Case study evaluation of size-resolved molecular composition and phase state of carbonaceous particles in wildfire influenced smoke from the Pacific Northwest†
Received
20th April 2023
, Accepted 28th July 2023
First published on 9th August 2023
Abstract
Wildfires are significant sources of carbonaceous particles in the atmosphere. Given the dependence of atmospheric processes on particle physical and molecular properties, the interplay between particle size, phase state and chemical composition is investigated here for aerosol influenced by a 2021 Pacific Northwest wildfire event. Both micro-spectroscopy and high resolution mass spectrometry analyses highlight a similarity in particle compositions independent of both particle size (0.1–0.32 μm particle diameters) and day/night cycle influences. Microscopy techniques revealed similar phase states for periods of both day and night, with increases in liquid-like character for smaller particles. Finally, we apply an evaporation kinetics model on estimated volatility distributions from assigned molecular formulae, similarly revealing a slight increase in liquid-like character for smaller particles with no significant day/night dependency. While the observations here are limited to a case study, the lack of influence from the day/night cycle on chemical composition and phase state of particles in a wildfire influenced plume is of particular note given that dependences are otherwise commonly observed for different environments/sources. This observation, combined with the lack of compositional dependencies for size-resolved wildfire-influenced particles, may have substantial implications for wildfire particle optical properties, transport, and atmospheric models.
Environmental significance
Wildfires are a key source of atmospheric particles. As climate implications may vary significantly depending on the particles' composition and morphology, the detailed interrogation of wildfire-influence particles is warranted. Here, we find that the characteristics of particles from a wildfire-influenced event in the Pacific Northwest do not appear to have significant dependencies upon the day/night cycle. Furthermore, the composition of these particles appears unrelated to their size. Conventionally, dependencies to these variables have been observed for atmospheric particles from different sites and sources. While the observations contained herein are limited to a case study, they present substantial implications for wildfire influence particles. In particular, this study shows that the atmospheric evolution of wildfire-influence particles may differ substantially from other types of atmospheric particles.
|
1 Introduction
Wildfires are significant sources of carbonaceous particles with substantial environmental impacts, including implications on radiative forcing.1–3 With respect to quantifying atmospheric emissions, a previous study estimated that 33.9 Tg per year of organic carbon are emitted globally, with open burning responsible for 74% of the atmospheric organic carbon mass.4 However, there is a limited understanding of the current particle emissions that are directly related to wildfire events and how the emitted particles evolve over time in the atmosphere.4–6 Additionally, recent studies have suggested that wildfire-related emissions have increased and are expected to continue doing so, driven by changing climactic factors such as warming temperatures, reduced snow-pack and evolving anthropogenic activities.7–10 Given these uncertainties, and that atmospheric aerosol loading has been identified as a ‘planetary boundary’ for continued health of Earth's multifaceted systems,11 continued interrogation of wildfire atmospheric aerosol composition is warranted.
While aerosol emitted into the atmosphere (including those from wildfires) may affect climate in a variety of ways, many of these processes are significantly influenced by particle physical properties, such as size and phase state. For example, the cloud-forming properties of cloud condensation nuclei (CCN) are heavily dependent upon particle size,12,13 and more solid-like particles will be transported differently than liquid-like counterparts.1 Therefore, given the importance of aerosol particle physical properties, many research efforts have targeted a further understanding of the interplay between aerosol size, phase state, and chemical composition14–21 with some specifically focusing on the chemical composition of size-resolved biomass burning (including wildfires).22–27 As examples, size-dependent aerosol mixing states have been observed from biomass burning events in China,25,26 and brown carbon was found to have a size-dependence in wildfire specific plumes in Northeast Canada.23 Size-resolved secondary organic aerosol (SOA) composition and evolution in wildfire aerosols remains as a significant knowledge gap though, particularly with respect to how molecular level information influences particle physical properties. It is furthermore necessary to appreciate the potential for wildfire derived particle composition and physical properties to be influenced by the day/night cycle. While Slade et al. have shown an increase in solid-like phase characteristics at night for biogenic aerosol,28 it remains unclear how the differences in day/night atmospheric conditions affect the size-resolved phase state and chemical composition of specifically wildfire derived particles.
Here, we investigate the molecular-level composition of size-resolved atmospheric aerosol that was influenced by 2021 wildfire events in the Pacific Northwest. Specifically, we use high resolution mass spectrometry,29,30 an array of microscopy and spectroscopy tools, and auxiliary in situ aerosol measurements to examine the connection between particle size, chemical composition, and phase state of wildfire-influenced atmospheric particles for periods of both day and nighttime.
2 Materials and methods
2.1 Particle collection and auxiliary aerosol measurements
Size-resolved aerosol particles (stage 7 (0.32–0.56 μm), stage 8 (0.18–0.32 μm), stage 9 (0.10–0.18 μm)) were collected in Richland, WA via a Micro-Orifice Uniform Deposition Impactors (MOUDI; Model 110-R, MSP, Inc.) during periods of day (August 2, 2021) and night (August 3, 2021) as described in the ESI (Table S1).† HYSPLIT back trajectory frequencies are shown in Fig. S1.† Air quality index contours for every two days ranging from July 23 to August 8 are shown in Fig. S2.† Particle electrical-mobility size distribution from scanning mobility particle sizer (SMPS) and columnar volume particles size distribution from AErosol RObotic NETwork (AERONET) Version 3.0 algorithm products at quality level 1.5 are described in the ESI (Fig. S3 and S4).† The SMPS data (Fig. S3†) was used to inform which particle diameters (i.e., stages) were representative of the sampling period, justifying the analyses of MOUDI stages 7–9. Monthlong (August) volume particles size distribution and aerosol optical depth Ångström exponent data from AERONET are shown in Fig. S4.† The average total particle concentrations for day and nighttime sampling periods were comparable (∼2200 particles per cm3 and ∼1700 particles per cm3, respectively).
2.2 Microspectroscopic analysis of individual particles
Environmental scanning electron microscopy (ESEM; Quanta 3D, Thermo Fisher) with a FEI Quanta digital field emission gun (20 kV and 480 pA) and a tilted stage (75°) was used to probe the phase state via the aspect ratio measurements (particle width to particle height ratio) of individual impacted particles. While some of the experimental conditions used here may bias the end measurements against water content and volatile–semivolatile species (e.g., analyses are conducted at 293 K and ∼2 × 10−6 torr), this technique has been previously validated for phase-state measurements. Additionally, the strategy used herein was importantly found to not be biased by particle size for the investigated particle size ranges here.31,32 Further description of these limitations and experimental parameters are described elsewhere31–33 and in the ESI.†
Computer-controlled scanning electron microscopy with energy-dispersive X-ray spectroscopy (CCSEM-EDX) and scanning transmission X-ray microscopy with near-edge X-ray absorption fine structure spectroscopy (STXM-NEXAFS) were used to characterize the chemical composition of wildfire aerosol particles via methods similarly described elsewhere.33–35 For CCSEM-EDX, an EDX spectrometer (EDAX, Inc.) was used to collect spectra for 10
176 total particles across all samples. Using K-mean clustering (elbow method),36 particles were classified based on their elemental percentages of C, O, N, S, Na, Mg, Al, Si, P, Cl, K, Ca, Zn, Mn, and Fe. A k-mean clustering algorithm was used to guide the number of particle classes, and a rule-based algorithm (Fig. S5†) was eventually used to finally sort particles into 5 different clusters: carbonaceous (CNO), carbonaceous sulfur (CNOS), Na-rich particles and dust particles. STXM-NEXAFS analyses were conducted at beamline 5.3.2.2 of the Advanced Light Source (ALS) at the Lawrence Berkeley National Laboratory.32,33,37,38 The chemical composition and mixing states of each particle were then inferred from the spatially resolved X-ray spectra. Particles were classified into three different categories: ‘OC’ (dominant and homogeneous distribution of organic carbon), ‘EC’ (elemental carbon; particles containing organic function groups and higher amount of sp2 C
C hybridized bonds), and ‘IN’ (inorganic contributions).38–40 STXM-NEXAFS was used to infer particle phase state,38,41–43 and also used for spectral deconvolution analysis to infer functional group contribution44,45 (Fig. S6 and Table S2) as further described in the ESI.†
2.3 Nanospray desorption electrospray ionization with 21 tesla Fourier ion cyclotron resonance mass spectrometry (nano-DESI 21 T FTICR MS)
A similar nanospray desorption electrospray ionization (nano-DESI) system coupled to the 21 T FTICR MS system to the one employed here has been previously described.30 Full scan analyses were conducted over the range m/z 150–700 in negative ion mode at a mass resolving power of 600k (at m/z 400). Similar strategies to those used here for data analysis, including blank subtraction and data filtering, have been previously described.46 Molecular formulae assignment was conducted using MFAssignR,47 an open source software tool. Assigned molecular formula were limited to C, H, N (maximum of 2 per individual formula) and O elemental constituents of deprotonated, singly-charged ion types. Sulfur constituents were found to not contribute to the plumes in this study as part of preliminary analyses, and sulfur was therefore not considered in final compositional analyses. For all samples, 77–82% of mass spectral features were assigned a molecular formula for the given restrictions. Using the assigned molecular formulae, volatilities were parametrized according to the methods developed by Li et al.48,49 Data for m/z less than 150 was not collected due to low mass transmission limitations of the 21 T FTICR MS.29 To specifically evaluate levoglucosan (C6H10O5) signal intensities (a lower m/z ion not well transmitted by the 21 T FTICR MS system), a LTQ Velos Orbitrap mass spectrometer (Thermo Scientific, Waltham) was used with identical scan and nano-DESI parameters with the exception of lower mass resolution (100k at m/z 400). Further details are available in the ESI.†
2.4 Evaporation kinetics modelling
Time dependent evaporation of multicomponent SOA particles was modelled using the parametrized volatility data from the nano-DESI 21 T FTICR MS data. The dynamic gas-particle partitioning properties of wildfire-influenced OA within a simulated organic gas-free environment at room temperature are represented by a 14-bin volatility basis sets with saturation mass concentrations (C*) ranging from 10−9 to 104 μg m−3. The initial particle diameter corresponding to each of the three impactor stages (7, 8, and 9) and corresponding normalized mass fractions estimated from weighted mass spectrometry signal intensities were used within the kinetic particle evaporation model, and are shown in Table S3.† Further modeling details are available in the ESI (Tables S3, S4 and Fig. S7)† and in Vaden et al.50
3 Results and discussion
3.1 Observations from auxiliary datasets
As shown in Fig. S3,† the size mode at the time of sample collection was around 0.25 μm, which is larger than the typical size modes of freshly emitted wildfire particles (otherwise around 0.1 μm).51–53 These large particles may therefore be products of long-range transport and/or secondary formation.54,55 Correlations between HYSPLIT back trajectory frequency matrices (72 hours),VIIRS fire map data (Fig. S1†) and air quality index contours (Fig. S2†) show that the plumes studied herein have some degree of influence from several wildfire events. The wildfire-influence is further supported by the observation of high signal intensities for levoglucosan (C6H10O5; m/z 161.0455) in high resolution mass spectrometry datasets across all samples (0.11–0.46 normalized signal intensity range; Fig. S1†). Both the molecular composition and subsequent connection to particles phase state are explored in detail next.
3.2 Size-resolved composition of wildfire-influenced particles
The composition of individual particles as revealed through STXM-NEXAFS analyses is shown in Fig. 1, S5 and S7.† Across all stages, the particles observed here are dominated by OC and EC. Specifically, the percentage of particles classified as OC ranged from 84% (daytime, stage 9) to 98% (daytime, stage 7) for both day and nighttime particles, with the remaining particles in these states classified as EC. Relative contributions of organic functional groups may be inferred for the organic (OC) portion of the aerosol (Fig. S6 and Table S2†) and are summarized here in Fig. 1 and Table S5.† Both the particle classifications and relative abundances of different organic functional groups as revealed by the spectral deconvolution of the STXM-NEXAFS data are generally consistent across stages, as well as between day and nighttime periods.
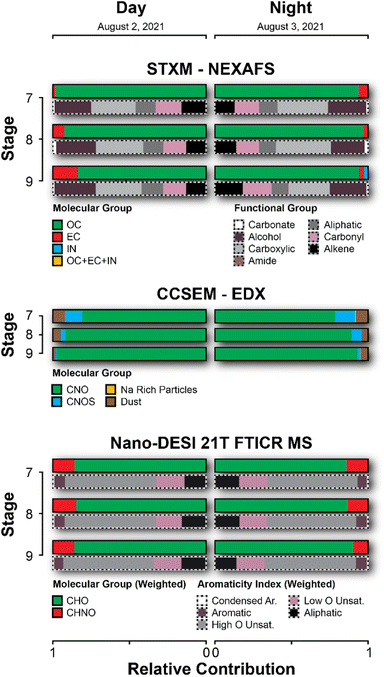 |
| Fig. 1 Summary of chemical composition data for wildfire aerosol (stages 7 through 9) from periods of both day and nighttime. Represented data is the result of separate analyses by scanning transmission X-ray microscopy with near-edge X-ray absorption fine structure spectroscopy (STXM-NEXAFS), computer-controlled scanning electron microscopy with energy-dispersive X-ray spectroscopy (CCSEM-EDX), and nanospray desorption electrospray ionization with 21 tesla Fourier transform ion cyclotron resonance mass spectrometry (nano-DESI 21 T FTICR MS). All represented data is normalized to totals per stage (i.e., normalized per sample). Nano-DESI 21 T FTICR MS datasets are weighted according to signal intensity. | |
Fig. 1 additionally provides per-particle level characterizations by CCSEM-EDX (Table S6†). For stages 7 through 9, the CCSEM-EDX data is likewise suggestive of a significant carbonaceous (organic) contribution, with a proportion of carbon, nitrogen and oxygen containing particles (CNO) that is dominant across all stages (Table S6†). There again is a high degree of similarity in particle composition for periods of both day and nighttime, which is reinforced by comparable results from the STXM-NEXAFS dataset. The observed lack of dependence on day and night chemistries is again notable, as nighttime nitrate oxidation typically results in varied composition, and consequently, distinct physical characteristic consequences for atmospheric particles.1,56,57
To further validate the consistent compositional results from microscopy, nano-DESI MS was used to assess the molecular formulae of the bulk organic aerosol in each stage. Specifically, a 21 T FTICR MS system was used for relatively increased confidence in the observed molecular formulae due to the elevated mass resolving power.30,58 It must be noted that the nano-DESI 21 T FTICR MS methodology with the employed data analysis strategy is limited to the interpretation of solubilized organics, is not quantitative,59 and does not allow for inferences regarding connectivity of atoms (i.e., isomers are not separated).60 However, similar chemical composition was again noted across all stages for the employed experimental conditions as assessed via the both the weighted signal intensities (Fig. 1) and number of assigned molecular formulae (Table S7†). The weighted contributions of species (according to signal intensity) that contained carbon, hydrogen, and oxygen (‘CHO’) ranged from 85 to 91% and ‘CHNO’ species (additional inclusion of nitrogen) comprised 9–15% of the total weighted abundance observed in both day and nighttime samples. Aromaticity indexes,61,62 which were calculated according to the assigned molecular formulae, were also similar across all stages and between day and nighttime sampling periods (Fig. 1 and Table S7†). Notably, elevated O/C ratios (average of 0.6 for all stages) and dominance of ‘high O unsaturated’ compounds (52–56% of all molecular formulae) were observed from the nanoDESI MS data (Table S7†). Brege et al. have previously observed an increase in O/C ratios from fresh (0.43–0.48) to aged (0.50–0.58) biomass burning aerosol in Italy.63 A separate study from Brege et al. highlighted lower average O/C ratios (<0.4) in a tar ball dominated plume from Richland, WA (same sampling location as this study). While different fuel characteristics (e.g., moisture content) and burning conditions (e.g., temperature) will assuredly impact particle characteristics, the comparatively high O/C ratios observed here (0.6) may nonetheless be suggestive of atmospheric aging and is in accordance with the observed large particle size distribution (Fig. S3†).1,63,64
3.3 Further assessment of wildfire-influenced particle composition via mass spectrometry
All observed molecular formulae via MS were aligned into one dataset next to assess if any of the observed molecular formulae are unique to a particular particle size range (i.e., impactor stage). Parametrized volatility49 was then plotted against the normalized signal intensity of each detected molecular formula for day and nighttime periods as shown in Fig. 2 to create pseudo-mass spectra. Inset ‘UpSet’ plots65 detail the intersections observed between stages according to the weighted abundances of unique/commonly detected molecular formulae. The data intersections as visualized via color-coding in the Fig. 2 pseudo-mass spectra appear highly stratified, with the intersections ‘common to stages 7, 8, and 9’ featuring the most intense signals for both day and night samples; all other intersections (e.g., unique to stages 8 and 9) featured significantly lower comparative abundances. While some of these lower signal intensities may be due to unique molecular formulae, the comparative nature of these low intensities may instead be suggestive of a concentration effect (related to sample loading) to explain the unique features across stages. In addition to similarity across stages, the pie chart shown in Fig. 2 reinforces the similarities between day and nighttime samples: molecular formulae commonly detected between both periods comprise 98% of the overall signal intensity weighted abundance between both sample sets. Fig. 2 therefore provides further evidence of the chemical similarity for dominant spectral features across periods of day and nighttime for this wildfire-influenced aerosol, as well as across stages.
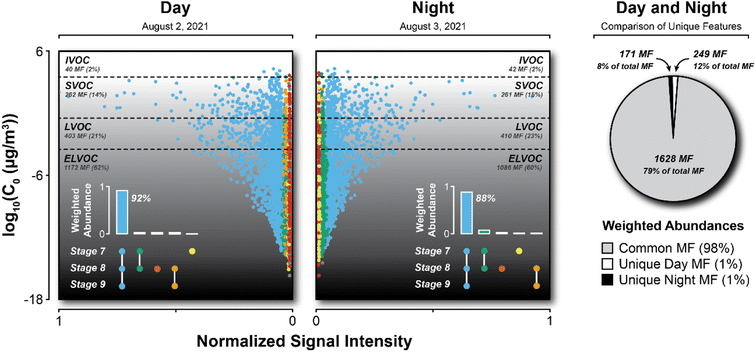 |
| Fig. 2 Parametrized volatility plotted against the normalized signal intensity for each assigned molecular formula from nanospray desorption electrospray ionization 21 tesla Fourier transform ion cyclotron resonance mass spectrometry (nano-DESI 21 T FTICR MS) analysis. Inset ‘UpSet’ plots show the degree of similarity across stages according to the weighted signal intensities of assigned molecular formulae for periods of both day and night, and the mass spectra are color coded accordingly. Sample/stage intersections that represented <0.75% of the weighted abundance (e.g., unique stage 9 species) are not shown. For inset pie chart, all stages were aligned for respective periods of day and night, creating two datasets for comparison/assessment of unique day/night features according to weighted abundance. | |
Combined with the results shown in Fig. 1, it is possible that size-resolved compositional differences may be observed for fresher biomass burning particles or for particles with a greater overall wildfire influence.66–68 However, the combined results here from micro-spectroscopy (CCSEM-EDX, STXM-NEXAFS) and mass spectrometry (nano-DESI MS) techniques suggest that these plumes do in fact show evidence of wildfire influence (e.g., elevated levoglucosan signal intensities from MS data) while also not having significant compositional differences according to either particle size or to day/nighttime dependencies.
3.4 Connection of wildfire-influenced particle composition to phase state via microscopy
The phase state of these wildfire influenced particles was explored next to explore a potential link between particle composition and physical properties. Fig. 3 shows the phase state distribution and representative tilted view images of particles for periods of both day and nighttime sample collection as measured by SEM. Consistent with the data from Fig. S3,† the highest particle loading is observed for stage 8. Tar balls, which are spherical particles often observed with organic dominated wildfire smoke (as is the case in this study) especially in the smoldering phase of fire,6,69 were not highly abundant as part of this plume. However, they were still observed in stage 7 (21% and 29% of day and nighttime particles, respectively) as further detailed in Table S5.†
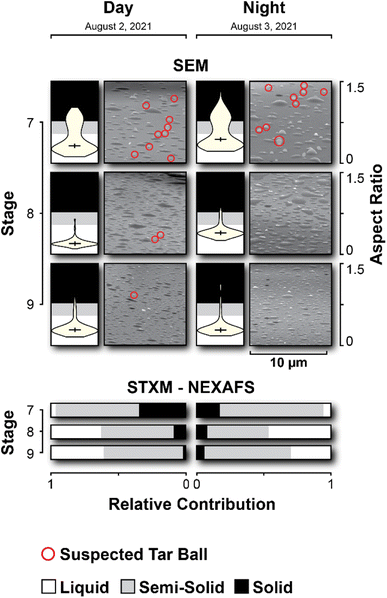 |
| Fig. 3 Multimodal evaluation of size and day/night resolved wildfire particle phase states. Scanning electron microscopic (SEM) images of size-resolved wildfire aerosol are from a tilted stage (75°). Inset violin plots describe the phase state distributions as calculated via the aspect ratio of impacted aerosol particles. Suspected tar balls (i.e., solid-like particles) are indicated via dashed red circles (note that inset pictures are not representative of all particles studied per sample). Data from STXM-NEXAFS is calculated on a per-particle basis. | |
For these suspected aged particles, the violin plots in Fig. 3 show a clear increase in liquid-like character for particles collected on the stages for smaller particle sizes. Stage 7 exhibits some solid phase influence likely due to a limited number of tar ball-like particles, while stages 8 and 9 exhibit similar liquid-like phase state distributions. Even though some liquid representation may be exaggerated due to particle coalescence in stages 8 and 9 (due to high overall sample loading), the trend of increasing liquid-like character for smaller particles is nonetheless evident. To further investigate particle phase state via STXM-NEXAFS, total carbon absorbance was plotted against particle diameter for individual particles (both as measured by STXM-NEXAFS; Fig. S9†), allowing each particle to be classified as either liquid, semi-solid, or solid.41,42 As summarized in Fig. 3, the same trend towards more liquid-like particles first shown in the SEM dataset is also observed here for increasingly smaller particle sizes. Only a slight increase in solid-like character is observed for the nighttime period relative to the day in the SEM dataset, whereas the phase states as described by STXM-NEXAFS have minimal day/night dependencies. While this is consistent in principle with observations from Slade et al. for a forested environment (i.e., more solid-like particles at night),28 the significantly lessened differences observed here for aged wildfire-influenced particles are consistent with the minor differences observed for day/night particle composition. This observation (i.e., dominance of liquid-like particles in biomass burning) was similarly observed by Liu et al. (not strictly related to wildfire burning events), where they also establish that numerous chamber-related studies have underestimated the liquid character of true, ambient biomass burning particles.27
3.5 Modelling of time-dependent secondary organic aerosol evaporation
While similar SOA molecular formulae are noted for both size-resolved and day/night wildfire-influenced aerosol, the composition of phase state of atmospheric particles may also change over time. Evaporation kinetics, which are dependent upon the volatility distributions (determined from their mass spectra) and particle size,49 were therefore modelled next as one type of approach to observe this evolution. The box model applied herein is previously described by Vaden et al., which simulates SOA evaporation of particles in an evaporation chamber lined with activated charcoal at room temperature.50Fig. 4 shows the evaporation kinetics over 24 hours for size-resolved particles during periods of day and night, revealing that smaller particles evaporate at a faster rate. This is supported by Table S4† which shows an increase in the weighted MS volatilities for the smaller particles and is consistent with more liquid-like properties of smaller wildfire particles as revealed through SEM and STXM-NEXAFS (Fig. 3). The trend was largely conserved when the model was re-run with the mean size-resolved volatility distribution for each period of day and night (i.e., day run with mean distribution of stages 7–9 from daytime, night run with mean distribution of stages 7–9 from nighttime). This finding therefore reinforces the compositional similarities between day and night, and that evaporation here is mostly driven by particle size.
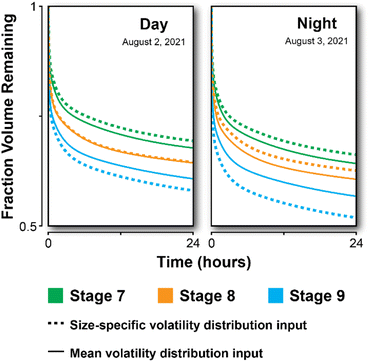 |
| Fig. 4 Simulated evaporation kinetics for size-resolved wildfire organic aerosol using mass spectrometry signal intensity weighted volatility distribution data inputs. | |
4 Conclusions
Overall, through the combination of microscopy, spectroscopy and high-resolution mass spectrometry analyses, the composition of wildfire-influenced carbonaceous particles was found to be largely independent of particle size for this case study. While smaller particles were found to have increased liquid-like character, these phase state differences were found to not have a significant association with the molecular composition of the particle itself. Furthermore, both the particle phase state and composition for this wildfire-influenced plume were found to be largely independent of day/night variations. The dominance of liquid-like character for these particles is particularly interesting, as previous studies of Pacific Northwest wildfires have conversely shown that up to 95% of atmospheric particles were tar balls (solid-like).70 This finding therefore reinforces that varying combustion and fuel conditions of fire may have substantive impacts on eventual tar ball formation. Subsequent aging/atmospheric transport of wildfire derived particles will consequently be impacted; for example, a recent study demonstrated that tar ball formation allowed for substantive amounts of brown carbon to be transported to remote Himalayan regions, resulting in a heating effect in the Himalayan atmosphere.71
This case study also suggests that the molecular composition of larger particles (observed in stage 7), which constitute relatively higher number fractions of viscous tar balls compared to smaller particles (stages 8 and 9), may not always be appreciably different from more liquid-like wildfire particle counterparts; this inference is supported by the overall lack of size-resolved molecular composition dependence for wildfire-influenced particles demonstrated here. As suggested by Pósfai et al., tar balls (formed under the smoldering phase of wildfire) do not experience significant physical growth upon atmospheric aging and eventually may dissolve upon extended atmospheric aging due to interaction with other water-bearing particles.72 This narrative, along with the elevated O/C ratios, may potentially describe the atmospheric evolution of wildfire-influenced particles observed in this study.
The presence of liquid-like phase states in atmospherically aged wildfire-influenced particles warrants specific investigation in the future and has significant implications, as atmospheric particles are typically thought of and modelled to become more solid-like (or glassy) with time after interaction with sunlight.73,74 However, this case study of wildfire-influenced particles presents an ‘outlier’ case from this generalization due to the combined observations of liquid-like phase states and elevated O/C ratios (i.e., suggestive of atmospheric aging). Particle optical properties, diffusion rates (faster for more liquid-like particles), and atmospheric transport (greater transport observed for more solid-like particles) may therefore all be affected. It should also be reiterated that while consistent molecular formulae were observed here across particle sizes and day/night, it is possible that molecular-level information that was unobservable here (e.g., quantitative abundances, SOA isomer speciation) may vary across particle size and collection time. While it may not be mechanistically investigated with the presented data, the highly specific examination of wildfire derived SOA evolution over time will also be evaluated in future. As the observations described herein are limited to a case study, they should not be considered as a universal description of wildfire-influenced particles. However, the depth of multimodal analyses employed here for these select samples nevertheless lends a high degree of confidence for the particular events investigated here, with many significant implications as described.
In general, the importance of understanding wildfire-derived particles is rapidly growing alongside the escalating climate crisis.75 To exemplify the significance of this topic, a recent study by Damany-Pearce et al. showcased that the largest stratospheric warming event since the eruption of Mount Pinatubo in 1991 was due to the 2019–2020 eastern Australia wildfires.76 Therefore, as the contribution of wildfire particles to the total atmospheric particle load increases, so do the implications for proper characterization and subsequent incorporation of wildfire particles in climactic models. As a result, the continued investigation of wildfire aerosol over more events, distinct geographical locals, and greater timescales is needed.
Author contributions
Conceptualization: G. V. and S. C. Formal analysis: G. V., N. N. L., S. M., Z. C., and J. Z. Investigation: G. V., N. N. L., S. M., A. I., Z. C., and W. K. Methodology: all authors. Resources: A. S., Z. C., and G. K. Validation: S. C., L. M., and M. S. Visualization: G. V., N. N. L., S. M., and Z. C. Writing-original draft: G. V. Writing-review and editing: all authors.
Conflicts of interest
The authors declare no conflicts of interest.
Acknowledgements
A portion of this research was performed on a project award (https://doi.org/10.46936/lser.proj.2020.51355/60000178) from the Environmental Molecular Sciences Laboratory, a DOE Office of Science User Facility sponsored by the Biological and Environmental Research (BER) program under contract no. DE-AC05-76RL01830. We acknowledge funding from the U.S. Department of Energy, Office of Science, Office of BER, Atmospheric System Research. M. S. and J. Z. acknowledge support from the DOE Office of Science and the Office of BER Early Career Research Program. STXM-NEXAFS analysis at beamline 5.3.2 of the Advanced Light Source at Lawrence Berkeley National Laboratory is supported by the Director, Office of Science, Office of Basic Energy Sciences of the U.S. Department of Energy under contract no. DE-AC02-05CH11231.
References
- M. Shrivastava, C. D. Cappa, J. Fan, A. H. Goldstein, A. B. Guenther, J. L. Jimenez, C. Kuang, A. Laskin, S. T. Martin, N. L. Ng, T. Petaja, J. R. Pierce, P. J. Rasch, P. Roldin, J. H. Seinfeld, J. Shilling, J. N. Smith, J. A. Thornton, R. Volkamer, J. Wang, D. R. Worsnop, R. A. Zaveri, A. Zelenyuk and Q. Zhang, Recent Advances in Understanding Secondary Organic Aerosol: Implications for Global Climate Forcing, Rev. Geophys., 2017, 55(2), 509–559, DOI:10.1002/2016RG000540.
- J. Chen, C. Li, Z. Ristovski, A. Milic, Y. Gu, M. S. Islam, S. Wang, J. Hao, H. Zhang, C. He, H. Guo, H. Fu, B. Miljevic, L. Morawska, P. Thai, Y. F. Lam, G. Pereira, A. Ding, X. Huang and U. C. Dumka, A Review of Biomass Burning: Emissions and Impacts on Air Quality, Health and Climate in China, Sci. Total Environ., 2017, 579, 1000–1034, DOI:10.1016/j.scitotenv.2016.11.025.
-
J. Seinfeld and P. Spyros, Atmospheric Chemistry and Physics: From Air Pollution to Climate Change, John Wiley & Sons, Hoboken, NJ, USA, 3rd edn, 2016 Search PubMed.
- T. C. Bond, D. G. Streets, K. F. Yarber, S. M. Nelson, J. H. Woo and Z. Klimont, A Technology-Based Global Inventory of Black and Organic Carbon Emissions from Combustion, J. Geophys. Res. Atmos., 2004, 109(D14), D14203, DOI:10.1029/2003JD003697.
- T. C. Bond, S. J. Doherty, D. W. Fahey, P. M. Forster, T. Berntsen, B. J. DeAngelo, M. G. Flanner, S. Ghan, B. Kärcher, D. Koch, S. Kinne, Y. Kondo, P. K. Quinn, M. C. Sarofim, M. G. Schultz, M. Schulz, C. Venkataraman, H. Zhang, S. Zhang, N. Bellouin, S. K. Guttikunda, P. K. Hopke, M. Z. Jacobson, J. W. Kaiser, Z. Klimont, U. Lohmann, J. P. Schwarz, D. Shindell, T. Storelvmo, S. G. Warren and C. S. Zender, Bounding the Role of Black Carbon in the Climate System: A Scientific Assessment, J. Geophys. Res. Atmos., 2013, 118(11), 5380–5552, DOI:10.1002/jgrd.50171.
- S. China, C. Mazzoleni, K. Gorkowski, A. C. Aiken and M. K. Dubey, Morphology and Mixing State of Individual Freshly Emitted Wildfire Carbonaceous Particles, Nat. Commun., 2013, 4(1), 2122, DOI:10.1038/ncomms3122.
- A. L. Westerling, H. G. Hidalgo, D. R. Cayan and T. W. Swetnam, Warming and Earlier Spring Increase Western U.S. Forest Wildfire Activity, Science, 2006, 313(5789), 940–943, DOI:10.1126/science.1128834.
- A. P. Williams, J. T. Abatzoglou, A. Gershunov, J. Guzman-Morales, D. A. Bishop, J. K. Balch and D. P. Lettenmaier, Observed Impacts of Anthropogenic Climate Change on Wildfire in California, Earth's Future, 2019, 7(8), 892–910, DOI:10.1029/2019EF001210.
- T. Kitzberger, D. A. Falk, A. L. Westerling and T. W. Swetnam, Direct and Indirect Climate Controls Predict Heterogeneous Early-Mid 21st Century Wildfire Burned Area across Western and Boreal North America, PLoS One, 2017, 12(12), e0188486 CrossRef PubMed.
- T. M. Ellis, D. M. J. S. Bowman, P. Jain, M. D. Flannigan and G. J. Williamson, Global Increase in Wildfire Risk Due to Climate-Driven Declines in Fuel Moisture, Glob.
Change Biol., 2022, 28(4), 1544–1559, DOI:10.1111/gcb.16006.
- J. Rockström, W. Steffen, K. Noone, Å. Persson, F. S. Chapin, E. F. Lambin, T. M. Lenton, M. Scheffer, C. Folke, H. J. Schellnhuber, B. Nykvist, C. A. de Wit, T. Hughes, S. van der Leeuw, H. Rodhe, S. Sörlin, P. K. Snyder, R. Costanza, U. Svedin, M. Falkenmark, L. Karlberg, R. W. Corell, V. J. Fabry, J. Hansen, B. Walker, D. Liverman, K. Richardson, P. Crutzen and J. A. Foley, A Safe Operation Space for Humanity, Nature, 2009, 461(September), 472–475 CrossRef PubMed.
- U. Dusek, G. P. Frank, L. Hildebrandt, J. Curtius, J. Schneider, S. Walter, D. Chand, F. Drewnick, S. Hings, D. Jung, S. Borrmann and M. O. Andreae, Size Matters More Than Chemistry for Cloud-Nucleating Ability of Aerosol Particles, Science, 2006, 312(5778), 1375–1378, DOI:10.1126/science.1125261.
- J. H. Seinfeld, C. Bretherton, K. S. Carslaw, H. Coe, P. J. DeMott, E. J. Dunlea, G. Feingold, S. Ghan, A. B. Guenther, R. Kahn, I. Kraucunas, S. M. Kreidenweis, M. J. Molina, A. Nenes, J. E. Penner, K. A. Prather, V. Ramanathan, V. Ramaswamy, P. J. Rasch, A. R. Ravishankara, D. Rosenfeld, G. Stephens and R. Wood, Improving Our Fundamental Understanding of the Role of Aerosol-cloud Interactions in the Climate System, Proc. Natl. Acad. Sci. U. S. A., 2016, 113(21), 5781–5790, DOI:10.1073/pnas.1514043113.
- S. D. D'Andrea, S. A. K. Häkkinen, D. M. Westervelt, C. Kuang, E. J. T. Levin, V. P. Kanawade, W. R. Leaitch, D. V. Spracklen, I. Riipinen and J. R. Pierce, Understanding Global Secondary Organic Aerosol Amount and Size-Resolved Condensational Behavior, Atmos. Chem. Phys., 2013, 13(22), 11519–11534, DOI:10.5194/acp-13-11519-2013.
- S. Sandrini, D. van Pinxteren, L. Giulianelli, H. Herrmann, L. Poulain, M. C. Facchini, S. Gilardoni, M. Rinaldi, M. Paglione, B. J. Turpin, F. Pollini, S. Bucci, N. Zanca and S. Decesari, Size-Resolved Aerosol Composition at an Urban and a Rural Site in the Po Valley in Summertime: Implications for Secondary Aerosol Formation, Atmos. Chem. Phys., 2016, 16(17), 10879–10897, DOI:10.5194/acp-16-10879-2016.
- E. J. T. Levin, A. J. Prenni, B. B. Palm, D. A. Day, P. Campuzano-Jost, P. M. Winkler, S. M. Kreidenweis, P. J. DeMott, J. L. Jimenez and J. N. Smith, Size-Resolved Aerosol Composition and Its Link to Hygroscopicity at a Forested Site in Colorado, Atmos. Chem. Phys., 2014, 14(5), 2657–2667, DOI:10.5194/acp-14-2657-2014.
- M. Petters and S. Kasparoglu, Predicting the Influence of Particle Size on the Glass Transition Temperature and Viscosity of Secondary Organic Material, Sci. Rep., 2020, 10(1), 15170, DOI:10.1038/s41598-020-71490-0.
- Y. Cheng, H. Su, T. Koop, E. Mikhailov and U. Pöschl, Size Dependence of Phase Transitions in Aerosol Nanoparticles, Nat. Commun., 2015, 6(1), 5923, DOI:10.1038/ncomms6923.
- T. Koop, J. Bookhold, M. Shiraiwa and U. Pöschl, Glass Transition
and Phase State of Organic Compounds: Dependency on Molecular Properties and Implications for Secondary Organic Aerosols in the Atmosphere, Phys. Chem. Chem. Phys., 2011, 13(43), 19238–19255, 10.1039/c1cp22617g.
- M. Shiraiwa, Y. Li, A. P. Tsimpidi, V. A. Karydis, T. Berkemeier, S. N. Pandis, J. Lelieveld, T. Koop and U. Pöschl, Global Distribution of Particle Phase State in Atmospheric Secondary Organic Aerosols, Nat. Commun., 2017, 8, 1–7, DOI:10.1038/ncomms15002.
- A. J. Sedlacek III, E. R. Lewis, T. B. Onasch, P. Zuidema, J. Redemann, D. Jaffe and L. I. Kleinman, Using the Black Carbon Particle Mixing State to Characterize the Lifecycle of Biomass Burning Aerosols, Environ. Sci. Technol., 2022, 56(20), 14315–14325, DOI:10.1021/acs.est.2c03851.
- W. Xu, C. Chen, Y. Qiu, C. Xie, Y. Chen, N. Ma, W. Xu, P. Fu, Z. Wang, X. Pan, J. Zhu, N. L. Ng and Y. Sun, Size-Resolved Characterization of Organic Aerosol in the North China Plain: New Insights from High Resolution Spectral Analysis, Environ. Sci.: Atmos., 2021, 1(6), 346–358, 10.1039/D1EA00025J.
- R. A. Di Lorenzo, B. K. Place, T. C. VandenBoer and C. J. Young, Composition of Size-Resolved Aged Boreal Fire Aerosols: Brown Carbon, Biomass Burning Tracers, and Reduced Nitrogen, ACS Earth Space Chem., 2018, 2(3), 278–285, DOI:10.1021/acsearthspacechem.7b00137.
- L. P. McLaughlin and E. L. Belmont, Size-Resolved Aerosol Emissions from Lignocellulosic Biomass and Biomass Constituent Pyrolysis under Variable Dilution Temperatures, J. Aerosol Sci., 2021, 151, 105679, DOI:10.1016/j.jaerosci.2020.105679.
- J. Zhai, X. Lu, L. Li, Q. Zhang, C. Zhang, H. Chen, X. Yang and J. Chen, Size-Resolved Chemical Composition, Effective Density, and Optical Properties of Biomass Burning Particles, Atmos. Chem. Phys., 2017, 17(12), 7481–7493, DOI:10.5194/acp-17-7481-2017.
- W. Hu, M. Hu, W.-W. Hu, H. Niu, J. Zheng, Y. Wu, W. Chen, C. Chen, L. Li, M. Shao, S. Xie and Y. Zhang, Characterization of Submicron Aerosols Influenced by Biomass Burning at a Site in the Sichuan Basin, Southwestern China, Atmos. Chem. Phys., 2016, 16(20), 13213–13230, DOI:10.5194/acp-16-13213-2016.
- Y. Liu, X. Meng, Z. Wu, D. Huang, H. Wang, J. Chen, J. Chen, T. Zong, X. Fang, T. Tan, G. Zhao, S. Chen, L. Zeng, S. Guo, X. Huang, L. He, L. Zeng and M. Hu, The Particle Phase State during the Biomass Burning Events, Sci. Total Environ., 2021, 792, 148035, DOI:10.1016/j.scitotenv.2021.148035.
- J. H. Slade, A. P. Ault, A. T. Bui, J. C. Ditto, Z. Lei, A. L. Bondy, N. E. Olson, R. D. Cook, S. J. Desrochers, R. M. Harvey, M. H. Erickson, H. W. Wallace, S. L. Alvarez, J. H. Flynn, B. E. Boor, G. A. Petrucci, D. R. Gentner, R. J. Griffin and P. B. Shepson, Bouncier Particles at Night: Biogenic Secondary Organic Aerosol Chemistry and Sulfate Drive Diel Variations in the Aerosol Phase in a Mixed Forest, Environ. Sci. Technol., 2019, 53(9), 4977–4987, DOI:10.1021/acs.est.8b07319.
- J. B. Shaw, T.-Y. Lin, F. E. Leach, A. V. Tolmachev, N. Tolić, E. W. Robinson, D. W. Koppenaal and L. Paša-Tolić, 21 Tesla Fourier Transform Ion Cyclotron Resonance Mass Spectrometer Greatly Expands Mass Spectrometry
Toolbox, J. Am. Soc. Mass Spectrom., 2016, 27(12), 1929–1936, DOI:10.1007/s13361-016-1507-9.
- G. W. Vandergrift, W. Kew, J. K. Lukowski, A. Bhattacharjee, A. V. Liyu, E. A. Shank, L. Paša-Tolić, V. Prabhakaran and C. R. Anderton, Imaging and Direct Sampling Capabilities of Nanospray Desorption Electrospray Ionization with Absorption-Mode 21 Tesla Fourier Transform Ion Cyclotron Resonance Mass Spectrometry, Anal. Chem., 2022, 94(8), 3629–3636, DOI:10.1021/acs.analchem.1c05216.
- B. Wang, T. H. Harder, S. T. Kelly, D. S. Piens, S. China, L. Kovarik, M. Keiluweit, B. W. Arey, M. K. Gilles and A. Laskin, Airborne Soil Organic Particles Generated by Precipitation, Nat. Geosci., 2016, 9(6), 433–437, DOI:10.1038/ngeo2705.
- Z. Cheng, N. Sharma, K.-P. Tseng, L. Kovarik and S. China, Direct Observation and Assessment of Phase States of Ambient and Lab-Generated Sub-Micron Particles upon Humidification, RSC Adv., 2021, 11(25), 15264–15272, 10.1039/D1RA02530A.
- Z. Cheng, M. Morgenstern, B. Zhang, M. Fraund, N. N. Lata, R. Brimberry, M. A. Marcus, L. Mazzoleni, P. Fialho, S. Henning, B. Wehner, C. Mazzoleni and S. China, Particle Phase-State Variability in the North Atlantic Free Troposphere during Summertime Is Determined by Atmospheric Transport Patterns and Sources, Atmos. Chem. Phys., 2022, 22(13), 9033–9057, DOI:10.5194/acp-22-9033-2022.
- A. Laskin, T. W. Wietsma, B. J. Krueger and V. H. Grassian, Heterogeneous Chemistry of Individual Mineral Dust Particles with Nitric Acid: A Combined CCSEM/EDX, ESEM, and ICP-MS Study, J. Geophys. Res. Atmos., 2005, 110(D10), D10208, DOI:10.1029/2004JD005206.
- A. Laskin, J. P. Cowin and M. J. Iedema, Analysis of Individual Environmental Particles Using Modern Methods of Electron Microscopy and X-Ray Microanalysis, J. Electron Spectrosc. Relat. Phenom., 2006, 150(2), 260–274, DOI:10.1016/j.elspec.2005.06.008.
- M. Cui, Introduction to the K-Means Clustering Algorithm Based on the Elbow Method, J. Account. Audit. Finance, 2020, 1(1), 5–8 Search PubMed.
- A. L. D. Kilcoyne, T. Tyliszczak, W. F. Steele, S. Fakra, P. Hitchcock, K. Franck, E. Anderson, B. Harteneck, E. G. Rightor, G. E. Mitchell, A. P. Hitchcock, L. Yang, T. Warwick and H. Ade, Interferometer-Controlled Scanning Transmission X-Ray Microscopes at the Advanced Light Source, J. Synchrotron Radiat., 2003, 10(2), 125–136 CrossRef CAS PubMed.
- N. N. Lata, B. Zhang, S. Schum, L. Mazzoleni, R. Brimberry, M. A. Marcus, W. H. Cantrell, P. Fialho, C. Mazzoleni and S. China, Aerosol Composition, Mixing State, and Phase State of Free Tropospheric Particles and Their Role in Ice Cloud Formation, ACS Earth Space Chem., 2021, 5(12), 3499–3510, DOI:10.1021/acsearthspacechem.1c00315.
- D. A. Knopf, P. A. Alpert, B. Wang, R. E. O’Brien, S. T. Kelly, A. Laskin, M. K. Gilles and R. C. Moffet, Microspectroscopic Imaging and Characterization of Individually Identified Ice Nucleating Particles from a Case Field Study, J. Geophys. Res.: Atmos., 2014, 119, 10365–10381, DOI:10.1002/2014JD021866.
-
R. Moffet, A. Tivanski and M. Gilles, in Fundamentals and Applications in Aerosol Spectroscopy, ed. R. Signorell and J. P. Reid, CRC Press, Boca Raton, 2010, 1st edn, vol. 1, ch. 17, pp. 243–272 Search PubMed.
- R. E. O'Brien, A. Neu, S. A. Epstein, A. C. MacMillan, B. Wang, S. T. Kelly, S. A. Nizkorodov, A. Laskin, R. C. Moffet and M. K. Gilles, Physical Properties of Ambient and Laboratory-Generated Secondary Organic Aerosol, Geophys. Res. Lett., 2014, 41(12), 4347–4353, DOI:10.1002/2014GL060219.
- J. M. Tomlin, K. A. Jankowski, F. A. Rivera-Adorno, M. Fraund, S. China, B. H. Stirm, R. Kaeser, G. S. Eakins, R. C. Moffet, P. B. Shepson and A. Laskin, Chemical Imaging of Fine Mode Atmospheric Particles Collected from a Research Aircraft over Agricultural Fields, ACS Earth Space Chem., 2020, 4(11), 2171–2184, DOI:10.1021/acsearthspacechem.0c00172.
- J. P. Reid, A. K. Bertram, D. O. Topping, A. Laskin, S. T. Martin, M. D. Petters, F. D. Pope and G. Rovelli, The Viscosity of Atmospherically Relevant Organic Particles, Nat. Commun., 2018, 9(1), 956, DOI:10.1038/s41467-018-03027-z.
- R. C. Moffet, T. C. Rödel, S. T. Kelly, X. Y. Yu, G. T. Carroll, J. Fast, R. A. Zaveri, A. Laskin and M. K. Gilles, Spectro-Microscopic Measurements of Carbonaceous Aerosol Aging in Central California, Atmos. Chem. Phys., 2013, 13(20), 10445–10459, DOI:10.5194/acp-13-10445-2013.
- D. Q. Pham, R. O'Brien, M. Fraund, D. Bonanno, O. Laskina, C. Beall, K. A. Moore, S. Forestieri, X. Wang, C. Lee, C. Sultana, V. Grassian, C. D. Cappa, K. A. Prather and R. C. Moffet, Biological Impacts on Carbon Speciation and Morphology of Sea Spray Aerosol, ACS Earth Space Chem., 2017, 1(9), 551–561, DOI:10.1021/acsearthspacechem.7b00069.
- G. W. Vandergrift, A. S. M. Shawon, D. N. Dexheimer, M. A. Zawadowicz, F. Mei and S. China, Molecular Characterization of Organosulfate-Dominated Aerosols over Agricultural Fields from the Southern Great Plains by High-Resolution Mass Spectrometry, ACS Earth Space Chem., 2022, 6(7), 1733–1741, DOI:10.1021/acsearthspacechem.2c00043.
- S. K. Schum, L. E. Brown and L. R. Mazzoleni, MFAssignR: Molecular Formula Assignment Software for Ultrahigh Resolution Mass Spectrometry Analysis of Environmental Complex Mixtures, Environ. Res., 2020, 191, 110114, DOI:10.1016/j.envres.2020.110114.
- Y. Li, D. A. Day, H. Stark, J. L. Jimenez and M. Shiraiwa, Predictions of the Glass Transition Temperature and Viscosity of Organic Aerosols from Volatility Distributions, Atmos. Chem. Phys., 2020, 20(13), 8103–8122, DOI:10.5194/acp-20-8103-2020.
- Y. Li, U. Pöschl and M. Shiraiwa, Molecular Corridors and Parameterizations of Volatility in the Chemical Evolution of Organic Aerosols, Atmos. Chem. Phys., 2016, 16(5), 3327–3344, DOI:10.5194/acp-16-3327-2016.
- T. D. Vaden, D. Imre, J. Beránek, M. Shrivastava and A. Zelenyuk, Evaporation Kinetics and Phase of Laboratory and Ambient Secondary Organic Aerosol, Proc. Natl. Acad. Sci. U. S. A., 2011, 108(6), 2190–2195, DOI:10.1073/pnas.1013391108.
- J. S. Reid, R. Koppmann, T. F. Eck and D. P. Eleuterio, A Review of Biomass Burning Emissions Part II: Intensive Physical Properties of Biomass Burning Particles, Atmos. Chem. Phys., 2005, 5(3), 799–825, DOI:10.5194/acp-5-799-2005.
- C. D. McClure, C. Y. Lim, D. H. Hagan, J. H. Kroll and C. D. Cappa, Biomass-Burning-Derived Particles from a Wide Variety of Fuels – Part 1: Properties of Primary Particles, Atmos. Chem. Phys., 2020, 20(3), 1531–1547, DOI:10.5194/acp-20-1531-2020.
- P. Guyon, G. P. Frank, M. Welling, D. Chand, P. Artaxo, L. Rizzo, G. Nishioka, O. Kolle, H. Fritsch, M. A. F. Silva Dias, L. V. Gatti, A. M. Cordova and M.
O. Andreae, Airborne Measurements of Trace Gas and Aerosol Particle Emissions from Biomass Burning in Amazonia, Atmos. Chem. Phys., 2005, 5(11), 2989–3002, DOI:10.5194/acp-5-2989-2005.
- D. Shang, M. Hu, J. Zheng, Y. Qin, Z. Du, M. Li, J. Fang, J. Peng, Y. Wu, S. Lu and S. Guo, Particle Number Size Distribution and New Particle Formation under the Influence of Biomass Burning at a High Altitude Background Site at Mt. Yulong (3410 m), China, Atmos. Chem. Phys., 2018, 18(21), 15687–15703, DOI:10.5194/acp-18-15687-2018.
- Z. B. Wang, M. Hu, Z. J. Wu, D. L. Yue, L. Y. He, X. F. Huang, X. G. Liu and A. Wiedensohler, Long-Term Measurements of Particle Number Size Distributions and the Relationships with Air Mass History and Source Apportionment in the Summer of Beijing, Atmos. Chem. Phys., 2013, 13(20), 10159–10170, DOI:10.5194/acp-13-10159-2013.
- R. Atkinson and J. Arey, Atmospheric Chemistry of Gas-Phase Polycyclic Aromatic Hydrocarbons: Formation of Atmospheric Mutagens, Environ. Health Perspect., 1994, 102(suppl. 4), 117–126, DOI:10.1289/ehp.94102s4117.
- N. L. Ng, S. S. Brown, A. T. Archibald, E. Atlas, R. C. Cohen, J. N. Crowley, D. A. Day, N. M. Donahue, J. L. Fry, H. Fuchs, R. J. Griffin, M. I. Guzman, H. Herrmann, A. Hodzic, Y. Iinuma, J. L. Jimenez, A. Kiendler-Scharr, B. H. Lee, D. J. Luecken, J. Mao, R. McLaren, A. Mutzel, H. D. Osthoff, B. Ouyang, B. Picquet-Varrault, U. Platt, H. O. T. Pye, Y. Rudich, R. H. Schwantes, M. Shiraiwa, J. Stutz, J. A. Thornton, A. Tilgner, B. J. Williams and R. A. Zaveri, Nitrate Radicals and Biogenic Volatile Organic Compounds: Oxidation, Mechanisms, and Organic Aerosol, Atmos. Chem. Phys., 2017, 17(3), 2103–2162, DOI:10.5194/acp-17-2103-2017.
- A. Ijaz, W. Kew, S. China, S. K. Schum and L. R. Mazzoleni, Molecular Characterization of Organophosphorus Compounds in Wildfire Smoke Using 21-T Fourier Transform-Ion Cyclotron Resonance Mass Spectrometry, Anal. Chem., 2022, 94(42), 14537–14545, DOI:10.1021/acs.analchem.2c00916.
- W. Kew, A. Myers-Pigg, C. Chang, S. Colby, J. Eder, M. Tfaily, J. Hawkes, R. Chu and J. Stegen, Reviews and Syntheses: Use and Misuse of Peak Intensities from High Resolution Mass Spectrometry in Organic Matter Studies: Opportunities for Robust Usag, EGUsphere, 2022 DOI:10.5194/egusphere-2022-1105.
- J. A. Hawkes, C. Patriarca, P. J. R. Sjöberg, L. J. Tranvik and J. Bergquist, Extreme Isomeric Complexity of Dissolved Organic Matter Found across Aquatic Environments, Limnol. Oceanogr. Lett., 2018, 3(2), 21–30, DOI:10.1002/lol2.10064.
- B. P. Koch and T. Dittmar, From Mass to Structure: An Aromaticity Index for High-Resolution Mass Data of Natural Organic Matter, Rapid Commun. Mass Spectrom., 2006, 20(5), 926–932, DOI:10.1002/rcm.2386.
- B. P. Koch and T. Dittmar, From Mass to Structure: An Aromaticity Index for High-Resolution Mass Data of Natural Organic Matter, Rapid Commun. Mass Spectrom., 2016, 30(1), 250, DOI:10.1002/rcm.7433.
- M. Brege, M. Paglione, S. Gilardoni, S. Decesari, M. C. Facchini and L. R. Mazzoleni, Molecular Insights on Aging and Aqueous-Phase Processing from Ambient Biomass Burning Emissions-Influenced Po Valley Fog and Aerosol, Atmos. Chem. Phys., 2018, 18(17), 13197–13214, DOI:10.5194/acp-18-13197-2018.
- L. D. Yee, K. E. Kautzman, C. L. Loza, K. A. Schilling, M. M. Coggon, P. S. Chhabra, M. N. Chan, A. W. H. Chan, S. P. Hersey, J. D. Crounse, P. O. Wennberg, R. C. Flagan and J. H. Seinfeld, Secondary Organic Aerosol Formation from Biomass Burning Intermediates: Phenol and Methoxyphenols, Atmos. Chem. Phys., 2013, 13(16), 8019–8043, DOI:10.5194/acp-13-8019-2013.
- J. R. Conway, A. Lex and N. Gehlenborg, UpSetR: An R Package for the Visualization of Intersecting Sets and Their Properties, Bioinformatics, 2017, 33(18), 2938–2940, DOI:10.1093/bioinformatics/btx364.
- J. Li, J. Li, G. Wang, T. Zhang, W. Dai, K. F. Ho, Q. Wang, Y. Shao, C. Wu and L. Li, Molecular Characteristics of Organic Compositions in Fresh and Aged Biomass Burning Aerosols, Sci. Total Environ., 2020, 741, 140247, DOI:10.1016/j.scitotenv.2020.140247.
- D. M. Smith, T. Cui, M. N. Fiddler, R. P. Pokhrel, J. D. Surratt and S. Bililign, Laboratory Studies of Fresh and Aged Biomass Burning Aerosol Emitted from East African Biomass Fuels – Part 2: Chemical Properties and Characterization, Atmos. Chem. Phys., 2020, 20(17), 10169–10191, DOI:10.5194/acp-20-10169-2020.
- J. H. Slade, R. Thalman, J. Wang and D. A. Knopf, Chemical Aging of Single and Multicomponent Biomass Burning Aerosol Surrogate Particles by OH: Implications for Cloud Condensation Nucleus Activity, Atmos. Chem. Phys., 2015, 15(17), 10183–10201, DOI:10.5194/acp-15-10183-2015.
- G. Girotto, S. China, J. Bhandari, K. Gorkowski, B. V. Scarnato, T. Capek, A. Marinoni, D. P. Veghte, G. Kulkarni, A. C. Aiken, M. Dubey and C. Mazzoleni, Fractal-like Tar Ball Aggregates from Wildfire Smoke, Environ. Sci. Technol. Lett., 2018, 5(6), 360–365, DOI:10.1021/acs.estlett.8b00229.
- M. A. Brege, S. China, S. Schum, A. Zelenyuk and L. R. Mazzoleni, Extreme Molecular Complexity Resulting in a Continuum of Carbonaceous Species in Biomass Burning Tar Balls from Wildfire Smoke, ACS Earth Space Chem., 2021, 5(10), 2729–2739, DOI:10.1021/acsearthspacechem.1c00141.
- Q. Yuan, J. Xu, L. Liu, A. Zhang, Y. Liu, J. Zhang, X. Wan, M. Li, K. Qin, Z. Cong, Y. Wang, S. Kang, Z. Shi, M. Pósfai and W. Li, Evidence for Large Amounts of Brown Carbonaceous Tarballs in the Himalayan Atmosphere, Environ. Sci. Technol. Lett., 2021, 8(1), 16–23, DOI:10.1021/acs.estlett.0c00735.
- M. Pósfai, A. Gelencsér, R. Simonics, K. Arató, J. Li, P. V. Hobbs and P. R. Buseck, Atmospheric Tar Balls: Particles from Biomass and Biofuel Burning, J. Geophys. Res. Atmos., 2004, 109(D6), D06213, DOI:10.1029/2003JD004169.
- T. Koop, J. Bookhold, M. Shiraiwa and U. Pöschl, Glass Transition and Phase State of Organic Compounds: Dependency on Molecular Properties and Implications for Secondary Organic Aerosols in the Atmosphere, Phys. Chem. Chem. Phys., 2011, 13(43), 19238–19255, 10.1039/C1CP22617G.
- V. J. Baboomian, G. V. Crescenzo, Y. Huang, F. Mahrt, M. Shiraiwa, A. K. Bertram and S. A. Nizkorodov, Sunlight Can Convert Atmospheric Aerosols into a Glassy Solid State and Modify Their Environmental Impacts, Proc. Natl. Acad. Sci. U. S. A., 2022, 119(43), e2208121119, DOI:10.1073/pnas.2208121119.
- Y. Zhang, J. Fan, M. Shrivastava, C. R. Homeyer, Y. Wang and J. H. Seinfeld, Notable Impact of Wildfires in the Western United States on Weather Hazards in the Central United States, Proc. Natl. Acad. Sci. U. S. A., 2022, 119(44), e2207329119, DOI:10.1073/pnas.2207329119.
- L. Damany-Pearce, B. Johnson, A. Wells, M. Osborne, J. Allan, C. Belcher, A. Jones and J. Haywood, Australian Wildfires Cause the Largest Stratospheric Warming since Pinatubo and Extends the Lifetime of the Antarctic Ozone Hole, Sci. Rep., 2022, 12(1), 12665, DOI:10.1038/s41598-022-15794-3.
Footnotes |
† Electronic supplementary information (ESI) available. See DOI: https://doi.org/10.1039/d3ea00058c |
‡ Current address: Laboratoire de Chimie Environnement, Université d'Aix-Marseille, Marseille, 13007, France. |
§ Current address: Earth and Environmental Sciences Division, Los Alamos National Laboratory, Los Alamos, NM 87545, USA. |
|
This journal is © The Royal Society of Chemistry 2023 |
Click here to see how this site uses Cookies. View our privacy policy here.