and the significantly decreased intensity of the shoulder at ∼1642 cm−1 indicate lower content of α-helix, which is consistent with the degradation of human serum albumin within the T1DM progress.16,17 Saccharides and lipids present in blood plasma are demonstrated by the bands at 958 cm−1 and 1450 cm−1, respectively.14,29 Their slightly decreased intensities might correspond to diabetic metabolic disruption.
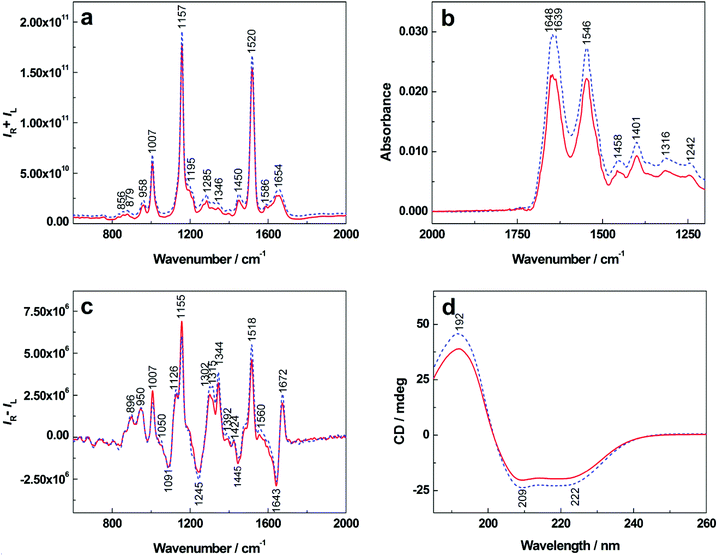 |
| Fig. 1 The average Raman (a), IR (b), ROA (c) and ECD (d) spectra of human blood plasma showing differences between T1DM patients (solid line) and healthy controls (dashed line). | |
Infrared spectroscopy
Fig. 1b shows average infrared spectra containing two significant bands with their maxima around 1648 and 1546 cm−1. The band at 1648 cm−1 occurs in the amide I region and its accurate position and shape are influenced by the type and content of the protein secondary structure.30 The band localized at 1546 cm−1 (amide II) results from in-plane N–H bending and C–N stretching of the peptide bond.31,32 In this case, both of these bands and their relative intensities may indicate alterations in the secondary structure of plasmatic proteins. In the obtained patient IR spectra, we observed a significant decrease in band intensities as well as a change in the amide I/amide II ratio in comparison with healthy controls. Moreover, changes in the shape of amide I band may indicate variations in the secondary structure of plasmatic proteins that occur during T1DM due to protein degradation processes. While the 1639 cm−1 band maintained its position in the IR spectra of patients and controls, the main amide I band at 1648 cm−1 became more structured with a maximum at ∼1652 cm−1 and a low-intensity shoulder at ∼1647 cm−1. Lower intensity was also observed for the bands at 1458 and 1401 cm−1 that correspond to CH2 scissoring and COO− stretching vibrations of aliphatic side chains, respectively.32
Raman optical activity
The average ROA spectra in Fig. 1c demonstrate several bands in the amide I and amide III regions that are typical for proteins with a high content of α-helical structures.14,33 The positive bands (1302, 1315, 1344 cm−1) allow us to distinguish between hydrated and unhydrated α-helices while the negative band (1245 cm−1) corresponds primarily to unhydrated and hydrated β-sheet structures.34 In the spectra of T1DM patients, we observed more pronounced intensity of the negative band at 1245 cm−1 indicating a higher content of β-sheets, which can be explained as the result of albumin unfolding and cleavage during the disease. The shapes of the positive bands at 1302, 1344 cm−1 and a shoulder at 1315 cm−1 represent proteins mainly in the α-helical conformation.34 The relative intensity of the bands at 1302 and 1315 cm−1 in the spectra of healthy controls is equal, while the 1315 cm−1 band disappears in the T1DM patient spectrum. A decreased intensity can also be observed in the band at 1344 cm−1 in the spectrum of T1DM patients. These changes may be interpreted as a result of the degradation of albumin to several intermediates that are used for the synthesis of other essential plasmatic proteins to maintain body homeostasis during T1DM.16,17 The prominent positive bands at 1007, 1155 and 1518 cm−1 can be assigned to carotenoids, some of which overlap with the bands of aromatic amino-acid residues.14
Electronic circular dichroism
The average ECD spectra are shown in Fig. 1d. The simultaneously recorded absorption (Fig. S1 in ESI†) varied between the control group and patients, but the changes were not so significant if compared to ECD in the same region corresponding to protein secondary structures. The ECD spectra are dominated by three distinct bands that are characteristic for the protein secondary structure.11,27 Their shape corresponds with the pattern of proteins with a high content of α-helix,11,35 which are represented mainly by human serum albumin. A positive band at 192 nm and two partially overlapping negative bands at 209 and 222 nm arise from the π→π* and n→π* transitions of amide groups, respectively. The intensities and shapes of these bands vary depending on peptide backbone geometry.14,36 Clearly, intensities of all three bands decreased in the case of T1DM patients, which may have two main causes. First, the values of the total plasma protein decrease while the proteins maintain their native chiral structure. Second, albumin as the negative protein of the acute phase unfolds itself and is cleaved to achiral structures resulting in an increased production of positive inflammatory proteins (α1 and α2-globulins) in order to maintain normal levels of other plasmatic proteins, which is consistent with the pathophysiology of T1DM.16,17
Linear discriminant analysis
Since some of the spectral differences between T1DM patients and healthy controls were barely visible to the naked eye, the obtained data sets were evaluated by means of chemometrics. Our aim was to differentiate T1DM patients and healthy controls (spectral pattern recognition), assess the sensitivity and specificity of the used spectroscopic methods and prove the reliability of the mathematical model.
Fig. 2 shows sample discrimination according to the clinical diagnosis after performing LDA. To emphasize the differences between individual samples, the results are plotted in squared Mahalanobis distances that describe the distance between groups and also the distance of individual group members (samples) from the group center.37 For Raman spectroscopy (Fig. 2a), T1DM patients and healthy controls were separated with an overall accuracy of 75% that decreased to only 45% after LOOCV (Table 2). In the case of IR spectroscopy (Fig. 2b), 90% of the samples were classified correctly. The following cross-validation resulted in 90% accuracy. Although sample separation into two groups was achieved, one control sample remained misclassified. This sample was provided by a sibling of a T1DM patient; thus, the misclassification can be explained as the result of a possible genetic link.17 The LDA of ROA data (Fig. 2c) yielded 85% correct assignments, which led to the formation of two partially overlapping groups of samples. After cross-validating the results, 70% of the samples were discriminated correctly. The partial separation occurred also in the case of ECD (Fig. 2d). In total, 90% of the samples were differentiated properly, leaving 60% overall accuracy after LOOCV.
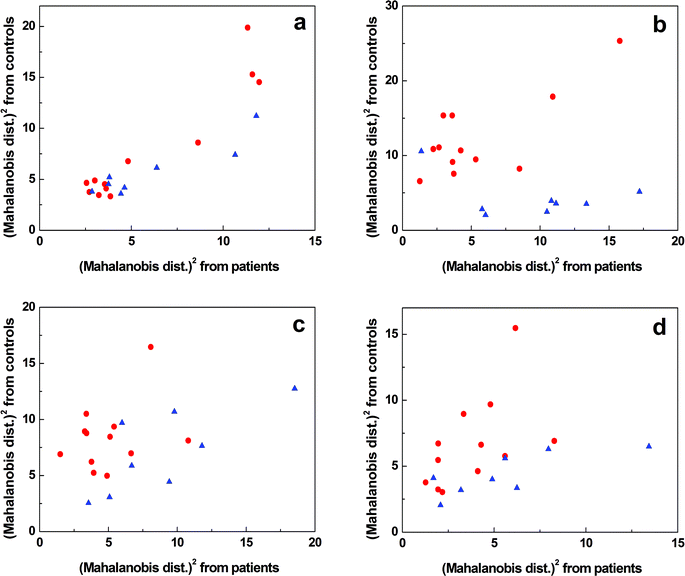 |
| Fig. 2 The graphical representation of the results of linear discriminant analysis for Raman (a), IR (b), ROA (c) and ECD (d) spectroscopy showing the differentiation of T1DM patients ( ) and healthy controls ( ). | |
Table 2 Sensitivity, specificity and overall accuracy values for the estimation sample and leave-one-out cross-validation calculated from LDA
Method |
Estimation sample |
Leave-one-out cross-validation (LOOCV) |
Sensitivity [%] |
Specificity [%] |
Accuracy [%] |
Sensitivity [%] |
Specificity [%] |
Accuracy [%] |
Raman |
83 |
63 |
75 |
58 |
25 |
45 |
IR |
92 |
88 |
90 |
92 |
88 |
90 |
ROA |
92 |
75 |
85 |
75 |
63 |
70 |
ECD |
92 |
88 |
90 |
75 |
38 |
60 |
Combination |
100 |
100 |
100 |
92 |
100 |
95 |
The results were not satisfactory enough for each individual method to classify the plasma samples according to the clinical diagnosis, especially after the cross-validation (Table 2). As the chiroptical methods generally exhibit higher sensitivity to the molecular structure; and thus, provide supplementary information to the conventional Raman and IR spectroscopies, we created a model combining all the four above mentioned spectroscopic techniques (Fig. 3). We observed a complete separation of the group of T1DM patients from the control group and the overall accuracy of sample discrimination was improved to 100%. The specificity and sensitivity of the statistical model were high even after LOOCV; 100% and 92%, respectively, maintaining a high value (95%) of overall accuracy (Table 2).
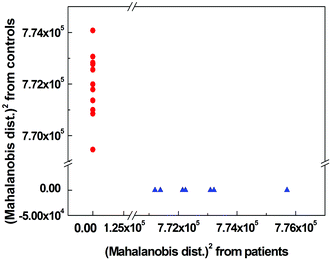 |
| Fig. 3 The graphical representation of the results of linear discriminant analysis for the combination of Raman, IR, ROA and ECD spectroscopic data showing the differentiation of T1DM patients ( ) and healthy controls ( ). | |
Conclusion
We have measured real clinical blood plasma samples by Raman optical activity and electronic circular dichroism and identified spectral regions that are most likely affected by T1DM. Based on our observations, the most significant spectral differences between T1DM patients and healthy controls occurred within the amide regions corresponding primarily to the protein secondary structure that changes during the disease. The subsequent multivariate analysis of spectral data proved that the chiroptical methods are able to detect a more complex signal of plasmatic biomolecules than conventional Raman and IR spectroscopy. In addition, combining ROA and ECD with Raman and IR analyses, we have improved the specificity and sensitivity of sample discrimination after cross-validation to 100% and 92%, respectively. The obtained results suggest that chiroptical spectroscopy may provide appropriate supplementary information to the well-established clinical procedures; and therefore, might become a useful complementary tool for clinical diagnostics and T1DM screening.
Acknowledgements
The work was supported by Specific University Research MSMT (no. 20/2014) – A1_FCHI_2014_003 and A2_FCHI_2014_005, and by the Czech Science Foundation (no. P208/11/0105) and the PRVOUK project No. P31 from the Charles University in Prague. Authors also thank “Operational Program Prague – Competitiveness” (CZ.2.16/3.1.00/22197) and “National Program of Sustainability” (NPU I (LO) MSMT – 34870/2013). The methodology for the measurement of blood-based samples and statistical data analysis was developed thanks to the project no. NT13259-3 by the Ministry of Health of the Czech Republic.
References
-
Biomedical Vibrational Spectroscopy, ed. P. Lasch and J. Kneipp, John Wiley & Sons, Inc., New York, 2008 Search PubMed.
- A. L. Mitchell, K. B. Gajjar, G. Theophilou, F. L. Martin and P. L. Martin-Hirsch, J. Biophotonics, 2014, 7, 153–165 CrossRef CAS PubMed.
- W. Petrich, Appl. Spectrosc. Rev., 2001, 36, 181–237 CrossRef CAS PubMed.
- J. Ollesch, S. L. Drees, H. M. Heise, T. Behrens, T. Bruning and K. Gerwert, Analyst, 2013, 138, 4092–4102 RSC.
- I. Barman, N. C. Dingari, J. W. Kang, G. L. Horowitz, R. R. Dasari and M. S. Feld, Anal. Chem., 2012, 84, 2474–2482 CrossRef CAS PubMed.
- S. Olsztyńska-Janus, K. Szymborska-Malek, M. Gasior-Glogowska, T. Walski, M. Komorowska, W. Witkiewicz, C. Pezowicz, M. Kobielarz and S. Szotek, Acta Bioeng. Biomech., 2012, 14, 101–115 Search PubMed.
- G. Lu and B. Fei, J. Biomed. Opt., 2014, 19, 010901–010923 CrossRef PubMed.
- A. Hye, J. Riddoch-Contreras, A. L. Baird, N. J. Ashton, C. Bazenet, R. Leung, E. Westman, A. Simmons, R. Dobson, M. Sattlecker, M. Lupton, K. Lunnon, A. Keohane, M. Ward, I. Pike, H. D. Zucht, D. Pepin, W. Zheng, A. Tunnicliffe, J. Richardson, S. Gauthier, H. Soininen, I. Kloszewska, P. Mecocci, M. Tsolaki, B. Vellas and S. Lovestone, Alzheimer's Dementia, 2014, 10, 799–807 CrossRef PubMed.
- H. M. Schipper, C. S. Kwok, S. M. Rosendahl, D. Bandilla, O. C. Maes, C. Melmed, D. Rabinovitch and D. H. Burns, Biomarkers Med., 2008, 2, 229–238 CrossRef CAS PubMed.
- D. H. Burns, S. Rosendahl, D. Bandilla, O. C. Maes, H. M. Chertkow and H. M. Schipper, J. Alzheimer's Dis., 2009, 17, 391–397 CAS.
-
Comprehensive Chiroptical Spectroscopy: Applications in Stereochemical Analysis of Synthetic Compounds, Natural Products, and Biomolecules, ed. N. Berova, K. Nakanishi, P. L. Polavarapu and R. W. Woody, John Wiley & Sons Inc., New Jersey, 2012 Search PubMed.
-
L. A. Nafie, Vibrational Optical Activity, John Wiley & Sons, Chichester, 2011 Search PubMed.
- M. Tatarkovič, A. Synytsya, L. Šťovíčková, B. Bunganič, M. Miškovičová, L. Petruželka and V. Setnička, Anal. Bioanal. Chem., 2015 DOI:10.1007/S00216-014-8358-7.
- A. Synytsya, M. Judexová, T. Hrubý, M. Tatarkovič, M. Miškovičová, L. Petruželka and V. Setnička, Anal. Bioanal. Chem., 2013, 405, 5441–5453 CrossRef CAS PubMed.
- M. Tatarkovič, Z. Fišar, J. Raboch, R. Jirák and V. Setnička, Chirality, 2012, 24, 951–955 CrossRef PubMed.
-
D. Levy, Type 1 Diabetes, Oxford University Press, Oxford, 2011 Search PubMed.
-
Diabetes Mellitus: A Fundamental and Clinical Text, ed. D. LeRoith, S. I. Taylor and J. M. Olefsky, Lippincott Williams & Wilkins, Philadelphia, 3rd edn, 2004 Search PubMed.
- C. Bai, T. L. Graham and M. A. Arnold, Anal. Lett., 2008, 41, 2773–2793 CrossRef CAS.
- S. K. Vashist, Anal. Chim. Acta, 2012, 750, 16–27 CrossRef CAS PubMed.
- N. S. Oliver, C. Toumazou, A. E. G. Cass and G. Johnston, Diabetic Med., 2009, 26, 197–210 CrossRef CAS PubMed.
- M. Člupek, P. Matějka and K. Volka, J. Raman Spectrosc., 2007, 38, 1174–1179 CrossRef.
-
R. A. Dunne, in A Statistical Approach to Neural Networks for Pattern Recognition, ed. R. A. Dunne, John Wiley & Sons, Inc., New Jersey, 2006, pp. 19–34 Search PubMed.
-
Z. David, J. Xiao-Yuan and Y. Jian, in Biometric Image Discrimination Technologies: Computational Intelligence and its Applications Series, ed. Z. David, J. Xiao-Yuan and Y. Jian, IGI Global, Hershey, PA, USA, 2006, pp. 41–64 Search PubMed.
-
Encyclopedia of Machine Learning, ed. C. Sammut and G. I. Webb, Springer, New York, 2010 Search PubMed.
- L. Feltl, V. Pacáková, K. Štulík and K. Volka, Curr. Anal. Chem., 2005, 1, 93–102 CrossRef.
- R. Withnall, B. Z. Chowdry, J. Silver, H. G. M. Edwards and L. Oliveira, Spectrochim. Acta, Part A, 2003, 59, 2207–2212 CrossRef.
- S. F. Parker, S. M. Tavender, N. M. Dixon, H. K. Herman, K. P. J. Williams and W. F. Maddams, Appl. Spectrosc., 1999, 53, 86–91 CrossRef CAS.
- M. N. Kinalwa, E. W. Blanch and A. J. Doig, Anal. Chem., 2010, 82, 6347–6349 CrossRef CAS PubMed.
- M. Tsuboi, M. Suzuki, S. A. Overman and G. J. Thomas, Biochemistry, 2000, 39, 2677–2684 CrossRef CAS PubMed.
-
T. A. Keiderling and A. Lakhani, in Comprehensive Chiroptical Spectroscopy: Applications in Stereochemical Analysis of Synthetic Compounds, Natural Products, and Biomolecules, ed. N. Berova, K. Nakanishi, P. L. Polavarapu and R. W. Woody, John Wiley & Sons, New Jersey, 2012, vol. 2, pp. 707–758 Search PubMed.
- P. Carmona, P. Molina, M. Calero, F. Bermejo-Pareja, P. Martínez-Martín, I. Alvarez and A. Toledano, Anal. Bioanal. Chem., 2012, 402, 2015–2021 CrossRef CAS PubMed.
- I. Daidone, M. Aschi, L. Zanetti-Polzi, A. Di Nola and A. Amadei, Chem. Phys. Lett., 2010, 488, 213–218 CrossRef CAS PubMed.
- F. Zhu, N. W. Isaacs, L. Hecht and L. D. Barron, Structure, 2005, 13, 1409–1419 CrossRef CAS PubMed.
- L. D. Barron, F. Zhu, L. Hecht, G. E. Tranter and N. W. Isaacs, J. Mol. Struct., 2007, 834–836, 7–16 CrossRef CAS PubMed.
- J. D. Hirst, K. Colella and A. T. B. Gilbert, J. Phys. Chem. B, 2003, 107, 11813–11819 CrossRef CAS.
- L. Whitmore and B. A. Wallace, Biopolymers, 2008, 89, 392–400 CrossRef CAS PubMed.
- R. De Maesschalck, D. Jouan-Rimbaud and D. L. Massart, Chemom. Intell. Lab. Syst., 2000, 50, 1–18 CrossRef CAS.
Footnote |
† Electronic supplementary information (ESI) available. See DOI: 10.1039/c4an01874e |
|
This journal is © The Royal Society of Chemistry 2015 |
Click here to see how this site uses Cookies. View our privacy policy here.