DOI:
10.1039/D3LC00821E
(Critical Review)
Lab Chip, 2024,
24, 1154-1174
Microfluidic synthesis of lipid-based nanoparticles for drug delivery: recent advances and opportunities
Received
28th September 2023
, Accepted 7th December 2023
First published on 2nd January 2024
Abstract
Microfluidic technologies are revolutionizing the synthesis of nanoscale lipid particles and enabling new opportunities for the production of lipid-based nanomedicines. By harnessing the benefits of microfluidics for controlling diffusive and advective transport within microfabricated flow cells, microfluidic platforms enable unique capabilities for lipid nanoparticle synthesis with precise and tunable control over nanoparticle properties. Here we present an assessment of the current state of microfluidic technologies for lipid-based nanoparticle and nanomedicine production. Microfluidic techniques are discussed in the context of conventional production methods, with an emphasis on the capabilities of microfluidic systems for controlling nanoparticle size and size distribution. Challenges and opportunities associated with the scaling of manufacturing throughput are discussed, together with an overview of emerging microfluidic methods for lipid nanomedicine post-processing. The impact of additive manufacturing on current and future microfluidic platforms is also considered.
Introduction
Lipid-based nanomedicines employ vesicles formed from lipid membranes to sequester therapeutic agents within the nanoscale particles. The vesicular structure serves to protect the internal cargo from metabolic activity while simultaneously enhancing bioavailability and reducing immunogenicity and systemic toxicity.1 The lipid membrane further provides a route to surface functionalization, enabling the nanoparticles to be tailored for targeted delivery to specific cells and tissues. Unlike many inorganic and synthetic polymer nanocarriers, lipid nanoparticles offer a high level of biocompatibility and may be loaded with a wide variety of therapeutic compounds including small molecules, polymers, peptides, and proteins, enabling lipid nanomedicines to be successfully harnessed for broad applications in chemotherapy,2 immunotherapy,3 vaccines delivery,4 and antimicrobial treatment,5 gene therapy,6 genome editing,7 and beyond.
A range of synthetic lipid constructs have been employed for drug delivery, as presented in Fig. 1. Nanoscale liposomes comprising lipid bilayer vesicles surrounding an aqueous core offer the ability to encapsulate both hydrophilic and hydrophobic compounds, making them well suited to the delivery of a wide variety of therapeutic agents with significant clinical success over the past several decades.8,9 Lipid nanoparticles containing cationic lipids have similarly emerged as attractive vehicles for the delivery of nucleic acids, with cationic lipids interacting electrostatically with negatively-charged nucleic acids during vesicle formation to generate stable lipoplex structures.10,11 While lipoplexes have been shown to provide high encapsulation efficiency, they can suffer from poor pharmacokinetics and toxicity due to the presence of charged lipids in the outer membrane surface. To overcome this limitation, ionizable lipids can be used to form charge-stabilized lipid nanoparticle (LNP) structures consisting of lipid vesicles surrounding lipid-nucleic acid complexes. When exposed to a pH shift under physiological conditions, charge neutralization of the ionizable lipid components occurs to enable efficient drug release.12,13 We note that while all nanoparticles depicted in Fig. 1 are lipid nanoparticles, the LNP acronym is often used to refer specifically to nanoparticles formed from ionizable lipids and nucleic acids, and this terminology is employed in this review. Lipid-based particles such as solid lipid nanoparticles (SLNs)14,15 and nanostructured lipid carriers (SLCs),16 which consist of a lipid or surfactant monolayer surrounding a solid lipid core or a mixture of solid and fluid lipids, respectively, have been developed to enable higher loading capacities for lipophilic drugs while also improving colloidal stability of the resulting nanoparticles. Lipid nanoemulsions consisting of lipid monolayers enclosing solvated liquid-phase lipid interiors can enable efficient delivery of water-insoluble agents.17 Liposome-like ethosomes retain a high concentration of solvent within the lipid bilayer to confer mechanical flexibility enabling efficiency penetration through dermal layers.18,19 Non-spherical cubosomes are formed from cubic phase lipids as a liquid crystal dispersion containing discrete aqueous chambers within the cubosome core.20,21 Lipid-coated nanoparticles represent a broad class of particles in which a solid core (typically a synthetic or natural polymer) is wrapped in a lipid membrane.22 These nanoparticles offer high drug loading levels and mechanical stability, together with improved bioavailability, biocompatibility, and surface functionalization provided by the lipid membrane.23,24 Various other vesicular nanoparticles not explicitly presented in Fig. 1 (e.g. transfersomes, bilosomes, and glycerosomes) have also been developed, and a number of non-vesicular lipid nanostructures such as lipid micelles have similarly been explored for drug delivery.25 Overall, the structural diversity of lipid-based nanoparticles supporting multifunctional drug loading, together with the wide range of lipid species available to control membrane characteristics, including tunable stimulus-responsive lipids,26 provides a high level of flexibility for the design of new nanomedicines with tailored properties.
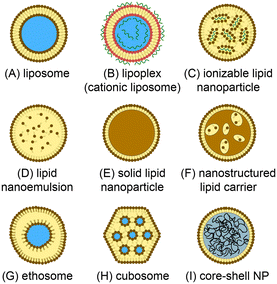 |
| Fig. 1 Lipid-based nanoparticles for drug delivery. (A) Unilamellar liposome with a bilayer lipid membrane surrounding an aqueous core. (B) Lipoplex containing cationic lipids and charge-immobilized nucleic acids. (C) Lipid nanoparticle formed with ionizable lipids encapsulating lipid/nucleic acid complexes. (D) Lipid nanoemulsion with a lipid monolayer enclosing a solvated lipid interior. (E) Solid phase nanoparticle with a lipid monolayer surrounding a solid-phase lipid core. (F) Nanostructured lipid carrier containing a mixture of lipid liquid and solid phases. (G) Ethosome with a high concentration of solvent within the lipid bilayer. (H) Cubosome formed from a lipid cubic phase. (I) Core–shell nanoparticle encapsulating a hydrophobic polymer core. | |
The modern era of lipid-based nanotherapeutics began in 1965 with the development of liposomes27 and their encapsulation of enzymes,28,29 chemotherapeutics,30 and immunologic adjuvants31 over the following decade. Although numerous organic and inorganic nanoparticles have since been explored for applications in drug delivery, lipid-based systems remain the most studied, and most commercially successful, class of nanotherapeutic vehicle. Liposomal doxorubicin (Doxil) was the first nanomedicine approved by the U.S. Food and Drug Administration (FDA) in 1995,32 and liposomes continue to represent the largest share of nanoparticle-enabled drugs used in clinical practice. Taken together, the development of nanotherapeutics based on liposomes, LNPs, and related lipid-based nanoparticles continues to experience significant growth for drug delivery applications ranging from vaccines to gene therapy. A recent analysis identified 126 U.S. clinical trials between 2016 and 2021 employing lipid-based nanoparticles, representing over half of all nanoparticle drugs undergoing trials during that period.33 Furthermore, of the 15 nanomedicines approved by the FDA during the same period, nearly half employed either liposomes or LNPs as drug carriers.
An important advantage of lipid-based nanomedicines is that the particles may be readily tailored to target selected cells or tissues through functionalization of the lipid membrane using ligands including small molecules, peptides, antibodies, or other proteins. Functionalization may be achieved by introducing lipids conjugated with the desired ligands during nanoparticle synthesis, or by modifying the membrane after vesicle formation.34–36 An effective strategy for membrane functionalization is by linking ligands to the terminus of polyethylene glycol (PEG) molecules conjugated with lipids used during nanoparticle synthesis. In addition to providing a flexible route to ligand attachment, the PEG chains can serve to tether the ligands away from the nanoparticle to improve interactions with target receptors on the cell surface. The inclusion of PEGylated lipids in the initial lipid mixture used for nanoparticle formation is also commonly used to generate so-called stealth nanoparticles that can bioavailability by shielding the particles from phagocytosis, significantly extending their time in blood circulation.37 Lipid mixtures may also be selected to achieve selective release of the drug cargo, for example by employing pH-sensitive lipids that are weakly anionic at neutral pH but lose their charge upon entering the acidic environment within tumor tissues, thereby destabilizing the nanoparticles to release their contents.38 An important application of pH-sensitive lipids is in the formation of ionizable LNPs encapsulating nucleic acids including siRNA and mRNA. These nanoparticles are typically produced by employing ionizable cationic lipids within the initial lipid mixture, presenting a positive charge at low pH to facilitate nucleic acid complexation and entrapment during LNP formation, but becoming neutral under physiologic conditions for efficient cargo release. LNPs have emerged as an important vehicle for mRNA delivery,39 having proven central to the success of COVID-19 mRNA vaccines from Moderna and Pfizer/BioNTech.4
The field of microfluidics has yielded powerful new approaches to the preparation of lipid nanomedicines. By leveraging microfabrication techniques to pattern high resolution and small scale channels and other fluidic structures with precise geometric control, microfluidic platforms can manipulate transport and reaction kinetics at length and time scales smaller than conventionally-machined fluidic systems. As a result, microfluidics may be harnessed to synthesize nanoparticles with improved control over properties including average size, size distribution, morphology, and surface functionality.40 Numerous reviews addressing the application of microfluidic technologies to nanomedicine preparation have been published in recent years,41–67 reflecting the significant growth in interest towards leveraging microfluidics for nanotherapeutic development. A diverse set of reviews specifically evaluating the state of the art in microfluidic systems for the synthesis of liposomes,68–70 LNPs,39,71–75 or a combination of both categories of nanoparticles76–82 has also appeared over the past decade. In the present review, we focus on evaluating the current state of microfluidic technologies for the production of lipid-based nanoparticles, with an emphasis on comparing the capabilities of these systems for lipid nanoparticle size control, evaluating progress in microfluidic platforms integrating multiple process steps in the nanomedicine production pipeline, and summarizing recent advances towards the development of microfluidic technologies designed for high-throughput lipid nanomedicine production. In this context, the impact of additive manufacturing on the field is considered, and challenges and opportunities for future microfluidic systems in lipid-based nanoparticle synthesis are discussed.
Discussion
Conventional methods for lipid-based nanoparticle preparation
Lipid-based nanoparticle formation occurs through a self-assembly process, with final particle morphology dictated by a combination of thermodynamic state, inter-lipid interaction forces, and molecular geometry.83 Classical methods for the production of lipid nanoparticles may be categorized by the physical mechanism controlling the kinetics of self-assembly, with the most widely used processes based on mechanical homogenization, solvent dilution, or detergent removal.84,85 A summary of common techniques leveraging each mechanism is presented in Fig. 2. Other mechanisms not represented in this figure include emulsification through the replacement of a water-immiscible solvent by the aqueous phase, e.g. via reverse-phase evaporation in which inverted micelles are converted to bilayer vesicles upon solvent evaporation,86–88 aqueous phase removal via lyophilization by removing water ice from a frozen aqueous solution containing the lipid formulation by sublimation,89 and various supercritical fluid-enabled nanoparticle production methods.90,91
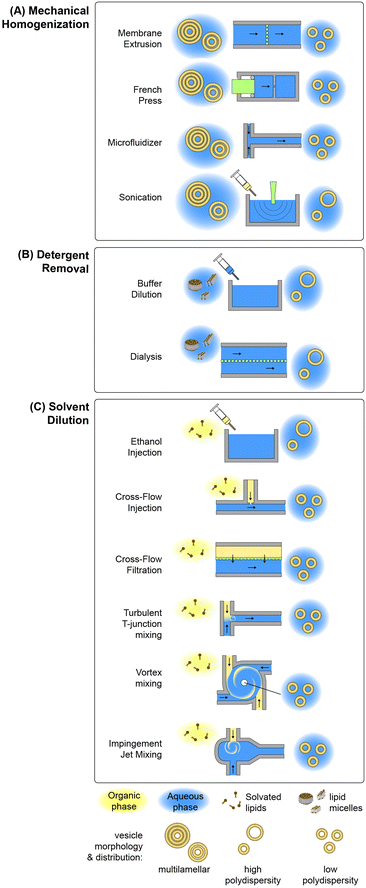 |
| Fig. 2 Conventional lipid-based nanoparticle preparation methods. Summary of conventional techniques for lipid-based nanoparticle self-assembly employing (A) mechanical shear-based homogenization, (B) solvent diffusion, or (C) detergent removal. | |
In the case of mechanical homogenization, large and polydisperse multilamellar vesicles (MLVs) are first prepared using a technique such as lipid film hydration, and the resulting emulsion is exposed to high pressure gradients or shear forces that serve to break apart the large vesicles, exposing the hydrophobic membrane core to the aqueous phase and allowing the resulting fragments to reform as smaller unilamellar vesicles. An advantage of mechanical disruption is that the initial MLV solution is aqueous, eliminating the need for organic solvents in the preparation process. Sonication is a common mechanical technique for small-volume liposome synthesis in which ultrasonic energy generates cavitation within the lipid solution, resulting in high transmembrane pressure gradients that rupture the multilamellar vesicles.92 While simple to implement, this technique can degrade lipids under high ultrasonic power, and tends to result in polydisperse vesicles with limited size control. Improved size control can be achieved using a French pressure cell,93 which belongs to the class of high pressure homogenization methods. In this process, an MLV suspension contained in a sealed chamber is forced through a narrow orifice under high pressure (>100 atm), resulting in membrane disruption by the generation of high shear stresses. A variation on the French cell that can be operated in a continuous-flow mode is the microfluidizer, which employs merging channels with diameters on the order of 100 μm to produce high shear stresses while operating at flow rates up to several tens of liters per minute.94 The colliding lipid streams meet in a chamber where turbulent mixing and cavitation further contribute to the formation of smaller vesicles.95 Membrane extrusion is a related method than can achieve smaller and more uniform vesicles than other homogenization techniques.96 In this process, MLVs are forced through an array of nanoscale pores in a polycarbonate track-etched membrane, resulting in unilamellar vesicles with diameters only slightly larger than the pore dimensions.96–98
Detergent removal.
Detergent removal-based methods begin with lipid micellar structures stabilized in aqueous solution using detergents. As with mechanical disruption, this preparation route avoids the use of solvent in the initial lipid solution. Because detergents exhibit higher aqueous solubility than lipids, removal or dilution of detergent within the surrounding medium leads to a rapid reduction in detergent concentration within the micelles, resulting in destabilization and conversion of the micelles into spherical vesicles.99,100 Detergent removal may be performed using dialysis101,102 or by rapid dilution of the micelles with aqueous buffer.103 Detergent removal can operate at high throughput, but tends to generate larger and more polydisperse particles than other techniques due in part to limited control over size and morphology of the initial micellar structures.
Solvent dilution.
Unlike mechanical disruption and detergent removal, lipid-based nanoparticle formation by solvent dilution is performed using a solution of lipids dispersed in a water-miscible organic solvent such as ethanol. The solvent dilution process is a form of flash nanoprecipitation, wherein molecular solubility is rapidly reduced to induce particle precipitation from the constituent solutes. The first implementation of this technique for lipid-based nanoparticle production involved the injection of ethanol-solvated lipids into aqueous buffer,104 resulting in relatively large (>200 nm) and polydisperse unilamellar vesicles. To improve size control over ethanol injection while also enabling continuous-flow nanoparticle production, a cross-flow injection technique was later developed.105 In this approach, solvated lipids are injected through an orifice into a buffer stream, with orifice dimensions, lipid injection pressure, and buffer flow rate selected to enhance mixing kinetics. Rapid mixing may also be achieved by taking advantage of chaotic flows within a turbulent mixing cell. The earliest turbulent mixers for lipid nanovesicle production employed millimeter-scale T-junction channels operated at high Reynolds numbers in the transitional or turbulent regimes.106,107 A related topology is the vortex mixer, which employs turbulent mixing in a vortical flow field formed within a circular chamber with diameter around 5 mm.108,109 Various vortex mixer designs have been successfully demonstrated for the production of larger liposomes with modal diameters above 150 nm.110,111 A particularly impactful turbulent mixing technology for flash nanoprecipitation is the impingement jet mixer,112,113 which served as a critical technology in the preparation of nucleic acid-encapsulating LNPs for the scalable production of COVID-19 mRNA vaccines.114 Similar to the turbulent T-junction mixers, impingement jet mixers employ two opposing inlets to inject solvated lipids and aqueous buffer into a mixing zone, but unlike the T-junction design the colliding streams meet in a larger chamber with diameter approximately 5× larger than the inlet and outlet channels to enhance the mixing time scale.
Microfluidic-enabled lipid-based nanoparticle synthesis
Microfluidic methods for lipid-based nanoparticle synthesis are based primarily on nanoprecipitation via solvent dilution. In these systems, nanoparticle self-assembly is driven by steep spatial and temporal solubility gradients induced by rapid mixing controlled by convective flows within microchannels with characteristic dimensions that are typically on the order of several tens to hundreds of micrometers. Mixing occurs in these systems using channel designs that have been developed to enhance mixing performance by optimizing diffusive transport, advective transport, or a combination of both mechanisms, as depicted in Fig. 3 and summarized in the following.
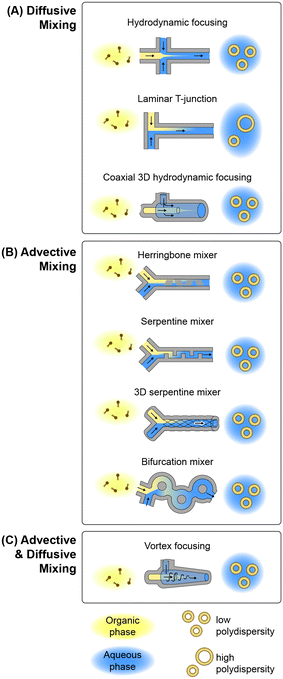 |
| Fig. 3 Microfluidic lipid nanoparticle production technologies. Overview of microfluidic device topologies for lipid nanoparticle synthesis employing hydrodynamic focusing with (A) solely diffusive mixing, (B) mixing via chaotic advection, and (C) combined advective and diffusive mixing. | |
Microfluidic hydrodynamic focusing.
The first microfluidic technology explored for lipid-based nanoparticle synthesis was based on a microfluidic hydrodynamic focusing (MHF) technique.115,116 In this process, a central stream of solvated lipids is sheathed by a pair of outer aqueous buffer streams that serve to focus the solvent stream into a narrow sheet. The reduced diffusion length scales for solvent, water, and lipids within the laminar mixing zone result in a large solubility gradient, reducing the time scale available for the growth of the intermediate lipid fragments and thereby constraining the resulting vesicle size.117 Compared with conventional homogenization techniques, the MHF process can yield unilamellar lipid nanoparticles with low polydispersity in a single pass through the continuous-flow mixing zone. Furthermore, because the diffusion length scale can be readily adjusted by modifying the buffer
:
solvent flow rate ratio (FRR), the MHF technique enables the resulting nanoparticle size to be tuned by adjusting the ratio, with higher FRR values narrowing the solvent stream and yielding smaller particles. While the MHF process was initially demonstrated for liposome production using silicon devices with low aspect ratio channels, later versions of the platform increased the aspect ratio for improved size control.118,119 Modified versions of the MHF design employing secondary focusing channels have also been explored to allow hydrophilic drugs to be injected between the outer sheath flow and inner lipid solution, resulting in drug encapsulation during the self-assembly process with high efficiency.119,120
A limitation associated with planar MHF devices is that the parabolic flow profile within the rectangular channels prevents complete focusing over the channel height, since the solvent flow field is constrained by the no-slip condition on the upper and lower channel surfaces. To overcome this issue, a radially-symmetric device was developed using a silica capillary bundle to achieve coaxial 3D hydrodynamic focusing.121 When operated at high FRR above 1000
:
1, the system was capable of achieving exceptionally low polydispersity. For example, 56 nm liposomes with polydispersity index (PDI) values as low as 0.005 were demonstrated.
Numerous variations on the MHF technology have been developed and applied to nanomedicine development over the past several decades. Examples including the demonstration of folate receptor-targeted liposomes for chemotherapeutic delivery,122 lipid–polymer core–shell nanoparticles for delivery of siRNA drugs,123 and various lipoplexes for nucleic acid delivery and gene transfection.124–127
While the MHF technique provides tunable control over lipid nanoparticle size with low size variance, the focusing process results in dilution of the final product when operating the devices at high FRR. A simpler microfluidic technology that avoids this constraint is based on diffusional mixing within a laminar T-junction. This approach is conceptually similar to conventional T-junction mixers for lipid precipitation but relies on diffusive mixing across the interface of a laminar solvent/buffer co-flow within a microchannel rather than turbulent mixing within a larger channel. While there are a number of examples of lipid-based therapeutics synthesized by nanoprecipitation using laminar T-junction devices,128–133 the long mixing times inherent to diffusion-based solvent dilution result in large and polydisperse particles that make this technique poorly-suited for nanomedicine manufacture where vesicle size and uniformity are important parameters to control.
Microfluidic chaotic advection.
To overcome the slow mixing speeds of laminar T-junction mixers, various microchannel designs capable of manipulating the streamlines within the mixing channel have been explored to induce rapid mixing by chaotic advection. In the chaotic advection process, mixing occurs in low Reynolds number flows by the stretching and folding of merging fluid domains to form striations that promote rapid diffusion across the domain boundaries.134 Channel geometries employed for this purpose include periodic turns, grooves, obstacles, or bifurcating flow paths that disrupt the linear streamlines.135–141 While both advection and diffusion contribute to mixing in these systems, mixing speed is largely dictated by enhancement of the advective mixing process. Here we refer to this class of platforms as microfluidic chaotic advection (MCA) mixers. As with MHF devices, MCA mixers serve to dilute the solvent concentration below the lipid solubility limit at a rate faster than the characteristic lipid fragment growth rate. The earliest application of chaotic advection to lipid-based nanoparticle production employed a staggered herringbone mixer, consisting of periodic angled protrusions in the base of the mixing channel. Herringbone mixers were introduced as a general tool for rapid microfluidic mixing in 2002,142 and first applied to liposome and solid lipid nanoparticle synthesis in 2012.143 Small LNPs with minimum diameters approaching 20 nm were achieved at a FRR of 3
:
1, demonstrating the ability of the herringbone mixer to provide excellent nanoparticle size control without the high levels of dilution associated with the MHF technique.144 In the case of liposome production, size uniformity in these devices can be particularly sensitive to FRR, with low PDI around 0.2 achieved for larger vesicles above 150 nm when operating at FRR = 1 but increasing to 0.5 at FRR = 5 for the generation of smaller 60 nm particles.145 Similar behavior was observed when encapsulating a lipophilic drug (propofol) in the liposome membrane during synthesis.146 In related work, the loading of both hydrophilic and lipophilic drugs in lipid vesicles was evaluated by adding each agent to the buffer or lipid stream, respectively, with peak encapsulation efficiencies of 25% and 43% achieved.147 Other studies performed using herringbone mixers have evaluated the impact of cholesterol and PEG-lipid concentrations on liposome size,148 together with the impact of buffer concentration149 and solvent selection150 on liposome properties.
Other MCA channel topologies have also been leveraged for lipid-based nanoparticle production. One such approach employs a periodic serpentine mixing channel, wherein a curved channel is used to induce secondary chaotic Dean vortices within the flow that fold across one other with each change in curvature.151 This concept has been applied to the synthesis of both liposomes and LNPs using various serpentine channel designs.152–154 A related technology employs a trio of intertwined channels fabricated by soft lithography using manually-twisted threads to define the channel mold. The resulting 3D serpentine mixer was found to enable liposome synthesis within a narrow operational range, with a minimum PDI around 0.1 and relatively large 160 nm vesicles demonstrated.155 Another promising MCA topology employs a periodically-bifurcating channel structure consisting of paired channels with flow paths that intersect at multiple points along their length. Bifurcating micromixers were first developed in 2010,156 with several variants later studied, such as a split-and-recombine mixer157 that is similar to a toroidal design recently employed for lipid-based nanoparticle synthesis.158 This latter work reported the formation of anionic, neutral, and cationic liposomes using a toroidal mixer, together with the encapsulation of proteins and nucleic acids, with modal diameters ranging from approximately 40–60 nm and PDI values between 0.1–0.2. Encapsulation efficiency approaching 100% was achieved during lipoplex formation between cationic lipids and polyadenylic acid, while up to 35% efficiency was demonstrated for ovalbumin encapsulation in neutral liposomes.
Combined flow focusing and advective mixing.
A vortex focusing technique recently introduced by our group employs a combination of MHF and MCA in a single chamber for enhanced mixing during nanoparticle synthesis.159 Unlike conventional vortex mixers, which use planar mixing chambers for nanoparticle production in a turbulent vortical flow,108,109 vortex focusing involves injecting solvated lipid through an axial inlet into a tapered conical mixing chamber, while simultaneously injecting aqueous buffer through a tangential inlet port to generate a laminar spiral flow path of buffer sheathing the central lipid stream. The outer vortical flow focuses the lipid solution to reduce the radial diffusive length scale in a manner similar to the MHF technique, while also transferring rotational momentum to the inner flow, resulting in striation of the interface for enhanced mixing by chaotic advection. As with MHF, the flow rate ratio may be adjusted to control the degree of focusing for the lipid solution. Using this process, PEGylated liposomes as small as 27 nm with PDI below 0.05 were achieved using a neutral lipid mixture.
Commercial microfluidic technologies.
It is notable that as the field of microfluidic-enabled nanomedicine development has matured, a variety of systems based on microfluidic technologies for lipid-based nanoparticle synthesis have been introduced commercially in recent years. For example, herringbone and bifurcating channel MCA mixers are commercially available from Precision Nanosystems Inc. (Vancouver, Canada) as part of their NanoAssemblr™ platform, while serpentine mixers for nanoparticle preparation termed iLiNP chips are marketed by Shin-Etsu Chemical Co. (Tokyo, Japan). Microfluidic flow focusing chips and systems are available from multiple vendors, such as Dolomite Microfluidics (Royston, UK) which markets the Automated Nanoparticle System platform. While less commonly employed for lipid-based nanoparticle production due to their limited size control, microfluidic T-junction mixers are also available as the PureNano Continuous Crystallizer from the IDEX subsidiary Microfluidics International Corp. (Newton, MA, USA).
Nanoparticle size control
Controlling nanoparticle size and size variance is critical for optimizing the biodistribution, therapeutic effect, and safety of lipid nanomedicines.160–163 Nanoparticle size can directly impact bioavailability and targeting efficiency as well as cell uptake and cell localization.161,164–166 Smaller particles below approximately 150 nm exhibit significantly longer circulation times due to reduced uptake by cells of the mononuclear phagocyte system (MPS), improving their ability to reach and enter target tissues.162,167 Nanoparticle size is also a key parameter impacting overall biodistribution and toxicity, with larger particles commonly tending to accumulate in healthy tissues including the liver and spleen.161,168,169 The impact of size on the efficiency of drug delivery can be seen across diverse tissues and cell types. For delivery to solid tumors, the enhanced permeability and retention (EPR) effect170 allows smaller nanoparticles to accumulate in the target tissues due to higher vascular permeability within the tumors.171,172 Nanoparticles in the range of 20–100 nm have been reported to efficiently enter bone marrow,163 and lymph node entry and CD8+ dendritic cell uptake was found to be significantly higher for small 30 nm LNPs compared with large 100 nm particles.167 Liposomes below approximately 100 nm have also been shown to pass the blood–brain barrier,173–175 and smaller vesicles in the 30–40 nm range can significantly enhance transport across the dermal barrier compared with larger particles above 100 nm.176 Multiple studies have shown that smaller nanoparticles below approximately 60 nm can yield increased cell uptake.1,177,178 Lipid-based nanoparticles generally require active transport across the cell membrane via endocytosis, with smaller particles reported to be taken up by phagocytosis and larger particles by pinocytosis.1 In our own work, particle size was found to strongly influence cellular uptake mechanism when delivering microfluidic-enabled liposomes to human colorectal adenocarcinoma cells. Transport of particles with modal diameters above 100 nm was weakly sensitive to the clathrin-dependent pathway, while smaller liposomes were affected by a combination of clathrin-mediated, caveolin-mediated, pinocytosis, and dynamin-dependent pathways, resulting in significantly higher cellular accumulation for smaller liposomes in the 40–70 nm range compared with larger vesicles.165
Tunable size control.
Compared with conventional methods of lipid-based nanoparticle synthesis, microfluidics offers benefits for size control by precisely defining laminar convective flow profiles to generate large and well-controlled solubility gradients for rapid mixing through diffusive or advective transport. Additionally, while most batch manufacturing techniques are designed to produce a specific particle size based on the physical properties of the system, microfluidic synthesis supports the formation of nanoparticles of tunable size by dynamically modulating buffer and lipid flow rates within the devices. In addition to the specific microfluidic process used for nanoparticle formation, and the parameters selected for operation of the system such as total flow rate (TFR), buffer
:
solvent flow rate ratio (FRR), and processing temperature, many other factors can influence nanoparticle size including solvent selection, buffer strength, lipid species and ratios, degree of PEGylation, encapsulant type and concentration, and post-processing conditions. As just one example, a high concentration of cholesterol is commonly included within the initial lipid mixture used for liposome formation to enhance stability of the resulting vesicles by decreasing membrane fluidity and increasing membrane rigidity.179 However, higher bending stiffness for intermediate lipid structures during the solvent dilution process can lead to the formation of significantly larger vesicles due to increased bending energy for cholesterol-laden membranes.
Given these complexities, comparing the performance of published synthesis techniques is challenging. It is instead instructive to consider the factors that impact the mixing time scales. For both MHF and MCA techniques, vesicles form by the self-assembly of amphiphilic lipid molecules into metastable bilayer structures that become energetically favored as solvent concentration is reduced. Below some critical solvent concentration, determined by lipid characteristics including the free energy of the exposed bilayer edge and bilayer bending stiffness, these fragments close upon themselves to minimize the total free energy of the system.180,181 Using molecular dynamics simulations, membrane fragment formation was found to initiate on a time scale below 0.1 ms,182 with fragment growth continuing in a rate limited process through recruitment of free lipids and fusion with other bilayer fragments.183–185 Experimental evidence of this bilayer fragment growth process during MHF has also been reported using cryo-SEM imaging following flash freezing of the solution during flow focusing, allowing metastable lipid fragments to be directly observed.117 The time over which the solvent concentration remains in a range where lipid fragment growth can occur is thus expected to dictate the final nanoparticle size. The solvent concentration at which metastable lipid structures begin to form has been studied experimentally using neutral DMPC lipids in ethanol.186 In this work, particle anisotropy was evaluated in bulk solution using light scattering, revealing the formation of disk-like micelles at an ethanol mole fraction of 0.5. More recently, a microfluidic herringbone mixer was used to study vesicle formation for neutral POPC lipids using confocal microscopy to map the lipid concentration across the channel cross-section.187 Lipid fragment formation was found to begin at an ethanol mole fraction around 0.8, with vesicle closure occurring before the system reached a mole fraction of 0.6. In this study, vesicle size was found to scale with the residence time within the given concentration range, with a minimum vesicle size of 30 nm associated with a 10 ms residence time.
Because the residence time within the critical solubility range scales inversely with total flow rate, smaller particles are expected to be generated when operating a device at higher throughput. This behavior has been observed in conventional solvent dilution methods such as ethanol injection,188 and the majority of studies employing microfluidic devices based on both diffusive and advective mixing also report an inverse relationship between TFR and nanoparticle size. An inverse relationship also exists between FRR and particle size for both MHF and MCA techniques. In the case of hydrodynamic focusing, increased FRR reduces the width of the focused solvent stream to generate a steeper spatial solubility gradient across which diffusive mixing occur, while in the case of MCA it yields a steeper temporal solubility gradient during the initial stage of the advective mixing process. Studies exploring sufficiently high flow rates and flow rate ratios also reveal that nanoparticle size tends reach a limiting value beyond further size reduction is not possible.143,159,189,190 Under ideal mixing conditions, this particle size limit reflects the smallest thermodynamically-stable structure that can be achieved for the given lipid composition.143
Size variance.
In concert with modal diameter, size variance also plays an important role in nanomedicine performance. The polydispersity index, defined as the particle size variance normalized by the square of the mean diameter,191 is a common metric of nanoparticle size uniformity, with higher PDI values reflecting increasing polydispersity. The FDA identifies size distribution as a critical quality attribute (CQA) for liposomal drugs, with guidelines emphasizing the need for size variance data in the submission of new drug applications.192,193 The importance of maintaining low polydispersity can be seen by considering a hypothetical case where nanoparticles smaller than 100 nm deliver their payloads with ideal efficiency, while larger particles are cleared from circulation. Given two nanoparticle populations with identical modal diameters of 80 nm but different size variance with PDI values of either 0.3 or 0.05, the amount of drug reaching the target can be found by assuming a log-normal particle size distribution194,195 and integrating the product of the probability density function by the size-dependent particle volume., with results presented in Fig. 4.159 For PDI = 0.3, less than 5% of the total nanoparticle volume is associated with particles smaller than 100 nm, while over 50% of the drug is within particles below this cutoff for the more uniform population with PDI = 0.05. While this analysis is based on idealized assumptions, it reveals the significant impact that polydispersity can have on nanomedicine delivery efficiency and toxicity.
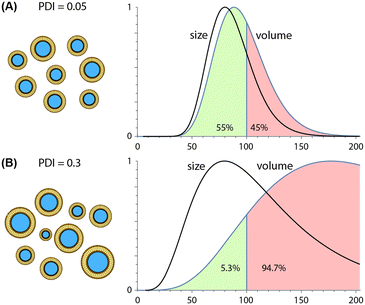 |
| Fig. 4 Impact of nanoparticle polydispersity on drug delivery. Comparison of nanoparticle populations with identical modal diameters of 80 nm but different size distributions. Each distribution is defined by a probability density function (PDF) reflecting a PDI value of either (A) 0.05 or (B) 0.3. For each case, both size (diameter) and volume distributions (PDF scaled by the size-dependent particle volume) are presented. Plots are normalized by modal peak values. | |
Due to their ability to minimize variations in lipid solubility gradients during mixing, microfluidic techniques are capable of producing more uniform nanoparticle populations than conventional synthesis methods, with PDI values well below 0.1 routinely reported for different classes of lipid-based nanoparticles. Unlike average particle size, which scales inversely with TFR and FRR values during both hydrodynamic focusing and chaotic advection mixing, trends for polydispersity are technology-dependent. PDI values tend to increase with higher FRR values during MCA145,146 but remain nearly constant during MHF,159,189,190 particularly when operating at lower flow rate ratios. In contrast, increasing TFR tends to yield nearly constant or reduced size variance for both MHF159,189 and MCA144,146 techniques. Unlike the consistent inverse relationship observed between TFR and size, however, this trend can vary significantly with both the specific device design and nanoparticle composition, e.g. liposomes vs. LNPs.
Microfluidic post-processing of lipid nanomedicines
Beyond nanoparticle formation, the production of lipid-based nanomedicines involves multiple process steps including surface functionalization, drug encapsulation, and purification. Further processing steps such as nanoparticle concentration or dilution may also be required. Specific post-processing requirements can depend on the nanoparticle production method employed. For example, MHF tends to yield dilute nanoparticles due to the use of high flow rate ratios during synthesis, requiring additional concentration of the final product, while extended buffer exchange may needed for nanomedicines prepared using MCA mixers due to the high concentration of solvent in the output stream. Microfluidic technologies create new opportunities for the integration of these and other production steps for the continuous manufacturing of lipid nanomedicines.
In conventional batch processing, purification is typically performed by tangential flow filtration to remove solvents and non-encapsulated drug from the solution while simultaneously supporting buffer exchange. Additional purification is often performed by direct membrane filtration to remove larger particles above several hundred nanometers, and a final filtration step through a 200 nm pore filter serves to yield a sterile product prior to aseptic vial filling. These discrete steps can significantly increase the complexity and reliability of batch manufacturing. For example, in the case of Doxil, the first FDA-approved nanomedicine comprising the doxorubicin encapsulated in PEGylated liposomes with a modal diameter of approximately 100 nm,32 the manufacturing process requires 17 different process vessels to generate a single drug batch over a 5 day period. Poor manufacturing reliability was responsible for Doxil being forced off the market for an extended period between 2011 and 2013, leading to major shortages of the drug.196,197 Indeed, nearly half of all drug shortages are due to issues of manufacturing quality and reliability,198 and lipid nanoparticle drugs are particularly prone to reliability issues due to the multi-step preparation processes inherent to batch production.199
Microfluidics offers a path to overcoming this challenge by integrating multiple functionalities into a single continuous flow process, thereby reducing the number of discrete manufacturing steps required for nanomedicine production. Here we review progress towards the microfluidic integration of nanoparticle synthesis with nanomedicine purification, concentration, drug loading, and functionalization.
Surface functionalization.
Nanomedicine surface modifications are often desirable to control in vivo characteristics including stability, bioavailability, targeting, and release.200 While lipid nanoparticle surface functionalization is commonly performed during vesicle synthesis through the addition of modified lipids or membrane-intercalating molecules within the initial lipid mixture, post-synthesis modifications can expand the range of functional agents that can be attached to the surface, such as ligands that are not soluble in the organic phase. Several examples of post-synthesis vesicle functionalization using microfluidics have been reported. For example, the insertion of peptide ligands into PEGylated liposomes was demonstrated in a continuous-flow process using a microfluidic herringbone mixer.201 Lipids containing a serine–glycine repeat spacer to enhance ligand presentation were conjugated with targeting peptides and dispersed into aqueous solution to form micelles. The micellar suspension was then co-injected into the staggered herringbone chip with pre-formed PEGylated liposomes, where rapid mixing destabilized the micelles, allowing them to integrate into the liposome membranes. The technique was found to work with similar efficiency as a previously-demonstrated bulk process, but with insertion times nearly 2 orders of magnitude faster within the microfluidic device. In another example, liposomes formed by conventional membrane extrusion were prepared with N-glutaryl-DPPE lipids in the mixture to present carboxylic group on the vesicle surface.202 Amine-functionalized magnetic nanoparticles (MNPs) were first immobilized within a microchamber by applying a static magnetic field to the chip. The modified liposomes were then perfused into the chamber and activated with a linker solution to covalently bind the vesicles to the MNPs. Free binding sites were deactivated by adding ethanolamine before releasing the magnetic field, allowing the final MNP-functionalized liposomes to be removed from the system.
Another study successfully demonstrated the full integration of lipid nanoparticle formation and post-synthesis functionalization into a single continuous-flow process. In this work, a multi-stage system combining MCA and MHF was employed to synthesize transferrin-conjugated lipoplexes for targeted delivery of siRNA to tumor cells.203 A herringbone device was used in the first stage to mix cationic lipids in ethanol with siRNA in buffer via MCA to form lipoplexes. The resulting nanoparticles were then delivered to a second MHF stage where the lipoplex solution was sheathed by an aqueous solution of transferrin conjugated to cholesterol through a PEG linker (Tf-PEG-Chol). During flow focusing, the cholesterol subunits integrated into the liposome membranes, resulting in functionalization of the outer surface of the vesicles with transferrin.
Drug loading.
As with surface functionalization, drug loading within microfluidic-enabled lipid nanomedicines typically occurs during the nanoparticle formation process through the encapsulation of hydrophilic and lipophilic compounds added to the aqueous and organic phases, respectively. Despite the simplicity of this approach, low encapsulation efficiencies are often observed for some classes of drug compounds, while other agents with low solubility cannot be efficiently captured within the particles. Post-synthesis loading affords additional flexibility in the nanomedicine production process, providing a path towards enhanced encapsulation efficiency, increasing the total amount of drug loaded into lipid-based nanoparticles, and expanding the range of therapeutic agents that may be integrated within the nanoparticles. Several microfluidic techniques have been reported for drug loading after nanoparticle synthesis. For example, a fully integrated device was developed to complex nucleic acids with preformed cationic liposomes to yield lipoplexes.204 A two stage MHF chip employed a first stage to generate liposomes via flow focusing, after which the stabilized liposome stream was split to form the sheath flows used to focus a solution of pDNA in the second stage, followed by an extended mixing zone to promote effective condensation of nucleic acids on the vesicles. A benefit of this process is that the focusing step serves to reduce reagent waste that would otherwise occur during flow focusing in a single-stage mixer. A related technique employing active drug loading employed a multistage microfluidic device combining MHF for liposome formation, counterflow microdialysis for buffer exchange, and drug mixing to enable remote loading of amphipathic drugs into the vesicles.205 Amphiphilic compounds such as anthracyclines are difficult to encapsulate by passive methods since the molecules can diffuse out through the lipid membrane. In the remote loading process, a transmembrane ion gradient is used to trap amphiphilic drug molecules within the liposomes by effecting a sharp drop in solubility after drug diffuses into the core, for example by forming a salt with a suitable counterion within the vesicle.206 In the microfluidic implementation of this process, liposomes were formed by MHF using ammonium sulfate buffer for the sheath flow. The resulting vesicles were directed through a serpentine counterflow microdialysis cell consisting of a pair of channels separated by a membrane with 4 nm pore size, allowing ion exchange between the compartments and shifting the local pH surrounding the vesicles. In the final stage, a drug simulant was injected through a pair of side channels to mix with the liposomes in an incubation channel, resulting in salt crystallization within the liposomes with encapsulation efficiencies above 50%.
Purification.
A limitation of microfluidic solvent dilution for nanomedicine production is the presence of solvent in the final product. Ethanol is commonly used for lipid nanoparticle preparation by solvent dilution due to its low toxicity, with concentrations up to 0.5% by volume are considered acceptable under both U.S.207 and E.U.208 guidelines. However, significantly higher amounts can be present in microfluidic formulations, particularly for the case of MCA mixers where final solvent concentrations above 20% are common. One microfluidic approach explored for reducing solvent concentration involves the addition of a buffer dilution stage following lipid nanoparticle synthesis.152 While solvent concentration can be reduced in this process, commensurate dilution of the nanoparticles also occurs. To avoid this limitation, microfluidic counterflow microdialysis technology developed for on-chip remote drug loading can perform efficient buffer exchange without dilution,205 but requires careful design to ensure that sufficient residence time within the dialysis cell can be achieved at higher system flow rates. Alternately, a hybrid approach to continuous-flow purification has been demonstrated by eluting liposomes from a herringbone mixer into a collection vial, and using an off-chip pumping loop to add diafiltration buffer to the vial and deliver the diluted solution through a tangential flow filtration (TFF) cell to support continuous-flow purification.209
Other approaches to microfluidic purification have been reported by selectively immobilizing lipid nanoparticles within a chip through surface interactions or external fields, followed by elution of the purified particles after perfusing rinse buffer through the system. In one such approach, an array of silicon micropillars patterned with nanowires were successfully used to trap liposomes smaller than 120 nm, followed by 24 h immersion in PBS buffer to degrade the nanowires and release the vesicles,210 although the single-use nature of this technique makes it poorly suited to applications in nanomedicine production. A related approach employs a microchannel-integrated nanofiber poly(vinyl alcohol) mat modified with cationic agents to capture anionic liposomes from solution, with selective release achieved through a pH shift following buffer exchange.211 Repeated buffer exchange cycles can be implemented using this approach. Selective immobilization of liposomes encapsulating magnetic nanoparticles has also been demonstrated by application of an external magnetic field during sample perfusion, trapping the particles in an on-chip chamber for purification by buffer exchange.212
A range of microfluidic technologies have been developed for the separation and purification of other classes of nanoparticles beyond synthetic lipid nanoparticles.213 In particular, extracellular vesicles (EVs) including exosomes and microvesicles have direct relevance to lipid-based nanomedicine manufacture due to their physiochemical similarities to liposomes and direct utility as drug delivery vehicles.214 Microfluidic platforms for EV purification have been demonstrated based on multiple physical separation mechanisms employing techniques including centrifugal microfluidics,215 mechanical on-chip filtration,216,217 acoustic focusing,218,219 viscoelastic microfluidics,220 and deterministic lateral displacement.221 However, the primary goal in EV purification is the removal of larger particulates such as cell debris rather than the removal of small molecules and ions, limiting their utility for lipid nanomedicine processing.
Concentration.
Bulk-scale manufacturing processes commonly concentrate dilute nanomedicines using closed-loop TFF as a final processing step, allowing the continuous removal of buffer until the desired concentration factor is achieved. While a similar approach has been employed for processing nanomedicines generated from microfluidic devices,209 this technique is not readily scalable to larger numbers of synthesis units, and requires additional instrumentation and interconnections that can negatively impact process purity and reliability. An alternative investigated for on-chip concentration of lipid nanoparticles is the use of electrokinetic transport. An early example of this approach employed direct electrophoretic mobilization of liposomes.222 By applying an electric field across a thin polyacrylamide gel membrane photolithographically patterned at a channel junction, liposomes with negative zeta potential were electrophoretically transported from an inlet reservoir in the absence of hydrodynamic flow, and concentrated at the membrane surface using a 150 V bias. An order-of-magnitude increase in concentration was achieved, with the concentrated vesicles periodically collected in an outlet reservoir by shifting the bias electrode (Fig. 5A). A related approach is based on the use of the ion concentration polarization effect, in which a conductive membrane is used to form an ion depletion region that generates opposing electrophoretic and electroosmotic forces that serve to focus target particles at the membrane boundary. In a recent demonstration of this technique, a nanoporous Nafion membrane was integrated into the base on a microchannel to generate a local ion depletion zone, with liposome concentration factors up to 160 achieved with an applied voltage of 100 V in 10 min (Fig. 5B).223 Ion concentration polarization has similarly been employed for EV concentration using device designs with potential for application to synthetic lipid nanoparticle production. For example, a microfluidic device containing a cation-selective polymer membrane deposited within a chamber positioned between a pair of reservoirs was demonstrated for EV capture, with concentration increased by two orders of magnitude within 30 min using a 45 V bias (Fig. 5C).224 While this device was not designed to operate as part of a continuous-flow system, an alternate microfluidic system supporting the continuous delivery of nanovesicles by hydrodynamic flow in a main channel has also been reported, with electrokinetic particle capture occurring within a connected side channel containing a cation exchange membrane to form the desired ion depletion zone (Fig. 5D).225 When using an 100 V cm−1 electric field across the membrane, capture and concentration of up to 80% of all perfused exosomes was reported. While promising, a limitation of existing electrophoretic methods towards on-line nanomedicine concentration is the relatively throughput offered by these designs, with typical flow rates below 1 mL h−1. Additional advances in this area will require new designs and device implementations capable of supporting significantly higher sample flow rates matched to the selected nanoparticle synthesis process.
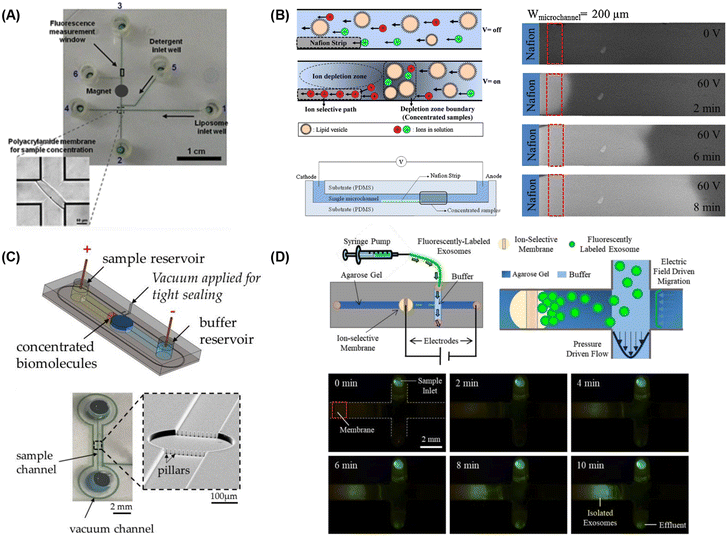 |
| Fig. 5 Microfluidic lipid vesicle concentration techniques. (A) Electrophoretic concentration of liposomes against a photolithographically-patterned polyacrylamide gel membrane (reproduced from ref. 222 with permission from Springer, copyright 2011). Liposome concentration via microfluidic ion concentration polarization utilizing (B) a Nafion membrane patterned on a microchannel floor (reproduced from ref. 223 with permission from Elsevier, copyright 2016), (C) conductive nanoporous polymer deposited in a sealed chamber (reproduced from ref. 224 with permission from MDPI, copyright 2018), and (D) capture of nanoparticles at the surface of an ion-selective membrane during continuous sample perfusion (reproduced from ref. 225 with permission from Wiley, copyright 2018). | |
Nanoparticle characterization.
Process monitoring for nanomedicine manufacture is critical for maintaining consistent drug product in the production setting. As a step towards integrating sensors capable of in-line processing monitoring with microfluidic nanomedicine synthesis, a system containing interdigitated capacitive electrodes within a microfluidic flow loop was developed to enable assessment of lipid nanoparticle composition and stability using impedance spectroscopy.226 Using this system, unique impedance signatures could be generated based on the intrinsic electrical properties of the vesicles including lipid composition, surface functionalization, and encapsulant. After training a principal component analysis model with collected spectra, this platform was able to accurately differentiate between 6 different liposome formulations. As an alternate sensing modality, organic electrochemical transistors capable of operating in a liquid environment were similarly integrated into a simple microfluidic flow cell for monitoring of lipid-based nanoparticles.227 Fabricated devices were able to successfully quantify nanoparticle concentration and differentiate liposomes functionalized with chitosan in real-time. Further advances in lipid vesicle sensing techniques, together with future work towards the integration of in-line sensing with nanoparticle synthesis, offers significant potential for the development of fully-integrated microfluidic reagent-to-nanomedicine processing platforms with real-time monitoring and feedback for manufacturing process control.
Scaling manufacturing throughput
The small channel dimensions inherent to microfluidic devices designed for nanoparticle synthesis, together with the need for maintaining laminar flow during device operation, constrain the maximum flow rates that can be employed in these systems. While early microfluidic-enabled platforms offered sufficient throughput to prepare nanomedicine volumes suitable for benchtop and limited preclinical studies, the transition to large-scale nanomedicine manufacturing demands significantly greater production throughput. Here we discuss the evolution of microfluidic mixer designs targeting this challenge.
High-throughput micromixer designs.
A wide range of device topologies have been optimized for high-throughput lipid nanoparticle production. For devices based on hydrodynamic focusing, it is desirable to minimize microchannel width within the flow focusing region to enhance control over diffusive mixing length scales. Typical MHF devices employ channel widths below 100 μm and channel aspect ratios on the order of 5
:
1.228 To maintain laminar flow conditions during focusing, peak lipid flow rates within these devices are typically limited to approximately 0.1 mL min−1. Because volumetric flow rate scales with the characteristic channel width for a constant Reynolds number, a simple strategy to enhance nanoparticle production throughput in MHF is to increase the channel dimensions. Various MHF devices with larger channel geometries have been developed for high-throughput lipid nanoparticle production. For example, a flow-focusing design with 400 μm wide and deep channels was successfully operated with lipid flow rates up to 10 mL min−1 for liposome production (Fig. 6A). However, the larger diffusive length scales associated with this design limited minimum vesicle size to approximately 100 nm, with high polydispersity observed (PDI > 0.4).229 Other efforts to increase throughput by utilizing larger focusing channels have similarly reported limited control over nanoparticle size and high size variance,230,231 as expected due to the higher diffusive length scale associated with the wider focusing channels used in these efforts.
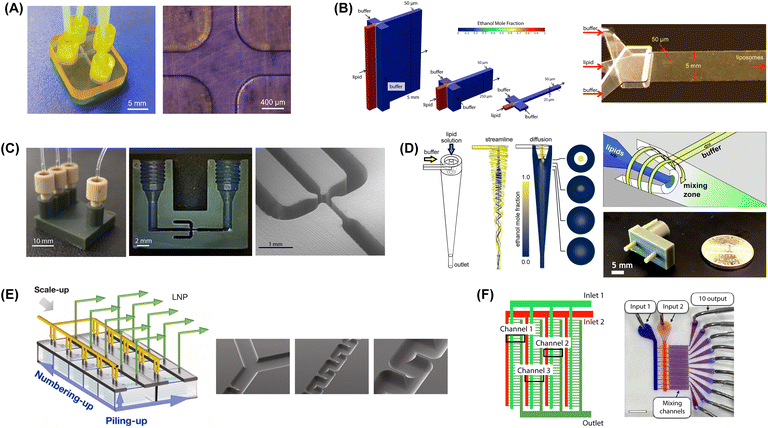 |
| Fig. 6 Microfluidic mixers for high-throughput lipid-based nanoparticle production. (A) 3D printed MHF design with 400 μm channels to support high lipid flow rate (reproduced from ref. 229 with permission from Frontiers Media S.A., copyright 2021). (B) Vertical flow focusing device with 100 : 1 channel aspect ratio and 50 μm critical dimensions (reproduced from ref. 189 with permission from Wiley, copyright 2015). (C) 3D printed VFF device with 40 : 1 channel aspect ratio and 200 μm critical dimensions (reproduced from ref. 190 with permission from Wiley, copyright 2019). (D) Microfluidic vortex focusing process combining advective mixing in a hydrodynamically-focused vortical fluid stream (reproduced from ref. 159 with permission from Nature, copyright 2022). (E) MCA array consisting of 8 parallel chips, each containing 5 stacked serpentine micromixers (reproduced from ref. 231 with permission from Elsevier, copyright 2023). (F) MCA array with 128 parallel staggered herringbone micromixers in a single integrated chip (reproduced from ref. 232 with permission from American Chemical Society, copyright 2021). | |
To avoid the loss of size control during scale-up, an effective strategy is to increase the channel height while minimizing dimensions for the channel width. To this end, MHF devices possessing a 5 mm tall and 50 μm wide (100
:
1 aspect ratio) focusing channel were developed to implement a method termed vertical flow focusing (VFF) since the focusing axis was oriented normal to the plane of the chip (Fig. 6B).189 The VFF design was found to increase liposome production throughput by 2 orders of magnitude over conventional planar MHF designs while enabling liposomes with modal diameters below 80 nm and low polydispersity. Furthermore, maximum Reynolds number was limited to only Re = 28, and the upper flow rate was defined by failure of the fluidic interfaces at higher inlet pressures rather than fundamental physics of the mixing process, suggesting that higher throughput is feasible with the VFF design. To address fabrication and interfacing challenges associated with the 3-layer VFF chips, a related device was later implemented using 3D printing to achieve a focusing zone with 200 μm critical dimensions and a channel aspect ratio of 40 (Fig. 6C).190 This device was capable of generating liposomes with minimum modal diameters of 75 nm and low size variance while operating at lipid flow rates up to 2 mL min−1.
Unlike devices based on hydrodynamic focusing that rely on high flow rate ratios to reduce diffusive mixing length scales, chaotic advection mixers are designed to operate at typical FRR values of 5
:
1 or below.145 As a result, they can process larger volumes of lipid than MHF under equivalent channel dimensions and total flow rates, enabling higher throughput from a single device. Early MCA devices employing staggered herringbone mixers were capable of operation with lipid flow rates in the range of 1 mL min−1,145 while later work investigated even higher rates. For example, a lipid flow rate of 3 mL min−1 was used to produce liposomes below 100 nm with moderate polydispersity,147 and lipid flow rates over 10 mL min−1 were demonstrated for the production of small LNPs with PDI values below 0.2.74 Novel MCA mixer topologies designed to support high throughput operation have also been developed, such as a twisted 3D microchannel mixer design supporting lipid flow rates up to 10 mL min−1, although with relatively large and polydisperse liposomes generated from this device.155 Significantly, a bifurcating mixer design with a toroidal geometry introduced commercially by Precision NanoSystems was reported to support liposome synthesis at a lipid flow rate of 67 mL min−1 when operating at FRR = 3, with PDI below 0.2 and modal diameters ranging from 50–90 nm depending on lipid composition.74,158 While details of the toroidal device design and operation have not been disclosed in the literature, the increased throughput achieved using this platform represents a significant advance.
Beyond these various implementations of hydrodynamic focusing and chaotic advection mixing, other emerging rapid mixing techniques also hold promise for high-throughput synthesis. Microfluidic vortex focusing is one such technology that has been investigated for scalable production of lipid-based nanoparticles. A hybrid mixing technology combining both advective and diffusive mixing in a single process, microfluidic vortex focusing was used by our group for liposome synthesis at lipid flow rates up to 8 mL min−1 (Fig. 6D).159 Significantly, the process was found to provide excellent control over the nanoparticle size, with modal diameters below 70 nm for DMPC-based liposomes with PDI values as small as 0.05. When adding PEG-conjugated lipids to form stealth liposomes, vesicles below 30 nm were also achieved. While smaller vesicles with higher size variance are typically observed for PEGylated liposomes, PDI values between 0.1–0.15 were maintained over a wide range of FFR and TFR values when using this technique.
Parallelization.
Rather than optimizing individual mixer designs to increase nanoparticle synthesis rates, an alternate strategy is through the operation of multiple microfluidic devices in parallel. Parallelization was essential for scaling manufacturing throughput of the Pfizer/BioNTech COVID-19 vaccine, with 100 million doses per month achieved by employing up to 100 independent impingement jet mixers operated in parallel on a single manufacturing line.114 However, unlike conventional nanoparticle production methods including impingement jet mixers, microfluidics offers the potential for integrating multiple mixing structures, fluid distribution networks, and collection reservoirs within a single integrated cartridge, offering benefits to scale-out for microfluidic-based mixers. Several recent demonstrations of nanoparticle production using parallel MCA mixers highlight the benefits of this approach. In one example, a glass microfluidic chip containing 5 stacked serpentine MCA mixing channels with shared buffer and lipid inlets was fabricated, and 8 chips were operated in tandem for a total of 40 mixing units (Fig. 6E). Using this system, mRNA-loaded LNPs were successfully synthesized at a total lipid flow rate around 3 mL min−1, while uniform POPC lipid-based nanoparticles in the 30 nm size range were achieved with a total lipid flow rate of 7 mL min−1.231 Similarly, individual microfluidic chips containing up to 128 parallel staggered herringbone mixers was designed to operate from a single pair of buffer and lipid inlets, with the fluid distribution system designed to minimize flow variations across the mixer array (Fig. 6f). In this example, LNPs containing both siRNA or mRNA were produced with FRR around 3 and TFR of 1.26 mL min−1 within each channel, for a total lipid flow rate of approximately 50 mL min−1.232 Further scaling of this platform was recently reported using a 256-element silicon/glass mixer array enabling LNP synthesis at exceptionally high total flow rates up to 283 mL min−1.233
Scale invariance.
An important consideration for process scale-up is the sensitivity of nanoparticle properties to changes in production throughput. Because lipid-based nanomedicines can be particularly sensitive to changes in manufacturing scale, FDA guidance emphasizes full drug recharacterization when changing methods at each production scale.193 The ideal process for lipid nanomedicine synthesis would thus be scale-invariant, allowing the same manufacturing method to be used for each step in the drug development, evaluation, and manufacturing process to minimize or eliminate the need for recharacterization as throughput requirements expand. An advantage of parallelization for enhancing throughput is that the geometry of individual mixing units may be optimized for the desired level of control over nanoparticle size, with additional units added as needed to meet target production rates without impacting the underlying physics of the nanoparticle self-assembly process. However, because maintaining uniform performance across parallel mixing elements becomes increasingly challenging as array density increases, there remains a need for technologies capable of providing scale-invariant nanoparticle production using a single mixing element. While both size and polydispersity tend to be highly sensitive to changes in total flow rate for the majority of MCA or MHF device designs, several platforms have demonstrated varying degrees of scale invariance. For example, the VFF technique has been shown to generate liposomes with size and PDI that are insensitive to TFR when operating above a lipid flow rate of approximately 0.1 mL min−1. Toroidal MCA mixers have similarly demonstrated low sensitivity to TFR while operating over a wide lipid flow rate dynamic range between 4–67 mL min−1.
Impact of additive manufacturing
The field of microfluidics emerged from the broader discipline of microsystems technology, which established itself by leveraging photolithography and related fabrication techniques from the semiconductor industry. As a result, conventional microfluidic systems, including established microfluidic platforms for lipid-based nanoparticle synthesis, are typically planar devices with geometries that are constrained by the available microfabrication techniques. In contrast, additive manufacturing using 3D printing vastly widens the potential design space, offering new opportunities for creating microfluidic devices with geometries that are optimized for specific functionalities rather than compatibility with established microfabrication processes. The use of 3D printing for microfluidic applications was first explored for the fabrication of micromixers with geometries that could not be readily achieved by conventional planar microfabrication.234 Stereolithography (SLA) based 3D printing techniques are most commonly used for microfluidic device development due to their ability to realize a wider range of microchannel geometries with higher resolution and better surface finish than alternate 3D printing methods such as filament-based fused deposition modeling (FDM). In SLA-based printers, a photopolymer is selectively polymerized by a laser or LED in a layer-by-layer process. The ability SLA-based printers to achieve nearly arbitrary 3D structures with surface finish than can exceed that of conventional microfabrication techniques makes the technology very well-suited for the fabrication of microfluidic device requiring complex 3D geometries.235,236 Recent generations of consumer-grade SLA printers are capable of reproducing channel features with critical dimensions on the order of several hundred micrometers, and significantly higher resolutions with channel widths as small as 20 μm have been demonstrated using optimized resins and custom projectors.237,238 Furthermore, lithography-based direct laser writing (DLW) systems that employ two-photon polymerization are capable of features down to several hundred nanometers.239,240 While DLW is currently limited by relatively small print volumes, several approaches enabling DLW patterning of nanoscale channels within larger thermoplastic microfluidic substrates have been reported to overcome this constraint.241,242
A number of studies have explored 3D printing as a route for low-cost fabrication process of established microfluidic topologies for lipid-based nanoparticle synthesis. Simple 2-port laminar mixers employing millimeter-scale channels have been demonstrated using both FDM and SLA.243 In this work, liposomes with modal diameters above 200 nm and PDI between 0.2–0.3 were achieved, with a slight reduction in both size and polydispersity observed when loading curcumin into the vesicles. At the relatively large size scale of the microchannels employed for this study, no clear benefit for SLA over FDM printing was reported. Other 3D printed devices have been developed with internal structures designed to promote mixing by chaotic advection.243–246 Bifurcation mixers with 1 mm wide intertwined channels have been explored by several groups for the preparation of liposomes loaded with lyzozyme244 and cannabidiol,246 resulting in vesicles with modal diameters ranging from approximately 100–200 nm and PDI values between 0.1–0.2. Surprisingly, smaller 100 nm vesicles were achieved for a bifurcating channel design fabricated via FDM, despite the millimeter-scale channel dimensions.246 Other studies introducing various internal protrusions to the channel floor to promote rapid mixing in SLA-printed devices have reported little benefit to these design modifications.244,245 Several devices based on hydrodynamic flow-focusing have also been explored using 3D printing, although with limited size control demonstrated.247,248 For example, large (>100 nm) and polydisperse ethosomes encapsulating glycyrrhetinic acid were demonstrated using a chip fabricated by FDM-based printing.247
While additive manufacturing enables agile prototyping of new device designs, 3D printing is significantly more expensive than commonly-used replication-based methods such as hot embossing and injection molding for mass production. While 3D printing offers little benefit for high-volume manufacture, new device designs leveraging the unique capabilities of 3D printing can provide benefits not readily achieved using replication-based fabrication methods. For example, 3D printing can be used to overcome the fabrication challenges associated with our previous VFF devices189 for liposome production, which required a challenging multi-layer fabrication process. By employing an SLA process leveraging digital light processing (DLP) for image projection, minimum channel dimensions of 200 μm were realized in an integrated VFF device with a focusing channel aspect ratio of 40.190 Although the minimum vesicle diameter was limited to approximately 90 nm due to the channel resolution provided by the DLP-SLA printing process, PDI values as low as 0.05 were achieved. Significantly, the use of 3D printing also allowed for threaded fittings to be directly integrated into the device during fabrication, enabling leak-free high-pressure operation for lipid processing rates up to 4 mg min−1. We leveraged the same DLP-SLA technology to develop the vortex focusing technology combining diffusive and advective mixing in a single process (Fig. 3C).159 In addition to enabling the complex 3D geometry required to establish a vortical field within a focused lipid stream emerging from a 300 μm diameter injection channel, the printing process was optimized to achieve minimum feature dimensions of 150 μm at the tip of the tapered channel, allowing the critical dead volume at the initial lipid/buffer mixing interface to be minimized. As higher-resolution 3D printing methods continue to advance and become more widely available, new fabrication methods based on these tools will likely enable new microfluidic geometries and designs that will open the door to improved lipid nanomedicine synthesis techniques that are not possible with existing additive manufacturing or planar microfabrication methods.
Regardless of the fabrication process, microfluidic devices for lipid nanoparticle synthesis require materials that are compatible with the reagents and processes used for nanomedicine production. Solvent compatibility is of particular importance to prevent leaching of contaminants from into the drug product. Unlike devices fabricated from inorganic materials including silicon and glass, which are not affected by solvent exposure, photopolymer resins used in stereolithographic 3D printing generally contain toxic monomers and photoinitiators that can potentially be extracted from device substrates upon exposure to both water and nonpolar solvents. Post-processing steps including aggressive rinsing and thorough photopolymer curing can greatly reduce the presence of these unreacted agents in final SLA parts.249,250 Similar concerns exist for devices fabricated from common thermoplastics used for microfluidics, such as polycarbonate which can leach bisphenols and phthalates upon exposure to water,251 but these issues can be largely mitigated through the use of alternate thermoplastics with significantly improved solvent compatibility and lower levels of chemical leaching such as cyclic olefin polymer (COP).252 A related issue impacting the selection of device material is the potential for unwanted substrate deformation through mechanisms such as solvent swelling or stress-induced surface crazing following solvent exposure. For example, significant solvent absorption and substrate swelling occurs in microfluidic devices fabricated from polydimethylsiloxane (PDMS), making this material inappropriate for application to lipid nanoparticle synthesis. In contrast, silicon and glass devices are impermeable to organic solvents, while selected thermoplastics including COP exhibit negligible solvent absorption, making them suitable materials for lipid nanoparticle production. Solvent swelling of microfluidic components manufactured by SLA-based 3D printing is dependent on the selected resin and curing conditions. For the case of common acrylate-based SLA resins, absorption of ethanol has been reported to vary by more than an order of magnitude depending on the resin chemistry and curing time, with minimum swelling levels below 1%.253 Thus while appropriate levels of solvent compatibility can be attained using additive manufacturing, specific materials and processing conditions must be carefully evaluated during the development process.
Conclusion
Following the initial development of microfluidic technologies for lipid-based nanoparticle synthesis over two decades ago, the field has seen enormous progress and growth. While conventional methods for lipid nanoparticle formation continue to evolve, microfluidic platforms are now routinely used for nanomedicine preparation in preclinical studies, and new microfluidic systems optimizing and extending the performance of microfluidic hydrodynamic focusing and chaotic advection mixing are being developed at a rapid pace. The expansion of the field is also evident from the availability of multiple commercial instruments for microfluidic-enabled lipid nanoparticle production, which have made significant inroads in both academia and industry. The advantages offered by microfluidics for tuning nanoparticle size and reducing size variance have been a primary driver of increased interest in the field, and ongoing advances in microfluidic device designs that serve to further enhance size control are likely to continue this trend going forward.
As techniques for improving nanoparticle size control have matured, there has been increased focus on adapting and extending these microfluidic platforms to achieve higher manufacturing throughput and scalable nanomedicine production. These efforts have led to a range of high-throughput device designs based on both diffusive and advective mixing, as well as successful demonstrations of devices employing large arrays of parallel mixing elements that take advantage of repeatable channel geometries achieved through microfabrication. Both single-device scaling and parallelization strategies have shown promise for scale-invariant nanoparticle production, although further work is needed to extend the dynamic range of these devices and improve size uniformity over the full operational range.
In recent years, additive manufacturing has played an important role in allowing new microchannel geometries to be explored for lipid-based nanoparticle production, while also expanding accessibility to these technologies. Due to the resolution limits of typical 3D printing tools, additive manufacturing has been particularly useful for developing microfluidic systems capable of higher throughput operation. However, as the resolution of stereolithography-based systems continues to increase and accessibility to instruments employing two-photon polymerization for nanoscale 3D printing expands, microfluidic designs leveraging the geometric freedom offered these tools may enable new capabilities and define new performance limits that cannot be achieved by planar microfabrication methods.
In contrast to nanoparticle size control and throughput enhancement, less attention has been paid to the development of capabilities supporting the conversion of lipid nanoparticles into fully-processed nanomedicines. While various techniques for nanoparticle surface functionalization and active drug loading have been reported, and several approaches to lipid nanomedicine purification and concentration based on both on-chip and off-chip integration have been investigated, post-processing of lipid nanomedicines represents an area that is ripe for further development. Ultimately, microfluidic technology offers the potential to yield platforms capable of generating final packaged doses directly from initial feedstocks within a fully-integrated device, simplifying nanomedicine production and reducing the likelihood of process contamination. The continuous-flow nature of these microfluidic platforms also creates new possibilities for real-time on-chip nanoparticle monitoring, with the potential to significantly enhance process reliability and agility over conventional batch production methods where periodic sampling is required to ensure product quality. The development of new technologies enabling in-line characterization of nanoparticle properties and process feedback control represents an emerging opportunity within the field of microfluidic-enabled lipid nanomedicine synthesis.
Conflicts of interest
The authors have no conflicts to declare.
Acknowledgements
This work was supported by the National Institutes of Health through grant R21HL159590, and the National Science Foundation through grant CMMI1950234.
References
- M. J. Mitchell, M. M. Billingsley, R. M. Haley, M. E. Wechsler, N. A. Peppas and R. Langer, Nat. Rev. Drug Discovery, 2021, 20(2), 101–124 CrossRef CAS PubMed.
- J. Shi, P. W. Kantoff, R. Wooster and O. C. Farokhzad, Nat. Rev. Cancer, 2016, 17(1), 20–37 CrossRef PubMed.
- M. S. Goldberg, Nat. Rev. Cancer, 2019, 19(10), 587–602 CrossRef CAS PubMed.
- L. Milane and M. Amiji, Drug Delivery Transl. Res., 2021, 11, 1309–1315 CrossRef CAS PubMed.
- X. Lai, M. L. Han, Y. Ding, S. H. Chow, A. P. Le Brun, C. M. Wu, P. J. Bergen, J. Hang Jiang, H. Y. Hsu, B. W. Muir, J. White, J. Song, J. Li and H. H. Shen, Nat. Commun., 2022, 13(1), 1–12 Search PubMed.
- C. Zylberberg, K. Gaskill, S. Pasley and S. Matosevic, Gene Ther., 2017, 24(8), 441–452 CrossRef CAS PubMed.
- H. Yin, K. J. Kauffman and D. G. Anderson, Nat. Rev. Drug Discovery, 2017, 16(6), 387–399 CrossRef CAS PubMed.
- E. Beltrán-Gracia, A. López-Camacho, I. Higuera-Ciapara, J. B. Velázquez-Fernández and A. A. Vallejo-Cardona, Cancer Nanotechnol., 2019, 10, 11 CrossRef.
- W. Gu, G. P. Andrews and Y. Tian, International Journal of Drug Discovery and Pharmacology, 2023, 52–59 CrossRef.
- D. Harries, S. May, W. M. Gelbart and A. Ben-Shaul, Biophys. J., 1998, 75, 159–173 CrossRef CAS PubMed.
- A. R. Thierry, J. Liposome Res., 2008, 7, 143–159 CrossRef.
- G. Guerrini, D. Magrì, S. Gioria, D. Medaglini and L. Calzolai, Nat. Nanotechnol., 2022, 17(6), 570–576 CrossRef CAS PubMed.
- S. C. Semple, S. K. Klimuk, T. O. Harasym, N. Dos Santos, S. M. Ansell, K. F. Wong, N. Maurer, H. Stark, P. R. Cullis, M. J. Hope and P. Scherrer, Biochim. Biophys. Acta, Biomembr., 2001, 1510, 152–166 CrossRef CAS PubMed.
- R. H. Müller, K. Mäder and S. Gohla, Eur. J. Pharm. Biopharm., 2000, 50, 161–177 CrossRef PubMed.
- W. Mehnert and K. Mäder, Adv. Drug Delivery Rev., 2001, 47, 165–196 CrossRef CAS PubMed.
- R. H. Müller, M. Radtke and S. A. Wissing, Adv. Drug Delivery Rev., 2002, 54, S131–S155 CrossRef PubMed.
- M. Dhaval, P. Vaghela, K. Patel, K. Sojitra, M. Patel, S. Patel, K. Dudhat, S. Shah, R. Manek and R. Parmar, Drug Delivery Transl. Res., 2022, 12, 1616–1639 CrossRef CAS PubMed.
- R. Jukanti, S. Sheela, S. Bandari and P. R. Veerareddy, J. Pharm. Sci., 2011, 100, 3208–3222 CrossRef CAS PubMed.
- E. Touitou, N. Dayan, L. Bergelson, B. Godin and M. Eliaz, J. Controlled Release, 2000, 65, 403–418 CrossRef CAS PubMed.
- K. Larsson, J. Phys. Chem., 1989, 93, 7304–7314 CrossRef CAS.
- P. T. Spicer, Curr. Opin. Colloid Interface Sci., 2005, 10, 274–279 CrossRef CAS.
- A. L. Troutier, T. Delair, C. Pichot and C. Ladavière, Langmuir, 2005, 21, 1305–1313 CrossRef CAS PubMed.
- S. K. E. Messerschmidt, A. Musyanovych, M. Altvater, P. Scheurich, K. Pfizenmaier, K. Landfester and R. E. Kontermann, J. Controlled Release, 2009, 137, 69–77 CrossRef CAS PubMed.
- L. Zhang, J. M. Chan, F. X. Gu, J. W. Rhee, A. Z. Wang, A. F. Radovic-Moreno, F. Alexis, R. Langer and O. C. Farokhzad, ACS Nano, 2008, 2, 1696–1702 CrossRef CAS PubMed.
- V. Torchilin, Curr. Drug Delivery, 2005, 2, 319–327 CrossRef CAS PubMed.
- A. I. Antoniou, S. Giofrè, P. Seneci, D. Passarella and S. Pellegrino, Drug Discovery Today, 2021, 26, 1794–1824 CrossRef CAS PubMed.
- A. D. Bangham, M. M. Standish and J. C. Watkins, J. Mol. Biol., 1965, 13, 238–252 CrossRef CAS PubMed.
- G. Gregoriadis, P. D. Leathwood and B. E. Ryman, FEBS Lett., 1971, 14, 95–99 CrossRef CAS PubMed.
- G. Gregoriadis and B. E. Ryman, Biochem. J., 1972, 129, 123 CrossRef CAS PubMed.
- G. Gregoriadis, FEBS Lett., 1973, 36, 292–296 CrossRef CAS PubMed.
- A. C. Allison and G. Gregoriadis, Nature, 1974, 252(5480), 252 CrossRef CAS PubMed.
- Y. C. Barenholz, J. Controlled Release, 2012, 160, 117–134 CrossRef CAS PubMed.
- E. D. Namiot, A. V. Sokolov, V. N. Chubarev, V. V. Tarasov and H. B. Schiöth, Int. J. Mol. Sci., 2023, 24, 787 CrossRef CAS PubMed.
- P. Marqués-Gallego and A. I. P. M. De Kroon, BioMed Res. Int., 2014, 2014, 129458 Search PubMed.
- M. K. Riaz, M. A. Riaz, X. Zhang, C. Lin, K. H. Wong, X. Chen, G. Zhang, A. Lu and Z. Yang, Int. J. Mol. Sci., 2018, 19(1), 195 CrossRef PubMed.
- L. Nobs, F. Buchegger, R. Gurny and E. Allémann, J. Pharm. Sci., 2004, 93, 1980–1992 CrossRef CAS PubMed.
- D. Papahadjopoulos, T. M. Allen, A. Gabizon, E. Mayhew, K. Matthay, S. K. Huang, K. D. Lee, M. C. Woodle, D. D. Lasic, C. Redemann and F. J. Martin, Proc. Natl. Acad. Sci. U. S. A., 1991, 88, 11460–11464 CrossRef CAS PubMed.
- S. R. Paliwal, R. Paliwal and S. P. Vyas, Drug Delivery, 2015, 22, 231–242 CrossRef CAS PubMed.
- C. Lopes, J. Cristóvão, V. Silvério, P. R. Lino and P. Fonte, Expert Opin. Drug Delivery, 2022, 19, 1381–1395 CrossRef CAS PubMed.
- S. Marre and K. F. Jensen, Chem. Soc. Rev., 2010, 39, 1183–1202 RSC.
- Y. Liu, G. Yang, Y. Hui, S. Ranaweera, C.-X. Zhao, Y. Liu, G. Yang, S. Ranaweera, C.-X. Zhao and Y. Hui, Small, 2022, 18, 2106580 CrossRef CAS PubMed.
- S. J. Shepherd, D. Issadore and M. J. Mitchell, Biomaterials, 2021, 274, 120826 CrossRef CAS PubMed.
- E. Jaradat, E. Weaver, A. Meziane and D. A. Lamprou, Nanomaterials, 2021, 11(12), 3440 CrossRef CAS PubMed.
- L. Zhang, Q. Chen, Y. Ma and J. Sun, ACS Appl. Bio Mater., 2020, 3, 107–120 CrossRef CAS PubMed.
- M. A. Tomeh and X. Zhao, Mol. Pharmaceutics, 2020, 17, 4421–4434 CrossRef CAS PubMed.
- Q. Ma, J. Cao, Y. Gao, S. Han, Y. Liang, T. Zhang, X. Wang and Y. Sun, Nanoscale, 2020, 12, 15512–15527 RSC.
- P. Shrimal, G. Jadeja and S. Patel, Chem. Eng. Res. Des., 2020, 153, 728–756 CrossRef CAS.
- S. Streck, L. Hong, B. J. Boyd and A. McDowell, Pharm. Nanotechnol., 2019, 7, 423–443 CrossRef CAS PubMed.
- X. Luo, P. Su, W. Zhang and C. L. Raston, Adv. Mater. Technol., 2019, 4, 1900488 CrossRef CAS.
- J. P. Martins, G. Torrieri and H. A. Santos, Expert Opin. Drug Delivery, 2018, 15, 469–479 CrossRef CAS PubMed.
- D. Liu, H. Zhang, F. Fontana, J. T. Hirvonen and H. A. Santos, Adv. Drug Delivery Rev., 2018, 128, 54–83 CrossRef CAS PubMed.
- J. Ahn, J. Ko, S. Lee, J. Yu, Y. T. Kim and N. L. Jeon, Adv. Drug Delivery Rev., 2018, 128, 29–53 CrossRef CAS PubMed.
- R. Ran, Q. Sun, T. Baby, D. Wibowo, A. P. J. Middelberg and C. X. Zhao, Chem. Eng. Sci., 2017, 169, 78–96 CrossRef CAS.
- J. Ma, S. M. Y. Lee, C. Yi and C. W. Li, Lab Chip, 2017, 17, 209–226 RSC.
- D. Liu, H. Zhang, F. Fontana, J. T. Hirvonen and H. A. Santos, Lab Chip, 2017, 17, 1856–1883 RSC.
- K. Illath, S. Kar, P. Gupta, A. Shinde, S. Wankhar, F. G. Tseng, K. T. Lim, M. Nagai and T. S. Santra, Biomaterials, 2022, 280, 121247 CrossRef CAS PubMed.
- S. Garg, G. Heuck, S. Ip and E. Ramsay, J. Drug Targeting, 2016, 24, 821–835 CrossRef CAS PubMed.
- Q. Feng, J. Sun and X. Jiang, Nanoscale, 2016, 8, 12430–12443 RSC.
- I. U. Khan, C. A. Serra, N. Anton and T. F. Vandamme, Expert Opin. Drug Delivery, 2015, 12, 547–562 CrossRef PubMed.
- P. M. Valencia, O. C. Farokhzad, R. Karnik and R. Langer, Nat. Nanotechnol., 2012, 7, 623–629 CrossRef CAS PubMed.
- L. Capretto, D. Carugo, S. Mazzitelli, C. Nastruzzi and X. Zhang, Adv. Drug Delivery Rev., 2013, 65, 1496–1532 CrossRef CAS PubMed.
- J. P. Martins, G. Torrieri and H. A. Santos, Expert Opin. Drug Delivery, 2018, 15, 469–479 CrossRef CAS PubMed.
- M. Rawas-Qalaji, R. Cagliani, N. Al-hashimi, R. Al-Dabbagh, A. Al-Dabbagh and Z. Hussain, Pharm. Dev. Technol., 2023, 28, 61–77 CrossRef CAS PubMed.
- Z. Ma, B. Li, J. Peng and D. Gao, Pharmaceutics, 2022, 14, 434 CrossRef CAS PubMed.
- A. Forigua, R. L. Kirsch, S. M. Willerth and K. S. Elvira, J. Controlled Release, 2021, 333, 258–268 CrossRef CAS PubMed.
- S. T. Sanjay, W. Zhou, M. Dou, H. Tavakoli, L. Ma, F. Xu and X. J. Li, Adv. Drug Delivery Rev., 2018, 128, 3–28 CrossRef CAS PubMed.
- M. Björnmalm, Y. Yan and F. Caruso, J. Controlled Release, 2014, 190, 139–149 CrossRef PubMed.
- G. Zhang and J. Sun, Int. J. Nanomed., 2021, 16, 7391 CrossRef CAS PubMed.
- D. Carugo, E. Bottaro, J. Owen, E. Stride and C. Nastruzzi, Sci. Rep., 2016, 6(1), 1–15 CrossRef PubMed.
- D. Van Swaay and A. Demello, Lab Chip, 2013, 13, 752–767 RSC.
- M. Maeki, S. Uno, A. Niwa, Y. Okada and M. Tokeshi, J. Controlled Release, 2022, 344, 80–96 CrossRef CAS PubMed.
- G. Prakash, A. Shokr, N. Willemen, S. M. Bashir, S. R. Shin and S. Hassan, Adv. Drug Delivery Rev., 2022, 184, 114197 CrossRef CAS PubMed.
- T. Nakamura and H. Harashima, Adv. Drug Delivery Rev., 2020, 167, 78–88 CrossRef CAS PubMed.
- C. B. Roces, G. Lou, N. Jain, S. Abraham, A. Thomas, G. W. Halbert and Y. Perrie, Pharmaceutics, 2020, 12, 1–19 CrossRef PubMed.
- M. J. W. Evers, J. A. Kulkarni, R. van der Meel, P. R. Cullis, P. Vader, R. M. Schiffelers, M. J. W. Evers, R. van der Meel, P. Vader, R. M. Schiffelers, J. A. Kulkarni and P. R. Cullis, Small Methods, 2018, 2, 1700375 CrossRef.
- A. Vogelaar, S. Marcotte, J. Cheng, B. Oluoch and J. Zaro, Pharmaceutics, 2023, 15, 1053 CrossRef CAS PubMed.
- K. Osouli-Bostanabad, S. Puliga, D. R. Serrano, A. Bucchi, G. Halbert and A. Lalatsa, Pharmaceutics, 2022, 14, 1940 CrossRef CAS PubMed.
- B. G. Carvalho, B. T. Ceccato, M. Michelon, S. W. Han and L. G. de la Torre, Pharmaceutics, 2022, 14, 141 CrossRef CAS PubMed.
- M. S. Ali, N. Hooshmand, M. El-Sayed and H. I. Labouta, ACS Appl. Bio Mater., 2021, 17, 45 Search PubMed.
- C. Liu, Q. Feng and J. Sun, Adv. Mater., 2019, 31, 1804788 CrossRef CAS PubMed.
- M. Maeki, N. Kimura, Y. Sato, H. Harashima and M. Tokeshi, Adv. Drug Delivery Rev., 2018, 128, 84–100 CrossRef CAS PubMed.
- L. Xu, X. Wang, Y. Liu, G. Yang, R. J. Falconer and C.-X. Zhao, Adv. NanoBiomed Res., 2022, 2, 2100109 CrossRef CAS.
- J. N. Israelachvili, D. J. Mitchell and B. W. Ninham, Biochim. Biophys. Acta, Biomembr., 1977, 470, 185–201 CrossRef CAS PubMed.
- D. Lombardo and M. A. Kiselev, Pharmaceutics, 2022, 14, 543 CrossRef CAS PubMed.
- V. V. S. N. L. Andra, S. V. N. Pammi, L. V. K. P. Bhatraju and L. K. Ruddaraju, Bionanoscience, 2022, 12, 274–291 CrossRef PubMed.
- F. Szoka and D. Papahadjopoulos, Proc. Natl. Acad. Sci. U. S. A., 1978, 75, 4194–4198 CrossRef CAS PubMed.
- R. Cortesi, E. Esposito, S. Gambarin, P. Telloli, E. Menegatti and C. Nastruzzi, J. Microencapsulation, 1999, 16, 251–256 CrossRef CAS PubMed.
-
N.-Q. Shi and X.-R. Qi, Liposome-Based Drug Delivery Systems, 2018, pp. 1–10 Search PubMed.
- D. Peer and R. Margalit, Arch. Biochem. Biophys., 2000, 383, 185–190 CrossRef CAS PubMed.
- K. Otake, T. Shimomura, T. Goto, T. Imura, T. Furuya, S. Yoda, Y. Takebayashi, H. Sakai and M. Abe, Langmuir, 2006, 22, 2543–2550 CrossRef CAS PubMed.
- K. Otake, T. Imura, H. Sakai and M. Abe, Langmuir, 2001, 17, 3898–3901 CrossRef CAS.
- R. Mendez and S. Banerjee, Methods Mol. Biol., 2017, 1609, 255–260 CrossRef CAS PubMed.
- R. L. Hamilton, J. Goerke, L. S. Guo, M. C. Williams and R. J. Havel, J. Lipid Res., 1980, 21, 981–992 CrossRef CAS.
- E. Mayhew, R. Lazo, W. J. Vail, J. King and A. M. Green, Biochim. Biophys. Acta, 1984, 775, 169–174 CrossRef CAS PubMed.
- A. Vidal-Naquet, J. L. Gossage, T. P. Sullivan, J. W. Haynes, B. H. Gilruth, R. L. Beissinger, L. R. Sehgal and A. L. Rosen, Biomater., Artif. Cells, Artif. Organs, 2009, 17, 531–552 CrossRef PubMed.
- F. Olson, C. A. Hunt, F. C. Szoka, W. J. Vail and D. Papahadjopoulos, Biochim. Biophys. Acta, Biomembr., 1979, 557, 9–23 CrossRef CAS PubMed.
- N. Berger, A. Sachse, J. Bender, R. Schubert and M. Brandl, Int. J. Pharm., 2001, 223, 55–68 CrossRef CAS PubMed.
- P. Guo, J. Huang, Y. Zhao, C. R. Martin, R. N. Zare and M. A. Moses, Small, 2018, 14, 1703493 CrossRef PubMed.
- J. Leng, S. U. Egelhaaf and M. E. Cates, Biophys. J., 2003, 85, 1624–1646 CrossRef CAS PubMed.
- M. Ollivon, S. Lesieur, C. Grabielle-Madelmont and M. Paternostre, Biochim. Biophys. Acta, Biomembr., 2000, 1508, 34–50 CrossRef CAS PubMed.
- O. Zumbuehl and H. G. Weder, Biochim. Biophys. Acta, Biomembr., 1981, 640, 252–262 CrossRef CAS PubMed.
- V. Rhoden and S. M. Goldin, Biochemistry, 1979, 18, 4173–4176 CrossRef CAS PubMed.
- P. Schurtenberger, M. Svärd, E. Wehrli and B. Lindman, Biochim. Biophys. Acta, Gen. Subj., 1986, 882, 465–468 CrossRef CAS PubMed.
- S. Batzri and E. D. Korn, Biochim. Biophys. Acta, Biomembr., 1973, 298, 1015–1019 CrossRef CAS PubMed.
- A. Wagner, K. Vorauer-Uhl, G. Kreismayr and H. Katinger, J. Liposome Res., 2002, 12, 259–270 CrossRef CAS PubMed.
- S. Hirota, C. T. de Ilarduya, L. G. Barron and F. C. Szoka, BioTechniques, 1999, 27, 286–290 CrossRef CAS PubMed.
- L. B. Jeffs, L. R. Palmer, E. G. Ambegia, C. Giesbrecht, S. Ewanick and I. MacLachlan, Pharm. Res., 2005, 22, 362–372 CrossRef CAS PubMed.
- Y. Liu, C. Cheng, Y. Liu, R. K. Prud'homme and R. O. Fox, Chem. Eng. Sci., 2008, 63, 2829–2842 CrossRef CAS.
- C. E. Markwalter and R. K. Prud'homme, J. Pharm. Sci., 2018, 107, 2465–2471 CrossRef CAS PubMed.
- H. Zheng, H. Tao, J. Wan, K. Y. Lee, Z. Zheng and S. S. Y. Leung, Pharmaceutics, 2022, 14(6), 1223 CrossRef CAS PubMed.
- M. A. Tomeh, M. H. Mansor, R. Hadianamrei, W. Sun and X. Zhao, Int. J. Pharm., 2022, 620, 121762 CrossRef CAS PubMed.
- B. K. Johnson and R. K. Prud'homme, AIChE J., 2003, 49, 2264–2282 CrossRef CAS.
- B. K. Johnson, W. Saad and R. K. Prud'homme, ACS Symp. Ser., 2006, 924, 278–291 CrossRef CAS.
- N. Warne, M. Ruesch, P. Siwik, P. Mensah, J. Ludwig, M. Hripcsak, R. Godavarti, A. Prigodich and M. Dolsten, Nat. Biotechnol., 2023, 41(2), 183–188 CAS.
- A. Jahn, W. N. Vreeland, M. Gaitan and L. E. Locascio, J. Am. Chem. Soc., 2004, 126, 2674–2675 CrossRef CAS PubMed.
- A. Jahn, W. N. Vreeland, D. L. DeVoe, L. E. Locascio and M. Gaitan, Langmuir, 2007, 23, 6289–6293 CrossRef CAS PubMed.
- A. Jahn, F. Lucas, R. A. Wepf and P. S. Dittrich, Langmuir, 2013, 29, 1717–1723 CrossRef CAS PubMed.
- A. Jahn, S. M. Stavis, J. S. Hong, W. N. Vreeland, D. L. DeVoe and M. Gaitan, ACS Nano, 2010, 4, 2077–2087 CrossRef CAS PubMed.
- A. Jahn, J. E. Reiner, W. N. Vreeland, D. L. DeVoe, L. E. Locascio and M. Gaitan, J. Nanopart. Res., 2008, 10, 893–1087 CrossRef.
- R. V. Tien Sing Young and M. Tabrizian, Biomicrofluidics, 2015, 9(4), 046501 CrossRef PubMed.
- R. R. Hood, D. L. DeVoe, J. Atencia, W. N. Vreeland and D. M. Omiatek, Lab Chip, 2014, 14, 2403–2409 RSC.
- R. R. Hood, C. Shao, D. M. Omiatek, W. N. Vreeland and D. L. DeVoe, Pharm. Res., 2013, 30, 1597–1607 CrossRef CAS PubMed.
- X. Huang, R. J. Lee, Y. Qi, Y. Li, J. Lu, Q. Meng, L. Teng and J. Xie, Oncotarget, 2017, 8, 96826 CrossRef PubMed.
- T. A. Balbino, J. M. Serafin, A. A. Malfatti-Gasperini, C. L. P. De Oliveira, L. P. Cavalcanti, M. B. De Jesus and L. G. De La Torre, Langmuir, 2016, 32, 1799–1807 CrossRef CAS PubMed.
- T. A. Balbino, A. R. Azzoni and L. G. De la Torre, Colloids Surf., B, 2013, 111, 203–210 CrossRef CAS PubMed.
- C. G. Koh, X. Zhang, S. Liu, S. Golan, B. Yu, X. Yang, J. Guan, Y. Jin, Y. Talmon, N. Muthusamy, K. K. Chan, J. C. Byrd, R. J. Lee, G. Marcucci and L. J. Lee, J. Controlled Release, 2010, 141, 62–69 CrossRef CAS PubMed.
- Z. Yang, B. Yu, J. Zhu, X. Huang, J. Xie, S. Xu, X. Yang, X. Wang, B. C. Yung, L. J. Lee, R. J. Lee and L. Teng, Nanoscale, 2014, 6, 9742–9751 RSC.
- T. Panagiotou, S. V. Mesite and R. J. Fisher, Ind. Eng. Chem. Res., 2009, 48, 1761–1771 CrossRef CAS.
- K. Tahara, M. Nishikawa, K. Matsui, K. Hisazumi, R. Onodera, Y. Tozuka and H. Takeuchi, Pharm. Res., 2016, 33, 2259–2268 CrossRef CAS PubMed.
- H. Zhao, J. X. Wang, Q. A. Wang, J. F. Chen and J. Yun, Ind. Eng. Chem. Res., 2007, 46, 8229–8235 CrossRef CAS.
- Y. Dong, W. K. Ng, J. Hu, S. Shen and R. B. H. Tan, Powder Technol., 2014, 268, 424–428 CrossRef CAS.
- H. S. M. Ali, P. York, A. M. A. Ali and N. Blagden, J. Controlled Release, 2011, 149, 175–181 CrossRef CAS PubMed.
- J. X. Wang, Q. X. Zhang, Y. Zhou, L. Shao and J. F. Chen, Chem. Eng. J., 2010, 162, 844–851 CrossRef CAS.
- H. Aref, J. Fluid Mech., 1984, 143, 1–21 CrossRef.
- N. T. Nguyen and Z. Wu, J. Micromech. Microeng., 2005, 15(2), R1–R16 CrossRef.
- V. Hessel, H. Löwe and F. Schönfeld, Chem. Eng. Sci., 2005, 60, 2479–2501 CrossRef CAS.
- G. Cai, L. Xue, H. Zhang and J. Lin, Micromachines, 2017, 8(9), 274 CrossRef PubMed.
- Y. K. Suh and S. Kang, Micromachines, 2010, 1, 82–111 CrossRef.
- K. Ward and Z. H. Fan, J. Micromech. Microeng., 2015, 25(9), 094001 CrossRef PubMed.
- C. Y. Lee, C. L. Chang, Y. N. Wang and L. M. Fu, Int. J. Mol. Sci., 2011, 12, 3263–3287 CrossRef CAS PubMed.
- C. Y. Lee, W. T. Wang, C. C. Liu and L. M. Fu, Chem. Eng. J., 2016, 288, 146–160 CrossRef CAS.
- A. D. Stroock, S. K. W. Dertinger, A. Ajdari, I. Mezić, H. A. Stone and G. M. Whitesides, Science, 2002, 295, 647–651 CrossRef CAS PubMed.
- I. V. Zhigaltsev, N. Belliveau, I. Hafez, A. K. K. Leung, J. Huft, C. Hansen and P. R. Cullis, Langmuir, 2012, 28, 3633–3640 CrossRef CAS PubMed.
- N. M. Belliveau, J. Huft, P. J. Lin, S. Chen, A. K. Leung, T. J. Leaver, A. W. Wild, J. B. Lee, R. J. Taylor, Y. K. Tam, C. L. Hansen and P. R. Cullis, Mol. Ther.--Nucleic Acids, 2012, 1, e37 CrossRef PubMed.
- E. Kastner, R. Kaur, D. Lowry, B. Moghaddam, A. Wilkinson and Y. Perrie, Int. J. Pharm., 2015, 477, 361–368 CrossRef PubMed.
- E. Kastner, V. Verma, D. Lowry and Y. Perrie, Int. J. Pharm., 2015, 485, 122–130 CrossRef CAS PubMed.
- S. Joshi, M. T. Hussain, C. B. Roces, G. Anderluzzi, E. Kastner, S. Salmaso, D. J. Kirby and Y. Perrie, Int. J. Pharm., 2016, 514, 160–168 CrossRef CAS PubMed.
- I. V. Zhigaltsev, Y. K. Tam, A. K. K. Leung and P. R. Cullis, J. Liposome Res., 2016, 26, 96–102 CAS.
- G. Lou, G. Anderluzzi, S. Woods, C. W. Roberts and Y. Perrie, Eur. J. Pharm. Biopharm., 2019, 143, 51–60 CrossRef CAS PubMed.
- C. Webb, S. Khadke, S. Tandrup Schmidt, C. B. Roces, N. Forbes, G. Berrie and Y. Perrie, Pharmaceutics, 2019, 11, 653 CrossRef CAS PubMed.
- F. Jiang, K. S. Drese, S. Hardt, M. Küpper and F. Schönfeld, AIChE J., 2004, 50, 2297–2305 CrossRef CAS.
- N. Kimura, M. Maeki, Y. Sato, Y. Note, A. Ishida, H. Tani, H. Harashima and M. Tokeshi, ACS Omega, 2018, 3, 5044–5051 CrossRef CAS PubMed.
- N. Kimura, M. Maeki, Y. Sato, A. Ishida, H. Tani, H. Harashima and M. Tokeshi, ACS Appl. Mater. Interfaces, 2020, 12, 34011–34020 CrossRef CAS PubMed.
- R. R. López, I. Ocampo, L. M. Sánchez, A. Alazzam, K. F. Bergeron, S. Camacho-León, C. Mounier, I. Stiharu and V. Nerguizian, Micromachines, 2020, 11(3), 235 CrossRef PubMed.
- P. C. O. S. Firmino, S. S. V. Vianna, O. M. M. M. da Costa, A. A. Malfatti-Gasperini, A. L. Gobbi, R. S. Lima and L. G. de la Torre, Lab Chip, 2021, 21(15), 2971–2985 RSC.
- M. A. Ansari, K. Y. Kim, K. Anwar and S. M. Kim, J. Micromech. Microeng., 2010, 20, 055007 CrossRef.
- G. Xia, J. Li, X. Tian and M. Zhou, Ind. Eng. Chem. Res., 2012, 51, 7816–7827 CrossRef CAS.
- C. Webb, N. Forbes, C. B. Roces, G. Anderluzzi, G. Lou, S. Abraham, L. Ingalls, K. Marshall, T. J. Leaver, J. A. Watts, J. W. Aylott and Y. Perrie, Int. J. Pharm., 2020, 582, 119266 CrossRef CAS PubMed.
- H. Y. Han, J. La Fiandra and D. L. DeVoe, Nat. Commun., 2022, 13, 6997 CrossRef PubMed.
- Y. H. Bae and K. Park, J. Controlled Release, 2011, 153, 198–205 CrossRef CAS PubMed.
- A. Nagayasu, K. Uchiyama and H. Kiwada, Adv. Drug Delivery Rev., 1999, 40, 75–87 CrossRef CAS PubMed.
- T. Ishida, H. Harashima and H. Kiwada, Biosci. Rep., 2002, 22, 197–224 CrossRef CAS PubMed.
- M. Danaei, M. Dehghankhold, S. Ataei, F. Hasanzadeh Davarani, R. Javanmard, A. Dokhani, S. Khorasani and M. R. Mozafari, Pharmaceutics, 2018, 10(2), 57 CrossRef PubMed.
- D. E. Large, R. G. Abdelmessih, E. A. Fink and D. T. Auguste, Adv. Drug Delivery Rev., 2021, 176, 113851 CrossRef CAS PubMed.
- A. U. Andar, R. R. Hood, W. N. Vreeland, D. L. DeVoe and P. W. Swaan, Pharm. Res., 2014, 31, 401–413 CrossRef CAS PubMed.
- D. C. Litzinger, A. M. Buiting, N. van Rooijen and L. Huang, Biochim. Biophys. Acta, 1994, 1190, 99–107 CrossRef CAS PubMed.
- T. Nakamura, M. Kawai, Y. Sato, M. Maeki, M. Tokeshi and H. Harashima, Mol. Pharmaceutics, 2020, 17, 944–953 CrossRef CAS PubMed.
- V. M. Shah, D. X. Nguyen, P. Patel, B. Cote, A. Al-Fatease, Y. Pham, M. G. Huynh, Y. Woo and A. W. Alani, Nanomedicine, 2019, 18, 146–156 CrossRef CAS PubMed.
- E. Blanco, H. Shen and M. Ferrari, Nat. Biotechnol., 2015, 33, 941–951 CrossRef CAS PubMed.
- R. Langer, Science, 1990, 249, 1527–1533 CrossRef CAS PubMed.
- D. Peer, J. M. Karp, S. Hong, O. C. Farokhzad, R. Margalit and R. Langer, Nat. Nanotechnol., 2007, 2, 751–760 CrossRef CAS PubMed.
- S. K. Hobbs, W. L. Monsky, F. Yuan, W. G. Roberts, L. Griffith, V. P. Torchilin and R. K. Jain, Proc. Natl. Acad. Sci. U. S. A., 1998, 95, 4607–4612 CrossRef CAS PubMed.
- F. C. Lam, S. W. Morton, J. Wyckoff, T. L. Vu Han, M. K. Hwang, A. Maffa, E. Balkanska-Sinclair, M. B. Yaffe, S. R. Floyd and P. T. Hammond, Nat. Commun., 2018, 9, 1991 CrossRef PubMed.
- M. Agrawal, Ajazuddin, D. K. Tripathi, S. Saraf, S. Saraf, S. G. Antimisiaris, S. Mourtas, M. Hammarlund-Udenaes and A. Alexander, J. Controlled Release, 2017, 260, 61–77 CrossRef CAS PubMed.
- I. P. Kaur, R. Bhandari, S. Bhandari and V. Kakkar, J. Controlled Release, 2008, 127, 97–109 CrossRef CAS PubMed.
- R. R. Hood, E. L. Kendall, M. Junqueira, W. N. Vreeland, Z. Quezado, J. C. Finkel and D. L. DeVoe, PLoS One, 2014, 9, e92978 CrossRef PubMed.
- L. Kou, Y. D. Bhutia, Q. Yao, Z. He, J. Sun and V. Ganapathy, Front. Pharmacol., 2018, 9, 27 CrossRef.
- N. Hoshyar, S. Gray, H. Han and G. Bao, Nanomedicine, 2016, 11, 673–692 CrossRef CAS.
- S. Chakraborty, M. Doktorova, T. R. Molugu, F. A. Heberle, H. L. Scott, B. Dzikovski, M. Nagao, L. R. Stingaciu, R. F. Standaert, F. N. Barrera, J. Katsaras, G. Khelashvili, M. F. Brown and R. Ashkar, Proc. Natl. Acad. Sci. U. S. A., 2020, 117, 21896–21905 CrossRef CAS PubMed.
- D. D. Lasic, Biochem. J., 1988, 256, 1–11 CrossRef CAS PubMed.
- J. N. Israelachvili, D. J. Mitchell and B. W. Ninham, Biochim. Biophys. Acta, Biomembr., 1977, 470, 185–201 CrossRef CAS PubMed.
- S. J. Marrink, E. Lindahl, O. Edholm and A. E. Mark, J. Am. Chem. Soc., 2001, 123, 8638–8639 CrossRef CAS PubMed.
- H. Noguchi and G. Gompper, J. Chem. Phys., 2006, 125(16), 164908 CrossRef PubMed.
- H. Noguchi and M. Takasu, Phys. Rev. E: Stat., Nonlinear, Soft Matter Phys., 2001, 64, 041913 CrossRef CAS PubMed.
- S. Yamamoto, Y. Maruyama and S. A. Hyodo, J. Chem. Phys., 2002, 116, 5842–5849 CrossRef CAS.
- P. A. M. M. Aarts, O. L. J. Gijzeman, J. M. H. Kremer and P. H. Wiersema, Chem. Phys. Lipids, 1977, 19, 267–274 CrossRef CAS PubMed.
- M. Maeki, Y. Fujishima, Y. Sato, T. Yasui, N. Kaji, A. Ishida, H. Tani, Y. Baba, H. Harashima and M. Tokeshi, PLoS One, 2017, 12, e0187962 CrossRef PubMed.
- A. Gouda, O. S. Sakr, M. Nasr and O. Sammour, J. Drug Delivery Sci. Technol., 2021, 61, 102174 CrossRef CAS.
- R. R. Hood and D. L. DeVoe, Small, 2015, 11, 5790–5799 CrossRef CAS PubMed.
- Z. Chen, J. Y. J. Y. Han, L. Shumate, R. Fedak and D. L. DeVoe, Adv. Mater. Technol., 2019, 4, 1800511 CrossRef.
-
A. D. McNaught and A. Wilkinson, IUPAC Compendium of Chemical Terminology, Blackwell Scientific Publications, Oxford, 1997 Search PubMed.
- U.S. Food and Drug Administration, Center for Drug Evaluation and Research.
-
Liposome Drug Products: Chemistry, Manufacturing, and Controls; Human Pharmacokinetics and Bioavailability; and Labeling Documentation, Silver Spring, MD, 2018 Search PubMed.
- B. G. Tenchov, T. K. Yanev, M. G. Tihova and R. D. Koynova, Biochim. Biophys. Acta, Biomembr., 1985, 816, 122–130 CrossRef CAS PubMed.
- B. A. Korgel, J. H. van Zanten and H. G. Monbouquette, Biophys. J., 1998, 74, 3264–3272 CrossRef CAS PubMed.
- X. Liu and H. Meng, View, 2021, 2, 20200190 CrossRef CAS.
- J. L. Berger, A. Smith, K. K. Zorn, P. Sukumvanich, A. B. Olawaiye, J. Kelley and T. C. Krivak, OncoTargets Ther., 2014, 7, 1409–1413 CAS.
-
A Review of FDA's Approach to Medical Product Shortages, 2011 Search PubMed.
- A. Wagner and K. Vorauer-Uhl, J. Drug Delivery, 2011, 2011, 591325 Search PubMed.
- S. Priya, V. M. Desai and G. Singhvi, ACS Omega, 2023, 8(1), 74–86 CrossRef CAS PubMed.
- Y. Sugimoto, T. Suga, N. Kato, M. Umino, A. Yamayoshi, H. Mukai and S. Kawakami, Int. J. Nanomed., 2022, 17, 6675 CrossRef CAS PubMed.
- C. A. Hermann, M. Mayer, C. Griesche, F. Beck and A. J. Baeumner, Analyst, 2021, 146, 997–1003 RSC.
- Y. Li, R. J. Lee, X. Huang, Y. Li, B. Lv, T. Wang, Y. Qi, F. Hao, J. Lu, Q. Meng, L. Teng, Y. Zhou, J. Xie and L. Teng, Nanomedicine, 2017, 13, 371–381 CrossRef CAS PubMed.
- T. A. Balbino, J. M. Serafin, A. Radaic, M. B. de Jesus and L. G. de la Torre, Colloids Surf., B, 2017, 152, 406–413 CrossRef CAS PubMed.
- R. R. Hood, W. N. Vreeland and D. L. DeVoe, Lab Chip, 2014, 14, 3359–3367 RSC.
- Y. Barenholz, Curr. Opin. Colloid Interface Sci., 2001, 6, 66–77 CrossRef CAS.
- United States Pharmacopeia – National Formulary (USP–NF), 2023.
- European Pharmacopoeia 11.2 (Ph. Eur.), 2023.
- N. Dimov, E. Kastner, M. Hussain, Y. Perrie and N. Szita, Sci. Rep., 2017, 7(1), 1–13 CrossRef CAS PubMed.
- Z. Wang, H. J. Wu, D. Fine, J. Schmulen, Y. Hu, B. Godin, J. X. J. Zhang and X. Liu, Lab Chip, 2013, 13, 2879–2882 RSC.
- L. Matlock-Colangelo, D. Cho, C. L. Pitner, M. W. Frey and A. J. Baeumner, Lab Chip, 2012, 12, 1696–1701 RSC.
- C. E. Torres, J. Cifuentes, S. C. Gómez, V. Quezada, K. A. Giraldo, P. R. Puentes, L. Rueda-Gensini, J. A. Serna, C. Muñoz-Camargo, L. H. Reyes, J. F. Osma and J. C. Cruz, Pharmaceutics, 2022, 14, 315 CrossRef CAS PubMed.
- T. Salafi, K. K. Zeming and Y. Zhang, Lab Chip, 2016, 17, 11–33 RSC.
- B. Talebjedi, N. Tasnim, M. Hoorfar, G. F. Mastromonaco and M. De Almeida Monteiro Melo Ferraz, Front. Vet. Sci., 2020, 7, 620809 CrossRef PubMed.
- J. C. Yeo, Kenry, Z. Zhao, P. Zhang, Z. Wang and C. T. Lim, Biomicrofluidics, 2018, 12(2), 024103 CrossRef PubMed.
- H. K. Woo, V. Sunkara, J. Park, T. H. Kim, J. R. Han, C. J. Kim, H. Il Choi, Y. K. Kim and Y. K. Cho, ACS Nano, 2017, 11, 1360–1370 CrossRef CAS PubMed.
- L. G. Liang, M. Q. Kong, S. Zhou, Y. F. Sheng, P. Wang, T. Yu, F. Inci, W. P. Kuo, L. J. Li, U. Demirci and S. Q. Wang, Sci. Rep., 2017, 7, 46224 CrossRef PubMed.
- M. Wu, Y. Ouyang, Z. Wang, R. Zhang, P. H. Huang, C. Chen, H. Li, P. Li, D. Quinn, M. Dao, S. Suresh, Y. Sadovsky and T. J. Huang, Proc. Natl. Acad. Sci. U. S. A., 2017, 114, 10584–10589 CrossRef CAS PubMed.
- A. Ku, H. C. Lim, M. Evander, H. Lilja, T. Laurell, S. Scheding and Y. Ceder, Anal. Chem., 2018, 90, 8011–8019 CrossRef CAS PubMed.
- C. Liu, J. Guo, F. Tian, N. Yang, F. Yan, Y. Ding, J. Wei, G. Hu, G. Nie and J. Sun, ACS Nano, 2017, 11, 6968–6976 CrossRef CAS PubMed.
- B. H. Wunsch, J. T. Smith, S. M. Gifford, C. Wang, M. Brink, R. L. Bruce, R. H. Austin, G. Stolovitzky and Y. Astier, Nat. Nanotechnol., 2016, 11, 936–940 CrossRef CAS PubMed.
- S. Kondapalli, J. T. Connelly, A. J. Baeumner and B. J. Kirby, Microfluid. Nanofluid., 2011, 11, 537–544 CrossRef CAS.
- S. J. Lee, H. Rhee, T. J. Jeon and D. Kim, Sens. Actuators, B, 2016, 229, 276–280 CrossRef CAS.
- L. S. Cheung, S. Sahloul, A. Orozaliev and Y.-A. Song, Micromachines, 2018, 9(6), 306–318 CrossRef PubMed.
- S. Marczak, K. Richards, Z. Ramshani, E. Smith, S. Senapati, R. Hill, D. B. Go and H. C. Chang, Electrophoresis, 2018, 39, 2029–2038 CrossRef CAS PubMed.
- G. Birnbaumer, S. Küpcü, C. Jungreuthmayer, L. Richter, K. Vorauer-Uhl, A. Wagner, C. Valenta, U. Sleytr and P. Ertl, Lab Chip, 2011, 11, 2753–2762 RSC.
- G. Tarabella, A. G. Balducci, N. Coppedè, S. Marasso, P. D'angelo, S. Barbieri, M. Cocuzza, P. Colombo, F. Sonvico, R. Mosca and S. Iannotta, Biochim. Biophys. Acta, 2013, 1839, 4374–4380 CrossRef PubMed.
- M. Michelon, D. R. B. Oliveira, G. D. Furtado, L. G. de la Torre and R. L. Cunha, Colloids Surf., B, 2017, 156, 349–357 CrossRef CAS PubMed.
- H. Shan, Q. Lin, D. Wang, X. Sun, B. Quan, X. Chen and Z. Chen, Front. Bioeng. Biotechnol., 2021, 9, 773705 CrossRef PubMed.
- D. Carugo, E. Bottaro, J. Owen, E. Stride and C. Nastruzzi, Sci. Rep., 2016, 6, 1–15 CrossRef PubMed.
- M. Maeki, Y. Okada, S. Uno, K. Sugiura, Y. Suzuki, K. Okuda, Y. Sato, M. Ando, H. Yamazaki, M. Takeuchi, A. Ishida, H. Tani, H. Harashima and M. Tokeshi, Appl. Mater. Today, 2023, 31, 101754 CrossRef.
- S. J. Shepherd, C. C. Warzecha, S. Yadavali, R. El-Mayta, M.-G. Alameh, L. Wang, D. Weissman, J. M. Wilson, D. Issadore and M. J. Mitchell, Nano Lett., 2021, 21, 5671–5680 CrossRef CAS PubMed.
- S. J. Shepherd, X. Han, A. J. Mukalel, R. El-Mayta, A. S. Thatte, J. Wu, M. S. Padilla, M. G. Alameh, N. Srikumar, D. Lee, D. Weissman, D. Issadore and M. J. Mitchell, Proc. Natl. Acad. Sci. U. S. A., 2023, 120, e2303567120 CrossRef CAS PubMed.
- A. Bertsch, S. Heimgartner, P. Cousseau and P. Renaud, Lab Chip, 2001, 1(1), 56–60 RSC.
- R. Su, F. Wang and M. C. McAlpine, Lab Chip, 2023, 23, 1279–1299 RSC.
- A. V. Nielsen, M. J. Beauchamp, G. P. Nordin and A. T. Woolley, Annu. Rev. Anal. Chem., 2020, 13, 45–65 CrossRef PubMed.
- H. Gong, B. P. Bickham, A. T. Woolley and G. P. Nordin, Lab Chip, 2017, 17, 2899–2909 RSC.
- H. Gong, A. T. Woolley and G. P. Nordin, Lab Chip, 2016, 16, 2450–2458 RSC.
- Z. Huang, G. Chi-Pong Tsui, Y. Deng and C. Y. Tang, Nanotechnol. Rev., 2020, 9, 1118–1136 CrossRef CAS.
- A. J. G. Otuka, N. B. Tomazio, K. T. Paula and C. R. Mendonça, Polymers, 2021, 13, 1994 CrossRef CAS PubMed.
- J. Y. Han, S. Warshawsky and D. L. DeVoe, Sci. Rep., 2021, 11, 10980 CrossRef CAS PubMed.
- A. T. Alsharhan, R. Acevedo, R. Warren and R. D. Sochol, Lab Chip, 2019, 19, 2799–2810 RSC.
- G. Ballacchino, E. Weaver, E. Mathew, R. Dorati, I. Genta, B. Conti and D. A. Lamprou, Int. J. Mol. Sci., 2021, 22, 8064 CrossRef CAS PubMed.
- F. Sommonte, E. Weaver, E. Mathew, N. Denora and D. A. Lamprou, Pharmaceutics, 2022, 14, 2484 CrossRef CAS PubMed.
- E. Weaver, E. Mathew, J. Caldwell, A. Hooker, S. Uddin and D. A. Lamprou, J. Pharm. Pharmacol., 2023, 75, 245–252 CrossRef PubMed.
- M. Tiboni, M. Tiboni, A. Pierro, M. Del Papa, S. Sparaventi, M. Cespi and L. Casettari, Int. J. Pharm., 2021, 599, 120464 CrossRef CAS PubMed.
- M. Tiboni, S. Benedetti, A. Skouras, G. Curzi, D. R. Perinelli, G. F. Palmieri and L. Casettari, Int. J. Pharm., 2020, 584, 119436 CrossRef CAS PubMed.
- H. Shan, Q. Lin, D. Wang, X. Sun, B. Quan, X. Chen and Z. Chen, Front. Bioeng. Biotechnol., 2021, 9, 773705 CrossRef PubMed.
- M. Walpitagama, M. Carve, A. M. Douek, C. Trestrail, Y. Bai, J. Kaslin and D. Wlodkowic, Aquat. Toxicol., 2019, 213, 105227 CrossRef CAS PubMed.
- J. E. Krechmer, B. Phillips, N. Chaloux, R. Shomberg, C. Daube, G. Manchanda, S. Murray, A. McCarthy, R. Fonseca, J. Thakkar, B. Loose, S. C. Herndon, J. T. Jayne, D. R. Worsnop and M. R. Canagaratna, ACS Omega, 2021, 6, 30726–30733 CrossRef CAS PubMed.
- G. R. Warner and J. A. Flaws, Toxicol. Sci., 2018, 166, 246–249 CrossRef CAS PubMed.
- D. Jenke, J. Pharm. Sci., 2007, 96, 2566–2581 CrossRef CAS PubMed.
- I. K. Cingesar, M. P. Marković and D. Vrsaljko, Addit. Manuf., 2022, 55, 102813 CAS.
|
This journal is © The Royal Society of Chemistry 2024 |
Click here to see how this site uses Cookies. View our privacy policy here.