DOI:
10.1039/D3LC01055D
(Paper)
Lab Chip, 2024,
24, 2208-2223
Vascularized tissue on mesh-assisted platform (VT-MAP): a novel approach for diverse organoid size culture and tailored cancer drug response analysis†
Received
8th December 2023
, Accepted 14th March 2024
First published on 27th March 2024
Abstract
This study presents the vascularized tissue on mesh-assisted platform (VT-MAP), a novel microfluidic in vitro model that uses an open microfluidic principle for cultivating vascularized organoids. Addressing the gap in 3D high-throughput platforms for drug response analysis, the VT-MAP can host tumor clusters of various sizes, allowing for precise, size-dependent drug interaction assessments. Key features include capability for forming versatile co-culture conditions (EC, fibroblasts and colon cancer organoids) that enhance tumor organoid viability and a perfusable vessel network that ensures efficient drug delivery and maintenance of organoid health. The VT-MAP enables the culture and analysis of organoids across a diverse size spectrum, from tens of microns to several millimeters. The VT-MAP addresses the inconsistencies in traditional organoid testing related to organoid size, which significantly impacts drug response and viability. Its ability to handle various organoid sizes leads to results that more accurately reflect patient-derived xenograft (PDX) models and differ markedly from traditional in vitro well plate-based methods. We introduce a novel image analysis algorithm that allows for quantitative analysis of organoid size-dependent drug responses, marking a significant step forward in replicating PDX models. The PDX sample from a positive responder exhibited a significant reduction in cell viability across all organoid sizes when exposed to chemotherapeutic agents (5-FU, oxaliplatin, and irinotecan), as expected for cytotoxic drugs. In sharp contrast, PDX samples of a negative responder showed little to no change in viability in smaller clusters and only a slight reduction in larger clusters. This differential response, accurately replicated in the VT-MAP, underscores its ability to generate data that align with PDX models and in vivo findings. Its capacity to handle various organoid sizes leads to results that more accurately reflect PDX models and differ markedly from traditional in vitro methods. The platform's distinct advantage lies in demonstrating how organoid size can critically influence drug response, revealing insights into cancer biology previously unattainable with conventional techniques.
Introduction
Researchers have utilized various models for cancer and drug development. Until now, animal models (in vivo) and 2D well plate culture (in vitro) models are dominantly used, leading to the accumulation of extensive data. However, these approaches have limitations in adequately mimicking the human body due to constraints in long experiment time, ethical problems, and inherent differences between humans and animals. To overcome these limitations, there has been growing attention and importance placed on new in vitro model, called organ chips, which can replicate the human body's cellular composition and physiological conditions. Organ chips are advanced technologies that mimic realistic human physiological conditions, enabling the evaluation of drug efficacy and the study of complex biological interactions in environments closely resembling the human body.1–4 Nevertheless, it is still hard to fully mimic the complexity and conditions within the body.5 Considering these differences among in vivo and in vitro models, the emergence of organ chips holds significant importance compared to conventional in vitro systems in terms of 3D tissue implementation outside the body,6 providing a more in vivo-like environment based on more accurate replication of cellular physiological functions. Organ chips offer the advantage of obtaining results closer to in vivo experiments by reproducing the complexity and interactions of tissues. Also, they can incorporate crucial biological functions, such as vascularization, allowing for the regulation of factors like blood flow,7–9 cell–cell interactions,10,11 and permeability.5 Therefore, organ chips are regarded as valuable tools in various applications, including drug development and disease mechanism research,12–16 as they bridge the advantages and limitations of in vivo, and in vitro models.
The use of microfluidics in organ chips has expanded the possibilities for 3D cell culture, including microfluidic systems that allow for greater control over the chemical and mechanical stimuli that cells experience, for example, by controlling the mechanical stress from fluid flow to match the natural flow within vasculature,8 enabling the culture of polarized epithelium at the air–liquid interface,6 and by matching the stiffness of the culture surface more closely to the stiffness modulus that exists in soft tissue.9 Despite these advancements, challenges still remain in the development of 3D cell culture systems. These challenges include throughput, customizability, manufacturability, reproducibility, and design modification to meet specific applications. Researchers continue to work on natural matter, developing new approaches to address these limitations and improve the capabilities of 3D cell culture systems.
There has been an increasing number of research findings utilizing 3D tissues or organoids similar to in vivo conditions.17–22 In particular, research on organoids has become very active in recent years.23,24 Organoids are large tissue structures cultured in vitro that contain functional components of real tissues, as opposed to simple cell aggregates like spheroids. They can be implemented to closely mimic the in vivo tumor microenvironment.13,25–27 For mimicking in vivo-like environment, it is necessary to not only culture organoids but also create a vascularized microenvironment. However, most existing in vitro models have primarily focused on small spheroid-based models.25,26 Consequently, there is a lack of models that allow for the co-culturing of large organoids.28 Furthermore, conventional tumor-on-a-chip models typically involve culturing a single spheroid in a well to mimic the tumor microenvironment. However, the tumor microenvironment consists of various clusters distributed in different sizes, making it challenging to replicate by an in vitro model.
There has been rapid progress in understanding the pathological characteristics of cancer and the importance of the tumor microenvironment.29 Consequently, there is a growing need for reliable experimental models in cancer therapy development and tumor biology research. In particular, with the discrepancies between animal experimental results and clinical outcomes and the ethical concerns surrounding animal experiments, the importance of in vitro models utilizing human cells has become even more pronounced.30In vitro models have been developed to reflect the complexity of the tumor microenvironment and provide valuable information for drug screening and tumor biology research.31–33
The emergence of scaled-up vascularized tissue or organoid models has opened up new possibilities for studying complex biological processes, regenerative medicine, and drug development. However, current in vitro platforms face challenges in maintaining scaled-up vascularization and the functionality of engineered tissues.34,35 Vascularization methods such as sacrificial templates or 3D bioprinting36,37 have limitations in terms of scalability, reproducibility, and complexity. Additionally, the development of vascularized tissue models that accurately mimic the spatial organization and physiological functions of the human body's native environment remains a significant challenge. Scaled-up vascularized tissue models have great potential in providing insights into disease mechanisms, personalized medicine, and drug screening. Therefore, there is a demand for innovative platforms that support the cultivation of scaled-up vascularized tissue or organoids and provide representative models that are more suitable for studying complex biological systems.38
Here, we present a novel trial to overcome the limitations of previous in vitro models and reconstruct a scaled-up vascularized tissue micromesh-assisted platform called VT-MAP (vascularized tissue on mesh-assisted platform), a promising approach as a combination of rail-guided and micromesh-assisted structure was used to design the scaled-up microfluidic platform. VT-MAP is an effective platform for the cultivation of tissues or organoids in large scale, specifically with fully covered vasculature over the entire height of the platform. Also, VT-MAP enables the extraction of diverse information in drug response test related to the size, growth rate, and viability of vascularized tissues collaborating with a novel quantification algorithm.
Experimental
Device design and fabrication
The device was designed through computer-aided design (CAD) and prototyped and fabricated using a 3D printer (Figure 4 Standalone, 3D systems). After 3D printing, it was washed with isopropanol and cured for more than 1 hour under 380 nm ultraviolet (UV) light. Once the device was completely dry, a pressure-sensitive adhesive (PSA) film (IS-08820, IZ solution) was applied to the bottom surface of the device to create a space for filling with hydrogel. While preparing cells before patterning the hydrogel on the device, sterilization was carried out under UV light in a bio-hazard safe bench. Additionally, the device surface was modified to facilitate hydrogel patterning by subjecting it to O2 plasma treatment for 3 minutes under conditions of 75 W and 50 kHz, creating a hydrophilic surface.
Cell culture
Human umbilical vein endothelial cells (HUVECs, Lonza) and lung fibroblasts (LFs, Lonza) were cultured in endothelial growth medium-2 (EGM-2, Lonza) and fibroblast growth medium-2 (FGM-2, Lonza), respectively, to reconstruct a 3D vascular network surrounding tumor organoid clusters. HUVECs and LFs were used at passage 6 and 7, respectively. Cells were cultured in a humidified incubator at 37 °C with 5% carbon dioxide (CO2).
Isolation and primary culture of cancer associated fibroblast (CAF)
For the isolation of cancer-associated fibroblasts (CAFs), fresh tumor samples were obtained from the Department of Surgery at Samsung Medical Center (Seoul, Korea) in accordance with protocols approved by the Institutional Review Boards (SMC 2017-07-131-020). Tumor tissues were cut into small pieces, washed with ice-cold 70% ethanol and phosphate-buffered saline (PBS) with 3% penicillin–streptomycin (Thermo Fisher Scientific Inc., Waltham, MA, USA), and enzymatically dissociated in Dulbecco's modified Eagle's medium (DMEM, Gibco, Thermo Fisher Scientific, Inc.) containing 1% penicillin–streptomycin (Thermo Fisher Scientific, Inc.), 2.5% fetal bovine serum (FBS, Biowest, Nuaille, France), 75 U mL−1 collagenase type IV (Gibco, Thermo Fisher Scientific, Inc.), and 125 μg mL−1 Dispase II (Life Technologies, Carlsbad, CA, USA) in a 37 °C incubator for 20–30 min using a tube rotator. Following digestion, samples were passed through a 100 μm cell strainer (Falcon; BD Biosciences, NJ, USA) and centrifuged at 1000 rpm for 3 min. Then, the pellet was washed with PBS and cultured in DMEM/F12 supplemented with 20% FBS and 1% penicillin–streptomycin for 7–10 days. Ten days after seeding, CAFs were maintained in DMEM/F12 containing 10% FBS and 1% penicillin–streptomycin to establish primary cultures and subsequent passages.
Tumor organoid culture
Colorectal cancer (CRC) organoids isolated from patient-derived xenograft tumor tissues were cultured as previously described.39 Tumor tissues collected from the PDX were washed with 70% ethanol and ice-cold PBS with 3% penicillin–streptomycin (Thermo Fisher Scientific Inc., Waltham, MA, USA), and minced prior to mechanical dissociation using a gentleMACS Dissociator (Miltenyi Biotec) followed by incubation at 37 °C in S/F M199 medium (Gibco) containing 200 U ml−1 collagenase (Gibco) and 0.1 mg ml−1 DNase (Roche) for 30 minutes. The dissociated cell suspension was passed through a 100 μm cell strainer (Falcon), washed with RPMI 1640 containing 10% FBS, and centrifuged at 1000 rpm for 3 minutes. The cell pellet was resuspended in Matrigel (BD Bioscience), and 50 μL of the cell–Matrigel mixture was plated in 24-well plates. After Matrigel polymerization, the cells were overlaid with 600–800 μL of complete medium composed of basal culture medium [advanced DMEM/F12 supplemented with 1% penicillin/streptomycin, hydroxyethylpiperazine ethanesulfonic acid (HEPES), 2 mM Glutamax, 1 × B27 (all from Gibco, Thermo Fisher Scientific, Inc.), 1.25 mM N-acetylcysteine (Sigma-Aldrich), 100 μg mL−1 Primocin (InvivoGen, San Diego, CA, USA), and 10 mM nicotinamide (Sigma-Aldrich)] supplemented with 1 μg mL−1 human R-Spondin 1 (Peprotech, NJ, USA), 100 ng mL−1 human Noggin (Biovision, CA, USA), 10 μM Y-27632 (Selleckchem, TX, USA), 500 nM A83-01 (Sigma-Aldrich), 3 μM SB202190 (Sigma-Aldrich), 50 ng mL−1 human EGF (Sigma-Aldrich), 10 nM prostaglandin E2 (Caymanchem, MI, USA), and 10 nM gastrin (Sigma-Aldrich).
Generation of patient-derived xenograft and in vivo drug treatment
The organoid–Matrigel mixture (1 × 106 cells) was subcutaneously implanted into the left flanks of 6–7-week-old female Balb/c nude mice (Orient Bio, Korea). When tumors reached a size of 50–100 mm3, mice were randomized into treatment groups, each consisting of 8 mice: (a) control; (b) 5-fluorouracil (5-FU) 40 mg kg−1; (c) oxaliplatin 6 mg kg−1 twice a week i.p.; and (d) 5-fluorouracil + oxaliplatin once a week i.p. All treatments were administered for 4 or 5 weeks, and mice were monitored twice a week. Tumor size was assessed biweekly using caliper measurements, calculated by the formula: tumor volume = (length × width2)/2. Relative tumor growth inhibition (TGI) was determined by dividing the relative tumor growth of treated mice by that of control mice. All animal experiments were conducted in accordance with protocols approved by the Institutional Review Boards of Samsung Medical Center (Seoul, Korea) and followed the Institute for Laboratory Animal Research Guide for the Care and Use of Laboratory Animals.
3D vascular network formation and reconstruction of tumor organoid microenvironment
To implement the tumor organoid microenvironment within a microfluidic device, tumor organoids were patterned in the form of small clusters within the device along with HUVECs, LFs, and hydrogel. Constructing a vascularized model while maintaining high cell viability for tumor organoids was considered to provide a more accurate comparison of drug responses, taking into account the proliferation rate differences between tumor cells and other cells. On day 0, HUVECs and LFs were mixed with a 2.5 mg mL−1 concentration of fibrinogen hydrogel (F3879, Sigma Aldrich) in the side channel of the device, with a HUVEC
:
LF ratio of 2
:
1, each having final concentrations of 4 × 106 cells per mL and 2 × 106 cells per mL, respectively. Over two days, a vascular network self-assembled, and on day 2, the cell culture medium within the device was completely aspirated. Subsequently, a mixture of tumor organoid clusters, HUVECs, LFs, and fibrinogen hydrogel was filled in the center channel to complete the model construction. Tumor organoid clusters were dissociated to achieve a final concentration of 2 × 106 cells per mL. The final concentration of HUVECs and LFs in the center channel was the same as that in the side channel. The cultures were then incubated at 37 °C with 5% CO2 until day 6.
Bead assay for perfusability
After establishing the 3D vascularized tumor organoid microenvironment within the device until day 6, a live bead assay was conducted using a confocal microscope. Dyed Red Aqueous Fluorescent Particles with a diameter of 2.0 μm (R0200, Thermo Fisher Scientific Inc.) were mixed with the cell culture medium and observed in real time as they moved from the medium reservoir into the blood vessels. Using the confocal microscope program, the positioning of microbeads within the lumen was confirmed through sectioned images.
Drug sensitivity test
To assess the response of tumor organoids to chemotherapeutics in 3D organoid culture, the following drug combinations were used: 5-FU, oxaliplatin, and 5-fluorouracil + oxaliplatin. Organoid viability in response to chemotherapeutics was measured using the CellTiter-Glo 3D cell viability assay (Promega) according to the manufacturer's instructions. Briefly, 5000 cells in 5 μL Matrigel were plated in triplicate in a 96-well plate and allowed to grow for 24 hours prior to drug treatment. After 72 hours of drug exposure, the CellTiter-Glo reagent and organoid culture medium were mixed with a volume ratio of 1
:
1. Luminescence was detected on a Mithras plate reader (Berthold Technologies).
For the observation of drug performance in the VT-MAP, drugs were treated on the vascularized tumor organoid microenvironment in the device. Oxaliplatin and irinotecan were treated as monotherapy or in combination with 5-FU. Control groups were treated with fresh EGM-2, and irinotecan and oxaliplatin were diluted to 200 mM in EGM-2 for monotherapy. 50 mM, 100 mM, and 200 mM 5-FU were mixed in equal concentrations of oxaliplatin or irinotecan for combination treatment, respectively. The drug treatment was sustained for 24 hours in an incubator to observe the drug performance.
Immunocytochemistry
After completing the cultivation or drug testing within the device, samples were fixed for observation of morphological differences through immunocytochemistry. Sample fixation involved washing with PBS (SH30256.01, SeouLin Bioscience) and proceeding with 4% paraformaldehyde (PFA, PC2031-050-00, Biosesang) for 20 minutes. Endothelial cells were stained with Ulex europaeus agglutinin I, fluorescein (UEA I, FL-1061, Vector Labs), while tumor organoid cells were stained with Alexa Fluor® 594 anti-human CD326 (epithelial cell adhesion molecule, EpCAM) antibody (324228, BioLegend), both mixed with 3% bovine serum albumin (BSA) solution (C0082-100, Research and Diagnostic Technology) at ratios of 500
:
1 and 200
:
1, respectively. The stained samples were stored by washing with PBS and rocking within the device overnight at 4 °C. The stained samples were observed using a confocal microscope (ECLIPSE Ti2, Nikon, Japan).
Live/dead assay
To assess the viability of tumor cells in response to drug treatment, samples within the device were stained through immunocytochemistry for live observation. Alexa Fluor® 488 anti-human CD326 (epithelial cell adhesion molecule, EpCAM) antibody (324210, BioLegend) and propidium iodide (PI, P3566, Thermo Fisher Scientific Inc.) were used to identify the areas of tumor organoid cells and the regions of dead cells within the device. Staining agents mixed in cell culture medium EGM-2 at ratios of 200
:
1 and 1000
:
1 were delivered to cells through the medium reservoir within the device. Imaging was performed 48 hours after drug treatment, taking into account the reaction times and toxicity of each staining agent. EpCAM was added at the onset of drug treatment, while PI was added 30 minutes before imaging. The EpCAM-stained areas were considered as tumor organoid clusters, and overlapping PI signals were calculated to compare the viability differences in tumor organoid clusters based on drug response with the control group.
Quantitative image analysis
The images captured through confocal microscopy were processed using imaging software, NIS-Elements and ImageJ. To quantify the viability and area of organoids, the confocal images were projected along the Z-axis using the max intensity method. As the organoid images exhibited various morphologies and contrasts, pre-processing involving histogram equalization and normalization was applied to detect their contour lines using Python 3.7 and the OpenCV package. Within the contour lines, green signal pixels indicating EpCAM staining for the entire organoid area and red signal pixels indicating PI staining for the dead area of the organoid were calculated to quantify the size and viability. We utilized a graphing program (Prism 9, GraphPad) to analyze pixel area data in terms of the size and viability of the organoid under different drug conditions.
Immunohistochemistry
Formalin-fixed, 4 μm paraffin-embedded tissue sections were dewaxed in xylene and rehydrated through a graded alcohol series. Endogenous peroxidase was blocked with 3% H2O2. Before applying the primary antibody, sections were immersed in 10 mM citrate buffer (pH 6.0), rinsed in Tris-buffered saline, and heated in a microwave oven for 5 minutes. The following primary antibodies were used: EpCAM (1
:
200; Dako Diagnostic) and cytokeratin 20 (CK20) (1
:
20; Dako Diagnostic, Glostrup, Denmark). Sections were subsequently treated with a secondary antibody for 30 minutes and incubated with the avidin–biotin–peroxidase complex for 30 minutes. Diaminobenzidine was used as the chromogen, followed by slight hematoxylin counterstaining. Organoid–Matrigel domes were fixed with 4% paraformaldehyde, and the fixed domes were placed in a mold containing optimal cutting temperature compound and stored at −80 °C. Frozen organoid sections (thickness, 10 μm) were subjected to immunohistochemistry staining and routine H&E staining for the examination of cell morphology.
Statistical analysis
GraphPad Prism 9 was utilized for data visualization, presenting values as mean ± SEM. Statistical analysis was conducted using unpaired t-tests. Significance was determined based on a p-value threshold; with * indicating p < 0.05, ** for p < 0.01, *** for p < 0.001, and **** for p < 0.0001. Non-significant results were denoted as ‘ns’.
Results and discussion
Concept of the VT-MAP
Building a vascularized tumor microenvironment (TME) within microfluidic chips is obviously helpful in understanding the physiological systems of tumors and developing drugs in response.40 Many anti-cancer drugs have been developed for various cancer types, leading to a significant increase in overall survival rates compared to the past. However, drug responses for metastatic cancers remain subtle, resulting in low survival rates. To address this, there is a need to simulate the growth and observe the developmental process at second tumor sites following tumor metastasis for the development of drugs. The VT-MAP is suitable for this purpose, particularly allowing observation of the development of each tumor cluster in different sizes and shapes at the second tumor site.
From a design perspective, the VT-MAP has a scaled-up cell culture area compared to traditional organ-on-a-chip devices. As shown in Fig. 1, the standard technique for the PDMS platform is post-guided patterning, whereas the injection molding platform utilizes rail-guided patterning. Additionally, a micro mesh structure is employed in 3D printing platforms at the platform schematic. With the patterning process, the first two methods are capable of patterning at heights below 300–400 μm, offering rapid patterning with high yield and stable outcomes. However, to fabricate larger organoids or tissue models as in vitro vascularized tissue models, a stable patterning method at greater heights (over 500 μm) is required. With rail-guided patterning, increasing the height to over 500 μm results in a reduced Laplace pressure of the fluid, making it difficult to maintain the height on the rail and causing the fluid to spread downwards, leading to unsuccessful patterning. To overcome this, an open lattice structure with a mesh design is employed. The mesh, due to its net-like form, can maintain a higher Laplace pressure compared to rail structures, thus effectively retaining fluid even at higher heights. Additionally, it enables selective patterning in complex structures. This approach allows for the creation of combination patterning structures that integrate both rail-guided and mesh designs. Vessel formation demonstrates the appearance of vascular structures under various patterning conditions. In particular, as demonstrated in Fig. 1, combination patterning enables the creation of complex-shaped patterns that encapsulate tissue areas, replicating a microenvironment where tissues or organoids are enveloped by blood vessels. This technique offers a platform capable of producing vascularized tissues. Such combination patterning is a principal concept and a key characteristic of the VT-MAP.
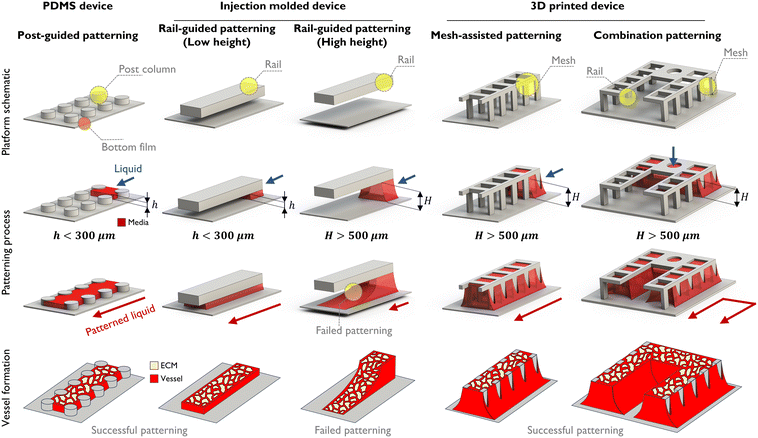 |
| Fig. 1 Evolution of liquid patterning techniques in Organ-on-a-Chip platforms. This image contrasts the design and operational principles of three major patterning methods. Initially, the PDMS platform utilized post columns as its core structural element. Subsequently, the injection molding technique introduced rail features for patterning, while the latest advancement, the 3D printing platform, incorporates a mesh structure for enhanced versatility. Notably, a hybrid approach merges rail and mesh structures for improved patterning efficacy. The depicted fluid injection direction (blue arrow) and liquid pattern formation (red arrows) highlight the operational dynamics of each platform at patterning process. While PDMS and injection molding platforms exhibit effective patterning at lower heights (h, under 300 μm), they encounter limitations at greater heights (H, 500 μm). In contrast, the 3D printing method ensures consistent patterning across complex, curved geometries and integrates seamlessly with rail structures, facilitating stable vascular pattern formation by cultured endothelial cells (HUVEC). | |
Design and fabrication of the VT-MAP
The VT-MAP device is composed of a center channel in the shape of a keyhole, a side channel enveloping the center channel, and outer channels adjacent to the outer side of the side channel (Fig. 2a and b). The center channel, with a central circular diameter of 1500 μm, is designed to accommodate cells of various sizes. The keyhole, with a width of 800 μm, is designed to prevent organoids with a diameter of over 1000 μm from exiting and ensures their positioning within the center circle. The keyhole shape of the center channel allows gas to escape when the side channels are initially filled, trapping the center channel. This facilitates gas exit when the center channel is filled with fluid, preventing difficulties in forming a complete interface. The curved side channel that surrounds the center channel is constructed with a rail structure that includes a micromesh, with both the channel height and the micromesh size being 500 μm (the micromesh has equal horizontal and vertical lengths). Placing the micromesh structure on the outermost part of the side channel ensures that only a fluid interface exists between the center and the side channels, maximizing the interactive surface area. Additionally, to enhance nutrient transfer efficiency into the hydrogel when a thick hydrogel is filled with a thickness of 1 mm and a height of 500 μm in the side channel, windows were created in the rail structure at intervals to maximize the direct interaction area with the medium reservoir. Finally, as the side channel is filled with fluid, the center channel and outer channel are separated, with the outer channel positioned adjacent to the side channel and the micromesh structure at the center. The fluid interaction between the side channel and the outer channel is possible through the vacant space between the micromesh structures. The medium reservoir is delineated by a wall positioned above the rail structure, following the micromesh structure. To efficiently discern various experimental conditions, each device has 14 wells, designed to conform to the standard SBS format of a 96-well plate for ease of imaging and experimental convenience. This ensures compatibility with various equipment, maximizing experimental efficiency.
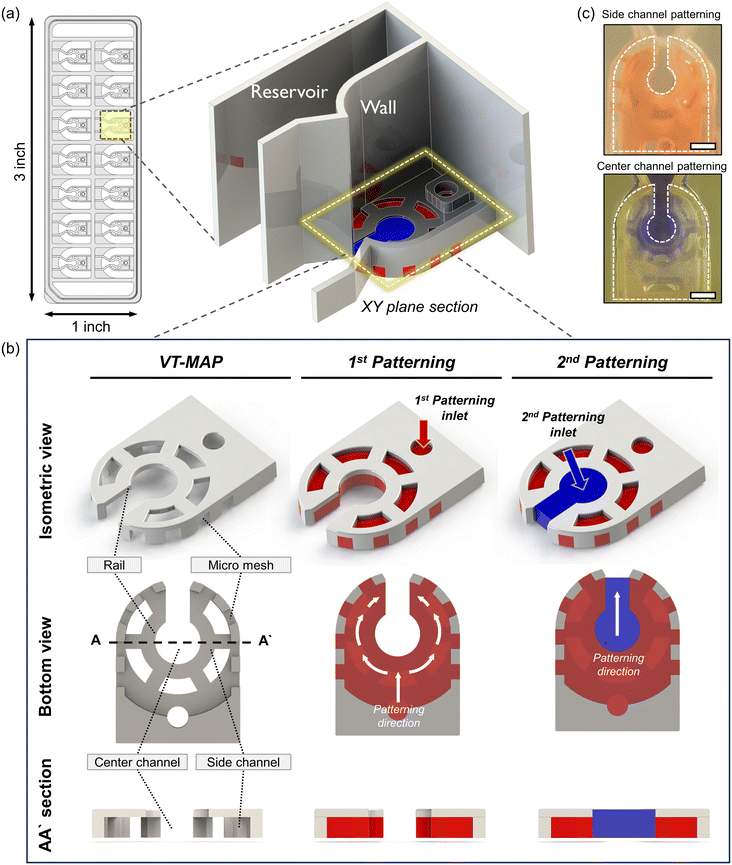 |
| Fig. 2 Device schematic of the VT-MAP and patterning process. (a) The VT-MAP is designed as a chip the size of a standard slide glass. Each well is segmented into three areas by two symmetry walls. The central area is designated for patterning, while the two side areas serve as reservoirs for medium supply. To demonstrate the chip's structure, one wall is rendered transparent, although in actuality, both walls are of the same height. Medium can be introduced into each of the three separated spaces independently. (b) The patterning process of the VT-MAP proceeds in two steps from the XY plane section view. The first step is filling the vascular area with the aim of forming a vascular network. The presence of a mesh structure prevents the fluid from spreading in other directions and allows patterning to occur only in the desired area. In this step, the vascular area is marked in red. The second step is filling the organoid area, with the goal of injecting large-scale organoids. In this step, the organoid area is marked in blue and interacts directly with the vascular area. Through these two steps, effective patterning and co-culture are possible, enabling the study and modeling of complex biological systems. (c) The side channel is patterned with a red dye, demonstrating the specific area covered. For the center channel, after filling the side channel with fibrin gel, the central region is patterned with a blue dye to highlight its distinct space. | |
For device fabrication, 3D printing was utilized for both prototyping and the final device production of the VT-MAP. After 3D printing, thorough washing with IPA was followed by a UV curing process for over an hour to reduce the toxicity of the photocurable resin to cells. After curing, the device was air-dried at room temperature, and a PSA film attached to the bottom surface of the device served as a substrate to create spaces for fluid patterning, completing the chip fabrication for experiments. The use of 3D printing allowed for significant productivity compared to photolithography, offering high design flexibility for complex designs not achievable in injection molding processes. Moreover, by modifying parameters such as width, length, and height for each well's design within the device, one can construct an optimized model tailored to experimental purposes, seeking to enhance experimental efficiency.
Patterning principle of the VT-MAP
Fluid patterning within the device is broadly divided into three stages: side channel patterning, center channel patterning, and outer channel patterning (Fig. 2b and c). Fluid is initially introduced into the side channel through a hole at the bottom of each well. Due to the substantial height of 500 μm, spontaneous force through rail-guides is relatively weak, and this was overcome by introducing a micromesh structure. Once approximately 15 μL of fluid fills the side channel, it separates the center channel and outer channel. The center channel requires about 10 μL of fluid, and through an open upper structure, bubbles are expelled, ensuring the formation of a well-defined hydrogel interface. Finally, the outer channel can accommodate 10 μL of fluid each, maintaining adjacency with the hydrogel in the side channel through surrounding structures, thus forming the well-defined interface.
The Young–Laplace pressure plays a pivotal role in elucidating the dynamics of fluid motion and serves as an instrumental mechanism for orchestrating fluid patterning (eqn (1)). The previous rail-assisted platforms41 and this principle direct fluid to delineate patterns along designated rails. However, the VT-MAP incorporates a novel mesh structure to prevent the fluid patterning from penetration into the central area while maintaining the intended forward pattern. The key parameters are L, representing the distance the fluid has advanced forward, and D, which denotes the width of the central channel and corresponds to the diameter of the circle with radius R1 (Fig. 3a). The Laplace pressure exerted in the direction of the center channel is named ‘burst Laplace pressure’ and is represented in green. In contrast, the pressure acting towards the side channel is identified as ‘forward Laplace pressure,’ depicted in blue (Fig. 3b). Both types of Laplace pressures can undergo transformation via a coordinate system transformation. To achieve patterning condition, two pressures, namely the forward Laplace pressure (FLP) and the burst Laplace pressure (BLP), are compared. In the VT-MAP, the diameter of the vascular area is denoted as R2, and the diameter of the organoid area is denoted as R1. By utilizing the principles of Young–Laplace pressure in this manner, more precise control over the desired pattern formation becomes possible.
| 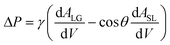 | (1) |
FLP represents the formation of a circular pattern along the mesh-assisted structure, preventing the fluid from bursting into the central area. This can be expressed mathematically by defining theta as the degree to which the fluid rotates in a circular manner around the origin and
w1 as the length of the forward face when FLP occurs. Additionally, the delta variable is used to represent changes over a short period of time. In this setup, each red point can be transformed into an orthogonal coordinate system composed of
R1 and
θ. Utilizing this, we can obtain the following equation (
eqn (2)), allowing us to understand the relationship between the rate of change in FLP and the various variables. By employing these equations, we can gain a more precise understanding and control of circular pattern formation in the VT-MAP. If BLP is smaller than the FLP value, the fluid is pushed toward the central area due to the Young–Laplace pressure toward the organoid region, preventing the formation of a circular pattern and hindering the desired patterning. In this case, the fluid tends to move toward the central area rather than spreading in a circular manner, and this can be expressed mathematically as an equation involving
R1 and
θ (
eqn (3)). The value of theta is substituted with
L (fluid forward distance), which is then incorporated into the FLP and BLP formulas to establish a relationship between
L and
D (
eqn (4)).
|  | (2) |
| 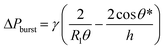 | (3) |
| 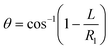 | (4) |
For the fluid to pattern in the desired manner, the forward Young–Laplace pressure must always be smaller than the burst pressure (
eqn (5)).
Fig. 3c represents the conditions that satisfy this criterion as a graph with respect to
L. The positive region in the graph represents successful conditions where stable patterning occurs along the circular path, while the negative region represents failure conditions where the fluid bursts towards the center instead of following the circular pattern. The graph depicts the conditions for
D values of 1.0, 1.5, 2.0, and 2.5 mm. In the VT-MAP, the actual
D value used is 1.5 mm, and the results from the image on this condition confirm the successful formation of the pattern. Conversely, for
D values of 2.0 and 2.5 mm, the pattern formation is observed to be failed in
Fig. 3d. The presence or absence of a mesh structure and the patterning behavior at different contact angles are demonstrated in Fig. S4a.
† Images illustrating the patterning process over time under successful patterning conditions (
D = 1.5 mm) are sequentially presented in Fig. S4b.
†
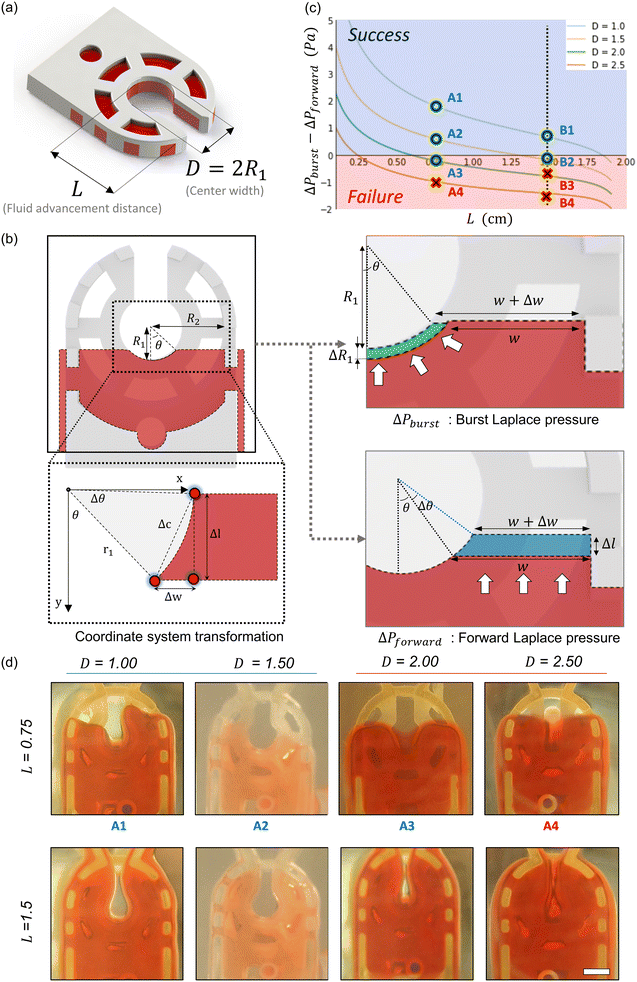 |
| Fig. 3 Patterning principle of the vascularized tissue mesh-assisted platform (VT-MAP). (a) The pivotal factor that affects the Young–Laplace pressure equation. Fluid advancement distance (L) of the VT-MAP and center width (D) of the VT-MAP are the main parameters. (b) Elucidation of the parameters influencing the forward and burst Laplace pressure conditions along the central hole axis, incorporating coordinate transformation for computational analysis. The Laplace pressure acting in the direction of the center channel is termed ‘burst Laplace pressure’ and is marked in green. The pressure exerted towards the side channel is designated as ‘forward Laplace pressure’, indicated in blue. Each of these Laplace pressures can be transformed through coordinate system transformation. (c) Depiction of successful and failed patterning indicated by blue and red areas, respectively, correlating to four distinct D values. (d) Representations of 3D printed models used to validate patterning efficacy for various D conditions and L lengths (scale bar = 1000 μm). | |
Vascularized tumor microenvironment on the VT-MAP
To reconstruct the vascularized tumor microenvironment (TME) with organoid or clusters within the device, endothelial cells (ECs), stromal cells, and tumor cells are required (Fig. 4a). Through the interaction with stromal cells within the hydrogel, endothelial cells construct a vascular network, while tumor cells expand their area through interaction with the surrounding environment. Due to differences in the proliferation rates between tumor cells and other cells, placing tumor cells in the same environment from the early stages when the vascular network is not yet formed is not suitable for observing the development process of the vascularized TME, as the number of tumor cells increases significantly. The VT-MAP allows fluid patterning in the side channel first, and over time, another fluid can be patterned in the center channel. In other words, the side channel can be filled first with a mixture of endothelial cells and stromal cells in hydrogel, allowing the construction of a vascular network over approximately two days. Afterward, in the center channel, a mixture of hydrogel, tumor cells, endothelial cells, and stromal cells is added to create a model of initial small tumor clusters located between healthy blood vessels. Tumor cells, derived from CRC patient-derived xenograft tumor tissues (Fig. S3a and b†), were cultured in the form of organoids. These organoids were embedded in a hydrogel matrix and introduced to the VT-MAP, facilitating the development of two distinct tumor microenvironments: a singular organoid and clusters of small organoids. The small organoid clusters were generated by dissociating the organoids to a single-cell level,42 emulating the dispersion of small tumor cells to secondary tumor sites, indicative of metastatic progression.43 CRC1 represents a patient who achieved complete recovery with anticancer drug treatment, while CRC2 originates from a patient whose cancer recurred after symptom relief, displaying resistance to anticancer drugs (Table 1). When the tumor is positioned in the center channel and cultured for about four more days, the vasculature sufficiently matured in the TME, and the vascular networks of the center channel and side channel connect (Fig. 4c). Moreover, the vascular network in the side channel is connected to the outer channel by introducing an EC suspension into the outer channel, attaching to the hydrogel interface, interacting with the infiltrated EC cells within the hydrogel, and resulting in an open lumen in the outer channel. A well-formed TME was confirmed by immune-cytochemistry, staining EC and tumor cells with fluorescence. The perfusability of the vascularized TME was observed by live imaging of the movement of microbeads through the open lumen (Fig. 4b), confirming their perfusability across the entire device, starting from one side of the outer channel, passing through the center channel, and reaching the opposite outer channel.
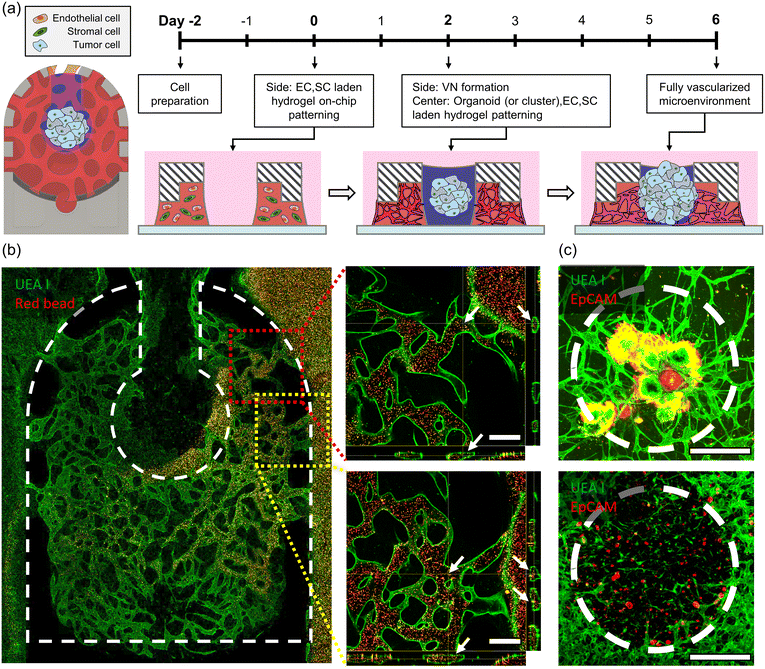 |
| Fig. 4 Vascularized tumor organoid in the VT-MAP. (a) Timeline of process to reconstruct vascularized tumor organoid microenvironment in the VT-MAP. EC: endothelial cell; SC: stromal cell; VN: vascular network. (b) Sectioned confocal image of vascular network in the VT-MAP. 2.0 μm fluorescent microbeads move into the perfusable vessel network. The red and yellow boxes respectively denote areas of perfused vessels on the right side of the VT-MAP. In the magnified image, cross sections of the perfused vessels (indicated by white arrows) and the presence of microbeads within the vessels can be observed (scale bar = 200 μm). (c) Confocal images of vascularized tumor organoid and tumor organoid cluster microenvironment in the VT-MAP (scale bar = 600 μm). | |
Table 1 Clinical information of colorectal cancer patients. MD: moderately differentiated; PD: poorly differentiated; MSS: microsatellite stable; XELOX: capecitabine and oxaliplatin; XELIRI: capecitabine and irinotecan
Case |
Age |
Sex |
Tumor location |
Cell type |
MSI status |
Stage |
1st chemotherapy |
2nd chemotherapy |
CRC 1 |
59 |
F |
Sigmoid colon |
MD |
MSS |
IV |
XELOX, 8 cycle |
|
CRC 2 |
60 |
F |
Splenic flexure colon |
PD |
MSS |
IV |
XELOX, 8 cycle |
XELIRI/simvastatin |
Drug treatment in tumor organoid cluster microenvironment on the VT-MAP
To investigate the drug responses of colorectal tumor organoid clusters, we utilized clinically used chemotherapeutics: 5-FU, oxaliplatin, and 5-FU + oxaliplatin for CRC1 and CRC2 samples.44,45 Tumor organoid clusters were placed in the center channel, and drug treatment was initiated two days later, with the drug responses confirmed 48 hours after treatment. The control group received no drug treatment, while the experimental groups had drugs mixed with the cell culture medium according to their concentrations and were reacted with the samples. After four days, when the tumor organoid clusters were almost matured, we compared the difference in drug concentration needed for a decrease in tumor cell viability between the second day of drug treatment and the control group matured for 48 hours. The drug concentrations used were screened log
2 based on the concentrations used in reported in vitro organ-on-a-chip and then adjusted for the IC50 in VT-MAP experiments. When cultivating a single tumor spheroid and treating drugs in a U-shaped well plate, the drug directly reached the tumor cells, showing sufficient response even at lower concentrations. However, the VT-MAP, using a scaled-up cell culture area with hydrogel and drug delivery through the vascular network, required relatively higher drug concentrations. There exists a gap compared to the concentrations used in clinical settings, which is a challenge to be addressed in the future. The VT-MAP focused on precise quantitative analysis of drug responses to confirm trends, emphasizing the need for accurate quantification in drug response analysis.
Quantification of drug response
The method of spheroid analysis typically involves cultivating spheroids in a well plate and then deriving results from a single spheroid per image.46–50 However, this approach is not suitable for high-throughput data analysis. In an experiment where an image contains spheroids of various sizes, the ability to obtain data on size-dependent drug responsiveness from a single image is essential for advancing to high-throughput analysis. By considering the characteristics of spheroids of different sizes, it is possible to uncover subtle data that may have been previously overlooked. In the VT-MAP, diverse tumor organoid clusters of various sizes and shapes constitute the TME within each well (Fig. 5a). Quantifying the individual cell viability of all tumor organoid clusters in the 14 wells of a single device requires significant labor. To streamline and quantify the analysis, we employed an algorithm using confocal images acquired from VT-MAP. The algorithm, developed based on OpenCV using Python, aims for more precise drug response analysis (Fig. 5b). All samples were stained with EpCAM and PI, where the green signal represents EpCAM-stained tumor cells, and the red signal indicates cell death due to PI staining (Fig. 5c). The existing algorithm has two drawbacks in calculating cell viability based on the red signal/green signal across the entire region of interest (ROI): (1) inclusion of dead signals from surrounding ECs and stromal cells hinders the quantification of drug response only for tumors, (2) the ability to compare drug response differences for individual tumor clusters is limited. To address these issues, we improved the algorithm by recognizing individual green signal areas within the ROI and calculating the overlapping dead signal for each individual area. This enhancement allowed us to confirm the drug response for each tumor cluster, revealing differences in drug response based on the size of tumor clusters. This finding is anticipated to be instrumental in drug development for metastatic situations.
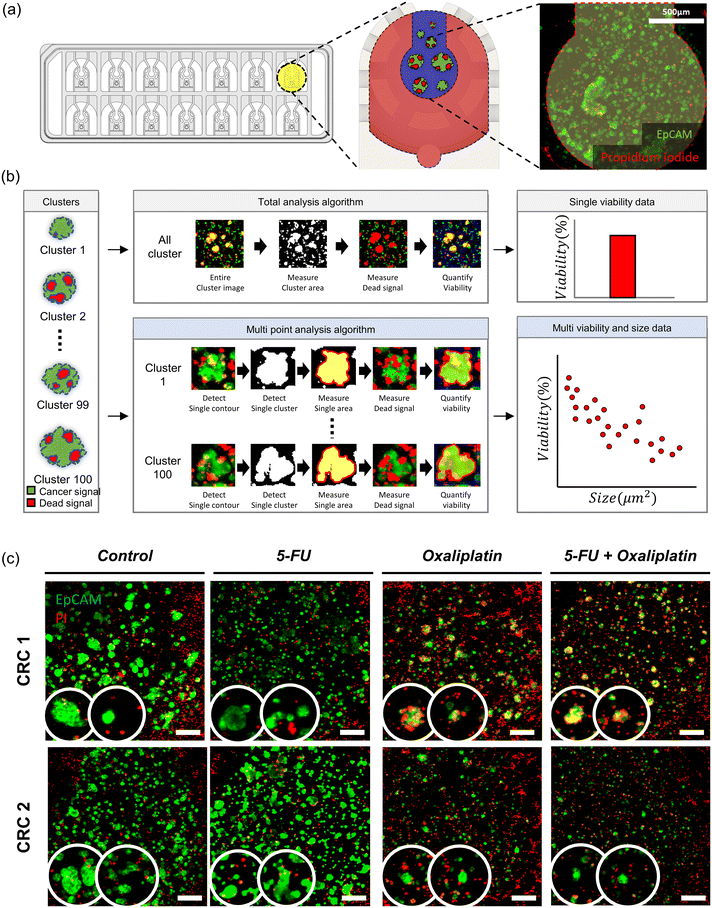 |
| Fig. 5 Advanced organoid cluster analysis algorithm by computer vision. (a) The provided image from the VT-MAP presents the live/dead assay results of drug treatment, illustrating clusters of diverse sizes. Although composed of identical cells, there is a noticeable difference in cluster viability contingent on the cluster size. (b) Traditionally, the analysis revolves around a singular cluster within a well, aggregating the overall viability by collectively considering both live and dead signals present in the image. The advanced algorithm facilitates individualized calculation of size-specific viability for every multi-cluster, thus deriving their interrelationship. (c) Confocal images of drug response from patient #1 and #2 in the VT-MAP (scale bar = 200 μm). | |
Images should undergo preprocessing due to numerous noises that interfere with the individual detection of tumor organoid clusters. Firstly, overall brightness was adjusted through histogram equalization and normalization techniques. Then, blob removal and blur processes were implemented to denoise images, facilitating the contour detection of each cluster. Thereafter, the contour of clusters was separated independently, and individual cluster viability was measured by computing red signals overlapped with the green signals. Finally, average viability was quantified in groups classified by cluster size in 500 μm2 intervals. This method enables the exploration of the relationship between cluster size and viability, unlike conventional methods.
Fig. 6c presents viability plotted based on cluster size, providing a more detailed analysis than a simple viability assessment. In the control group, CRC1 and CRC2 formed larger clusters with a wide size distribution, reaching up to approximately 6.0 × 104 μm2 and 3.2 × 104 μm2, respectively, with high viability ranging from 80% to 95%. In the drug-treated group, both 5-FU 200 μM and oxaliplatin 200 μM led to size reductions, with CRC1 clusters reaching a maximum of 2.0 × 104 μm2 and CRC2 clusters reaching a maximum of 1.0 × 104 μm2. Regarding 5-FU, both CRC1 and CRC2 showed viability similar to or slightly higher than the control for all cluster sizes, indicating that 5-FU had limited effects. In contrast, oxaliplatin showed a 20–50% cell viability depending on the cluster size in CRC1, and CRC2 exhibited 70–80% cell viability. This suggests that while the drugs commonly inhibit the increase in tumor size, there is a significant reduction in viability, particularly for larger clusters. Additionally, it can be observed that CRC2 exhibits high resistance to oxaliplatin.
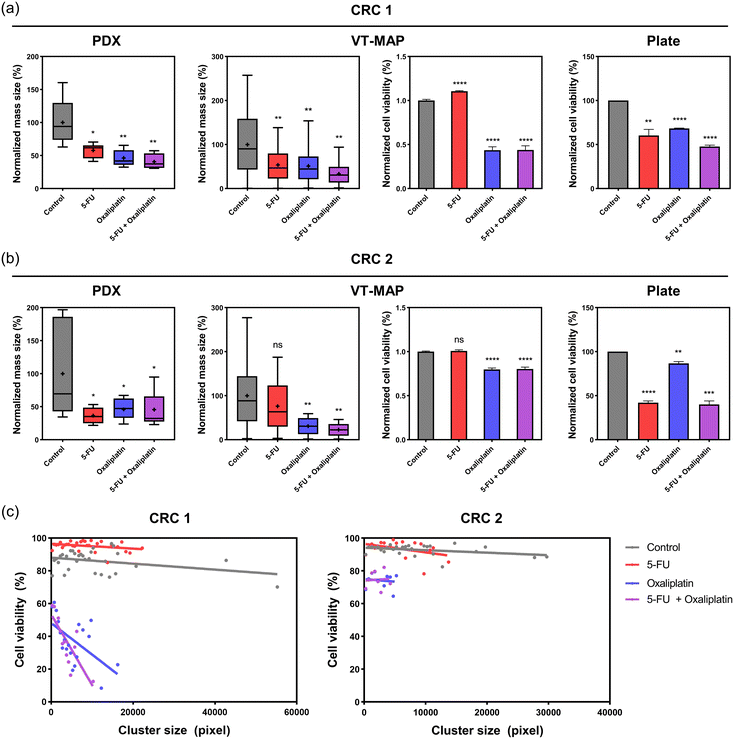 |
| Fig. 6 Quantitative analysis of drug response in terms of cell mass and cell viability in PDX, VT-MAP, and plate. (a) and (b) Changes in mass size and cell viability as drug response in PDX, VT-MAP, and plate. 5-FU, oxaliplatin, and the combination of both drugs were tested in each platform. Cancer cells from patient #2 showed drug-resistant results compared to that of patient #1. (c) Graphs of cell viability related to cluster size in the VT-MAP. The control group showed high cell viability with large cluster size. The 5-FU group showed high viability but small cluster size. Oxaliplatin and the combination group showed low cell viability with small cluster size. | |
Drug response on conventional in vitro model
Prior to assessing the drug sensitivity of vascularized tumor organoids cultured on the VT-MAP, we conducted an initial evaluation of the drug response of tumor organoids in 3D organoid culture using 96-well plates (Fig. S1a and b†). We assessed the first-line chemotherapies for CRC as on the VT-MAP. Tumor organoids were treated with two different doses of drugs for a duration of 3 days, following which organoid viability was determined. Notably, we observed disparate responses to first-line chemotherapies across tumor organoids derived from different CRC patients. Organoids from CRC1 showed over 50% cell viability for all drug groups, while organoids from CRC2 exhibited close to 90% viability only with oxaliplatin and showed values lower than 50% for the other two drug groups (Fig. 6a and b).
Drug response on xenograft
In parallel with confirming drug responses in the conventional in vitro model of 3D organoid culture in 96-well plates, we evaluated the drug sensitivity in patient-derived PDX models to compare with the results on the VT-MAP. Single drug groups (5-FU, oxaliplatin) were administered to mice once every three days over a span of eight doses, while the combination drug group (5-FU + oxaliplatin) was administered once every seven days over four doses (Fig. S2†). The drug experiments in xenografts were conducted over a period of 32 days. CRC1-derived xenograft tumor tissue showed a decrease of over 50% in mass size compared to the control for all drug groups. In contrast, CRC2-derived xenograft tumor tissue exhibited a decrease of over 50% in mass size for 5-FU, while displaying reductions of less than 50% for the other two drug groups (Fig. 6a and b).
Reliability of drug response in the VT-MAP
We compared the drug response evaluations of chemotherapeutics for CRC1 and CRC2 across patient PDX models, VT-MAP, and organoid culture in 96-well plates. Utilizing cells obtained from CRC patient-derived xenograft tumor tissues in all three platforms, we measured tumor mass size changes in PDX and cell viability changes in organoid culture in 96-well plates, depending on the platform's characteristics. The VT-MAP allows for the confirmation of both mass size and cell viability changes through fluorescence images of tumor organoid clusters of various sizes and shapes in a single well, establishing the correlation. This correlation revealed results that were not discernible when only analyzing mass size and cell viability separately. The drug showed a higher efficacy in killing larger clusters, while it was less effective on smaller clusters. Hence, based on our hypothesis, it was evident that the drug was less effective on smaller clusters, which correspond to the early stages of metastasis. Additionally, it was observed that clusters of similar size, regardless of their position within the center channel, exhibited comparable responses to the drug (Fig. 6c).
For CRC1 in PDX, there was an approximately 40% decrease in mass size compared to the control with 5-FU, and over 50% reduction in mass size for the other groups. In the 96-well plate, there was an approximately 40% decrease in cell viability with 5-FU and oxaliplatin, and around 50% decrease with 5-FU + oxaliplatin. The VT-MAP demonstrated approximately 50% mass size reduction with 5-FU and oxaliplatin, and about 60% mass size reduction with 5-FU + oxaliplatin. Cell viability showed almost no change with 5-FU and approximately 60% reduction for the other groups. Drug evaluations for CRC1 were consistent across all platforms (Fig. 6a).
For CRC2 in PDX, there was approximately 50% decrease in mass size for all drug treatment groups compared to the control. In the 96-well plate, oxaliplatin showed similar cell viability to the control, while the other two conditions exhibited over 60% reduction in cell viability. In the VT-MAP, there was approximately a 20% mass size reduction with 5-FU and over 50% reduction with the other two conditions. Unlike mass size changes, cell viability in the VT-MAP remained almost similar to the control with 5-FU and was around 80% for the other two conditions. Unlike CRC1, CRC2 exhibited platform-dependent differences in drug response (Fig. 6b).
Clinical results indicated improvement with capecitabine + oxaliplatin (XELOX) as the first treatment for CRC1, while CRC2 experienced recurrence post-XELOX and received capecitabine + irinotecan (XELIRI) therapy (Table 1). Therefore, CRC1 exhibited responsiveness to oxaliplatin, whereas CRC2 demonstrated resistance to oxaliplatin. Organoid culture in 96-well plates, considered a conventional in vitro model, showed lower responsiveness than clinical data for CRC1 but exhibited a similar trend. However, for CRC2, cell viability did not decrease with oxaliplatin, but a significant reduction was observed in combination with 5-FU. In contrast, the VT-MAP demonstrated trends similar to clinical data for both CRC1 and CRC2.
While in vitro devices have been extensively studied for various biological applications, animal experimentation results are more reliable for drug response evaluation in drug development. The lower reliability of in vitro devices is attributed to their lower complexity compared to in vivo models and the occurrence of patterns different from actual clinical responses. Unlike traditional in vitro models, the VT-MAP demonstrated drug response evaluations similar to clinical outcomes using patient samples. Additionally, the VT-MAP allows the observation and correlation of both mass size and cell viability changes, unlike PDX and 96-well plates, providing valuable insights.
Examining the drug response results for CRC1 and CRC2 in the VT-MAP reveals excellent efficacy in reducing the size of tumor organoid clusters. However, for larger clusters, there is a pronounced effect in reducing cell viability, whereas for smaller clusters, the impact on cell viability is relatively lower (Fig. 6c). This implies that the VT-MAP has the potential to quickly and efficiently assess drug responses when tumor clusters arise at a second tumor site in metastatic situations. Thus, the VT-MAP suggests potential applications in drug development and confirming patient-specific drug responses.
Conclusions
In this research, we introduced an innovative platform named VT-MAP, a scaled-up vascularized tissue micromesh-assisted system, for the assessment of organoids. Overcoming limitations inherent in prior rail-assisted models,17,23,51 the VT-MAP facilitates the efficient co-culture of scaled-up tissues, encompassing vascularized tissues and organoids. The VT-MAP faithfully reproduces the intricate tumor microenvironment, fostering the growth and interaction of clusters with diverse sizes and shapes.
From the experimental results, the VT-MAP is implied as a valuable instrument for comprehensive analyses, encompassing cluster size, viability, and drug responses. It not only enables the exploration of the intricate relationship between cluster size and viability, a challenge in prior in vitro models, but also facilitates a more detailed analysis. Critically, the experimental findings with the VT-MAP exhibited a resemblance to in vivo conditions, differentiating it from previous in vitro models. This research significantly contributes to replicating a realistic tumor microenvironment and investigating cellular behaviors based on cluster size and viability using the VT-MAP.
Moreover, the VT-MAP accurately mirrors the heterogeneity of organoid clusters, furnishing results more representative of in vivo conditions, thereby advancing drug screening and research. In conclusion, the VT-MAP stands as an authentic and advanced platform for researching scaled-up vascularized tissues or organoids, providing novel insights into the tumor microenvironment. Its role extends to enhancing our comprehension of cancer treatment and tumor biology, offering a potential cornerstone in the development of personalized therapeutic strategies.
Author contributions
Jungseub Lee: conceptualization, data curation, formal analysis, investigation, methodology, resources, software, validation, visualization, writing – original draft. Sangmin Jung: conceptualization, data curation, formal analysis, investigation, methodology, resources, validation, visualization, writing – original draft. Hye Kyoung Hong: conceptualization, data curation, formal analysis, investigation, methodology, resources, validation, visualization, writing – original draft. Hyeonsu Jo: investigation, validation. Stephen Rhee: investigation, validation. Ye-Lin Jeong: resources. Jihoon Ko: funding acquisition, writing – review & editing. Yong Beom Cho: conceptualization, data curation, funding acquisition, investigation, methodology, project administration, supervision, writing – review & editing. Noo Li Jeon: conceptualization, data curation, funding acquisition, investigation, methodology, project administration, supervision, writing – review & editing.
Conflicts of interest
There are no conflicts to declare.
Acknowledgements
This work was supported by the National Research Foundation of Korea (NRF) grant funded by the Korea government (MSIT) (NRF-2021R1A3B1077481, NRF-2022M3A9B6082680, NRF-2022M3A9G8084563), the Institute of Information & Communications Technology Planning & Evaluation (IITP) grant (no. 2019-0-00047, Development of a patient-derived tumor tissue-based 3D printed cancer chip for refractory cancer drug screening) and Future Medicine 20*30 Project of the Samsung Medical Center (#SMO1230021), and the Gachon University research fund of 2023 (GCU-202301150001).
Notes and references
- F. Pampaloni, E. G. Reynaud and E. H. K. Stelzer, Nat. Rev. Mol. Cell Biol., 2007, 8, 839–845 CrossRef CAS PubMed
.
- M. Kapałczyńska, T. Kolenda, W. Przybyła, M. Zajączkowska, A. Teresiak, V. Filas, M. Ibbs, R. Bliźniak, Ł. Łuczewski and K. Lamperska, Arch. Med. Sci., 2018, 14, 910–919 Search PubMed
.
- E. C. Costa, A. F. Moreira, D. de Melo-Diogo, V. M. Gaspar, M. P. Carvalho and I. J. Correia, Biotechnol. Adv., 2016, 34, 1427–1441 CrossRef PubMed
.
- M. Ravi, V. Paramesh, S. R. Kaviya, E. Anuradha and F. D. P. Solomon, J. Cell. Physiol., 2015, 230, 16–26 CrossRef CAS PubMed
.
- C. M. Leung, P. de Haan, K. Ronaldson-Bouchard, G.-A. Kim, J. Ko, H. S. Rho, Z. Chen, P. Habibovic, N. L. Jeon, S. Takayama, M. L. Shuler, G. Vunjak-Novakovic, O. Frey, E. Verpoorte and Y.-C. Toh, Nat. Rev. Methods Primers, 2022, 2, 33 CrossRef CAS
.
- D. Huh, H. J. Kim, J. P. Fraser, D. E. Shea, M. Khan, A. Bahinski, G. A. Hamilton and D. E. Ingber, Nat. Protoc., 2013, 8, 2135–2157 CrossRef CAS PubMed
.
- D. Kim, K. S. Hwang, E. U. Seo, S. Seo, B. C. Lee, N. Choi, J. Choi and H. N. Kim, Adv. Healthcare Mater., 2022, 11, 2102581 CrossRef CAS PubMed
.
- S. Kim, M. Chung, J. Ahn, S. Lee and N. L. Jeon, Lab Chip, 2016, 16, 4189–4199 RSC
.
- N. E. Clay, K. Shin, A. Ozcelikkale, M. K. Lee, M. H. Rich, D. H. Kim, B. Han and H. Kong, ACS Biomater. Sci. Eng., 2016, 2, 1968–1975 CrossRef CAS PubMed
.
- J. Shin, J. Ko, S. Jeong, P. Won, Y. Lee, J. Kim, S. Hong, N. L. Jeon and S. H. Ko, Nat. Mater., 2021, 20, 100–107 CrossRef CAS PubMed
.
- N. R. Wevers, D. G. Kasi, T. Gray, K. J. Wilschut, B. Smith, R. van Vught, F. Shimizu, Y. Sano, T. Kanda, G. Marsh, S. J. Trietsch, P. Vulto, H. L. Lanz and B. Obermeier, Fluids Barriers CNS, 2018, 15, 23 CrossRef PubMed
.
- D. E. Ingber, Nat. Rev. Genet., 2022, 23, 467–491 CrossRef CAS PubMed
.
- J. Ahn, D.-H. Kim, D.-J. Koo, J. Lim, T.-E. Park, J. Lee, J. Ko, S. Kim, M. Kim, K.-S. Kang, D.-H. Min, S.-Y. Kim, Y. Kim and N. L. Jeon, Acta Biomater., 2023, 165, 153–167 CrossRef CAS PubMed
.
- B. F. L. Lai, R. X. Z. Lu, Y. Hu, L. Davenport Huyer, W. Dou, E. Y. Wang, N. Radulovich, M. S. Tsao, Y. Sun and M. Radisic, Adv. Funct. Mater., 2020, 30, 2000545 CrossRef CAS PubMed
.
- Y. Nashimoto, R. Okada, S. Hanada, Y. Arima, K. Nishiyama, T. Miura and R. Yokokawa, Biomaterials, 2020, 229, 119547 CrossRef CAS PubMed
.
- H. Ragelle, K. Dernick, S. Khemais, C. Keppler, L. Cousin, Y. Farouz, C. Louche, S. Fauser, S. Kustermann, M. W. Tibbitt and P. D. Westenskow, Adv. Healthcare Mater., 2020, 9, 2001531 CrossRef CAS PubMed
.
- V. S. Shirure, Y. Bi, M. B. Curtis, A. Lezia, M. M. Goedegebuure, S. P. Goedegebuure, R. Aft, R. C. Fields and S. C. George, Lab Chip, 2018, 18, 3687–3702 RSC
.
- Y. Wang, L. Wang, Y. Guo, Y. Zhu and J. Qin, RSC Adv., 2018, 8, 1677–1685 RSC
.
- V. van Duinen, S. J. Trietsch, J. Joore, P. Vulto and T. Hankemeier, Curr. Opin. Biotechnol., 2015, 35, 118–126 CrossRef CAS PubMed
.
- S. H. Au, M. D. Chamberlain, S. Mahesh, M. V. Sefton and A. R. Wheeler, Lab Chip, 2014, 14, 3290–3299 RSC
.
- J. Lim, H. Ching, J. K. Yoon, N. L. Jeon and Y. Kim, Nano Convergence, 2021, 8, 12 CrossRef CAS PubMed
.
- A. Paradiso, M. Volpi, C. Rinoldi, N. Celikkin, N. Contessi Negrini, M. Bilgen, G. Dallera, F. Pierini, M. Costantini, W. Swieszkowski and S. Fare, Biomater. Sci., 2023, 11, 2988–3015 RSC
.
- N. Brandenberg, S. Hoehnel, F. Kuttler, K. Homicsko, C. Ceroni, T. Ringel, N. Gjorevski, G. Schwank, G. Coukos, G. Turcatti and M. P. Lutolf, Nat. Biomed. Eng., 2020, 4, 863–874 CrossRef CAS PubMed
.
- K. A. Homan, N. Gupta, K. T. Kroll, D. B. Kolesky, M. Skylar-Scott, T. Miyoshi, D. Mau, M. T. Valerius, T. Ferrante, J. V. Bonventre, J. A. Lewis and R. Morizane, Nat. Methods, 2019, 16, 255–262 CrossRef CAS PubMed
.
- Y. Kim, J. Ko, N. Shin, S. Park, S.-R. Lee, S. Kim, J. Song, S. Lee, K.-S. Kang, J. Lee and N. L. Jeon, Biotechnol. Bioeng., 2022, 119, 3678–3693 CrossRef CAS PubMed
.
- J. Ko, J. Ahn, S. Kim, Y. Lee, J. Lee, D. Park and N. L. Jeon, Lab Chip, 2019, 19, 2822–2833 RSC
.
- B. Lee, S. Kim, J. Ko, S.-R. Lee, Y. Kim, S. Park, J. Kim, S. Hyung, H.-Y. Kim and N. L. Jeon, NPG Asia Mater., 2022, 14, 6 CrossRef CAS
.
- M. Hofer and M. P. Lutolf, Nat. Rev. Mater., 2021, 6, 402–420 CrossRef CAS PubMed
.
- K. E. de Visser and J. A. Joyce, Cancer Cell, 2023, 41, 374–403 CrossRef CAS PubMed
.
- R. Baghban, L. Roshangar, R. Jahanban-Esfahlan, K. Seidi, A. Ebrahimi-Kalan, M. Jaymand, S. Kolahian, T. Javaheri and P. Zare, Cell Commun. Signaling, 2020, 18, 59 CrossRef PubMed
.
- L. Bejarano, M. J. C. Jordāo and J. A. Joyce, Cancer Discovery, 2021, 11, 933–959 CrossRef CAS PubMed
.
- Y. Shintani, T. Kimura, S. Funaki, N. Ose, T. Kanou and E. Fukui, Cancers, 2023, 15, 335 CrossRef CAS PubMed
.
- M. Chung, J. Ahn, K. Son, S. Kim and N. L. Jeon, Adv. Healthcare Mater., 2017, 6, 1700196 CrossRef PubMed
.
- R. K. Jain, P. Au, J. Tam, D. G. Duda and D. Fukumura, Nat. Biotechnol., 2005, 23, 821–823 CrossRef CAS PubMed
.
- C. J. Kirkpatrick, S. Fuchs and R. E. Unger, Adv. Drug Delivery Rev., 2011, 63, 291–299 CrossRef CAS PubMed
.
- S. V. Murphy and A. Atala, Nat. Biotechnol., 2014, 32, 773–785 CrossRef CAS PubMed
.
- S. Vijayavenkataraman, W.-C. Yan, W. F. Lu, C.-H. Wang and J. Y. H. Fuh, Adv. Drug Delivery Rev., 2018, 132, 296–332 CrossRef CAS PubMed
.
- S. Zhang, Z. Wan and R. D. Kamm, Lab Chip, 2021, 21, 473–488 RSC
.
- H. K. Hong, N. H. Yun, Y.-L. Jeong, J. Park, J. Doh, W. Y. Lee and Y. B. Cho, Cancer Med., 2021, 10, 5589–5598 CrossRef CAS PubMed
.
- F. Eduati, R. Utharala, D. Madhavan, U. P. Neumann, T. Longerich, T. Cramer, J. Saez-Rodriguez and C. A. Merten, Nat. Commun., 2018, 9, 2434 CrossRef PubMed
.
- Y. Lee, J. W. Choi, J. Yu, D. Park, J. Ha, K. Son, S. Lee, M. Chung, H.-Y. Kim and N. L. Jeon, Lab Chip, 2018, 18, 2433–2440 RSC
.
- H. K. Hong, D. H. Pyo, T. W. Kim, N. H. Yun, Y. S. Lee, S. J. Song, W. Y. Lee and Y. B. Cho, Oncol. Rep., 2019, 42, 2029–2038 CAS
.
- C. L. Chaffer and R. A. Weinberg, Science, 2011, 331, 1559–1564 CrossRef CAS PubMed
.
- S. Vodenkova, T. Buchler, K. Cervena, V. Veskrnova, P. Vodicka and V. Vymetalkova, Pharmacol. Ther., 2020, 206, 107447 CrossRef CAS PubMed
.
- R. M. McQuade, V. Stojanovska, J. C. Bornstein and K. Nurgali, Curr. Med. Chem., 2017, 24, 1537–1557 CrossRef CAS PubMed
.
- F. Mittler, P. Obeïd, A. V. Rulina, V. Haguet, X. Gidrol and M. Y. Balakirev, Front. Oncol., 2017, 7, 293 CrossRef PubMed
.
- T. S. Ramasamy, J. S. L. Yu, C. Selden, H. Hodgson and W. Cui, Tissue Eng., Part A, 2013, 19, 360–367 CrossRef CAS PubMed
.
- A. Sargenti, F. Musmeci, F. Bacchi, C. Delprete, D. A. Cristaldi, F. Cannas, S. Bonetti, S. Pasqua, D. Gazzola, D. Costa, F. Villa, M. R. Zocchi and A. Poggi, Front. Immunol., 2020, 11, 564887 CrossRef CAS PubMed
.
- M. Zoetemelk, M. Rausch, D. J. Colin, O. Dormond and P. Nowak-Sliwinska, Sci. Rep., 2019, 9, 7103 CrossRef PubMed
.
- X. Gong, C. Lin, J. Cheng, J. S. Su, H. Zhao, T. L. Liu, X. J. Wen and P. Zhao, PLoS One, 2015, 10(6), e0130348 CrossRef PubMed
.
- H. J. Pandya, K. Dhingra, D. Prabhakar, V. Chandrasekar, S. K. Natarajan, A. S. Vasan, A. Kulkarni and H. Shafiee, Biosens. Bioelectron., 2017, 94, 632–642 CrossRef CAS PubMed
.
|
This journal is © The Royal Society of Chemistry 2024 |
Click here to see how this site uses Cookies. View our privacy policy here.