Detection of Omicron variant in November 2021: a retrospective analysis through wastewater in Halifax, Canada†
Received
27th April 2024
, Accepted 21st June 2024
First published on 28th June 2024
Abstract
This study evaluates the efficacy of wastewater surveillance (WWS) for the early detection of the Omicron variant of SARS-CoV-2 in a university setting in Halifax, Canada. Utilizing an allele-specific RT-qPCR assay, that targets a distinctive Omicron–Lambda mutation (N: P13L; C28311T), we retrospectively analyzed wastewater samples collected from four university residences between 01 September and 31 December 2021. We analyzed 276 passive wastewater samples from four university residences and 51 composite wastewater samples from the wastewater treatment facility (WWTF) which is located downstream of the university. Our findings reveal the presence of the C28311T mutation in wastewater collected before the clinical identification of the Omicron variant in the province. Retrospective analysis of SARS-CoV-2-positive samples using the C28311T RT-qPCR assay showed detections in wastewater collected at the university on 05 November 2021 and 06 November 2021 and in the WWTF samples on 26 November 2021. SARS-CoV-2 N2 RNA was detected in 51 campus samples and 20 treatment facility samples (18 and 39% detection rate, respectively). The study emphasizes the utility of passive sampling for its cost-effectiveness and minimal maintenance, enabling rapid testing and prompt health interventions within an institutional setting. The comparison between the localized approach at the university and the broader community surveillance at the WWTF illustrates the nuanced understanding provided by targeted WWS. While the WWTF samples reflect a community-wide perspective with less variability, the university's targeted surveillance captures localized outbreaks, offering actionable insights for campus management. These findings underscore the strategic value of integrating passive wastewater sampling into public health strategies for variant detection and outbreak prevention, particularly in institutional settings with high-density populations.
Water impact
Wastewater surveillance has emerged as a key tool for monitoring community health during the COVID-19 pandemic. Our study enhances this by employing passive sampling and novel allele-specific RT-qPCR assays for early, localized detection of the SARS-CoV-2 Omicron variant. This approach paves the way for scalable and non-invasive monitoring methods, crucial for timely public health responses and broader surveillance applications.
|
1. Introduction
Response to COVID-19 has become increasingly complex due to the emergence of multiple variants of SARS-COV-2, the virus responsible for COVID-19. These variants emerge through mutations in the virus's genetic material and while many mutations have negligible effects, others significantly increase public health risks.1 In the first two years of the pandemic, the World Health Organization (WHO) identified five key variants of concern (VOCs). Among these, Omicron (B.1.1.529) was first identified in South Africa on 24 November 2021 and was quickly declared a VOC by the WHO on 26 November 2021.2,3 Subsequent investigations revealed Omicron's presence in Europe 10 days before the official identification in South Africa,4 raising questions about its origin and evolution.5 Retrospective analysis of wastewater in France,6 and the Netherlands7 revealed the presence of the Omicron variant in wastewater samples dated back to mid-November of 2021. These findings predate the initial identification of the variant in South Africa, suggesting an earlier-than-anticipated spread of the variant globally. This early circulation is further evidenced by wastewater sequencing in Utah, USA which identified the Omicron variant in samples from 19 November 2021, nearly 10 days before its detection through clinical sequencing. Following the variant's emergence, prevalence escalated across different sewersheds from December 2021 into January 2022, mirroring the uptick in clinically diagnosed cases.8
Omicron is distinguished by its high number of mutations, contributing to its enhanced transmissibility and infectivity.9 At the time, these mutations facilitated a rapid spread of the Omicron variant, leading to significant increases in case numbers globally, despite the ongoing vaccination efforts. The variant's emergence and subsequent dominance highlighted the adaptability of the virus and the persistent challenges in pandemic management,10 including the impact of reduced clinical testing capacities, the prevalence of asymptomatic or mild infections, and general pandemic fatigue, which all complicated efforts to accurately monitor and control the spread of the virus.11
Wastewater surveillance (WWS) emerged as a valuable tool for monitoring SARS-CoV-2 at the population level during the COVID-19 pandemic,12,13 including its use in the detection of VOCs like Omicron in community wastewater.11,14,15 Among the advancements in WWS, passive sampling has emerged as a particularly promising technique.16 Numerous studies have utilized passive samplers containing sorptive materials such as tampons,17,18 cottons buds (q-tips), cotton gauze or cheesecloth,19,20 electrostatically charged membranes,19–23 and, more recently, materials such as polyvinylidene fluoride and granular activated carbon have been reported to detect viruses in wastewater.24,25 Passive sampling offers a simplified, cost-effective approach for monitoring viral RNA in wastewater systems, enabling the capture of representative samples over extended periods without the need for complex infrastructure or frequent manual sampling.16 The adaptability and efficiency of passive sampling make it particularly effective for the timely and sensitive detection of SARS-CoV-2 within localized community settings, serving as an early warning tool that compares favourably with traditional sampling methods.26 Corchis-Scott et al. (2021) highlights the use of tampon-based samplers to monitor COVID-19 cases in a university residence outperformed grab samples.27 Similarly, gauze-based passive samplers targeting wastewater from a hospital admitting COVID-19 patients demonstrated more consistent SARS-CoV-2 detections then grab samples.22 Additional studies have shown passive samplers can provide comparable data to autosamplers26,28,29 and outperform grab sampling methods in detecting viral RNA in wastewater.30,31
While the majority of WWS efforts have focused on collecting samples from wastewater treatment facilities (WWTFs),32,33 targeted surveillance in specific settings such as universities, healthcare facilities, and residential communities can offer valuable insights into the prevalence of current and emerging VOCs.34–38 These settings enable more efficient tracking of incident cases and allow interventions to be implemented promptly upon the detection of the virus in wastewater systems. University campuses present a unique opportunity for monitoring COVID-19 infections due to their geographically diverse student populations, which include individuals who may travel internationally multiple times during their academic programs.
A noteworthy implementation of a campus wastewater monitoring program at Emory University (Atlanta, Georgia) led to the detection of SARS-CoV-2 from campus facilities, which in turn facilitated the identification of previously undetected COVID-19 cases within those premises.39 Likewise, Gibas et al. (2021) illustrated the efficacy of WWS in emergency response at the University of North Carolina, where positive viral signals in campus wastewater prompted a swift action plan.40 Consequently, an overnight lockdown was put into effect within merely 36 hours of sample collection, with students undergoing COVID-19 testing the next morning. The study underscored the high sensitivity of wastewater testing in detecting asymptomatic carriers, effectively identifying the presence of an asymptomatic individual in residential buildings accommodating 150 to 200 students, thus demonstrating the method's effectiveness in uncovering asymptomatic COVID-19 cases. Similarly, the University of Windsor's WWS program effectively prevented an outbreak by identifying the Alpha variant in wastewater samples, building on the demonstrated success of similar initiatives.34 Wright et al. (2022) demonstrated that combining WWS with clinical testing effectively enhances campus health monitoring, evidenced by a significant correlation between SARS-CoV-2 RNA in wastewater and clinical COVID-19 tests on a university campus in the Fall of 2020.41
The objective of this research was to determine the efficacy of passive sampling techniques in WWS for the early detection of SARS-CoV-2 VOCs within a localized population. By leveraging such methodologies, this research seeks to highlight the potential of wastewater surveillance as a non-invasive, comprehensive tool for pre-emptively identifying circulating VOCs, thereby informing public health responses and mitigating community transmission.
2. Methods
2.1. Description of university residence sampling sites
The university campus where sampling was carried out is largely surrounded by residential neighbourhoods and is adjacent to two large teaching hospitals and other health profession departments. Four university residence buildings were selected for wastewater monitoring in this study: residence A, residence B, residence C, and residence D. During the Fall 2021 semester, the capacity of each residence was estimated to be 376, 573, 352, and 261 occupants, for residence buildings A, B, C and D, respectively.42 However, due to COVID-19 precautions, the university reduced its residence capacity to 80% for the 2021/2022 academic year.43 These residences were chosen as part of the University's Health and Safety plan to monitor and detect potential infections within the residence community during the return to campus activities for the Fall 2021 period.44 Passive wastewater samples were collected at the confluence of two sewer lines outside of each residence building. Each sampling point received flow from a sanitary sewer line directly exiting the target building and flow from a stormwater sewer line. Because the sanitary sewer line intersected a stormwater sewer line at the collection point, the viral target in the collected wastewater samples was susceptible to dilution during rain or snow melt events.45 Descriptions of each residence sampling location are provided in the ESI† (Fig. S1).
2.2. Wastewater sample collection
Passive sampling at university residence buildings.
Wastewater samples were collected using a 3D-printed passive sampler containing 90 mm (0.2 μm pore size) electronegative filters.30 Two 47 mm diameter filters (0.1 μm pore size) were used when 90 mm filters were unavailable. Samplers were deployed in each sewershed for durations ranging from 24 and 72 h. Hayes et al. (2022) demonstrated that electronegative filters would achieve close to maximum adsorption capacity for SARS CoV-2 following 24 h of exposure to wastewater.21 The variability in deployment durations was primarily due to the availability of management staff and operators, as well as logistical considerations. However, wastewater systems are dynamic and despite the difference in deployment durations, the data obtained may be considered semi-quantitative, reflecting overall changes in viral detections and concentrations accumulated on the sampler.
Samples were collected at 9:30 AM on each sampling date and processed the same afternoon. Results were available the same evening by approximately 5:00 PM. Generally, wastewater testing results were communicated to the university within eight hours of sample collection. The first positive detection of SARS-CoV-2 RNA in any of the residence building passive wastewater samples resulted in triggering a daily sampling strategy, and following the persistence of SARS-CoV-2 RNA detection (≥3 consecutive days of positive RNA signal) daily wastewater sampling was triggered at all four residence buildings. Following 3 consecutive days of positive wastewater signal the university issued recommendations for more frequent COVID-19 rapid testing for asymptomatic residents.
A passive sampler was deployed at residence A before the study period, in January of 2021. At residence C and residence D, passive samplers were deployed on 05 September 2021 and residence B on 15 September 2021. Throughout the sampling period, the residence WWS strategy involved sample collection three times a week on Mondays, Wednesdays, and Fridays, except when the university was closed. Samples were routinely collected and immediately redeployed at 9:30 AM on the day of collection. For safety, the university's facilities management staff were on-site during sample collection to lift the manhole covers and to ensure safe levels within the sewer catchments using confined space gas monitors. Sampling continued until 23 December 2021, when the university closed for winter break.
Composite sampling at the wastewater treatment facility.
To benchmark campus WWS data, 24 h composite samples were collected by utility personnel from a WWTF in Halifax, Nova Scotia, Canada between 01 September and 31 December 2021. All composite wastewater samples were collected from the influent wastewater stream, post-screening, and pre-grit removal. Samples did not undergo any additional treatment before collection. The 1 L wastewater samples were collected three times per week on Mondays, Wednesdays, and Fridays. Following collection, the 1 L composite wastewater samples were transported to the university laboratory on ice and processed within the same day as sample collection. The processing method for these samples followed the protocol described by Parra-Guardado et al. (2021).46 Specifically, a 50 mL aliquot of raw wastewater influent was centrifuged for 5 minutes to obtain a 500 μL pellet. This pellet was eluted with 2 mL of a Tween®20-based elution buffer, and 1 mL of the eluate was then extracted for RNA using a direct magnetic bead-based extraction method. The WWTF receives flow from a combined sewer network that collects the wastewater from residential and commercial locations, including the university campus buildings. The facility services an area with an estimated 117
000 inhabitants, with a mean influent flow rate of approximately 108
000 m3 per day. A service map of the wastewater treatment catchment area is shown in Fig. S2.†
2.3. Reagents and materials
Deionized (DI) water was produced by a Milli-Q system (Reference A+, Millipore) with a total organic carbon (TOC) concentration <5 μg L−1 and resistivity of 18.2 MΩ cm−1. Electronegative filter membranes (4.7 cm, 0.1 μm or 9.0 cm, 0.2 μm cellulose nitrate membrane filters) and ethanol (EtOH) were purchased from Fisher Scientific (Ottawa, ON, CA). The viral elution buffer was made using Tween®20 and Tris-HCl sourced from Sigma Aldrich (Ottawa, ON, CA). The mixture consisted of 75 μL of Tween®20 and 250 μL of a 0.1 M Tris-HCl intermediate solution added to DI water for a total volume of 100 mL. Magnetic binding beads (50 g L−1), RNA isolation kits, and SARS-CoV-2 assay kits were obtained from LuminUltra Technologies Ltd (Fredericton, NB, CA). Bovine serum albumin (BSA) used to make a 1 mg mL−1 solution (10 mg lyophilized BSA in 10 mL DI water) was obtained from Alfa Aesar by ThermoFisher Scientific (Tewksbury, MA, US). All primers to detect the C28311T mutation associated with the Omicron variant were purchased through Integrated DNA Technologies (IDT, Coralville, IA, USA), and the TaqMan MGB probe was purchased from ThermoFisher Scientific (Burlington, ON, CA).
2.4. RNA extraction
All passive samples were eluted using 6 mL of 0.075% Tween®20 + 25 mM Tris HCl-based buffer; 1 mL of this eluate was used for subsequent RNA extraction. RNA extraction of influent wastewater and passive sampler filter eluate was carried out using a magnetic bead-based RNA extraction methodology described by Parra-Guardado et al. (2021) and Hayes et al. (2021), respectively.30,46 Briefly, a 1 mL aliquot from either sample type (composite sample solids pellet or filter tween-based eluate) was used to perform the extraction protocol according to the manufacturer's instructions (LuminUltra Technologies Ltd). For particularly soiled passive samples, the sampler eluates would be diluted 1
:
2 with nuclease-free water (NFW) to mitigate potential inhibition of RNA extraction or RT-qPCR analysis. Soiled eluates were identified based on visual inspection for excessive particulate matter. The extracted RNA (50 μL) was processed using RT-qPCR. Samples from the WWTF were extracted in duplicates. As the volume of passive sampler eluates was only 6 mL, samples were extracted in single aliquots to conserve raw eluates for subsequent analyses.
2.5. Molecular detection methods
SARS-CoV-2 N2-Gene RT-qPCR assay.
All RNA samples for the monitoring program, including those from passive samplers and the WWTF, were processed by RT-qPCR on a GeneCount® Q-96 instrument (LuminUltra Technologies Ltd, Fredericton, CA). The probe and primer sequences for targeting the SARS-CoV-2 N2 gene used in this study are shown in Table 1. The 20 μL reactions were prepared using the GeneCount SARS-CoV-2 Screening kit (LuminUltra Technologies Ltd, Fredericton, CA), containing 15 μL of Master Mix and 5 μL of template RNA. The RT-qPCR Master Mix utilized for the N2 assay contains MS2 bacteriophage as an internal amplification control (IAC). Thermocycling conditions were performed as follows: 15 min at 50 °C, 2 min at 95 °C, and 45 cycles of 10 s at 95 °C and 45 s at 55 °C; and a final hold step for 45 s at 55 °C. All RT-qPCR analyses performed in the GeneCount® Q-96 instrument included at least two no-template control (NTC) containing NFW. Each sample was analyzed at a single RNA dilution; if the first aliquot resulted in a non-detect, a second dilution was analyzed to minimize the likelihood of false negatives due to inhibition during analysis.
Table 1 RT-qPCR oligonucleotides for SARS-CoV-2 detection, including Omicron–Lambda allele-specific primer extension RT-qPCR assay targeting the N: P13L: C28311T mutation, the SARS-CoV-2 N2 gene, Alpha (B.1.1.7) and Delta (B.1.617) variant mutations. Each oligonucleotide is provided with its specific sequence and designated working concentration in nanomolar (nM). All primers and probes were purchased through Integrated Technologies (IDT, Coralville, IA, USA) and stored based on manufacturer's recommendations
Item |
Sequence type |
Conc. (nM) |
Sequence (5′–3′) |
C28311T |
Probe |
500 |
CCAAAATCAGCGAAATGAACT |
RP |
500 |
TCTGGTTACTGCCAGTTGAATCTG |
FP |
125 |
CCGCATTACGTTTGGTGGACCC |
N2 |
Probe |
667 |
TTACAAACATTGGCCGCAAA |
RP |
667 |
GCGCGACATTCCGAAGAA |
FP |
167 |
ACAATTTGCCCCCAGCGCTTCAG |
Delta (B.1.617) |
Probe |
200 |
TGGATGGAAAGTGGAGTTTATTCTAGT |
RP |
500 |
GGCTGAGAGACATATTCAAAAGTG |
FP |
500 |
GTTTATTACCACAAAAACAACAAAAG |
Alpha (B.1.1.7) |
Probe |
125 |
ACCCCGCATTACGTTTGGTGGACC |
RP |
500 |
TCTGGTTACTGCCAGTTGAATCTG |
FP |
500 |
CATCTAAACGAACAAACTAAATGTCTCT |
A positive control, four-point standard curve (102–105 copies per μL) was carried out using a plasmid containing the target N genes for SARS-CoV-2 (IDT, Coralville, IA, USA) and a serial 10-fold with NFW (∼2 × 105 copies per μL). Plasmid control was prepared and stored based on manufacturer recommendations. All points on the curve were run in duplicate and averaged to create one standard curve used to calculate copies per mL through cycle quantification (Cq) values. The efficiency of the N2 assay standard curve was ∼96%, with an R2 value of 0.99 and y-intercept of ∼38.4.
SARS-CoV-2 C28311T mutation RT-qPCR assay.
Retrospective analysis of RNA samples was carried out for the detection of the C28311T allele frequency, a single nucleotide variant specific for both Lambda and Omicron VOCs using a GeneCount® Q-96 instrument (LuminUltra Technologies Ltd, Fredericton, CA).14 The C28311T RT-qPCR assay was performed using an allele-specific forward primer and probe combined with the CDC N1 reverse primer.47 The RT-qPCR assay employed in this study is founded on a primer extension approach, specifically designed for the detection of the SARS-CoV-2 Omicron variant C28311T mutation in wastewater samples. The C28311T RT-qPCR assay targets a distinctive mutation (N: P13L; C28311T) of the Omicron variant in the N1 amplicon region, observed in the B.1.1.529 genomes deposited in GISAID in December 2021.48 The single nucleotide variant, C28311T, during the time of this study, was present in >97% of B.1.1.529 (Omicron; BA.1 and BA.2) and C.37 (Lambda) GISAID-deposited sequences and found in <0.5% of other sequences. Importantly, Lambda VOC prevalence in Canada throughout the pandemic was <0.01% (32/239,025 GISAID-deposited sequences as of 12 January 2022).
The sequences of the primers and probes, as well as their working concentrations, for the C28311T RT-qPCR assay are shown in Table 1. All C28311T RT-qPCR reactions were prepared using 3 μL of RNA template in a final volume of 20 μL. The thermocycling parameters were carried out as follows: 5 min at 50 °C, followed by 20 s at 95 °C, and 45 cycles of 3 s at 95 °C and 45 s at 55 °C. All samples were assessed in technical duplicates, each run at two separate RNA dilutions for a total of four reactions per sample. A 6-point standard curve (106–101 copies per μL) was generated from Twist synthetic SARS-CoV-2 RNA control 48 (B.1.1.529/BA.1) for C28311T analysis. The standards were prepared in single-use aliquots through a 10-fold dilution series of the stock solution (∼1 × 106 copies per μL). The standard curve had an R2 value of 0.99, an efficiency of 96% and a y-intercept of 39.1. A total of 70 N2-positive and 142 randomly selected N2-negative samples across all sampling sites between 01 September and 31 December 2021 were analyzed using the C28311T assay.
SARS-CoV-2 Alpha (B.1.1.7) and Delta (B.1.617) RT-qPCR assays.
Here, we describe the use of allele-specific RT-qPCR strategies to target mutations in the N gene of SARS-CoV-2 to allow for quantification of mutations associated with the Alpha (B.1.1.7) and Delta (B.1.617) variants of concern. These assays are capable of discriminating single nucleotide variants in wastewater samples and, therefore, were employed to determine the presence or absence of the these variants and distinguish between the presence of these other strains in samples that were positive using the Omicron (C28311T) assay. The Delta variant was chosen as it was the predominant VOC in Canada before the rapid spread of Omicron in November 2021.49 The Alpha and Omicron variants share a common mutation: the deletion of the S gene del (69–70).50 However, the Alpha variant has had a <1% presence in Canada as of 14 November 2021.49 Therefore, assessing the Alpha variant through specific targeting of a mutation independent of the Omicron variant (i.e., the D3L mutation, as used in this work) allowed us to further rule out the potential presence of the variant in our samples.
The Alpha RT-qPCR assay was carried out based on previous work by Graber et al., (2021) who implemented an allele-specific B.1.1.7 allele (D3L) RT-qPCR assay using a recently designed forward primer combined with the N1 probe and reverse primer (Table 1).51 The D3L forward primer used for this work incorporates a deletion mutant (A28271Del) which has been previously described as the dominant single nucleotide variant in the B.1.1.7 lineage. The paired non-B.1.1.7 alleles (D3) assay was not performed in this work, as the work was implemented to confirm the absence of the B.1.1.7 lineage. RT-qPCR reactions were prepared with TaqMan® Fast Virus 1-Step Master Mix (ThermoFisher, Ottawa, Canada) in duplicate reactions, each with 1.5 μL of RNA template in a final reaction volume of 10 μL. Thermal cycling conditions were as follows on the LuminUltra GeneCount® Q96, RT at 50 °C, 5 min, followed by polymerase activation and template denaturation at 95 °C for 20 s, and 45 cycles of denaturation (95 °C for 3 s), then annealing/extension (55 °C for 45 s). Each sample set analyzed on the RT-qPCR was run with at least two positive and negative controls. Only if the negative and positive controls passed (i.e., Cq values >40 and 38, respectively) were the sample results considered acceptable. The positive control (103 copies per μL) and standard curve utilized a synthetic B.1.1.7 RNA template (GISAID accession ID EPI_ISL_710528, Twist Biosciences); an average positive control Ct value of 30.4 was observed. The standard curve assay was performed with a six-point (101–106 copies per μL) serial dilution of the B.1.1.7 RNA template using NFW. Efficiencies, linearity, and y-intercepts were ∼91%, 0.99 and 38.6 respectively for the D3L standard curve.
RT-qPCR for the Delta variant (B.1.617) mutation was carried out on all RNA positive for N2 from 01 September to 31 December 2021 at the university residence and the region WWTF sites. The RT-qPCR assay was performed based on the workflow described by Yaniv et al. (2021),52 each reaction contained 5 μL of RNA sample with 5 μL TaqMan® Fast Virus 1-Step Master Mix (ThermoFisher, Ottawa, Canada), 7.6 μL of nuclease-free water, and 0.5 μM of each forward and reverse primer, and 0.2 μM of probe for a total of 20 μL solution mix (Table 1). Reactions were performed on the LuminUltra GeneCount® Q96 instrument under the following thermal cycling parameter, 5 min at 50 °C followed by 20 s at 95 °C for reverse transcription and 40 cycles were performed at 95 °C for 3 s followed by 30 s at 60 °C. 60 °C for annealing and amplification (30 s). All samples, standards and controls were performed in technical duplicates and the high concentration between replicates was reported as not all replicates were detected for each sample. Nuclease-free water served as a negative control in each RT-qPCR reaction. A known-positive DNA gene block template (103 copies per μL) functioned as a positive control (Table S1†). Sample results were only considered valid if negative and positive controls passed (i.e., Cq values >38 and <37, respectively). Using the DNA gene block template positive control, a six-point (101–106 copies per μL) standard curve was generated. Through linear regression of RT-qPCR resulting cycle threshold values plotted against log copy numbers the amplification efficiency was determined to be ∼92%, with an R2 value of 0.99 and a y-intercept of 40.88.
2.6. Quality control
Samples were analyzed for N2 gene detections via RT-qPCR directly after sample RNA was extracted. Following RT-qPCR analysis, RNA samples were stored at −80 °C for up to two months before being retrospectively analyzed for C28311T. Extraction blanks were included during RNA extraction to assess contamination during sample processing. All extraction blanks presented no detectable levels of SARS-CoV-2 RNA. Furthermore, to minimize contamination during sample processing, RNA extractions and RT-qPCR analyses were performed in separate laboratories, each equipped with a certified biosafety cabinet. NTCs were implemented into each RT-qPCR assay, and a failed NTC (Cq value ≤38) resulted in a re-analysis of all samples in the respective run. To alleviate inhibition, each sample was diluted up to three times (1
:
1, 1
:
5 and 1
:
10) using a BSA solution (1 mg mL−1). An additional criterion for the acceptance of SARS-CoV-2 results was the passing of the internal amplification control (MS2 bacteriophage, acquired from LuminUltra Technologies Ltd). Amplification controls were used to validate successful amplification in the RT-qPCR reaction, thereby preventing false negative results that may be caused by inhibitory compounds. However, under conditions where the IAC failed, samples that were non-detect for SARS-CoV-2 were re-analyzed by RT-qPCR with RNA dilutions. No contamination was observed during the study period at any of the sampling locations.
Standards outlined in minimum information for publication of quantitative real-time PCR experiments (MIQE) guidelines53 and environmental microbiology minimum information (EMMI) guidelines54 were referenced for evaluating RT-qPCR-based tests (Table S2†). Parra-Guardado et al. (2021) reported the experimentally determined method limit of detection (MLOD) of the N2 RNA extraction protocol for heat-inactivated SARS-CoV-2 spiked in wastewater as 5 × 101 gene copies (GC) per mL and observed N2 RNA concentrations from field samples as low as 1.7 Gc mL−1.46 The SARS-CoV-2 RNA concentration of 1.7 GC mL−1 observed by Parra-Guardado et al. (2021) was selected as the MLOD for all WWTF composite wastewater samples in this study.46 The recovery efficiency of the wastewater processing and RNA extraction protocols were determined to be ∼87% by Parra-Guardado et al. (2021).46 For wastewater collected via passive samplers, a SARS-CoV-2 RNA concentration of 89 GC mL−1, as experimentally determined by Hayes et al. (2022),21 was designated as the MLOD for this study. The RNA concentrations below these MLODs were considered non-detects. A process control to account for varying RNA extraction efficiencies was not utilized in this study, as there are no established process controls known which exhibit similar adsorption characteristics to SARS-CoV-2 for passive sampling experiments using electronegative filters. As well, considering the small catchment areas of the university residence sampling sites in this study, the use of a fecal indicator was not implemented. Small catchment areas experience significant daily fluctuations, making normalization of SARS-CoV-2 signals using fecal indicators (e.g., PMMoV) ineffective in mitigating viral signal variability.55,56 These indicators exhibit location-specific variability and exhibit fluctuations due to the influence of individual dietary patterns. However, fecal indicators can still be useful for checking sample integrity and extraction performance, helping to identify potential outliers.
2.7. Statistical analysis and data reporting
All composite wastewater samples collected from the WWTF were analyzed for SARS-CoV-2 N2 and C28311T RNA in two biological replicates, while samples collected via passive sampling were processed as single aliquots. Two technical replicates were analyzed for each extracted RNA sample. The mean target RNA concentration from the technical duplicates was reported for all sample types analyzed. RNA concentrations obtained from the WWTF samples are reported as GC mL−1 of the 1 L composite wastewater sample collected and were calculated using eqn (1) based on the methods outlined in Parra-Guardado et al. (2021).46 For samples collected from passive samplers, RNA concentrations are reported as GC mL−1 of the 6 mL sampler eluate and were calculated using eqn (2).30 Plots were generated using GraphPad Prism version 4.00 for Windows (GraphPad Software, San Diego, California US) and RStudio (version 4.2.3), utilizing packages such as tidyverse, scale, and ggtext.57–59 A two-sample Welch's t-test assuming unequal variances was performed to compare the slopes of the linear trends in RNA concentrations between the N2 and C28311T targets. |  | (1) |
|  | (2) |
3. Results & discussion
3.1. University action plan for campus wastewater monitoring program
During the Fall 2021 academic semester, the university implemented a multifaceted public health strategy to ensure campus safety, which included on-campus vaccination, a comprehensive WWS program, and an extensive asymptomatic rapid antigen testing initiative.60,61 Tailored to university settings, this strategy aimed to mitigate the spread of COVID-19 on campus.
As part of this strategy, between 01 September and 31 December 2021, 272 passive wastewater samples from four campus residences, alongside 53 composite samples from the downstream WWTF, were analyzed. Notably, SARS-CoV-2 RNA (N2 gene) was detected in 51 samples from the university residences and 20 WWTF samples (Table 2).
Table 2 Summary of passive and composite wastewater samples collected from 01 September 2021 to 31 December 2021, at the university residences (passive) and the WWTF (composite), along with the count of SARS-CoV-2 N2 RNA detections observed at each sampling site
Month |
Residence A |
Residence B |
Residence C |
Residence D |
Total passive |
WWTF |
Passive |
Passive |
Passive |
Passive |
Composite |
Sept |
0/12 |
0/6 |
0/10 |
0/10 |
0/38 |
0/13 |
Oct |
1/16 |
6/15 |
0/16 |
1/16 |
8/63 |
7/13 |
Nov |
11/24 |
1/25 |
0/25 |
0/25 |
12/99 |
5/13 |
Dec |
11/20 |
15/19 |
5/18 |
0/15 |
31/72 |
8/14 |
Total |
23/72 |
22/65 |
5/69 |
1/66 |
51/272 |
20/53 |
Fig. 1 demonstrates the consecutive detections in passive wastewater samples collected from sewershed locations outside of the university residence A and residence B, marked by red and blue dotted lines, respectively. The sudden increase of detections at residence A and B during October and November 2021 coincides with the return of students post-summer break, while a following increase in December points to an extended phase of transmission within the university dormitory community, likely exacerbated by increased indoor social interactions due to colder weather. The detection clusters of the N2 gene in November and December of 2021 played a crucial role in activating the university's rapid response protocols. The initial detections at residence A and residence B prompted immediate, campus-wide testing efforts, leading to the identification of six COVID-19 cases.62 This underscores the effectiveness of WWS in supporting public health actions by providing early warnings of potential outbreaks.
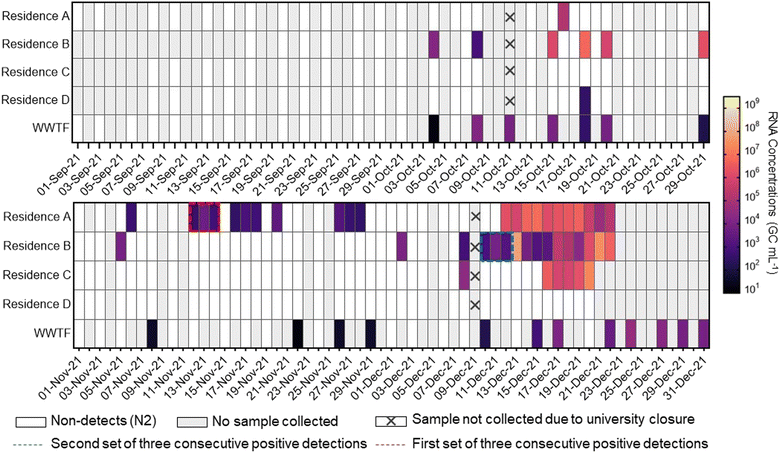 |
| Fig. 1 Time series of SARS-CoV-2 (N2 Gene) RNA concentrations in wastewater from the four university residences and the local WWTF (01 September–22 December 2021). Red and blue dotted lines highlight consecutive detections at residence A and residence B, respectively. The MLODs were ∼89 GC mL−1 for passive samples and ∼1.7 GC mL−1 for composite samples. | |
In response to the increased viral load detected in the residence wastewater samples, where three or more consecutive viral detections were observed in November and December 2021, the university launched a targeted communication and testing strategy. The university reported to the communities of each residence, targeting all student residents and support staff, including those in residence life, operations, custodial, security, and food services. Rapid antigen test kits were made widely available in residence lobbies and other strategic high-traffic areas on campus, with support personnel providing guidance and clear instructions for follow-up actions. These efforts were instrumental in identifying an additional 23 COVID-19 cases by 17 December 2021,63 highlighting the importance of integrated surveillance and response strategies in managing potential outbreaks.
Throughout the semester, the campus completed 2025 in-person COVID-19 tests and distributed approximately 108
929 self-tests,64 alongside the collection of 273 passive wastewater samples from sewersheds directly outside the four university residence buildings. This integrated approach, featuring WWS as a complementary strategy to other COVID-19 mitigation strategies, mirrors the strategies employed by numerous institutions worldwide.34,40,65–67 Other studies, such as those by Betancourt et al. (2021) and Gibas et al. (2021), emphasize the critical role of sewer sampling within university residences in identifying clusters for targeted testing and facilitating early isolation to prevent outbreaks.40,65 However, it is important to acknowledge that the efficacy of WWS cannot be merely evaluated by the number of detected cases or the speed of outbreak detection. Various factors, including campus density, the proportion of remote versus in-person participation, and adherence to public health guidelines, may significantly influence the overall effectiveness of any strategy. At the University of Notre Dame, the introduction of tampon-based passive samplers for building-level WWS underscored the complexity of such interventions. The study highlighted limitations including methodological performance variability across different wastewater sources and the potential for non-resident RNA shedding, complicating data interpretation.17 Likewise, researchers at the University of Calgary, reported that the younger demographic's higher asymptomatic rates and voluntary case reporting further complicated the efficacy of WWS, emphasizing the critical role of campus dynamics and public health compliance in shaping the outcomes of these surveillance efforts.68
3.2. Retrospective Omicron detection in wastewater by allele-specific RT-qPCR
Following the initial detection of Omicron cases in Nova Scotia on December 13, 2021,69 we conducted a focused retrospective analysis on 71 wastewater samples that had previously tested positive for the SARS-CoV-2 N2 gene in attempts to detect the Omicron C28311T mutation using an allele-specific RT-qPCR assay. The singleplex single nucleotide variant RT-qPCR assay is sensitive to a single nucleotide variation in the N gene, indicative of the N: P13L non-synonymous amino acid change, a mutation characteristic of the SARS-CoV-2 Omicron variant.70 This retrospective analysis confirmed the presence of the C28311T mutation in 46/71 wastewater samples collected from university residences A and B and WWTF locations during the study sampling period (Fig. 2). Mean RNA concentrations measured were approximately 1.1 × 107 GC mL−1 for residence A, ∼3.0 × 106 GC mL−1 for residence B, and 1.3 × 106 GC mL−1 for the WWTF. The frequent detection of the C28311T mutation in these wastewater samples indicates that there was possibly a substantial presence of the Omicron variant across the sampled locations. This is supported by research that has demonstrated wastewater to effectively capture the emergence and dominance of the Omicron variant, aligning with epidemiological projections.71
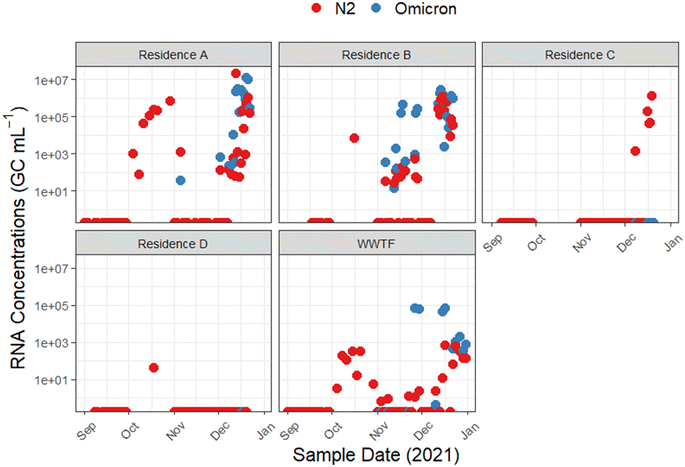 |
| Fig. 2 Time series of SARS-CoV-2 N2 (red) and Omicron C28311T (blue) gene copies in wastewater from September 2021 to January 2022. Passive samples from four university residences and composite samples from a WWTF are displayed. Sampling dates are shown on the x-axis, while the y-axis presents the average concentration in GC mL−1. | |
Importantly, all 142 N2-gene negative samples, collected from 01 September and 31 December 2021, lacked the C28311T mutation, suggesting that the absence of the mutation is consistent with the negative N2 gene results and the absence of this mutation circulating during this period. Additional RT-qPCR analysis for other VOCs using identified no Alpha (D3L mutation) or Delta (Δ157-158 mutation) variants in Omicron-positive samples from the university residences. In contrast, 5/20 of the N2-positive samples from the WWTF showed the Δ157-158 mutation, highlighting variant diversity in the broader community.
Fig. 2 illustrates the temporal distribution of SARS-CoV-2 N2 RNA and C28311T RNA detections in passive wastewater samples from four university residences and the influent stream of a downstream WWTF, spanning from 01 September 2021 to 31 December 2021. The initial detections of the C28311T mutation in wastewater predated the first clinically confirmed Omicron cases in the region on 13 December 2021,72 with early C28311T detections emerging from residence A on 5 November 2021 (average RNA concentration ∼3.6 × 101 GC mL−1), and then at residence B the following day, 6 November 2021 (average RNA concentration ∼4.9 × 102 GC mL−1). The first C28311T detection at the WWTF was not observed until 26 November 2021 (RNA concentration ∼7.0 × 104 GC mL−1). Throughout the study, periods of non-detects followed by clusters of SARS-CoV-2 RNA detections were observed at the WWTF for both C28311T and N2 target genes. As well, the variance between SARS-CoV-2 N2 and C28311T RNA concentrations at the university residences compared to the WWTF highlights the influence of different sampling scales and methods. In this study, composite wastewater samples from the WWTF generally showed more gradual fluctuations in viral concentrations over time compared to the university residences. However, the university residences exhibited significantly higher RNA concentrations for more N2 and C28311T. For instance, the WWTF samples displayed a maximum C28311T RNA concentration of ∼9.1 × 104 GC mL−1 on 15 December 2021, with more consistent and gradual changes observed throughout the sampling period. In contrast, residence A showed a surge in C28311T RNA concentrations with peaks reaching up to ∼2.1 × 107 GC mL−1 on 20 December 2021, while residence B displayed a peak RNA concentration of ∼3.0 × 107 GC mL−1 on 14 December 2021. This pattern can be attributed to greater dilution effects and population heterogeneity often noted at WWTF compared to smaller catchment sampling.73 Whereas, the passive samplers deployed at the university residences adeptly capture transient surges in viral load, which may be indicative of acute localized outbreaks that may be missed when sampling at WWTFs.74,75 However, the variations in RNA concentrations across sampling sites may also reflect differences in population density, infection rates, or sampling methodologies. Nonetheless, the contrast in detections between sampling locations underscores the importance of sampling resolution in WWS, suggesting that passive sampling at a granular level may be critical for early outbreak detection and targeted public health responses.
The peak C28311T RNA concentration at residence A, on 21 December 2021, suggests the variants escalating presence on campus since its initial detection in early November 2021. This trend suggests not only the rapid spread of the variant but also the possibility of its sustained presence on campus since early November 2021. However, the marked increase in RNA levels in December 2021 may reflect a more cumulative effect of widespread exposure among residents, compounded by the prolonged shedding of viral RNA in wastewater after infection, resulting in persistently high viral loads in the residence's wastewater.76 The Omicron variant has been characterized to have a lower minimal infective dose compared to the wild-type SARS-CoV-2 leading to potentially more rapid transmission and widespread infection.77 Champredon et al. (2024) observed that the Omicron variant's spread in Canada took less than a month to become dominant, compared to the three to four months required for the Delta variant to become the prevalent strain.78
The temporal distribution of SARS-CoV-2 detections offers valuable insights into the potential epidemiological trends on campus and the surrounding community during the sampling period. The episodic fluctuations in viral RNA concentrations at the university residences align with potential individual cases or more widespread outbreaks on campus. For example, residences A and B observed an increase in N2 gene detections in October 2021, possibly coinciding with the influx of the student population returning to campus in September 2021. However, during this period, detections of the C28311T allele were absent in all wastewater samples, suggesting the predominance of other SARS-CoV-2 strains at this time. Conversely, residence C and D presented the fewest number of N2 detections and no C28311T detections, possibly due to effective containment measures or low residence occupancy protocols. The absence of detections at residences C and D may be attributed to differences in student population infection rates among the residents, and varying levels of adherence to health protocols. While the number of students was unknown in each residence during the time of study, the university's COVID-19 protocols at the time limited guests in the residences.
While the C28311T RNA concentrations at the WWTF began to rise sharply in late November 2021 into December 2021, the N2 RNA concentrations showed a more gradual increase during this period. Statistical analysis comparing the slopes of the linear trends for RNA concentrations (p = 0.00021) confirmed that the increase in C28311T RNA concentrations was significantly faster than that of N2 RNA concentrations during this sampling period. Discrepancies between the C28311T detections and N2 detections may be a reflection of the rapid emergence and spread of the C28311T mutation, or the technical nuances between these RT-qPCR assays impacting their sensitivity. For instance, the N2 assays sensitivity may have been impacted by the multiplexing of the assay with an additional target for internal amplification control.79 We also noted that inhibitors inherent to wastewater impacted the assays differently, where the N2 assay often required template RNA to be diluted to 1
:
10 during RT-qPCR reactions to alleviate inhibition, whereas the C28311T assay required no dilution or only a 1
:
1 dilution. Additionally, there is potential for the N2 assay to reflect an N gene dropout effect, where additional mutations may lead to decreased sensitivity or failure of the assay.80 While there are no specific reports of the C28311T mutation causing an N2 dropout, the high mutation rate in the N gene and the documented impact of other mutations suggest that it may be a plausible concern. These factors highlight the importance of using a multi-target RT-qPCR approach to ensure comprehensive detection of evolving variants and to mitigate the risk of false negatives. A limitation of this study was that additional SARS-CoV-2 targets (e.g., N1 gene) were not evaluated, which could provide additional insights into the detections observed in this work. Furthermore, the absence of public clinical data for the catchment area limited a more granular analysis of the fluctuating viral signals observed in this study. However, the utility of integrating WWS data with broader epidemiological information is evident. Such integration would be vital for crafting targeted interventions in the future, specifically during the academic year's beginning and end, when population densities and social behaviours may change.
3.3. Significance of C28311T detection and other VOC-associated mutations in wastewater
The findings from this WWS program indicate the presumptive presence of the Omicron variant, based on the detection of the C28311T mutation in Nova Scotia as early as 5 November 2021. This detection notably precedes the initial identification of the Omicron variant in South Africa on 24 November 2021.2,3 However, it is important to recognize that the C28311T mutation targeted in this study is also associated with the SARS-CoV-2 Lambda variant. Although, the presence of the Lambda variant was low in Canada (<0.01%, 32/239025 GISAID-deposited sequences as of 12 January 2022) during the study period,81 the possibility of residual circulation of Lambda or other non-named variants that share this mutation cannot be entirely excluded. Therefore, without confirmatory sequencing data the C28311T mutations observed in this work should be considered presumptive for the presence of the Omicron variant.
The absence of the Alpha and Delta variants in the university residence wastewater aligns with observations by Lee et al. (2021), who identified the predominance of a singular SARS-CoV-2 strain within an institutional context during their study period.35 Similarly, research conducted at the University of Cambridge, UK, demonstrated that the vast majority of SARS-CoV-2 genomes sequenced from student samples were part of a single genetic lineage.82 This was attributed to cases arising from a singular event, such as a social gathering off-campus, indicating a constrained entry of the virus into the campus community.
Retrospective wastewater sample analyses have been instrumental in identifying the presence of VOCs before confirmation by clinical epidemiological testing. For example, Joshi et al. (2021) identified Delta variant mutations in untreated wastewater samples more than a month before variant's clinical emergence in the region.83 Similarly, early indications of the Omicron variant were detected in community wastewater samples across multiple U.S states, with the earliest detection on November 21, 2021, predating the first clinically reported Omicron case on December 1, 2021, by the CDC.84 As well, a study by Novoa et al., (2022) revealed a significant correlation between the predominant variants identified in wastewater samples and the individuals testing positive clinically for those variants within the same geographic region.85 These examples, along with our observations, substantiate the utility of WWS for retrospective identification of variants, offering a critical window for timely public health interventions.
3.4. Limitations and interpretation
While RT-qPCR is recognized for its precision in detecting SARS-CoV-2, serving as a cornerstone for clinical diagnostics,86,87 this study extends its application to the surveillance of SARS-CoV-2 in wastewater, specifically for targeting the Omicron mutation N: P13L; C28311T. The development of RT-qPCR assays for identifying mutations indicative of VOCs in wastewater represents a significant advancement in viral surveillance.15,51,88–92 Despite its demonstrated effectiveness, it is important to acknowledge the inherent limitations of RT-qPCR methods. For example, RT-qPCR assays are limited to predefined genetic targets, which may miss novel mutations not incorporated in the assay. As well, RT-qPCR's sensitivity to primer and probe designs can also result in missed detections due to minor genetic variations.93 Although RT-qPCR is valuable for estimating viral loads, it does not offer the complete genomic context that sequencing does, limiting the depth of insight into the viral genome's evolution and interaction of mutations.
Importantly, the effectiveness of allele-specific RT-qPCR for detecting SARS-CoV-2 VOCs in wastewater relies on the validation before implementation to establish the assay's sensitivity and specificity.94 Equally critical is the adoption of robust quality assurance and quality control (QA/QC) measures to uphold the RT-qPCR results reliability.53 Our study ensured the reliability and accuracy of the allele-specific RT-qPCR assay for SARS-CoV-2 detection in wastewater by strictly adhering to QA/QC practices, including the use of extraction blanks, conducting sample analyses in dedicated laboratory spaces, internal amplification controls, positive-template control validation, and routine no-template controls in RT-qPCR runs.
The sensitivity and cross-reactivity of the C28311T allele specific RT-qPCR primers used in this study have validated in previous research, showing negligible cross-reactivity and providing high confidence in estimating the frequency of the C28311T mutation, which was shown to be the dominant circulating Omicron lineage in 2021 and 2022.70 The C28311T mutations was present in over 97% of the B.1.1.529 (Omicron; BA.1 and BA.2) and C.37 (Lambda) GISAID-deposited sequences (as of 12 January 2022; http://outbreak.info), while it is found in less than 0.5% of other sequences. The cross-reactivity of the allele specific RT-qPCR primers was further tested against RNA templates containing non-Omicron/Lambda sequences (i.e., ancestral/wild type, Delta, and Alpha variant sequences). The C28311T assay performed well across a linear range using Omicron/Lambda RNA templates, but was unable to amplify until over 1000 gene copies when using non-Omicron/Lambda template RNA.
While genomic sequencing remains vital for confirming the presence and identity of VOCs, its application in routine WWS is hindered by several practical challenges. These include high operational costs, the necessity for specialized technical expertise, and extended processing times.88,89 Moreover, the complex nature of wastewater samples, characterized by their propensity for RNA degradation and dilution, poses additional complexities for adequate genomic sequencing methods.95 Given these constraints, allele-specific RT-qPCR analysis emerges as a viable alternative for ongoing monitoring of VOC's in wastewater. Although RT-qPCR may not provide the comprehensive genomic insights provided by sequencing, when properly validated, its sensitivity, speed, and cost-effectiveness compared to sequencing make it a valuable tool for timely viral detection.
4. Conclusions
The utility of WWS in university residences, as demonstrated in this study, highlights its practicality as an approach to offer actionable insights for campus health management. The success of the WWS strategy employed was notably enhanced through passive sampling techniques, recognized for their low maintenance, cost-effectiveness, and extended monitoring capabilities, which played a pivotal role in the success of this surveillance initiative. This approach enables the rapid acquisition of test results, facilitating swift interventions to mitigate the spread of COVID-19 infections on campus. Additionally, this study revealed the emergence of the C28311T mutation, associated with the Omicron variant, through retrospective examination of samples. This analysis revealed the temporal and spatial variability of the C28311T mutation across both the university and broader community's wastewater throughout the study period. The findings of this study emphasize the value of allele-specific RT-qPCR assays in deepening our understanding of the evolutionary dynamics of variants as detected through WWS. This illustrates the program's capability to provide a better understanding of COVID-19 dynamics and assist in the implementation of targeted remedial actions.
5. Research ethics statement for wastewater surveillance studies
In consultation with the Research Ethics Board (REB) at Dalhousie University, it was determined that REB review was not required for research that involves analysis of anonymous human biological materials (such as municipal waste) without generating identifiable information. This research complies with article 2.4 described in the Tri-Council Policy Statement: Ethical Conduct for Research Involving Humans (TCPS 2, 2018).
Data availability
The data supporting this article have been included as part of the ESI.†
Conflicts of interest
There are no conflicts to declare.
Acknowledgements
This study received funding from a New Frontiers in Research Fund [Grant Number NFRFR-2021-00081], a Research Nova Scotia Special Initiatives Grant [Grant Number #1367], an NSERC Alliance Grant (ALLRP 568507 - 21) with support from LuminUltra Technologies Ltd, Mantech Inc., AGAT Laboratories, Halifax Water, AquiSense Technologies, CBCL Limited, City of Moncton, Cape Breton Regional Municipality. We also thank research associate, Genevieve Erjavec for their diligent work in wastewater sample collection and analysis. As well, we would like to extend thanks to Dr. Tyson Graber and Dr. Robert Delatolla, for sharing the primer sequences and methodology for the C28311T RT-qPCR assay and for their technical review of our manuscript.
References
-
World Health Organization, Tracking SARS-CoV-2 variants, https://www.who.int/emergencies/what-we-do/tracking-SARS-CoV-2-variants, (accessed January 6, 2022).
-
CDC, Science Brief: Omicron (B.1.1.529) Variant, https://www.cdc.gov/coronavirus/2019-ncov/science/science-briefs/scientific-brief-omicron-variant.html, (accessed January 6, 2022).
-
World Health Organization, Enhancing Readiness for Omicron (B.1.1.529): Technical Brief and Priority Actions for Member States, World Health Organization, Geneva, Switzerland, 2021 Search PubMed.
- S. Poudel, A. Ishak, J. Perez-Fernandez, E. Garcia, D. A. León-Figueroa, L. Romaní, D. K. Bonilla-Aldana and A. J. Rodriguez-Morales, Travel Med. Infect. Dis., 2022, 45, 102234 CrossRef CAS PubMed.
- X. He, W. Hong, X. Pan, G. Lu and X. Wei, MedComm, 2021, 2, 838–845 CrossRef CAS PubMed.
- V. M. Ferré, N. Peiffer-Smadja, B. Visseaux, D. Descamps, J. Ghosn and C. Charpentier, Anaesth. Crit. Care Pain Med., 2022, 41, 100998 CrossRef PubMed.
- Covid: Omicron variant in Netherlands earlier than thought. BBC News, 2021, https://www.bbc.com/news/world-europe-59473131 Search PubMed.
- P. Gupta, S. Liao, M. Ezekiel, N. Novak, A. Rossi, N. LaCross, K. Oakeson and A. Rohrwasser, Microbiol. Spectrum, 2023, 11(3), e00391-23 CrossRef PubMed.
- S. R. Kannan, A. N. Spratt, K. Sharma, H. S. Chand, S. N. Byrareddy and K. Singh, J. Autoimmun., 2022, 126, 102779 CrossRef CAS PubMed.
- X. Du, H. Tang, L. Gao, Z. Wu, F. Meng, R. Yan, S. Qiao, J. An, C. Wang and F. X.-F. Qin, Signal Transduction Targeted Ther., 2022, 7, 1–3 CrossRef.
- V. M. Ferré, N. Peiffer-Smadja, B. Visseaux, D. Descamps, J. Ghosn and C. Charpentier, Anaesth. Crit. Care Pain Med., 2022, 41, 100998 CrossRef PubMed.
-
D. G. Manuel, R. Delatolla, D. N. Fisman, M. Fuzzen, T. Graber, G. M. Katz, J. Kim, C. Landgraff, A. MacKenzie, A. Maltsev, A. Majury, R. M. McKay, J. Minnery, M. Servos, J. S. Weese, A. McGeer, K. B. Born, K. Barrett, B. Schwartz and P. Jüni, The Role of Wastewater Testing for SARS-CoV-2 Surveillance, Ontario COVID-19 Science Advisory Table, 2021 Search PubMed.
- G. Medema, L. Heijnen, G. Elsinga, R. Italiaander and A. Brouwer, Environ. Sci. Technol. Lett., 2020, 7, 511–516 CrossRef CAS PubMed.
- W. Ahmed, A. Bivins, W. J. M. Smith, S. Metcalfe, M. Stephens, A. V. Jennison, F. A. J. Moore, J. Bourke, S. Schlebusch, J. McMahon, G. Hewitson, S. Nguyen, J. Barcelon, G. Jackson, J. F. Mueller, J. Ehret, I. Hosegood, W. Tian, H. Wang, L. Yang, P. Bertsch, J. Tynan, K. V. Thomas, K. Bibby, T. E. Graber, R. Ziels and S. L. Simpson, Sci. Total Environ., 2022, 153171 CrossRef CAS.
-
W. L. Lee, X. Gu, F. Armas, F. Wu, F. Chandra, H. Chen, A. Xiao, M. Leifels, F. J. D. Chua, G. W. Kwok, J. Y. Tay, C. Y. Lim, J. Thompson and E. J. Alm, medRxiv, 2021, preprint, DOI:10.1101/2021.12.21.21268077.
- A. Bivins, D. Kaya, W. Ahmed, J. Brown, C. Butler, J. Greaves, R. Leal, K. Maas, G. Rao, S. Sherchan, D. Sills, R. Sinclair, R. T. Wheeler and C. Mansfeldt, Sci. Total Environ., 2022, 835, 155347 CrossRef CAS PubMed.
- A. Bivins, M. Lott, M. Shaffer, Z. Wu, D. North, E. K. Lipp and K. Bibby, Environ. Sci.: Water Res. Technol., 2022, 8, 173–183 RSC.
- J. L. Kevill, K. Lambert-Slosarska, C. Pellett, N. Woodhall, I. Richardson-O'Neill, I. Pântea, N. Alex-Sanders, K. Farkas and D. L. Jones, Sci. Total Environ., 2022, 838, 156580 CrossRef CAS PubMed.
- C. Schang, N. D. Crosbie, M. Nolan, R. Poon, M. Wang, A. Jex, N. John, L. Baker, P. Scales, J. Schmidt, B. R. Thorley, K. Hill, A. Zamyadi, C.-W. Tseng, R. Henry, P. Kolotelo, J. Langeveld, R. Schilperoort, B. Shi, S. Einsiedel, M. Thomas, J. Black, S. Wilson and D. T. McCarthy, Environ. Sci. Technol., 2021, 55, 10432–10441 CrossRef CAS PubMed.
- J. Habtewold, D. McCarthy, E. McBean, I. Law, L. Goodridge, M. Habash and H. M. Murphy, Environ. Res., 2022, 204, 112058 CrossRef CAS PubMed.
- E. K. Hayes, C. L. Sweeney, M. Fuller, G. B. Erjavec, A. K. Stoddart and G. A. Gagnon, ACS EST Water, 2022, 2(11), 1910–1920 CrossRef CAS PubMed.
- P. Liu, M. Ibaraki, J. VanTassell, K. Geith, M. Cavallo, R. Kann, L. Guo and C. L. Moe, Sci. Total Environ., 2022, 807, 151047 CrossRef CAS.
- J. Li, W. Ahmed, S. Metcalfe, W. J. M. Smith, B. Tscharke, P. Lynch, P. Sherman, P. H. N. Vo, S. L. Kaserzon, S. L. Simpson, D. T. McCarthy, K. V. Thomas, J. F. Mueller and P. Thai, Water Res., 2022, 218, 118481 CrossRef CAS.
- M. Breulmann, R. Kallies, K. Bernhard, A. Gasch, R. Müller, H. Harms, A. Chatzinotas and M. van Afferden, Sci. Total Environ., 2023, 887, 164143 CrossRef CAS PubMed.
- E. K. Hayes, A. K. Stoddart and G. A. Gagnon, Sci. Total Environ., 2022, 847, 57548 CrossRef PubMed.
- G. Cha, K. E. Graham, K. J. Zhu, G. Rao, B. G. Lindner, K. Kocaman, S. Woo, I. D'amico, L. R. Bingham, J. M. Fischer, C. I. Flores, J. W. Spencer, P. Yathiraj, H. Chung, S. Biliya, N. Djeddar, L. J. Burton, S. J. Mascuch, J. Brown, A. Bryksin, A. Pinto, J. K. Hatt and K. T. Konstantinidis, Sci. Total Environ., 2023, 866, 161101 CrossRef CAS.
- R. Corchis-Scott, Q. Geng, R. Seth, R. Ray, M. R. Beg, N. Biswas, L. Charron, K. D. Drouillard, R. D’Souza, D. Heath, C. Houser, F. Lawal, J. McGinlay, S. Menard, L. Porter, D. Rawlings, M. L. Scholl, K. Siu, Y. Tong, C. Weisener, S. Wilhelm and R. McKay, Microbiol. Spectrum, 2021, 9(12), e00792-21 CrossRef.
- M. Wilson, Y. Qiu, J. Yu, B. E. Lee, D. T. McCarthy and X. Pang, Pathogens, 2022, 11, 359 CrossRef CAS.
- M. Rafiee, S. Isazadeh, A. Mohseni-Bandpei, S. R. Mohebbi, M. Jahangiri-rad, A. Eslami, H. Dabiri, K. Roostaei, M. Tanhaei and F. Amereh, Sci. Total Environ., 2021, 790, 148205 CrossRef CAS PubMed.
- E. K. Hayes, C. Sweeney, L. E. Anderson, B. Li, G. B. Erjavec, M. T. Gouthro, W. Krkošek, A. Stoddart and G. A. Gagnon, Environ. Sci.: Water Res. Technol., 2021, 7(9), 1576–1586 RSC.
- N. W. West, J. Hartrick, M. Alamin, A. Vasquez, A. Bahmani, C. L. Turner, W. Shuster and J. L. Ram, Sci. Total Environ., 2023, 889, 164180–164180 CrossRef CAS PubMed.
- M. Hamouda, F. Mustafa, M. Maraqa, T. Rizvi and A. Aly Hassan, Sci. Total Environ., 2021, 759, 143493 CrossRef CAS PubMed.
- A. Green, Z. Song, C. Tran, S. Reifsnyder, D. Rosso, P. Hsia, N. Melitas, P. A. Holden and S. Jiang, Environ. Eng. Sci., 2024, 41, 7–17 CrossRef CAS.
- R. Corchis-Scott, Q. Geng, R. Seth, R. Ray, M. Beg, N. Biswas, L. Charron, K. D. Drouillard, R. D’Souza, D. D. Heath, C. Houser, F. Lawal, J. McGinlay, S. L. Menard, L. A. Porter, D. Rawlings, M. L. Scholl, K. W. M. Siu, Y. Tong, C. G. Weisener, S. W. Wilhelm and R. M. L. McKay, Microbiol. Spectrum, 2021, 9(2), e00792-21 CrossRef.
- W. L. Lee, M. Imakaev, F. Armas, K. A. McElroy, X. Gu, C. Duvallet, F. Chandra, H. Chen, M. Leifels, S. Mendola, R. Floyd-O'Sullivan, M. M. Powell, S. T. Wilson, K. L. J. Berge, C. Y. J. Lim, F. Wu, A. Xiao, K. Moniz, N. Ghaeli, M. Matus, J. Thompson and E. J. Alm, Environ. Sci. Technol. Lett., 2021, 8, 675–682 CrossRef CAS.
- A. Bivins and K. Bibby, Environ. Sci. Technol. Lett., 2021, 8, 792–798 CrossRef CAS.
- K. Reeves, J. Liebig, A. Feula, T. Saldi, E. Lasda, W. Johnson, J. Lilienfeld, J. Maggi, K. Pulley, P. J. Wilkerson, B. Real, G. Zak, J. Davis, M. Fink, P. Gonzales, C. Hager, C. Ozeroff, K. Tat, M. Alkire, C. Butler, E. Coe, J. Darby, N. Freeman, H. Heuer, J. R. Jones, M. Karr, S. Key, K. Maxwell, L. Nelson, E. Saldana, R. Shea, L. Salveson, K. Tomlinson, J. Vargas-Barriga, B. Vigil, G. Brisson, R. Parker, L. A. Leinwand, K. Bjorkman and C. Mansfeldt, Water Res., 2021, 204, 117613 CrossRef CAS PubMed.
- B. Malla, O. Thakali, S. Shrestha, T. Segawa, M. Kitajima and E. Haramoto, Sci. Total Environ., 2022, 853, 158659 CrossRef CAS PubMed.
- P. Liu, M. Ibaraki, J. VanTassell, K. Geith, M. Cavallo, R. Kann, L. Guo and C. L. Moe, Sci. Total Environ., 2022, 807, 151047 CrossRef CAS PubMed.
- C. Gibas, K. Lambirth, N. Mittal, M. A. I. Juel, V. B. Barua, L. Roppolo Brazell, K. Hinton, J. Lontai, N. Stark, I. Young, C. Quach, M. Russ, J. Kauer, B. Nicolosi, D. Chen, S. Akella, W. Tang, J. Schlueter and M. Munir, Sci. Total Environ., 2021, 782, 146749 CrossRef CAS.
- J. Wright, E. M. Driver, D. A. Bowes, B. Johnston and R. U. Halden, Sci. Total Environ., 2022, 152877 CrossRef CAS.
-
Dalhousie University, Residence Buildings, https://www.dal.ca/campus_life/residence_housing/residence/halifax-campus/res-buildings-halifax.html, (accessed January 12, 2022).
-
Dalhousie University, Update on Residence Application Process, https://www.dal.ca/campus_life/residence_housing/residence/residence_advisory/news-and-updates/2021/06/17/update_on_residence_applications_process.html, (accessed January 14, 2022).
-
Dalhousie University, Health & safety resources, https://www.dal.ca/covid-19-information-and-updates/covid-19-resources.html, (accessed January 12, 2022).
- X. Bertels, P. Demeyer, S. Van den Bogaert, T. Boogaerts, A. L. N. van Nuijs, P. Delputte and L. Lahousse, Sci. Total Environ., 2022, 820, 153290 CrossRef CAS PubMed.
- A. L. Parra-Guardado, C. L. Sweeney, E. K. Hayes, B. F. Trueman, Y. Huang, R. C. Jamieson, J. L. Rand, G. A. Gagnon and A. K. Stoddart, Environ. Sci.: Water Res. Technol., 2022, 8(1), 47–61 RSC.
- P. M. D'Aoust, X. Tian, S. T. Towhid, A. Xiao, E. Mercier, N. Hegazy, J.-J. Jia, S. Wan, M. P. Kabir, W. Fang, M. Fuzzen, M. Hasing, M. I. Yang, J. Sun, J. Plaza-Diaz, Z. Zhang, A. Cowan, W. Eid, S. Stephenson, M. R. Servos, M. J. Wade, A. E. MacKenzie, H. Peng, E. A. Edwards, X.-L. Pang, E. J. Alm, T. E. Graber and R. Delatolla, Sci. Total Environ., 2022, 853, 158547 CrossRef.
-
E. Arts, S. Brown, D. Bulir, T. C. Charles, C. T. DeGroot, R. Delatolla, J.-P. Desaulniers, E. A. Edwards, M. Fuzzen, K. Gilbride, J. Gilchrist, L. Goodridge, T. E. Graber, M. Habash, P. Jüni, A. Kirkwood, J. Knockleby, C. Kyle, C. Landgraff, C. Mangat, D. G. Manuel, R. M. McKay, E. Mejia, A. Mloszewska, B. Ormeci, C. Oswald, S. J. Payne, H. Peng, S. Peterson, A. F. Y. Poon, M. R. Servos, D. Simmons, J. Sun, M. Yang and G. Ybazeta, Community Surveillance of Omicron in Ontario: Wastewater-based Epidemiology Comes of Age, In Review, 2022 Search PubMed.
- daily epidemiology update. Public Health Agency of Canada, 2020, https://health-infobase.canada.ca/covid-19/epidemiological-summary-covid-19-cases.html#VO.
- W. Ahmed, A. Bivins, W. J. M. Smith, S. Metcalfe, M. Stephens, A. V. Jennison, F. A. J. Moore, J. Bourke, S. Schlebusch, J. McMahon, G. Hewitson, S. Nguyen, J. Barcelon, G. Jackson, J. F. Mueller, J. Ehret, I. Hosegood, W. Tian, H. Wang, L. Yang, P. M. Bertsch, J. Tynan, K. V. Thomas, K. Bibby, T. E. Graber, R. Ziels and S. L. Simpson, Sci. Total Environ., 2022, 820, 153171 CrossRef CAS.
- T. E. Graber, É. Mercier, K. Bhatnagar, M. Fuzzen, P. M. D'Aoust, H.-D. Hoang, X. Tian, S. T. Towhid, J. Plaza-Diaz, W. Eid, T. Alain, A. Butler, L. Goodridge, M. Servos and R. Delatolla, Water Res., 2021, 205, 117681 CrossRef CAS PubMed.
-
K. Yaniv, E. Ozer and A. Kushmaro, SARS-CoV-2 variants of concern, Gamma (P.1) and Delta (B.1.617), sensitive detection and quantification in wastewater employing direct RT-qPCR, Epidemiology, medRxiv, 2021, preprint, DOI:10.1101/2021.07.14.21260495.
- S. A. Bustin, V. Benes, J. A. Garson, J. Hellemans, J. Huggett, M. Kubista, R. Mueller, T. Nolan, M. W. Pfaffl, G. L. Shipley, J. Vandesompele and C. T. Wittwer, Clin. Chem., 2009, 55, 611–622 CrossRef CAS PubMed.
- M. A. Borchardt, A. B. Boehm, M. Salit, S. K. Spencer, K. R. Wigginton and R. T. Noble, Environ. Sci. Technol., 2021, 55, 10210–10223 CrossRef CAS PubMed.
-
T. Maere, J.-D. Therrien and P. VanRolleghem, Normalization practices for SARS-CoV-2 data in wastewater-based epidemiology, Université Laval, 2022 Search PubMed.
- A. Bivins, K. Crank, J. Greaves, D. North, Z. Wu and K. Bibby, Curr. Opin. Environ. Sci. Health, 2020, 16, 54–61 CrossRef.
- H. Wickham, M. Averick, J. Bryan, W. Chang, L. D. McGowan, R. François, G. Grolemund, A. Hayes, L. Henry, J. Hester, M. Kuhn, T. L. Pedersen, E. Miller, S. M. Bache, K. Müller, J. Ooms, D. Robinson, D. P. Seidel, V. Spinu, K. Takahashi, D. Vaughan, C. Wilke, K. Woo and H. Yutani, J. Open Source Softw., 2019, 4, 1686 CrossRef.
-
H. Wickham, T. Pedersen and D. Seidel, Scale Functions for Visualization (version R package version 1.3, https://github.com/r-lib/scales) https://scales.r-lib.org/, 2023.
-
C. O. Wilke and B. M. Wiernik, ggtext: Improved text rendering support for “ggplot2”, 2022.
-
Dalhousie University, Moving Forward: Dalhousie University Fall Return Guidance, Halifax, NS, 2021 Search PubMed.
-
Dalhousie University, Vaccine and testing requirements – update and clinic details, https://www.dal.ca/covid-19-information-and-updates/updates/2021/09/02/vaccine_and_testing_requirements___update_and_clinic_details.html, (accessed January 12, 2022).
-
Dalhousie University, COVID-19, https://www.dal.ca/covid-19-information-and-updates/updates/2021/12/11/covid_19__eight_presumptive_cases_identified.html, (accessed January 12, 2022).
-
Dalhousie University, COVID update, https://www.dal.ca/covid-19-information-and-updates/updates/2021/12/17/covid_update__online_start_to_winter_term.html, (accessed January 12, 2022).
-
Dalhousie University, Dalhousie vaccination and testing data, https://www.dal.ca/covid-19-information-and-updates/covid-19-resources/dalhousie-vaccination-and-testing-data.html, (accessed January 12, 2022).
- W. Q. Betancourt, B. W. Schmitz, G. K. Innes, S. M. Prasek, K. M. Pogreba Brown, E. R. Stark, A. R. Foster, R. S. Sprissler, D. T. Harris, S. P. Sherchan, C. P. Gerba and I. L. Pepper, Sci. Total Environ., 2021, 779, 146408 CrossRef CAS PubMed.
-
D. Barich and J. L. Slonczewski, medRxiv, 2021, preprint, DOI:10.1101/2021.01.09.21249505.
-
Berkeley University of California, Coronavirus Dashboard – Wastewater, https://coronavirus.berkeley.edu/dashboard/wastewater/, (accessed January 19, 2022).
- J. Lee, N. Acosta, B. J. Waddell, K. Du, K. Xiang, J. Van Doorn, K. Low, M. A. Bautista, J. McCalder, X. Dai, X. Lu, T. Chekouo, P. Pradhan, N. Sedaghat, C. Papparis, A. Buchner Beaudet, J. Chen, L. Chan, L. Vivas, P. Westlund, S. Bhatnagar, S. Stefani, G. Visser, J. Cabaj, S. Bertazzon, S. Sarabi, G. Achari, R. G. Clark, S. E. Hrudey, B. E. Lee, X. Pang, B. Webster, W. A. Ghali, A. G. Buret, T. Williamson, D. A. Southern, J. Meddings, K. Frankowski, C. R. J. Hubert and M. D. Parkins, Water Res., 2023, 244, 120469 CrossRef CAS PubMed.
-
Nova Scotia Department of Health and Wellness, 114 New Cases of COVID-19, Omicron Variant Cases, Long-Term Care Outbreak, https://novascotia.ca/news/release/?id=20211213006, (accessed January 12, 2022).
- O. Thakali, É. Mercier, W. Eid, M. Wellman, J. Brasset-Gorny, A. K. Overton, J. J. Knapp, D. Manuel, T. C. Charles, L. Goodridge, E. J. Arts, A. F. Y. Poon, R. S. Brown, T. E. Graber, R. Delatolla and C. T. DeGroot, Sci. Rep., 2024, 14, 3728 CrossRef CAS PubMed.
- M. Liddor Naim, Y. Fu, M. Shagan, I. Bar-Or, R. Marks, Q. Sun, R. Granek and A. Kushmaro, Viruses, 2023, 15, 1862 CrossRef PubMed.
-
National Collaborating Centre for Infectious Diseases, Updates on COVID-19 Variants of Concern (VOC), 2022 Search PubMed.
- A. Mitranescu, A. Uchaikina, A.-S. Kau, C. Stange, J. Ho, A. Tiehm, C. Wurzbacher and J. E. Drewes, ACS EST Water, 2022, 2, 2460–2470 CrossRef CAS PubMed.
- P. T. Acer, L. M. Kelly, A. Lover and C. S. Butler, Int. J. Environ. Res. Public Health, 2022, 19(18), 11245 CrossRef CAS PubMed.
- A. Aguayo-Acosta, M. G. Jiménez-Rodríguez, F. Silva-Lance, M. A. Oyervides-Muñoz, A. Armenta-Castro, O. de la Rosa, A. Ovalle-Carcaño, E. M. Melchor-Martínez, Z. Aghalari, R. Parra-Saldívar and J. E. Sosa-Hernández, Viruses, 2023, 15, 1941 CrossRef CAS PubMed.
- S. M. Prasek, I. L. Pepper, G. K. Innes, S. Slinski, W. Q. Betancourt, A. R. Foster, H. D. Yaglom, W. T. Porter, D. M. Engelthaler and B. W. Schmitz, Sci. Total Environ., 2023, 857, 159165 CrossRef CAS PubMed.
- M. Riediker, L. Briceno-Ayala, G. Ichihara, D. Albani, D. Poffet, D.-H. Tsai, S. Iff and C. Monn, Swiss Med. Wkly., 2022, 152, w30133 CrossRef CAS PubMed.
- D. Champredon, D. Becker, S. W. Peterson, E. Mejia, N. Hizon, A. Schertzer, M. Djebli, F. F. Oloye, Y. Xie, M. Asadi, J. Cantin, X. Pu, C. A. Osunla, M. Brinkmann, K. N. McPhedran, M. R. Servos, J. P. Giesy and C. Mangat, BMC Infect. Dis., 2024, 24, 139 CrossRef PubMed.
- D. Sint, L. Raso and M. Traugott, Methods Ecol. Evol., 2012, 3, 898–905 CrossRef PubMed.
- S. Isabel, M. Abdulnoor, K. Boissinot, M. R. Isabel, R. de Borja, P. C. Zuzarte, C. P. Sjaarda, K. R. Barker, P. M. Sheth, L. M. Matukas, J. B. Gubbay, A. J. McGeer, S. Mubareka, J. T. Simpson and R. Fattouh, Sci. Rep., 2022, 12, 14159 CrossRef CAS PubMed.
-
Public Health Agency of Canada, SARS-CoV-2 variants, https://www.canada.ca/en/public-health/services/diseases/2019-novel-coronavirus-infection/health-professionals/testing-diagnosing-case-reporting/sars-cov-2-variants-national-definitions-classifications-public-health-actions.html, (accessed January 12, 2022).
- D. Aggarwal, B. Warne, A. S. Jahun, W. L. Hamilton, T. Fieldman, L. du Plessis, V. Hill, B. Blane, E. Watkins, E. Wright, G. Hall, C. Ludden, R. Myers, M. Hosmillo, Y. Chaudhry, M. L. Pinckert, I. Georgana, R. Izuagbe, D. Leek, O. Nsonwu, G. J. Hughes, S. Packer, A. J. Page, M. Metaxaki, S. Fuller, G. Weale, J. Holgate, C. A. Brown, R. Howes, D. McFarlane, G. Dougan, O. G. Pybus, D. D. Angelis, P. H. Maxwell, S. J. Peacock, M. P. Weekes, C. Illingworth, E. M. Harrison, N. J. Matheson and I. G. Goodfellow, Nat. Commun., 2022, 13, 751 CrossRef CAS PubMed.
-
M. Joshi, M. Kumar, V. Srivastava, D. Kumar, D. Rathore, R. Pandit and C. G. Joshi, First detection of SARS-CoV-2 Delta variant (B.1.617.2) in the wastewater of (Ahmedabad), India, 2021 Search PubMed.
- A. E. Kirby, MMWR Morb. Mortal. Wkly. Rep., 2022 DOI:10.15585/mmwr.mm7103a5.
- B. Novoa, R. Ríos-Castro, I. Otero-Muras, S. Gouveia, A. Cabo, A. Saco, M. Rey-Campos, M. Pájaro, N. Fajar, R. Aranguren, A. Romero, A. Panebianco, L. Valdés, P. Payo, A. A. Alonso, A. Figueras and C. Cameselle, Sci. Total Environ., 2022, 833, 155140 CrossRef CAS PubMed.
- M. F. Khalid, K. Selvam, A. J. N. Jeffry, M. F. Salmi, M. A. Najib, M. N. Norhayati and I. Aziah, Diagnostics, 2022, 12, 110 CrossRef CAS PubMed.
-
D. Manuel, C. A. Amadei, J. R. Campbell, J.-M. Brault and J. Veillard, Strengthening Public Health Surveillance Through Wastewater Testing: An Essential Investment for the COVID-19 Pandemic and Future Health Threats, World Bank, Washington, DC, 2022 Search PubMed.
-
K. Yaniv, E. Ozer, N. Plotkin, N. S. Bhandarkar and A. Kushmaro, medRxiv, 2021, preprint, DOI:10.1101/2021.02.25.21252454.
- S. W. Peterson, R. Lidder, J. Daigle, Q. Wonitowy, C. Dueck, A. Nagasawa, M. R. Mulvey and C. S. Mangat, Sci. Total Environ., 2022, 810, 151283 CrossRef CAS PubMed.
- K. Yaniv, E. Ozer, Y. Lewis and A. Kushmaro, Water Res., 2021, 207, 117808 CrossRef CAS PubMed.
-
M. Wolfe, B. Hughes, D. Duong, V. Chan-Herur, K. R. Wigginton, B. J. White and A. B. Boehm, medRxiv, 2022, preprint, DOI:10.1101/2022.01.17.22269439.
- P. Foladori, F. Cutrupi, N. Segata, S. Manara, F. Pinto, F. Malpei, L. Bruni and G. La Rosa, Sci. Total Environ., 2020, 743, 140444 CrossRef CAS PubMed.
- H. Abbasi, H. R. Nikoo, F. Fotouhi and A. Khosravi, BMC Microbiol., 2023, 23, 335 CrossRef CAS PubMed.
- S. Broeders, I. Huber, L. Grohmann, G. Berben, I. Taverniers, M. Mazzara, N. Roosens and D. Morisset, Trends Food Sci. Technol., 2014, 37, 115–126 CrossRef CAS.
- G. Ni, J. Lu, N. Maulani, W. Tian, L. Yang, I. Harliwong, Z. Wang, J. Mueller, B. Yang, Z. Yuan, S. Hu and J. Guo, Environ. Sci. Technol. Lett., 2021, 8, 683–690 CrossRef CAS PubMed.
|
This journal is © The Royal Society of Chemistry 2025 |
Click here to see how this site uses Cookies. View our privacy policy here.