Quantitative sustainable design (QSD) for the prioritization of research, development, and deployment of technologies: a tutorial and review†
Received
6th June 2022
, Accepted 2nd September 2022
First published on 30th September 2022
Abstract
The pursuit of sustainability has catalyzed broad investment in the research, development, and deployment (RD&D) of innovative water, sanitation, and resource recovery technologies, yet the lack of transparent and agile methodologies to navigate the expansive landscape of technology development pathways remains a critical challenge. This challenge is further complicated by the higher levels of uncertainty that are intrinsic to early-stage technologies. In this work, we review and synthesize published literature on the sustainability analyses of water and related technologies to present quantitative sustainable design (QSD) – a methodology to expedite and support technology RD&D. With a shared lexicon and a structured approach, QSD facilitates interdisciplinary communication and research consistency. In introducing QSD, we review existing studies to highlight best practices and discuss them in the context of the specific steps of QSD, which include defining the problem space, establishing simulation algorithms, and characterizing system sustainability across economic, environmental, human health, and social dimensions. Next, we summarize tools for QSD execution and provide recommendations to account for uncertainty in this process. We further discuss applications of QSD in the fields of water/wastewater and beyond (e.g., renewable fuels, circular economy) in combination with uncertainty, sensitivity, and scenario analyses to generate the desired types of insight. Finally, we identify future research needs for sustainability analyses to advance technology RD&D. Ultimately, QSD can be used to elucidate the complex and intertwined connections among design decisions, technology characteristics, contextual factors, and sustainability indicators, thereby supporting transparent, consistent, and agile RD&D.
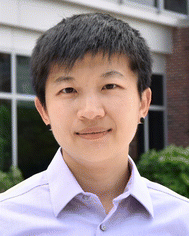 Yalin Li | Dr. Li is a Research Scientist in the Institute for Sustainability, Energy, and Environmental at the University of Illinois Urbana-Champaign. With an experimental background on the development of thermochemical and catalytic technologies for resource recovery from wastewater, Yalin is interested in advancing the sustainability of water and energy infrastructure through experimentation and quantitative sustainable design. Recent research projects span across sanitation and resource recovery systems, field-to-market bioeconomy value chain, and the development of open-source platforms for process design, sustainability analyses, as well as decision-making. |
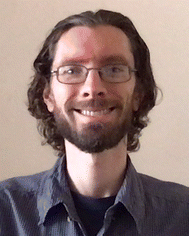 John Trimmer | Dr. Trimmer is the Deputy Director of Research at the Aquaya Institute, a non-profit research and consulting organization dedicated to safe water and sanitation. Based in Nairobi, Kenya, John develops and oversees Aquaya's research programs, with particular focus on safe and sustainable sanitation in urban and rural contexts. Previously, John studied the sustainability of resource recovery from sanitation as a post-doctoral research associate and doctoral student at the University of Illinois Urbana-Champaign. He also served for three years as a United States Peace Corps Volunteer in Uganda, where he supported the NGO Brick by Brick Uganda and studied Ecological Sanitation. |
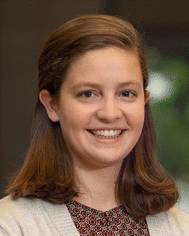 Diana Byrne | Dr. Byrne is an Assistant Professor in the Department of Civil Engineering at the University of Kentucky. Her research focuses on applying and adapting quantitative sustainable design tools such as life cycle assessment and quantitative microbial risk assessment to guide decision-making for water and sanitation infrastructure. She is interested in applications of sustainable design in various settings, with an emphasis on context-specific objectives and constraints. Within civil engineering, she engages in research and teaching that focus on applying sustainable design and engineering principles to pursue meaningful impacts for people and the environment. |
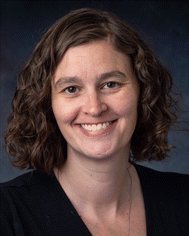 Sherri Cook | Dr. Cook is an Assistant Professor of Environmental Engineering at the University of Colorado Boulder. Her research group has three main research thrusts: charting pathways for sustainable water and waste management systems by applying existing evaluation methodologies, in particular life cycle assessment (LCA), to existing and novel technologies to enable sustainable water treatment technology development and implementation; advancing sustainability-based evaluations and engineering decisions by developing new methods and decision frameworks to promote universal and sustained sanitation; and advancing environmental biotechnology applications by investigating novel biological process capabilities to enable safe water reuse. |
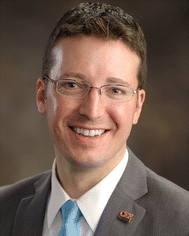 Jeremy Guest | Dr. Guest is an Associate Professor and the David S. Crawford Faculty Scholar in the Department of Civil and Environmental Engineering at the University of Illinois Urbana-Champaign (UIUC). His research and teaching centers on the development of technologies for sustainable water, sanitation, biofuels and bioproducts with applications in both high-income and underserved communities. In addition to research and teaching, he also serves as the Associate Director for Research for the Institute for Sustainability, Energy, and Environment (iSEE) and as the Illinois Thrust Lead for Sustainability for the IBM-Illinois Discovery Accelerator Institute, both at UIUC. |
Water impact
Research, development, and deployment (RD&D) of innovative technologies are often impeded by the lack of transparent, systematic, and agile approaches to prioritize investment across the expansive landscape of technologies and design/operational decisions. This tutorial review synthesizes research on sustainability analyses to present quantitative sustainable design (QSD) – a structured methodology to expedite the RD&D of water, sanitation, and resource recovery technologies.
|
1. Introduction
With roots in ancient civilizations,1–4 sustainability has gained public attention in recent decades with a series of landmark reports,5–8 events,9 and treaties.10,11 These efforts recognize the necessity to promote equitable and prosperous communities while simultaneously maintaining the ecosystems that support them.12–15 This need is particularly relevant for water technologies as they safeguard basic human rights (water and sanitation16–18) and are essential components of broader initiatives to re-envision other systems designed to meet societal needs (e.g., resource recovery,19,20 green chemistry,21–23 regenerative agriculture24,25).26–29 In line with this need, the field of sustainability science30–33 and sustainability engineering34 continue to evolve in support of the society's transition toward sustainability.35,36
To apply the concept of sustainability in the research, development, and deployment (RD&D) of technologies, implementable and systematic methodologies are necessary to quantitatively evaluate technologies as they mature.37,38 To this end, multiple sets of guiding principles (e.g., the Green Chemistry39 and Green Engineering21 Principles) and frameworks (e.g., the UN Inclusive Wealth framework40) have been proposed. These principles and frameworks often represent aspirational goals for technologies, engineered systems, or national entities, and are not intended to provide structured guidance to inform the prioritization of RD&D for specific technologies. Similarly, methodologies such as Life Cycle Sustainability Assessment have been proposed to address the “whole-picture” of sustainability,41 but methodologies such as this often lack quantitative, transparent strategies to navigate tradeoffs across dimensions of sustainability and technology-specific indicators of engineering performance (e.g., contaminant removal).42 More critically, there is a growing recognition of the importance of uncertainty, especially for early-stage technologies associated with higher levels of uncertainty.43,44 Nonetheless, the results of sustainability analyses are often presented as single values. This “false precision” overlooks aleatory (due to randomness) and epistemic (due to the lack of knowledge) uncertainty that is ubiquitous for early-stage technologies, thereby compromising the veracity of the results.45 Ultimately, these limitations undermine the accessibility and utility of sustainability analyses to inform decision-making for the RD&D of technologies, which is crucial in the society's pursuit of sustainability.8
In this paper, we review and synthesize published literature related to sustainability analyses to present a tutorial review on quantitative sustainable design (QSD). QSD integrates concepts associated with sustainability science and engineering to expedite and support the RD&D of technologies (Fig. 1). Through the lens of QSD, we establish a shared lexicon as the foundation for interdisciplinary communication and to support methodological transparency and consistency (Table 1). In presenting this methodology, we discuss published studies using the shared lexicon to put them in the context of QSD. Specifically, we begin by defining the problem space, which includes specifying the system of interest as well as relevant decision variables, technological parameters, and contextual parameters (section 2). Next, we establish design and process algorithms to generate the system inventory (mass and energy flows that enter and leave the system, section 3). Sustainability indicators can then be quantified using techniques that span economic, environmental, human health, and social dimensions (section 4). By compiling all algorithms used in system simulation and sustainability characterization, a system model can be created to quantify uncertainty and generate the desired types of insight (section 5.1). Through the review of existing tools, we discuss the status of tools for sustainability analyses and how the different steps of QSD can be executed under uncertainty (section 5.2). With literature examples and representative figures, we further illustrate how QSD can be used (i) to characterize sustainability indicators under uncertainty, (ii) to identify sustainability drivers, (iii) to set RD&D targets, (iv) to understand uncertainty drivers, (v) to explore alternative scenarios (i.e., distinct combinations of decision variables, technological parameters, and contextual parameters), and (vi) to inform practical deployment (section 5.3). Ultimately, QSD can be used to elucidate the complex and intertwined connections among design decisions, technology characteristics, contextual factors, and sustainability indicators, thus enabling transparent and agile planning and design processes. Finally, we identify future research needs for the continued development and application of sustainability analyses for technology RD&D, with the goal of supporting society's pursuit of sustainable development (section 6).
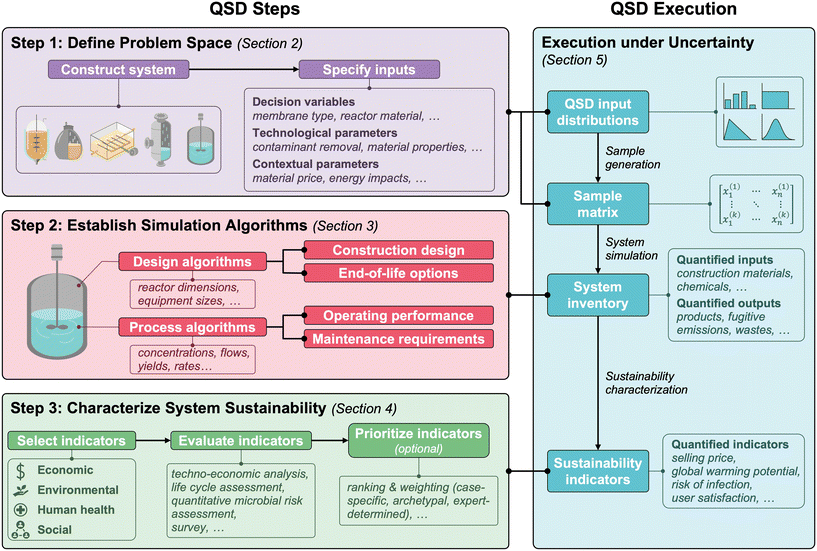 |
| Fig. 1 Overview of the quantitative sustainable design (QSD) methodology, which includes the steps of defining problem space, establishing simulation algorithms, and characterizing system sustainability (left panel); corresponding outputs from each step (and their integration under uncertainty) are included in the right panel. | |
Table 1 Summary of QSD terminologya
Term |
Definition |
Additional details about each term can be found in their respective sections of this tutorial review.
Unless specified with “background”, “system” and “unit processes” in QSD refers to the foreground system and unit processes.
|
Sustainability analyses |
Evaluation of a system's sustainability which may address one or multiple dimensions, including economic, environmental, human health, and social |
Quantitative sustainable design (QSD) |
A structured methodology for the sustainability analyses of technologies |
Section 2. Define problem space (step 1 of QSD) |
QSD input |
Variable or parameter that influences the sustainability of the system, including decision variables, technological parameters, and contextual parameters |
Decision variable |
Independent variable that can be controlled by the designer or the operator46 |
Technological parameter |
Parameter that is intrinsic to a technology (including components of the technology)46 |
Contextual parameter |
Non-technological parameters that influence the sustainability of a technology47 |
Problem space |
Conceptual N-dimensional space formed by the combination of all possible values of the N QSD inputs, across which the system will be evaluated |
Opportunity space |
A sub-space of the problem space in which the selected technology outcompetes alternative technologies |
Unit process |
The smallest element considered in QSD; mass and energy flows of unit processes are considered in the system inventory analysis48–50 |
System boundary |
Set of criteria specifying which unit processes and upstream/downstream sources of sustainability impact are included in the system49,50 |
System inventory |
Mass and energy flows that enter and leave the system |
Section 3. Establish simulation algorithms (step 2 of QSD) |
Life cycle stage |
Particular phase of the system, including construction, operation and maintenance, and end-of-life stages |
Design algorithm |
Set of equations or procedures to generate mass and energy flows during the construction and/or the end-of-life stages of all unit processes within the system |
Process algorithm |
Set of equations or procedures to generate mass and energy flows during the operation and maintenance stages of all unit processes within the system |
Section 4. Characterize system sustainability (step 3 of QSD) |
Goal |
Broad, qualitative statements about objectives12 |
Indicator |
Quantitative measures selected to assess the progress toward or away from a stated goal12 |
Target |
Specific goals with endpoints and timetables to reach desired indicator values12 |
Trend |
Change in the value of an indicator over time12 |
Driving force |
Process that influences trends and the ability to meet targets12 |
Life cycle costing (LCC) |
Technique to estimate the costs associated with the existence of the system51 |
Techno-economic analysis (TEA) |
Technique to assess the financial viability of the system when considering all associated costs and revenues through a discounted cash flow analysis52 |
Cost–benefit analysis (CBA) |
Technique to compare the projected or estimated costs and benefits (or opportunities) associated with a project to the society53 |
Life cycle assessment (LCA) |
Technique to compile and evaluate the inputs, outputs, and potential environmental impacts of the system throughout its life cycle49,50 |
Foreground (unit) processes |
Unit processes that can be controlled by designers or operators54 |
Background (unit) processes |
Unit processes that designers and operators exercise no direct influence upon, but are relevant to foreground processes54 |
(Foreground) systemb |
Group of foreground processes in specific order (including their inputs and outputs) to conceptually represent the technologies to be studied in QSD54 |
Background systemb |
Group of background processes (including their inputs and outputs)54 |
Life cycle inventory (LCI) |
Inventory of mass and energy flows for both foreground and background systems49,50 |
Quantitative risk assessment (QRA) |
Technique to quantify the risks associated with an engineering process55 |
Quantitative microbial risk assessment (QMRA) |
Technique to assess human health risks that arise from the exposure to pathogens56 |
Social (and social-economic) life cycle assessment (SLCA or S-LCA) |
Technique to assess social and socio-economic impacts of the system throughout its life cycle, including how stakeholders (e.g., technology users, regulators, governments) perceive and interact with technologies57 |
Section 5. Execution and applications |
System design |
Definition of the problem space and establishment of simulation algorithms (steps 1 and 2 of QSD) |
System simulation |
Execution of the simulation algorithms to generate the system inventory |
System sustainability characterization |
Quantification of sustainability indicators using system inventory; certain sustainability characterization techniques (e.g., LCA) may convert system inventory to system life cycle inventory in this process (step 3 of QSD) |
System model |
Compilation of all algorithms used in system simulation and sustainability characterization |
Monte Carlo method |
Statistical technique to use stochasticity (i.e., randomness) for problems that are deterministic in nature58 |
Monte Carlo sampling |
Sampling technique to generate samples by repeatedly drawing random values from defined distributions (i.e., the problem space) using random number generators (i.e., random sampling)59 |
Latin hypercube sampling |
Quasi-random sampling technique that ensures all portions of the problem space are sampled by first dividing the uncertainty range of each QSD input into N strata of equal marginal probability, then sampling once from each stratum60 |
Uncertainty analyses |
Systematic procedure to quantify the uncertainty of QSD results49,50 |
Sensitivity analyses |
Systematic procedure to study how the uncertainty in the model outputs can be apportioned to different sources of uncertainties in model inputs44 |
Scenario |
Individual set of QSD inputs based on a coherent and internally consistent set of assumptions about key driving forces and their key relationships61 |
Scenario analyses |
Systematic procedure to draw conclusions for certain sets of inputs with specific values (i.e., scenarios)19 |
2. Define problem space
The first step of QSD is to define the problem space. In essence, this is the process of specifying the technology of interest, what will be included or excluded from the analysis (i.e., the system boundary), and the ranges of assumptions that can be made for QSD inputs. QSD inputs comprise any assumptions that may influence the performance and sustainability of the system, including decisions about the system design or operation of the system (i.e., decision variables), characteristics of the technology or its components (i.e., technological parameters), and the context in which the technology can be deployed (i.e., contextual parameters). The QSD inputs form an N-dimensional “problem space” encompassing all possible combinations of values of the QSD inputs (N is the number of QSD inputs), and the sustainability of the system will be evaluated within this space through QSD. To help familiarize the reader with these terms and their meaning, a summary of terminology definitions can be found in Table 1 and specific examples from the literature can be found in Tables 2 and S1 (ESI†).
Table 2 Literature examples illustrating concepts, steps, and applications of QSDb
|
Example 1 |
Example 2 |
Non-exhaustive list in the example paper.
Refer to Table S1 in the ESI† for additional literature examples.
|
Title |
Evaluation of life cycle assessment (LCA) for roadway drainage systems306 |
Quantitative microbial risk assessments for drinking water facilities: evaluation of a range of treatment strategies143 |
QSD objectives |
Evaluate the cost and environmental impacts of roadway drainage systems to guide future drainage design and operation |
Estimate the risk associated with pathogens for different drinking water treatment processes and their potential failures |
Step 1. Define problem space |
System boundary |
Roadway drainage system components including basin (detention or retention), linear conveyance element (bioswale, grass swale, storm sewer), culvert, and pipe underdrain |
Drinking water treatment facilities |
Life cycle stages |
Construction, operations, maintenance, end-of-life |
Operations, maintenance |
Decision variablesa |
Type of linear conveyance element |
Treatment processes |
Technological parametersa |
Concrete mix design; removal efficacy of basins and swales |
Disinfection volume and flowrates; hydraulic baffling factor |
Contextual parametersa |
Precipitation; initial pollutant concentration in the runoff; interest rate; unit costs of materials; frequency of maintenance activities |
Temperature and pH of the raw water |
Step 2. Establish simulation algorithms |
Algorithm types and levels of complexitya |
Theoretical values: calculation of the characterization factor for fugitive CO2 emissions from degraded biochemical oxygen demand in the stormwater |
Theoretical values: illness relationships (i.e., infection rates) for pathogens without literature data |
Existing design & data: design of drainage system components based on design standards |
Existing design & data: disinfection log removal |
Design heuristics; empirical models: direct emissions from equipment and flow from road into the drainage system |
Design heuristics; empirical models: pathogen dose response curves |
Mechanistic models: hydraulic retention time distribution using the N-CSTR approach (N is the number of theoretical continuously stirred tank reactors) |
Step 3. Characterize system sustainability |
Sustainability dimensions |
Economic; environmental |
Human health |
Characterization techniques |
Life cycle costing (LCC); life cycle assessment (LCA) |
Quantitative microbial risk assessment (QMRA) |
Sustainability indicators |
Cost and environmental impacts (ozone depletion, climate change, smog, acidification, eutrophication, carcinogenics, noncarcinogenics, respiratory effects, ecotoxicity, fossil fuel depletion, cumulative energy demand) |
Disability-adjusted life years (DALYs) |
Execution and applications |
Execution toolsa |
SimaPro; MATLAB |
Not reported |
Analysesa |
Uncertainty analyses: Fig. 2 and S2† |
Uncertainty analyses: Fig. 1 and 4–6 |
Sensitivity analyses: Fig. S8–S10† |
Scenario analyses: Fig. 5 and S11† |
Scenario analyses: Fig. 3 |
2.1. Construct system
Constructing the system (at a conceptual level) requires the specification of the system boundary to identify unit processes and sources of impact that will be included in or excluded from QSD. This conceptual system should contain the technologies of interest and their interactions with upstream, downstream, and parallel unit processes. Notably, the system does not need to be limited to the boundary of any specific physical system, but it can include any related processes that may be impacted by changes to QSD inputs. In selecting the system boundary, an “all-inclusive” approach would involve all upstream and downstream unit processes that interact directly or indirectly with the technologies. However, this approach may result in the inclusion of unit processes that are inconsequential to system sustainability, add unnecessary layers of complexity, and introduce sources of uncertainty that demand additional resources to evaluate. For example, in comparing capacitive deionization and reverse osmosis (RO) for brackish water desalination, pretreatment and brine disposal were excluded from one study because of their high sensitivities to variables (feedwater composition and geographical location, respectively) that were not relevant to the objectives of the study.62 Excluding these unit operations allowed the researchers to focus on core comparisons between capacitive deionization and RO without introducing additional unknowns that were expected to be independent of desalination technology choice (e.g., brine disposal method). Moreover, depending on the objectives of the QSD study (e.g., the number of technologies to be evaluated), the scale of the system can vary from a single unit process to a network of multiple subsystems across different disciplines and industries. Similarly, resolution of the design, simulation, and analyses can be tailored to the different unit processes within the system. Therefore, the definition of the conceptual system can be an iterative process to maintain focus on unit processes that are relevant to the sustainability indicators of interest or the targeted insight.
2.2. Specify inputs
2.2.1. Decision variables.
Decision variables are independent QSD inputs that can be controlled by the designer or operator. Decision variables may include system configurations, detailed design decisions for individual unit operations, operating conditions, and end-of-life options. They can be continuous, taking on any value within a specified range (e.g., operating temperature, adjusted pH, disinfectant concentration); or discrete, selected from a set of pre-specified values (e.g., aerobic or anaerobic treatment, catalyst type, fate of biosolids). Moreover, decision variables can be fixed or adjustable during operation. For example, when designing a membrane bioreactor, decision variables may include membrane material (a discrete, fixed decision variable), membrane area (a continuous, fixed decision variable), and membrane flux (a continuous, adjustable decision variable).46
In determining the values and distributions of the decision variables, the maturity of the technology is often considered and can be represented by technology and/or manufacturing readiness level (TRL/MRL). TRL, in particular, is often used when technology development (as opposed to manufacturing) is the focus.63,64 For example, for established technologies such as RO (TRL of 965), typical ranges of rejections have been established for different applications (e.g., micropollutant treatment66), whereas decision variables for emerging technologies such as CO2 utilization (TRL of 2–4) may be highly uncertain as designers and/or operators characterize the landscape of technical feasibility.67 Therefore, the latter case may require evaluation across wide ranges of decision variables and robust consideration of uncertainty in QSD execution (section 5.2).
2.2.2. Technological parameters.
Technological parameters are parameters intrinsic to a technology's design and operations (e.g., material properties, reaction coefficients). Unlike decision variables, values of technological parameters are subject to the technology rather than the designer or the operator (e.g., maximum specific growth rate when designing a biological treatment process). Notably, if a parameter is not intrinsic and can instead be calculated (e.g., cell growth rates can be calculated using maximum specific growth rates, temperature, and relevant constituent concentrations), this parameter should be modeled through algorithms rather than being included as an independent input. Similar to decision variables, magnitudes of technological parameter uncertainty can be related to TRL/MRL, with early-stage technologies having larger uncertainty due to the lack of knowledge. However, one can leverage theoretical values or technological limitations to constrain parameter uncertainty during QSD execution (section 3.2), and the results of QSD can be used in turn to set targets for technological parameters in research and development (section 5.3).
2.2.3. Contextual parameters.
Contextual parameters represent non-technological values that influence the sustainability of technologies, especially at the deployment stage.36 These parameters are intended to capture the specific circumstances in which the technology would be deployed. They reflect the local and regional nature of primary stressors to humans and the environment,13 accounting for economic (e.g., tax rates, unit costs), environmental (e.g., ambient temperatures), social (e.g., household size), political (e.g., regulations), and other conditions in which the system exists.68,69 As one example, outcomes associated with energy-intensive technologies may be especially sensitive to the characteristics of the local electricity grid, which can vary widely across and within countries: for example, the price of the electricity varies from $0.04 in Oklahoma to $0.26 in Hawaii,70 and the greenhouse gas (GHG) emissions from electricity production vary from 0.081 kg CO2 eq. per kW h−1 for the Bonneville Power Administration to 1.0 kg CO2 eq. per kW h−1 for the Ohio Valley Electric Corporation.71–73 Additionally, although contextual parameters are independent of the technology being evaluated, they may nonetheless directly or indirectly affect the technical performance of the system. For example, solar irradiance could directly impact the efficacy of sunlight-mediated disinfection technologies,74 while local and national regulations could indirectly affect the performance of waste sludge treatment systems through the set legal limits (e.g., how much sludge can be accepted by a landfill facility).75
For contextual parameters, the resolution of the data is also an important consideration. While higher resolution data (i.e., data being more specific to the deployment site) will yield results that more accurately reflect the deployment context, researchers may also be limited by the availability of data or the feasibility of new data collection. Generally, objectives of the QSD study will determine the appropriate resolution of contextual parameters (e.g., locality-specific, regional, nationally, or internationally representative averages), and additional analyses can be performed to assess how uncertainty in these parameters impacts system sustainability.
3. Establish simulation algorithms
After defining the problem space, the next step in QSD is to automate the process of translating QSD inputs into a system inventory (i.e., all mass and energy flows entering and leaving the system), which can be accomplished by establishing mathematical representations of the system across its life cycle. This step in QSD leverages design algorithms and process algorithms, where design algorithms correspond to the construction and end-of-life stages and process algorithms correspond to the operation and maintenance stage. Both design and process algorithms are linked to QSD inputs such that the system performance – including mass and energy balances across the life cycle – responds to changes in decision variables, technological parameters, and contextual parameters (illustrated in the following section).
3.1. Algorithms across system life cycle stages
3.1.1. Design algorithms for construction and end-of-life.
Design algorithms are sets of equations for the construction (e.g., equipment sizing, material selection) or end-of-life (e.g., disposal, salvage) stages of unit processes within the system. These algorithms can vary in complexity. For example, a simple design algorithm can scale the dimensions or number of fermenters based on the required volume, but a more complex model may include the specification of fermenter height, wall thickness, and weight, which are calculated using factors such as aspect ratio, fractional weld efficiency, reactor pressure, and material properties.76 Compared to construction, the relative importance of the end-of-life stage can be minor and is often excluded from the analysis (e.g., for wastewater treatment facilities77). However, this should not be considered a general rule, as end-of-life assumptions may be impactful in some systems or particularly relevant to specific analyses (e.g., recycling of demolition waste from residential buildings78).
3.1.2. Process algorithms for operations and maintenance.
Process algorithms are used to calculate the mass and energy flows throughout the system during the operation and maintenance stage. These flows can be used to gauge the performance of the technology (e.g., calculating contaminant removal) and to determine the relevant inventory items required for sustainability characterization (e.g., raw chemicals consumed, generated products and wastes, electricity consumption, and fugitive emissions such as CH4, CO2, and N2O). Like design algorithms, the complexity of process algorithms can vary widely from assumed performance based on theoretical values to calibrated and validated mechanistic models.
3.2. Algorithm selection
Regardless of their type (i.e., design vs. process), the complexity of the algorithms should be tailored to the objectives of the QSD study. Additionally, algorithm complexity can also be constrained by data availability. For technologies with high TRLs/MRLs (7+), data are generally more abundant as these technologies have been applied across more diverse contexts. Consequently, selection of the algorithms is often not data constrained and the algorithms can range from assumed values to more mechanism-driven, complex models. In contrast, emerging technologies with low TRLs/MRLs (1–2) may be limited to simple algorithms or assumed values due to the scarcity of the data (Fig. 2).
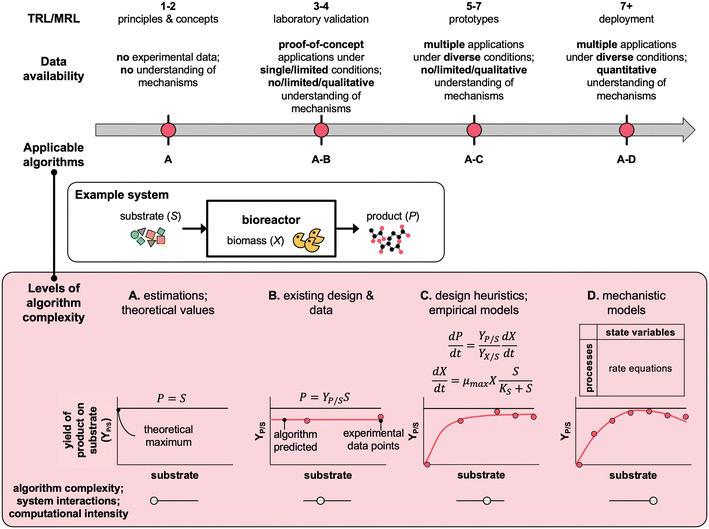 |
| Fig. 2 Illustration of algorithm selection with varying technology/manufacturing readiness levels (TRL/MRL) and data availability. The example system centers on the biological conversion of a substrate (S) to a product (P). For technologies with a higher TRL/MRL and more robust experimental data sets, algorithms that are more mechanism-driven can be developed to more explicitly represent underlying interactions within the system and elucidate hidden connections between QSD inputs and system sustainability. However, the availability of data should not dictate the choice of algorithms, as mechanism-driven models, which often have higher levels of complexity, can also require more computational resources and may introduce additional sources of uncertainty that are not relevant to the objectives of a QSD study. The illustration of algorithm complexity, system interactions, and computational intensity (bottom of the figure) for each type of the algorithms are solely for comparative purposes. In practical applications, they depend on multiple factors (e.g., the actual mechanisms) and could deviate from the typical ranges shown here. | |
In general, more complex algorithms can be more responsive to QSD inputs, but the complexity of an algorithm does not inherently correlate with usefulness or accuracy. As the algorithm becomes more mechanism-driven and complex, it may require additional or larger datasets for calibration, validation, and prediction, which may not be readily available. Its accuracy may also be affected by parameter uncertainty and missing mechanisms that introduce bias and skew results, and the increased complexity will increase demand for computational resources. For example, one study used a complex model for risk evaluation of radioactive waste disposal composed of 286 sub-models and thousands of parameters, but its utility was undermined by the large uncertainty of a single key variable.79 In contrast, the Arrhenius equation, an empirical equation describing the correlation between a reaction rate constant and temperature, consists of only two reaction-specific parameters, yet it is arguably one of the most widely used models in thermodynamics.
For technologies with a TRL/MRL of only 1–2 (observation of basic principles and formulation of concept), theoretical values or “back-of-the-envelope” estimations can be used. For example, a 100% thermodynamic conversion efficiency could be used for mass flow simulation,80 and industry averaged capital81 or utility82 costs from a similar technology could be used as an early estimate for capital and operating costs. If “proof-of-concept” studies have been conducted (TRL/MRL 3–4), existing designs or experimental data can be used for more realistic design and simulation of the system. When more designs and data become available with prototype implementation (TRL/MRL 5–7), engineering heuristics (e.g., the choice of separation technology, typical reactor aspect ratios83) and empirical models can be used to establish more mathematical connections between QSD inputs and the system inventory. For technologies with higher TRL/MRL (7+), these connections may be able to be mathematically represented based on first principles using mechanistic models in design and process algorithms. As examples, electricity consumption of a pump can be calculated using fluid flowrate, total dynamic head, and pump efficiency,46 and biomass growth can be modeled using metabolic reactions and fluxes.84 Notably, regardless of the level of complexity of the selected algorithms, uncertainty of the algorithm inputs and parameters should be considered; this uncertainty could be particularly important for early-stage technologies where the algorithms often rely on estimations, theoretical values, or very limited experimental data.
Overall, the complexity of design and process algorithms will dictate the connections (or the lack thereof) among specific QSD inputs and the system inventory, the latter of which directly influences system sustainability and the types of insight that can be generated. Consequently, the objectives of a QSD study can also inform the selection of algorithms. For example, when the objective is to evaluate a technology's site-suitability (e.g., sanitation-based resource recovery systems19,47,85), it will be essential to use a model which incorporates geo-spatial data and corresponding contextual parameters, but complex algorithms with many technological parameters may not be necessary. Alternatively, when the objective is to identify and elucidate the sustainability drivers for research prioritization (e.g., electrode specific capacitance and contact resistance in capacitive deionization technologies86), mechanistic algorithms can provide more pertinent insight.
4. Characterize system sustainability
After generating the system inventory, the next step in QSD links the mass and energy flows across the system's life cycle with quantitative sustainability indicators, the values of which can be used to track the progress toward or away from the stated project goals.12 This section discusses techniques to characterize system sustainability with respect to economic, environmental, human health, and social dimensions. These four categories are adapted from the tripartite conception of sustainability (economic, environmental, and social dimensions87), explicitly considering human health as its own dimension to highlight its significance in the context of environmental technologies and its distinct characterization techniques. Discussion of these four dimensions will focus on the steps of sustainability characterization including indicator selection, indicator evaluation, and (optionally) indicator prioritization. In the first step, sustainability indicators are selected based on the objectives of the QSD study and availability of the resources (e.g., data). Next, impact of the system inventory on each indicator is quantified. Finally, if desired, the indicators within each dimension can be ranked or aggregated (e.g., through weighted sum) to prioritize critical indicators and reduce the number of indicators from which to draw insight.
4.1. Economic
Economic analysis is an essential component of QSD for systems where costs often govern decision-making. Traditional cost and profitability indicators, such as investment cost, operating and maintenance costs, payback period, return on investment (ROI), and net present value (NPV), are commonly used in the literature.52,83,88,89 To compare products that are of equivalent utility but that were generated from different technologies,90 indicators such as levelized cost or minimum selling price (MSP) are often used. Both indicators quantify the average net present cost of a product over a system's entire lifetime, but they are typically used in different contexts: levelized cost is usually used in the context of energy (e.g., electricity)91 and (more recently) water92 systems, whereas MSP is often used in the context of biorefineries.52 The selection (and prioritization) of economic indicators will depend on stakeholder preferences. For instance, technology developers may focus on levelized cost to compare against benchmark technologies (i.e., the conventional technologies against which they are competing)62,91,93 while technology adopters (e.g., water or wastewater utilities) may focus on payback period.94,95
To obtain the values for these indicators, life cycle costing (LCC; e.g., ref. 46, 96 and 97) and techno-economic analysis (TEA; e.g., ref. 98–100) are two of the most commonly used techniques for economic indicator evaluation during technology development. Both techniques rely on the system inventory generated by system simulation. After linking those data to unit prices of cost inventory items, additional costs (e.g., labor, tax, insurance) are included with revenues from co-products (e.g., recovered nutrients) to calculate indicators such as the NPV of the system. During these calculations, costs and revenues in future years are discounted (using an interest rate) to account for the time value of money.83 In the case of LCC, costs and revenues over the lifetime of the system are converted to a common time (e.g., present value,101 annual value102) based on the preferences of decision-makers.103–105 LCC generally centers itself on one or more actors (e.g., producer, consumer, waste management operator), which should be chosen based on the objectives of the QSD study.51 LCC results can be reported for the project as a whole, or they can be normalized to the defined functional unit (a reference unit to quantify the performance of a system49,50). In contrast, TEA is typically used to determine the financial viability of the system through selling of the generated product(s), including the potential for acceptable risk and ROI.83 Specifically, a discounted cash flow rate of return analysis is often used to calculate a product's breakeven point, which is the point where the equivalent value of the sum of all cash flows at the base cost year equals zero (i.e., NPV = 0). At the breakeven point, the product cost or selling price is referred to as the leveraged cost or the MSP, and the discount rate is referred to as the internal (or investor's) rate of return (IRR).83 Appropriate IRR targets are typically set according to industry-specific standards based on the type of technology, the stage of development, and the level of risk associated with investment. For example, a 10% IRR is commonly used for a mature industry,98,106,107 but higher IRRs (e.g., 10–20%) may be required for projects with emerging technologies, and IRRs below 10% may be acceptable for lower risk ventures (e.g., infrastructure debt).108,109
In addition to LCC and TEA, cost–benefit analysis (CBA) is also a technique used for economic sustainability evaluation, but it is more often used for policy assessment (by considering external costs and benefits that are incurred by parties not directly involved) than technology development. For example, the U.S. Environmental Protection Agency has undertaken thousands of CBA analyses for relevant policies (e.g., the Clean Water Act).110 Therefore, discussions in this review will focus on LCC or TEA given their relevance to technology RD&D.
4.2. Environmental
To align societal development with the maintenance of resilient and accommodating environmental systems, it is critical to limit the environmental footprints of human activities within the “safe operating space” of planetary boundaries.111–114 Indicators in the environmental dimension can be generally categorized into ecosystem quality (e.g., eutrophication), resource scarcity (e.g., fossil resources), and human health (e.g., particulate matter). Many techniques, including life cycle assessment (LCA),49,50 eco-efficiency assessment,115 and environmental performance evaluation,116 have been developed for environmental management. Among these techniques, LCA is the most comprehensive and widely used to quantify the environmental impacts of a product (including goods and services)49,50 system throughout its life cycle.
LCA is comprised of four phases including goal and scope definition, inventory analysis, impact assessment, and interpretation.49 In the context of QSD, the first phase of goal and scope definition is realized through the definition of the problem space (step 1 of QSD), and the LCA can be tailored to include the life cycle stages of interest (e.g., cradle-to-grave, cradle-to-gate, cradle-to-cradle).49,50,117 For the last phase of interpretation, results from LCA are often discussed together with those from other dimensions, thereby providing a comprehensive evaluation of the system that considers trade-offs among these dimensions and potential improvements to the analysis (section 5.3).
Particularly relevant to sustainability characterization are the inventory analysis and life cycle impact assessment (LCIA) phases. For inventory analysis, different approaches can be used and LCA can therefore be classified as economic input–output LCA (EIO-LCA), process-based LCA, or hybrid LCA which combines the two.118–121 In QSD, process-based LCA is typically used as it is capable of characterizing the environmental implications associated with changes in QSD inputs. An additional distinction among LCAs is whether they are attributional or consequential, where attributional LCA focuses on the flows to/from the environment and consequential LCA focuses on how the flows may change in response to decisions.77,122 Either attributional or consequential LCA may be used in QSD, and the selection of one (over the other) should be based on the desired insight. Furthermore, there are a number of forward-looking LCA approaches focusing on emerging technologies, including prospective (model the technology in a future, more developed phase), ex ante (prior to the market introduction of the technology), dynamic (consider the dynamics of QSD inputs over time), anticipatory (engage stakeholders and emphasize on uncertainty), and combinatorial (consider alternative technologies) LCA.123–127 Despite the differences among these approaches, they can all be used to guide the RD&D decisions of a new technology before it is commercially implemented, with the goal of ensuring it is environmentally competitive or advantageous to the incumbent technology mix. Additionally, all relevant approaches require the system inventory be compiled from the foreground system to execute the inventory analysis, and converting the system inventory to the life cycle inventory (LCI) by considering impacts incurred in the background.54 For example, in operating a membrane reactor, the system inventory could include a specified number of membrane, while the LCI would include all mass and energy flows associated with these membrane modules across their life cycle.46
With regard to the life cycle impact assessment (LCIA) phase, many methods have been developed in recent years (e.g., ReCiPe,128,129 TRACI130–132). These LCIA methods provide characterization factors, which translate every individual emission or raw material requirement in the LCI into normalized, quantitative environmental impacts. These characterization factors can be developed at the midpoint or endpoint of the environmental impact cause-effect chain. While the midpoint characterization factors are developed for a particular impact indicator (e.g., 100-year global warming potential), they can be aggregated into endpoint characterization factors representing different impact categories (e.g., ecosystem quality, human health).129,133 In this process, varying groups of assumptions (e.g., time horizon) may be adopted to reflect the uncertainties and choices associated with these characterization factors.129 For example, based on the hierarchist perspective in ReCiPe, methane has a midpoint characterization factor of 34 kg CO2 eq. per kg−1 for 100-year global warming potential, which can be further translated to the human health endpoint using a factor of 9.28 × 10−7 disability-adjusted life years (DALYs) kg CO2 eq.−1.129
Finally, three optional elements in LCA – normalization, grouping, and weighting – can be included in the LCIA phase if their application is consistent with the goal and scope of the LCA. Specifically, normalization compares the magnitude of indicator results to reference values, grouping sorts and ranks the impact categories, and weighting converts and aggregates indicator results across impact categories by weighting categories relative to each other.50 With these elements, one may represent the environmental sustainability of a system with a single score, which can be easily compared across a range of systems in decision-making. However, as the scores are directly affected by subjective choices during normalization, grouping, and weighting, uncertainty and sensitivity analyses should be included to consider the impacts of these subjective decisions on final scores. Additionally, stakeholders should be engaged throughout this process to reflect their priorities in the final indicator values (section 4.4).
4.3. Human health
While global human health-related impacts can be quantified with LCA (e.g., carcinogenics and non-carcinogenics in TRACI130–132), LCIA methods often have toxicity models (e.g., USEtox in TRACI130–132) and embedded assumptions about the environmental fate of contaminants, human exposure, and human health effects (including dose response relationships).134 Consequently, the human health category within LCA includes modeled impacts spread over large spaces and time scales, and these “averaged” impacts do not explicitly consider localized effects from chemicals or pathogens that may be particularly relevant to project stakeholders. Therefore, when local health risks from exposure to chemical and microbial hazards are of concern, impact indicators (e.g., benchmark quotient of risk level135) should be selected and evaluated with corresponding techniques (e.g., QRA). In the human health dimension, quantified indicators often include probability of infection, probability of illness, or DALYs, all of which can be calculated from one another with certain assumptions. Additionally, there have been methods developed to calculate monetized indicators based on the contribution of pollutants to mortality and the value of a statistical life (VSL; e.g., human health damage from freight transportation136). However, this approach has not been widely adopted in the development of environmental technologies due to its shortcomings and controversies (e.g., how VSL is estimated).137–139
To quantify human health indicators, QRA can be performed following the steps of hazard identification, exposure assessment, dose response, risk characterization, and risk management.55,140 More specifically, to assess local human health risk due to pathogens, QSD inputs and process algorithms can be used to simulate pathogen concentrations, after which quantitative microbial risk assessment (QMRA)141 can be performed to quantify the human health risk. QMRA can be performed with generic (e.g., using generic pathogen concentrations in feces for evaluating water and sanitation systems142) or site-specific data (e.g., ref. 143–145 and example 2 (ref. 143) in Table 2). Additionally, there are examples where QMRA is hybridized with LCA56,146,147 to characterize trade-offs between human health risk and environmental impacts.148–150 Similarly, when health risk related to chemicals (e.g., contaminants of emerging concern) is the focus, quantitative chemical risk assessment (QCRA)151 can be applied.135 To facilitate the risk assessment, quantitative structure models (e.g., quantitative structure–activity relationship, QSAR;152 quantitative structure property relationship, QSPR153) can be used to predictively model the properties (e.g., toxicity, albeit with significant uncertainty154) of interest, and certain semi-quantitative techniques (e.g., CHEM21 guide155 as applied in ref. 156) can be used as a screening strategy to exclude technologies that have significant safety concerns.
4.4. Social
Social sustainability is generally intended to capture how stakeholders (e.g., technology users, regulators, governments) perceive and interact with technologies, which are often critical considerations to enable sustained adoption and long-term success of a project.9,157 Social sustainability is often neglected in sustainability analyses,158 partly stemming from the lack of widely used indicators and/or standardized evaluation techniques.159,160 Social life cycle assessment (SLCA) is one of the most developed techniques with guidelines161 and methodological sheets162 providing detailed instructions for indicator selection, data availability and collection methods.163,164 Nonetheless, the application of SLCA has been limited (in part) due to the challenges associated with data collection and the qualitative nature of social indicators.165 Though proxies (e.g., transparency, income level166) may be used to quantitatively represent qualitative social indicators, these approaches cannot fully capture the unique aspects of social sustainability (e.g., highly site-specific, unequal impacts on different societal groups).
To address these challenges, stakeholders can be engaged throughout the indicator selection, evaluation, and prioritization steps. When, how, and which stakeholders are engaged depends on the objectives of the QSD study and resource availability, and the engagement methods can range from low to high stakeholder input and influence (corresponding to the least to the most resource intensive). In the indicator selection step, indicators can be identified by domain experts (the lowest stakeholder input and influence option),167,168 selected or supplemented by stakeholders from pre-generated options or “master lists”,169–171 or generated by stakeholders through focus groups, photovoice, and interviews (the highest stakeholder input and influence).172,173
Likewise, during the indicator evaluation step, stakeholders can be engaged through data collection methods of varying stakeholder input and influence. For instance, some structured approaches rely on quantitative data from existing census data or with close-ended surveys (e.g., user cost,174–177 number of annual meetings,177,178 number of jobs167,179,180). These approaches are common in the technology development literature because they are often less resource intensive and enable data collection across multiple contexts with limited incremental cost (i.e., the cost of gathering data from another location or context is low).181–184 In contrast, approaches such as semi-structured interviews and case studies185 are more open to stakeholder elaboration, and they are therefore more resource intensive.
Finally, when needed, stakeholders can also be engaged in the indicator prioritization step. This can be performed in conjunction with MCDA to generate a single score or recommendation. When aggregating indicators, their weights can be determined by experts (the lowest stakeholder input and influence option),186 derived from archetypal schemes representing different societal groups,187 or developed for the specific study by stakeholders that directly interact with the technology (the highest stakeholder inputs and influence option).188,189
Overall, as each stakeholder engagement method has limitations, the selection of stakeholders should be a careful process that balances resource requirements, social dynamics, and stakeholder representation. For example, the focus group approach, while requiring less resources than interviewing each of the individuals, may not reflect individual goals190 and can suppress marginalized voices,191 resulting in a lack of comprehensive goals,172,192 influences,193 and decision criteria.194 Ultimately, substantively addressing social sustainability necessitates the engagement of experts from relevant domains (e.g., the social sciences and humanities) and, when explored in a specific deployment context, local stakeholders who will be directly or indirectly impacted by the project.
5. Execution and applications
5.1. Tools for QSD execution
5.1.1. System simulation.
To execute QSD, the first step is to simulate the system and generate the system inventory, for which multiple types of tools can be used. On the simpler end of the spectrum, designs from existing literature can be used and system inventories can be scaled from the literature design (e.g., scaled based on key flowrates). In this case, spreadsheets81 or programming languages (e.g., R,195,196 Python76,197) can be used for inventory scaling, and this process can be automated through spreadsheet built-in functions (e.g., Microsoft Excel Macros198,199) or add-in applications (e.g., Crystal Ball®,200 SimVoi®201). Though straightforward, this strategy relies on existing designs with limited capacity to accurately reflect the intrinsic correlations between QSD inputs and system sustainability. Additionally, as mass and energy flows within the system would be scaled using generic algorithms (often linear correlations) rather than being solved analytically or numerically to convergence, the results could be inaccurate as values of the QSD inputs diverge from the existing design. Therefore, this strategy is generally only appropriate for very preliminary evaluations or for the evaluation of relatively mature technologies for which well-tested scaling algorithms are available.
On the opposite end of the spectrum, high-fidelity, commercial process simulators (e.g., GPS-X™,202 BioWin,203 Aspen Plus®,204 SuperPro Designer,205 AnyLogic206) with rigorous design and process algorithms have been widely used for system design and simulation, especially for large-scale, multi-unit process systems (e.g., wastewater treatment trains,207,208 bio- or chemical refineries209–211). Supported by commercial companies, these simulators are well-tested and capable of solving complex algorithms, allowing deeper investigation into the dynamics among the QSD inputs, system inventory, and sustainability. However, because of their proprietary nature, these simulators are often less accessible and transparent. They may have also limited flexibility to evaluate early-stage technologies due to the lack of corresponding unit operations and associated design and process algorithms.52 Moreover, many of these simulators have no or limited capacity for advanced statistical analyses (e.g., global sensitivity analysis beyond correlation and regression methods). Thus, they often cannot independently fulfil the objectives of QSD studies.
Notably, in recent years, there has been a push to develop open-source tools for design and simulation of various systems in fields such as water and wastewater,212,213 waste valorization,214,215 chemical engineering,197,216–218 green chemistry,219,220 and transportation.221 Their open-source nature allows these tools to be freely used and continuously developed by the general community, and some of these tools have integrated (or are built with extendable capacities for) advanced statistical analyses, sustainability characterization, and multi-objective optimization (e.g., ref. 197 and 213). These features are especially beneficial for early-stage technologies which have not been included in commercial simulators and with higher levels of uncertainties due to the lack of data. However, despite their advantages, these open-source tools can be difficult to adopt (e.g., due to the lack of graphic user interfaces) and challenging to maintain without a central supporting organization. Therefore, laying the groundwork for community-led platforms is vital to the long-term success of these tools, which could be realized via collaborative platforms (e.g., GitHub,222 GitLab223) and preparation of easy-to-access documentation. Additionally, to support broader community engagement, it is beneficial if the developed open-source packages do not rely on commercial platforms (e.g., the waste-to-energy system simulation model WESyS relies on STELLA,214 a set of open-source wastewater treatment models rely on MATLAB and Simulink224).
5.1.2. Sustainability characterization.
Characterization of sustainability can be performed either within or outside the system simulation platform. For economic sustainability, capital and operating expenditures can often be extracted from the commercial simulators, and some of these simulators have functionalities for profitability and cash flow analyses. However, these built-in functionalities often have little or no support for users to adjust parameter values (e.g., equipment and chemical costs). Therefore, additional analyses are often conducted outside these simulators to explore an expanded QSD problem space (e.g., using spreadsheets210).
With regard to environmental sustainability, dedicated LCI databases (e.g., ecoinvent225) are often used to translate the system inventory into the system LCI, after which different LCIA methods can be used to quantify the environmental sustainability of the system. To streamline this process, environmental sustainability is often characterized using specific tools (e.g., SimaPro,226 GaBi,227 openLCA,228 Brighway2,229 GREET230). These tools generally have embedded LCI databases and/or allow the importing of external LCI databases, and some of them are equipped with built-in functions or mechanisms (e.g., via inter-process communication228) that allow the user to account for uncertainty.
To characterize human health indicators, relevant system inventory data (e.g., pathogen concentration), exposure assessment algorithms, and dose response models can be compiled using generic computation tools (e.g., spreadsheet, programming languages). Particularly for QMRA, recommendations by the Center for Advancing Microbial Risk Assessment231 can be followed to select pathogen-specific dose response parameters based on the type of the model (e.g., exponential, approximate beta-Poisson).
For the characterization of social sustainability, no universal tools have yet been developed for its evaluation. Though some LCA tools (e.g., SimaPro, openLCA, SEEbalance®232,233) include databases such as the Social Hotspots Database234 for SLCA, the primary goal of this approach is to evaluate social risks along a supply chain without including stakeholder input.235 These tools can facilitate indicator evaluation, but engagement methods described in this work should be followed to more holistically integrate social sustainability into QSD by incorporating stakeholder input across the indicator selection, evaluation, and prioritization steps (section 4.4).
As a range of tools may be used for system simulation and sustainability characterization, data organization and transfer between these tools can be challenging due to the heterogeneity in data requirements (e.g., file format), despite the fact that the same system inventory is used.236 For example, for a wastewater-based microalgal cultivation system that recirculates water internally, Aspen Plus® was used for system simulation, after which the system inventory was exported for TEA in a spreadsheet and LCA using SimaPro.237,238 Although programming languages can be used to facilitate data formatting and transfer, this nonetheless presents challenges in QSD execution when system simulation and sustainability characterization need to be repeated thousands of times or more to consider uncertainty (section 5.2). Alternatively, there are ongoing efforts in tool development to integrate system simulation and sustainability characterization on a single, open-source platform (e.g., for sanitation and resource recovery systems,213 biorefineries197). Though still at the early stage, these tools offer the opportunity for streamlined QSD execution with much greater flexibility, better consistency, and higher computational efficiency, all of which are critical to QSD and its application.
5.2. Accounting for uncertainty
5.2.1. Sources of uncertainty.
At its core, QSD relies on an aggregated, computational system model to represent the behaviors of physical technologies. This system model is compiled by connecting the algorithms used in system simulation and sustainability characterization to predict the quantities of interest across a range of QSD inputs. Prediction uncertainty of this system model can arise from multiple sources: (i) model structure, (ii) system non-deterministic behaviors, (iii) numerical error, and (iv) model inputs and parameters.239 Among these sources, the uncertainty of model structure can be considered by empirically comparing or aggregating the predictions of multiple viable models; the uncertainty due to a system's non-deterministic behaviors can be characterized by incorporating stochastic elements into a deterministic model; and the numerical error in calculating model results is usually much smaller than other sources of uncertainty.239
Consequently, most essential to QSD is the uncertainty from model inputs and parameters (i.e., QSD inputs), which can be aleatory, epistemic, or both. Aleatory uncertainty (also called variability or irreducible uncertainty) arises from randomness or variations due to “hidden” factors that are not included in the model. Epistemic uncertainty (i.e., true uncertainty, reducible uncertainty), on the other hand, derives from a lack of knowledge of the “true” values.240–244 Uncertainty associated with decision variables are inherently aleatory as their values are subject to the choice of the designer or the operator, and probability distributions can be used to reflect the designer's or operator's preference within the ranges of feasible decisions. In contrast, uncertainties associated with technological parameters and contextual parameters can be caused by randomness (aleatory), lack of knowledge (epistemic), or both. For example, a location's average temperature on a certain date in the future (a contextual parameter) is random to some extent (i.e., aleatory), and at the same time, practitioners may have imperfect knowledge of its “true” range of variability based on historic data (i.e., epistemic). For LCA, uncertainty may also come from the background systems (e.g., the LCI of the stainless steel), and more accurate (or localized) LCI data are needed to reduce the uncertainty.
5.2.2. Monte Carlo methods.
To quantitatively characterize the overall uncertainty introduced in QSD results, Monte Carlo methods are usually applied by using stochasticity (i.e., randomness) to solve problems that are deterministic in nature.58 In the context of QSD, the first step is to select a subset of QSD inputs to be included as input variables in the uncertainty analysis, after which their probability distributions are defined (e.g., through probability density functions) to quantitatively represent their uncertainties. While including more QSD inputs in the Monte Carlo simulation will likely provide more accurate characterization of the overall uncertainty of QSD outputs (i.e., sustainability indicators), it will also increase the required sample size and thus computational time. To address this, sensitivity analysis can be used to identify input variables that are key drivers of system uncertainty (section 5.3). Notably, as the selection of uncertain input variables can affect results of the sensitivity analysis, uncertainty and sensitivity analyses may be performed iteratively to narrow the input variable pool. Similarly, selection of the probability distributions should be based on the available information on the QSD inputs (e.g., the abundance and reliability of the collected data). To maintain consistency and transparency in this process, a workflow or a set of standard criteria is recommended to define which inputs will be included/excluded and the included inputs' associated probability distributions (e.g., as in ref. 245 and 246). Additionally, in LCA, when there are limited life cycle inventory data available, the pedigree matrix approach247–249 can be used to characterize the background uncertainty based on a semi-quantitative description of the data quality.
Next, a set of samples (i.e., the sample matrix) are generated from the joint probability distribution of all selected input variables to represent the entire problem space. When all input variables are independent of each other, generating samples from their joint probability distribution is equivalent to sampling separately from the probability distribution of each individual model input. Otherwise, correlations between input variables need to be characterized to properly define and generate samples from their joint probability distribution.250 Different techniques can be used for sample generation, and the selection of technique is often determined by the types of uncertainty and sensitivity analyses of interest (section 5.3). In practice, Monte Carlo sampling (i.e., random sampling) generates samples by repeatedly drawing random values from a defined distribution using random number generators.59 Alternatively, low-discrepancy, quasi-random sequences (e.g., Latin hypercube sampling,60 Sobol sequence251) can be used to significantly reduce the sample size (and thus the number of model evaluations) required to generate representative empirical distributions of model outputs.
With the generated samples, empirical distributions of sustainability indicators can be obtained by repeatedly simulating the system and characterizing its sustainability at each sample point, and descriptive statistics (e.g., 5th, 25th, 50th, 75th, and 95th percentiles for each indicator) can be calculated from these empirical distributions. With a representative set of samples, these distributions and the descriptive statistics can reflect the expected values and variations of the chosen sustainability indicators due to the uncertainties in QSD inputs, thus avoiding the “false accuracy” from a single simulation that only represents one point in the problem space.
Ideally, aleatory uncertainty and epistemic uncertainty should be propagated through the model separately.252 However, such separation is costly and not always possible. Nonetheless, this distinction should be reflected in the interpretation of the uncertainty and sensitivity analysis results.242 For example, when the prediction uncertainty of system performance is largely attributed to a lack of knowledge of the true value of a technological parameter, research should focus on reducing the epistemic uncertainty of this parameter (e.g., through more accurate experimental measurement to narrow the possible range of its true value). On the other hand, if decision variables are found to be the driver of the variation in the predicted system performance, development of the technology should focus on optimizing the design and operation decisions to limit the aleatory uncertainty.
5.3. Representative applications
5.3.1. Uncertainty analysis to prioritize technology targets.
Uncertainty analysis is the most straightforward application of the Monte Carlo methods as it involves minimal pre-simulation sample selection and post-simulation statistical computation. With uncertainty analysis, one can characterize sustainability indicators, identify the drivers of system sustainability, and set targets for future research and development.19,62,99,245,246,253–256
To summarize the results from Monte Carlo simulation, one can first characterize the sustainability indicators by reviewing their distributions and descriptive statistics of interest, which can help to understand the variability of these indicators and the likelihood of achieving a specific target (e.g., carbon-neutral or negative,257Fig. 3A; Fig. 1 of example 2 (ref. 143) in Table 1). Notably, to compare the sustainability of different technologies, joint Monte Carlo simulation (where the difference in indicator values between technologies is calculated for each set of input variables), rather than independent Monte Carlo simulation of each technology, should be performed to avoid the misinterpretation of overlap areas as indistinctive results (refer to Müller et al.80 for illustrative examples).
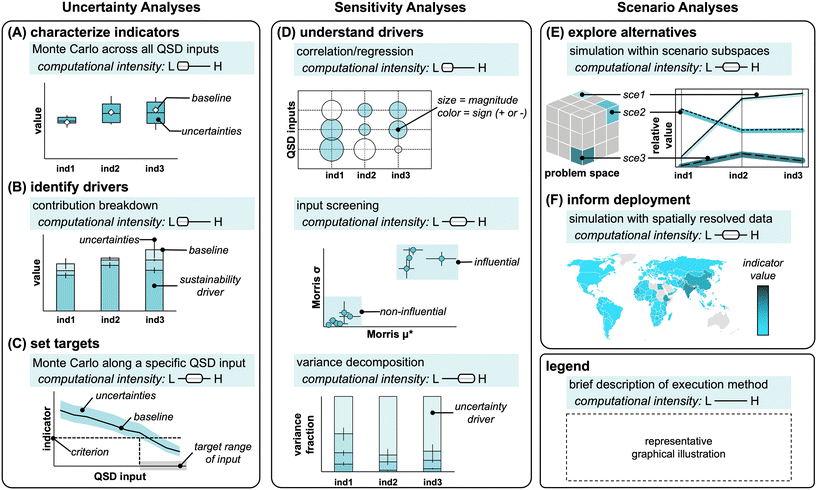 |
| Fig. 3 Representative applications of QSD including (A–C) uncertainty, (D) sensitivity, and (E and F) scenario analyses. Analyses are shown with a brief description of the execution method, the typical computational intensity, and a representative graphical illustration. Note that the computational intensity for each graph herein is solely for comparative purposes. In practical application, it depends on multiple factors (e.g., algorithm complexity, error tolerance, sample size) and could deviate from the typical range shown here. | |
To identify the drivers of system sustainability, values of key sustainability indicators (e.g., MSP) can be attributed across different sources (e.g., equipment, material, labor) based on their contributions (Fig. 3B). Given that sources with the largest contributions exert the most influence toward indicator values, they may be prioritized in RD&D to advance system sustainability. For example, because feedstock costs can account for roughly half (or more) of the MSP of algal biofuels, strategies to reduce the production cost of algae (e.g., development of high-productivity strains) should be prioritized.198,199,258,259
Finally, uncertainty analysis can also be conducted to set targets for parameter values, which are particularly relevant to early-stage technologies (Fig. 3C). This can be achieved by conducting a Monte Carlo simulation at each value (or each step point within the potential range) of the parameter of interest. For instance, when studying the catalytic treatment of spent ion exchange brines for nitrate removal, one key technological parameter could be the number of brine use cycles. Monte Carlo simulation can be executed at different numbers of brine use cycles to explore the minimum number of cycles required for the catalytic technology to be more sustainable than the conventional technology.253 Technological advancements that lead to a larger use cycle forms the opportunity space for this catalytic treatment technology, where it outcompetes the conventional alternative.
5.3.2. Sensitivity analysis to understand uncertainty drivers.
To understand what is driving the observed uncertainty in sustainability indicators for the system, sensitivity analysis is often used to apportion the uncertainty in the model outputs to different sources of uncertainties in the model inputs (i.e., the “effects” of the inputs49). The results of sensitivity analyses are represented as input sensitivity indices for each output of interest. In other words, for each model output, all uncertain model inputs will have one or more sensitivity index values to represent their relative importance to that output's uncertainty (Fig. 3D).260 Sensitivity analysis can be classified as local or global based on whether the effects of input variables are evaluated in the vicinity of a base point (local) or across the entire problem space (global).261,262 Local methods can be used for quick assessment of parameters' impact on the system's sustainability (e.g., with single-point sensitivity analysis at the minimum/maximum values of a parameter263,264), but global methods are recommended when possible because they are more robust and can be more informative (e.g., yielding more generalizable insight across the problem space).44,265 In QSD, global sensitivity analysis can be applied to facilitate research prioritization (e.g., to achieve sustainability targets), model improvement (e.g., to simplify models and reduce computational intensity), and data collection (e.g., to narrow probability distributions).46,74,144,266–272
Specifically, correlation- or regression-based methods (e.g., Spearman ranking;273 Fig. S8–S10† of example 1306 in Table 1) are the least computational intensive and sufficient for monotonic models, where “monotonic” indicates that the model output increases or decreases monotonically with individual model inputs over their ranges of uncertainty (e.g., water treatment plant annual material cost always increases with the increasing unit price of an input chemical).260 These methods are often performed in tandem with uncertainty analysis (i.e., using the results generated from the uncertainty analysis) as they do not require specific sampling techniques, and have been widely used sustainability analyses to highlight the significance of technological advancement or data collection (e.g., ref. 46, 144, 270 and 272).
Screening or variance-based methods are more robust for complex models as they do not rely on assumptions about the model structure (i.e., monotonic or not). Screening methods (e.g., Morris one-at-a-time274) are used to identify non-influential inputs that can be fixed at given values within their uncertainty ranges without significantly reducing the output variance.260 These methods are typically used in a qualitative manner (i.e., values of the sensitivity indices are only for comparative purposes), and smaller sample sizes are typically used to reduce model complexity by fixing non-influential inputs. In one study, for example, the Morris method was used to determine the most important individual and groups of input variables in estimating the yield of urban water supply systems.275
Unlike screening methods, variance-based methods (e.g., the Sobol method) decompose the variance in model output as the sum of effects associated with the model inputs.276,277 Due to their quantitative nature, variance-based methods are more computationally intensive than screening methods, especially when the quantification of interaction effects is desired. Thus, variance-based methods are often conducted for models with relatively small numbers of inputs or after fixing non-influential inputs. As an example, a variance-based sensitivity analysis was performed in one study to determine that 97% of the variance of a location's suitability for a hazardous waste landfill facility was jointly induced by only three variables, thus allowing the original model to be greatly simplified without compromising its accuracy.266
5.3.3. Scenario analysis to explore scenarios and inform deployment.
For complex systems with a large number of inputs and expansive problem space, scenario analysis can be used to draw conclusions for certain sets of inputs with specific values (i.e., scenarios, Fig. 3E). Each scenario should be based on a coherent and internally consistent set of assumptions about key driving forces (e.g., demographics and socio-economic development) and the relationships among these key driving forces.61 Scenario analysis is often placed in a future setting,278 and scenarios based on different sets of assumptions are often compared to inform decision-making. For example, to mitigate GHG emissions, different scenarios, each consisting of varying technologies, have been evaluated for decarbonization.61 Similar applications of scenario analysis can be found in other topics (e.g., water and wastewater treatment,46,145,279,280 renewable energy,199,254,257,281 waste management215), or for a certain technique (e.g., using different scenarios of electricity mix in LCA to assess the environmental sustainability of electric vehicles282). In essence, scenario analysis divides the entire problem space into discrete, representative sub-spaces. It reduces the dimensionality of the system (fewer or less uncertain inputs) and simulation needs, while providing a detailed understanding of distinct alternatives that may be of particular interest for policymaking or implementation in specific contexts.
When the primary objective of QSD is to inform technology deployment, spatial analysis is often used to account for site-specific parameters that can influence the sustainability of systems (Fig. 3F). At its core, spatial analysis can be considered as a special category of scenario analysis where each deployment site is a scenario, and the implications of deployment site are reflected through the values of contextual parameters that are site-specific. To facilitate spatial analysis, geospatial data that link locality-specific contextual parameters with location information are collected, and they can be further combined with temporal information to reveal the evolution of attributes over time.283 These geospatial data may include physical information (e.g., geological properties,266,284,285 distances,286,287 existing infrastructure288), policies,285 cultural preferences,272 and any other contextual parameters that could serve as QSD inputs (e.g., energy and water unit impacts,289,290 costs and impact characterization factors291). As contextual parameters can be particularly important for the human health and social dimensions of sustainability that are highly site-specific, measures should be taken to ensure the representativeness of these values (e.g., use high stakeholder input and influence methods discussed in section 4.4). To address spatial considerations, geographic information systems (GIS) is often used to capture, organize, calculate, and integrate multiple layers of spatial information into QSD (e.g., assessing spatial co-location of recoverable nutrients and agricultural demands for deployment of nutrient recovery sanitation systems292,293). Further, as uncertainties in these data (e.g., from different resolutions294) can directly affect the conclusions of sustainability analyses, uncertainty analysis is often incorporated in spatial analysis and tailored to the data (e.g., based on the data structure295).
6. Conclusions to guide the pursuit of more sustainable technologies
As society endeavors to pursue more sustainable water, sanitation, and resource recovery systems, a range of new technologies are required to replace existing infrastructure that was built to accomplish narrowly defined functions without considering externalities.23,296 To navigate through the vast opportunity space ahead of us, sustainability analyses must be integrated throughout the RD&D of technologies to ensure a trajectory toward sustainability. In support of this integration, we reviewed existing literature on the sustainability analyses of water/wastewater and broader environmental technologies and synthesized our findings into a structured methodology – QSD. We introduced QSD as a methodology that addresses four critical challenges in sustainability analyses that had been largely overlooked in the existing literature: (i) the lack of a shared lexicon to standardize terminology for interdisciplinary communication and research consistency; (ii) the lack of dynamic connections among decisions, parameters, and sustainability indicators that can be leveraged to generate the types of insight needed to advance sustainability; (iii) the lack of robust uncertainty and sensitivity analyses, and (iv) the lack of a guide for the planning and execution of sustainability analyses.
Through the discussion of and with examples from the literature, we illustrated how QSD can be leveraged to guide the RD&D of technologies and inform decision-making. With future development of sustainability characterization techniques, QSD can be enhanced to consider crucial but often overlooked areas (e.g., adding impact categories and indicators for biodiversity in LCA297,298). Meanwhile, the development of integrated, agile, open-source tools for sustainability analyses will streamline QSD execution and benefit its adoption across different disciplines.
Finally, the results of QSD can be used to inform decision-making, including in structured methodologies such as MCDA (e.g., quantified sustainability indicators can be used to evaluate, compare, and recommend technologies based on stakeholder priorities as in ref. 290 and 299). Tools and methods developed in the discipline of decision analysis can in turn be used in QSD for indicator selection (e.g., the MCDA Index Tool,300 the Delphi method301) and prioritization (e.g., the analytical hierarchy process [AHP],302 the preference ranking organization method for enrichment evaluations [PROMETHEE] approach303). Moreover, with objective functions established in MCDA, tools developed for sustainability analyses can potentially be expanded to automate the optimization of system design in early-stage research and development,304 or to support stakeholder engagement in transparent planning and design processes.305 Overall, QSD offers the potential to reveal the sustainability implications of technology innovations under a given context, thereby enabling stakeholders at all levels to understand and make informed decisions about the multidimensional effects of technologies, ultimately contributing to the society's transition toward sustainability.
Conflicts of interest
The authors declare that they have no known competing financial interests or personal relationships that could have appeared to influence the work reported in this paper.
Acknowledgements
This publication is based on research funded in part by the Bill & Melinda Gates Foundation. The findings and conclusions contained within are those of the authors and do not necessarily reflect positions or policies of the Bill & Melinda Gates Foundation.
References
- K. M. Kanene, Indigenous practices of environmental sustainability in the Tonga community of southern Zambia, Jamba: J. Disaster Risk Stud., 2016, 8, 7 Search PubMed.
- Y. Li, H. Cheng, R. J. S. Beeton, T. Sigler and A. Halog, Sustainability from a Chinese cultural perspective: the implications of harmonious development in environmental management, Environ. Dev. Sustain., 2016, 18, 679–696 CrossRef.
- M. Kala and A. Sharma, Traditional Indian beliefs: a key toward sustainable living, Environmentalist, 2010, 30, 85–89 CrossRef.
- C. Isendahl and M. E. Smith, Sustainable agrarian urbanism: The low-density cities of the Mayas and Aztecs, Cities, 2013, 31, 132–143 CrossRef.
-
G. H. Brundtland, M. Khalid, S. Agnelli, S. Al-Athel and B. Chidzero, Our common future, 1987 Search PubMed.
-
National Research Council, Our Common Journey: A Transition Toward Sustainability, The National Academies Press, Washington, DC, 1999 Search PubMed.
-
United Nations Department of Economic and Social Affairs, Transforming our world: the 2030 Agenda for Sustainable Development, United Nations, 2015 Search PubMed.
-
National Academies of Sciences, Engineering, and Medicine, Environmental Engineering for the 21st Century: Addressing Grand Challenges, 2018 Search PubMed.
- J. R. Mihelcic, J. C. Crittenden, M. J. Small, D. R. Shonnard, D. R. Hokanson, Q. Zhang, H. Chen, S. A. Sorby, V. U. James, J. W. Sutherland and J. L. Schnoor, Sustainability Science and Engineering: The Emergence of a New Metadiscipline, Environ. Sci. Technol., 2003, 37, 5314–5324 CrossRef CAS PubMed.
-
United Nations, Kyoto Protocol to the United Nations Framework Convention on Climate Change, Kyoto, 1997 Search PubMed.
-
United Nations, Framework Convention on Climate Change (2015) Adoption of the Paris Agreement, United Nations, Paris, 2015 Search PubMed.
- T. M. Parris and R. W. Kates, Characterizing a sustainability transition: Goals, targets, trends, and driving forces, Proc. Natl. Acad. Sci. U. S. A., 2003, 100, 8068–8073 CrossRef CAS PubMed.
-
R. W. Kates, in Sustainability Science: The Emerging Paradigm and the Urban Environment, ed. M. P. Weinstein and R. E. Turner, Springer New York, New York, NY, 2012, pp. 3–19 Search PubMed.
- R. W. Kates and T. M. Parris, Long-term trends and a sustainability transition, Proc. Natl. Acad. Sci. U. S. A., 2003, 100, 8062–8067 CrossRef CAS PubMed.
- R. W. Kates, W. C. Clark, R. Corell, J. M. Hall, C. C. Jaeger, I. Lowe, J. J. McCarthy, H. J. Schellnhuber, B. Bolin, N. M. Dickson, S. Faucheux, G. C. Gallopin, A. Grübler, B. Huntley, J. Jäger, N. S. Jodha, R. E. Kasperson, A. Mabogunje, P. Matson, H. Mooney, B. Moore, T. O'Riordan and U. Svedin, Sustainability Science, Science, 2001, 292, 641–642 CrossRef CAS PubMed.
- M. A. Montgomery and M. Elimelech, Water And Sanitation in Developing Countries: Including Health in the Equation, Environ. Sci. Technol., 2007, 41, 17–24 CrossRef PubMed.
- S. Cairncross, Sanitation in the developing world: current status and future solutions, Int. J. Environ. Health Res., 2003, 13, S123–S131 CrossRef PubMed.
- M. M. Mekonnen and A. Y. Hoekstra, Four billion people facing severe water scarcity, Sci. Adv., 2016, 2, e1500323 CrossRef PubMed.
- H. A. C. Lohman, J. T. Trimmer, D. Katende, M. Mubasira, M. Nagirinya, F. Nsereko, N. Banadda, R. D. Cusick and J. S. Guest, Advancing Sustainable Sanitation and Agriculture through Investments in Human-Derived Nutrient Systems, Environ. Sci. Technol., 2020, 54, 9217–9227 CrossRef CAS.
- C. O. Tuck, E. Pérez, I. T. Horváth, R. A. Sheldon and M. Poliakoff, Valorization of Biomass: Deriving More Value from Waste, Science, 2012, 337, 695–699 CrossRef CAS PubMed.
- P. T. Anastas and J. B. Zimmerman, Design Through the 12 Principles of Green Engineering, Environ. Sci. Technol., 2003, 37, 94A–101A CrossRef PubMed.
- H. C. Erythropel, J. B. Zimmerman, T. M. de Winter, L. Petitjean, F. Melnikov, C. Ho Lam, A. W. Lounsbury, K. E. Mellor, N. Z. Janković, Q. Tu, L. N. Pincus, M. M. Falinski, W. Shi, P. Coish, D. L. Plata and P. T. Anastas, The Green ChemisTREE: 20 years after taking root with the 12 principles, Green Chem., 2018, 20, 1929–1961 RSC.
- J. B. Zimmerman, P. T. Anastas, H. C. Erythropel and W. Leitner, Designing for a green chemistry future, Science, 2020, 367, 397–400 CrossRef CAS PubMed.
- H. Gosnell, N. Gill and M. Voyer, Transformational adaptation on the farm: Processes of change and persistence in transitions to ‘climate-smart’ regenerative agriculture, Glob. Environ. Change, 2019, 59, 101965 CrossRef.
- P. Newton, N. Civita, L. Frankel-Goldwater, K. Bartel and C. Johns, What Is Regenerative Agriculture? A Review of Scholar and Practitioner Definitions Based on Processes and Outcomes, Front. Sustain. Food Syst., 2020, 4, 194 Search PubMed.
-
W. Olson, in Sustainability Science and Engineering, ed. M. A. Abraham, Elsevier, 2006, vol. 1, pp. 91–112 Search PubMed.
- J. Liu, H. Mooney, V. Hull, S. J. Davis, J. Gaskell, T. Hertel, J. Lubchenco, K. C. Seto, P. Gleick, C. Kremen and S. Li, Systems integration for global sustainability, Science, 2015, 347, 1258832 CrossRef PubMed.
- S. A. Matlin, G. Mehta, H. Hopf and A. Krief, One-world chemistry and systems thinking, Nat. Chem., 2016, 8, 393–398 CrossRef CAS PubMed.
- M. M. McAlister, Q. Zhang, J. Annis, R. W. Schweitzer, S. Guidotti and J. R. Mihelcic, Systems Thinking for Effective Interventions in Global Environmental Health, Environ. Sci. Technol., 2022, 56, 732–738 CrossRef CAS.
- R. W. Kates, W. C. Clark, R. Corell, J. M. Hall, C. C. Jaeger, I. Lowe, J. J. McCarthy, H. J. Schellnhuber, B. Bolin, N. M. Dickson, S. Faucheux, G. C. Gallopin, A. Grübler, B. Huntley, J. Jäger, N. S. Jodha, R. E. Kasperson, A. Mabogunje, P. Matson, H. Mooney, B. Moore, T. O'Riordan and U. Svedin, Sustainability Science, Science, 2001, 292, 641–642 CrossRef CAS PubMed.
- W. C. Clark and N. M. Dickson, Sustainability science: The emerging research program, Proc. Natl. Acad. Sci. U. S. A., 2003, 100, 8059–8061 CrossRef CAS.
- C. I. Davidson, H. S. Matthews, C. T. Hendrickson, M. W. Bridges, B. R. Allenby, J. C. Crittenden, Y. Chen, E. Williams, D. T. Allen, C. F. Murphy and S. Austin, Viewpoint: Adding Sustainability to the Engineer's Toolbox: A Challenge for Engineering Educators, Environ. Sci. Technol., 2007, 41, 4847–4849 CrossRef CAS PubMed.
- C. S. Holling, Understanding the Complexity of Economic, Ecological, and Social Systems, Ecosystems, 2001, 4, 390–405 CrossRef.
- L. M. A. Bettencourt and J. Kaur, Evolution and structure of sustainability science, Proc. Natl. Acad. Sci. U. S. A., 2011, 108, 19540–19545 CrossRef CAS.
- T. M. Parris and R. W. Kates, Characterizing a sustainability transition: Goals, targets, trends, and driving forces, Proc. Natl. Acad. Sci. U. S. A., 2003, 100, 8068–8073 CrossRef CAS PubMed.
- W. C. Clark and A. G. Harley, Sustainability Science: Toward a Synthesis, Annu. Rev. Environ. Resour., 2020, 45, 331–386 CrossRef.
- J. S. Guest, S. J. Skerlos, J. L. Barnard, M. B. Beck, G. T. Daigger, H. Hilger, S. J. Jackson, K. Karvazy, L. Kelly, L. Macpherson, J. R. Mihelcic, A. Pramanik, L. Raskin, M. C. M. Van Loosdrecht, D. Yeh and N. G. Love, A New Planning and Design Paradigm to Achieve Sustainable Resource Recovery from Wastewater, Environ. Sci. Technol., 2009, 43, 6126–6130 CrossRef CAS PubMed.
- E. D. Schoolman, J. S. Guest, K. F. Bush and A. R. Bell, How interdisciplinary is sustainability research? Analyzing the structure of an emerging scientific field, Sustain. Sci., 2012, 7, 67–80 CrossRef.
-
P. T. Anastas and J. C. Warner, Green Chemistry: Theory and Practice, Oxford University Press, 2000 Search PubMed.
-
United Nations Environment Programme, Inclusive Wealth Report, 2018 Search PubMed.
- UNEP/SETAC Life Cycle Initiative, Towards a Life Cycle Sustainability Assessment, 2011 Search PubMed.
-
National Academies of Sciences, Engineering, and Medicine, Progress, Challenges, and Opportunities for Sustainability Science: Proceedings of a Workshop in Brief, 2021 Search PubMed.
- A. E. Björklund, Survey of approaches to improve reliability in lca, Int. J. Life Cycle Assess., 2002, 7, 64 CrossRef.
-
A. Saltelli, M. Ratto, T. Andres, F. Campolongo, J. Cariboni, D. Gatelli, M. Saisana and S. Tarantola, Global Sensitivity Analysis: The Primer, John Wiley & Sons, 2008 Search PubMed.
-
National Research Council, Assessing the Reliability of Complex Models: Mathematical and Statistical Foundations of Verification, Validation, and Uncertainty Quantification, 2012 Search PubMed.
- B. D. Shoener, C. Zhong, A. D. Greiner, W. O. Khunjar, P.-Y. Hong and J. S. Guest, Design of anaerobic membrane bioreactors for the valorization of dilute organic carbon waste streams, Energy Environ. Sci., 2016, 9, 1102–1112 RSC.
- J. T. Trimmer, R. D. Cusick and J. S. Guest, Amplifying Progress toward Multiple Development Goals through Resource Recovery from Sanitation, Environ. Sci. Technol., 2017, 51, 10765–10776 CrossRef CAS.
-
P. H. Groggins, Unit Processes in Organic Synthesis, McGraw-Hill Book Company, 1935 Search PubMed.
-
International Organization for Standardization, Environmental management — Life cycle assessment — Principles and framework, International Organization for Standardization (ISO), 2006 Search PubMed.
-
International Organization for Standardization, Environmental management — Life cycle assessment — Requirements and guidelines, International Organization for Standardization (ISO), 2006 Search PubMed.
- G. Rebitzer and D. Hunkeler, Life cycle costing in LCM: ambitions, opportunities, and limitations, Int. J. Life Cycle Assess., 2003, 8, 253–256 CrossRef.
- C. D. Scown, N. R. Baral, M. Yang, N. Vora and T. Huntington, Technoeconomic analysis for biofuels and bioproducts, Curr. Opin. Biotechnol., 2021, 67, 58–64 CrossRef CAS PubMed.
- D. Ratnaweera, A. Heistad and S. Navrud, The current use and potential of cost benefit analysis in water sector projects, Water Sci. Technol.: Water Supply, 2020, 21, 1438–1449 Search PubMed.
-
J. Cays, in An Environmental Life Cycle Approach to Design: LCA for Designers and the Design Market, ed. J. Cays, Springer International Publishing, Cham, 2021, pp. 79–101 Search PubMed.
-
D. J. Smith and K. G. L. Simpson, in The Safety Critical Systems Handbook (Fifth Edition), ed. D. J. Smith and K. G. L. Simpson, Butterworth-Heinemann, 2020, pp. 99–131 Search PubMed.
- R. Harder, G. M. Peters, N. J. Ashbolt and M. Svanström, Using quantitative microbial risk assessment and life cycle assessment to assess management options in urban water and sanitation infrastructures: Opportunities and unresolved issues, Microb. Risk Anal., 2017, 5, 71–77 CrossRef.
-
UNEP/SETAC, Guidelines for Social Life Cycle Assessment of Products, United Nations Environment Programme, 2009 Search PubMed.
- N. Metropolis and S. Ulam, The Monte Carlo Method, J. Am. Stat. Assoc., 1949, 44, 335–341 CrossRef CAS.
-
J. E. Gentle, Random Number Generation and Monte Carlo Methods, Springer Science & Business Media, 2003 Search PubMed.
- M. D. McKay, R. J. Beckman and W. J. Conover, A Comparison of Three Methods for Selecting Values of Input Variables in the Analysis of Output from a Computer Code, Technometrics, 1979, 21, 239–245 Search PubMed.
-
IPCC, Global Warming of 1.5°C, Intergovernmental Panel on Climate Change, 2018 Search PubMed.
- X. Liu, S. Shanbhag, T. V. Bartholomew, J. F. Whitacre and M. S. Mauter, Cost Comparison of Capacitive Deionization and Reverse Osmosis for Brackish Water Desalination, ACS ES&T Eng., 2021, 1, 261–273 Search PubMed.
- S. Gavankar, S. Suh and A. A. Keller, The Role of Scale and Technology Maturity in Life Cycle Assessment of Emerging Technologies: A Case Study on Carbon Nanotubes, J. Ind. Ecol., 2015, 19, 51–60 CrossRef CAS.
- G. A. Buchner, K. J. Stepputat, A. W. Zimmermann and R. Schomäcker, Specifying Technology Readiness Levels for the Chemical Industry, Ind. Eng. Chem. Res., 2019, 58, 6957–6969 CrossRef CAS.
- F. E. Ahmed, A. Khalil and N. Hilal, Emerging desalination technologies: Current status, challenges and future trends, Desalination, 2021, 517, 115183 CrossRef CAS.
- J. Radjenović, M. Petrović, F. Ventura and D. Barceló, Rejection of pharmaceuticals in nanofiltration and reverse osmosis membrane drinking water treatment, Water Res., 2008, 42, 3601–3610 CrossRef PubMed.
- K. Roh, A. Bardow, D. Bongartz, J. Burre, W. Chung, S. Deutz, D. Han, M. Heßelmann, Y. Kohlhaas, A. König, J. S. Lee, R. Meys, S. Völker, M. Wessling, J. H. Lee and A. Mitsos, Early-stage evaluation of emerging CO2 utilization technologies at low technology readiness levels, Green Chem., 2020, 22, 3842–3859 RSC.
- J. Liu, T. Dietz, S. R. Carpenter, M. Alberti, C. Folke, E. Moran, A. N. Pell, P. Deadman, T. Kratz, J. Lubchenco, E. Ostrom, Z. Ouyang, W. Provencher, C. L. Redman, S. H. Schneider and W. W. Taylor, Complexity of Coupled Human and Natural Systems, Science, 2007, 317, 1513–1516 CrossRef CAS PubMed.
- Z. Lu, O. A. Broesicke, M. E. Chang, J. Yan, M. Xu, S. Derrible, J. R. Mihelcic, B. Schwegler and J. C. Crittenden, Seven Approaches to Manage Complex Coupled Human and Natural Systems: A Sustainability Toolbox, Environ. Sci. Technol., 2019, 53, 9341–9351 CrossRef CAS PubMed.
-
EIA, Electric Power Monthly with Data for March 2020, U.S. Energy Information Administration, 2020 Search PubMed.
- L. Lu, J. S. Guest, C. A. Peters, X. Zhu, G. H. Rau and Z. J. Ren, Wastewater treatment for carbon capture and utilization, Nat. Sustain., 2018, 1, 750–758 CrossRef.
-
U.S. Environmental Protection Agency, Emissions & Generation Resource Integrated Database (eGRID), 2018 Search PubMed.
- J. A. de Chalendar, J. Taggart and S. M. Benson, Tracking emissions in the US electricity system, Proc. Natl. Acad. Sci. U. S. A., 2019, 116, 25497–25502 CrossRef CAS.
- X. Zhang, A. Lardizabal, A. I. Silverman, D. Vione, T. Kohn, T. H. Nguyen and J. S. Guest, Global Sensitivity Analysis of Environmental, Water Quality, Photoreactivity, and Engineering Design Parameters in Sunlight Inactivation of Viruses, Environ. Sci. Technol., 2020, 54, 8401–8410 CrossRef CAS.
- A. Murray, A. Horvath and K. L. Nelson, Hybrid Life-Cycle Environmental and Cost Inventory of Sewage Sludge Treatment and End-Use Scenarios: A Case
Study from China, Environ. Sci. Technol., 2008, 42, 3163–3169 CrossRef CAS PubMed.
- R. Shi and J. S. Guest, BioSTEAM-LCA: An Integrated Modeling Framework for Agile Life Cycle Assessment of Biorefineries under Uncertainty, ACS Sustainable Chem. Eng., 2020, 8, 18903–18914 CrossRef CAS.
- L. Corominas, D. M. Byrne, J. S. Guest, A. Hospido, P. Roux, A. Shaw and M. D. Short, The application of life cycle assessment (LCA) to wastewater treatment: A best practice guide and critical review, Water Res., 2020, 184, 116058 CrossRef CAS PubMed.
- P. Vitale, N. Arena, F. Di Gregorio and U. Arena, Life cycle assessment of the end-of-life phase of a residential building, Waste Manage., 2017, 60, 311–321 CrossRef PubMed.
- A. Saltelli, G. Bammer, I. Bruno, E. Charters, M. D. Fiore, E. Didier, W. N. Espeland, J. Kay, S. L. Piano, D. Mayo, R. Pielke Jr., T. Portaluri, T. M. Porter, A. Puy, I. Rafols, J. R. Ravetz, E. Reinert, D. Sarewitz, P. B. Stark, A. Stirling, J. van der Sluijs and P. Vineis, Five ways to ensure that models serve society: a manifesto, Nature, 2020, 582, 482–484 CrossRef CAS PubMed.
- L. J. Müller, A. Kätelhön, M. Bachmann, A. Zimmermann, A. Sternberg and A. Bardow, A Guideline for Life Cycle Assessment of Carbon Capture and Utilization, Front. Energy Res., 2020, 8, 15 CrossRef.
- M. B. Viswanathan, D. R. Raman, K. A. Rosentrater and B. H. Shanks, A Technoeconomic Platform for Early-Stage Process Design and Cost Estimation of Joint Fermentative–Catalytic Bioprocessing, Processes, 2020, 8, 229 CrossRef.
- A. A. Ghatta, J. D. E. T. Wilton-Ely and J. P. Hallett, From sugars to FDCA: a techno-economic assessment using a design concept based on solvent selection and carbon dioxide emissions, Green Chem., 2021, 23, 1716–1733 RSC.
-
W. D. Seider, D. R. Lewin, J. D. Seader, S. Widagdo, R. Gani and K. M. Ng, Product and Process Design Principles: Synthesis, Analysis and Evaluation, Wiley Global Education, 2016 Search PubMed.
- B. D. Shoener, S. M. Schramm, F. Béline, O. Bernard, C. Martínez, B. G. Plósz, S. Snowling, J.-P. Steyer, B. Valverde-Pérez, D. Wágner and J. S. Guest, Microalgae and cyanobacteria modeling in water resource recovery facilities: A critical review, Water Res.: X, 2019, 2, 100024 CAS.
- J. T. Trimmer, A. J. Margenot, R. D. Cusick and J. S. Guest, Aligning Product Chemistry and Soil Context for Agronomic Reuse of Human-Derived Resources, Environ. Sci. Technol., 2019, 53, 6501–6510 CrossRef CAS.
- S. Hand, X. Shang, J. S. Guest, K. C. Smith and R. D. Cusick, Global Sensitivity Analysis To Characterize Operational Limits and Prioritize Performance Goals of Capacitive Deionization Technologies, Environ. Sci. Technol., 2019, 53, 3748–3756 CrossRef CAS PubMed.
- B. Purvis, Y. Mao and D. Robinson, Three pillars of sustainability: in search of conceptual origins, Sustain. Sci., 2019, 14, 681–695 CrossRef.
- A. S. Goffi, F. Trojan, J. D. de Lima, M. Lizot and S. S. Thesari, Economic feasibility for selecting wastewater treatment systems, Water Sci. Technol., 2019, 78, 2518–2531 CrossRef PubMed.
- C. Cossio, J. Norrman, J. McConville, A. Mercado and S. Rauch, Indicators for sustainability assessment of small-scale wastewater treatment plants in low and lower-middle income countries, Environmental and Sustainability Indicators, 2020, 6, 100028 CrossRef.
-
S. Raikar and S. Adamson, in Renewable Energy Finance, ed. S. Raikar and S. Adamson, Academic Press, 2020, pp. 185–220 Search PubMed.
- M. H. Mostafa, S. H. E. Abdel Aleem, S. G. Ali, Z. M. Ali and A. Y. Abdelaziz, Techno-economic assessment of energy storage systems using annualized life cycle cost of storage (LCCOS) and levelized cost of energy (LCOE) metrics, J. Energy Storage, 2020, 29, 101345 CrossRef.
- D. B. Gingerich, T. V. Bartholomew and M. S. Mauter, Technoeconomic Optimization of Emerging Technologies for Regulatory Analysis, ACS Sustainable Chem. Eng., 2018, 6, 2370–2378 CrossRef CAS.
- T. D. Hasseler, A. Ramachandran, W. A. Tarpeh, M. Stadermann and J. G. Santiago, Process design tools and techno-economic analysis for capacitive deionization, Water Res., 2020, 183, 116034 CrossRef CAS PubMed.
-
R. S. Raucher, D. Garvey, K. C. Hallett, J. Henderson and C. Wagner, An Economic Framework for Evaluating the Benefits and Costs of Biosolids Management Options, IWA Publishing, 2007 Search PubMed.
-
R. Latimer and W. Khunjar, Towards a Renewable Future: Assessing Resource Recovery as a Viable Treatment Alternative State of the Science and Market Assessment, IWA Publishing, 2016 Search PubMed.
- O. Kavvada, W. A. Tarpeh, A. Horvath and K. L. Nelson, Life-Cycle Cost and Environmental Assessment of Decentralized Nitrogen Recovery Using Ion Exchange from Source-Separated Urine through Spatial Modeling, Environ. Sci. Technol., 2017, 51, 12061–12071 CrossRef CAS PubMed.
- R. Pretel, B. D. Shoener, J. Ferrer and J. S. Guest, Navigating environmental, economic, and technological trade-offs in the design and operation of submerged anaerobic membrane bioreactors (AnMBRs), Water Res., 2015, 87, 531–541 CrossRef CAS PubMed.
-
R. E. Davis, N. J. Grundl, L. Tao, M. J. Biddy, E. C. Tan, G. T. Beckham, D. Humbird, D. N. Thompson and M. S. Roni, Process Design and Economics for the Conversion of Lignocellulosic Biomass to Hydrocarbon Fuels and Coproducts: 2018 Biochemical Design Case Update, National Renewable Energy Lab. (NREL), Golden, CO (United States), 2018 Search PubMed.
- M. Yang, N. R. Baral, B. A. Simmons, J. C. Mortimer, P. M. Shih and C. D. Scown, Accumulation of high-value bioproducts in planta can improve the economics of advanced biofuels, Proc. Natl. Acad. Sci. U. S. A., 2020, 117, 8639–8648 CrossRef CAS PubMed.
- N. A. Huq, G. R. Hafenstine, X. Huo, H. Nguyen, S. M. Tifft, D. R. Conklin, D. Stück, J. Stunkel, Z. Yang, J. S. Heyne, M. R. Wiatrowski, Y. Zhang, L. Tao, J. Zhu, C. S. McEnally, E. D. Christensen, C. Hays, K. M. Van Allsburg, K. A. Unocic, H. M. Meyer, Z. Abdullah and D. R. Vardon, Toward net-zero sustainable aviation fuel with wet waste–derived volatile fatty acids, Proc. Natl. Acad. Sci. U. S. A., 2021, 118, e2023008118 CrossRef CAS.
- R. Wang and J. B. Zimmerman, Economic and Environmental Assessment of Office Building Rainwater Harvesting Systems in Various U.S. Cities, Environ. Sci. Technol., 2015, 49, 1768–1778 CrossRef CAS PubMed.
- T. S. Bixler, J. Houle, T. P. Ballestero and W. Mo, A spatial life cycle cost assessment of stormwater management systems, Sci. Total Environ., 2020, 728, 138787 CrossRef CAS PubMed.
- P. Gluch and H. Baumann, The life cycle costing (LCC) approach: a conceptual discussion of its usefulness for environmental decision-making, Build. Environ., 2004, 39, 571–580 CrossRef.
- M. M. Sesana and G. Salvalai, Overview on life cycle methodologies and economic feasibility for nZEBs, Build. Environ., 2013, 67, 211–216 CrossRef.
-
S. Yang, K. Ma, Z. Liu, J. Ren and Y. Man, in Life Cycle Sustainability Assessment for Decision-Making, ed. J. Ren and S. Toniolo, Elsevier, 2020, pp. 95–124 Search PubMed.
-
W. Short, D. J. Packey and T. Holt, A Manual for the Economic Evaluation of Energy Efficiency and
Renewable Energy Technologies, National Renewable Energy Laboratory, Golden, CO, 1995 Search PubMed.
-
P. D. Lavappa and J. D. Kneifel, Energy Price Indices and Discount Factors for Life-Cycle Cost Analysis: 2019, National Institute of Standards and Technology, Gaithersburg, Maryland, 2019 Search PubMed.
-
P. Partners, Investing in Infrastructure, 2012 Search PubMed.
-
P. Partners, Infrastructure Institutional Investor Trends: 2019 Survey Results, 2019 Search PubMed.
- D. A. Keiser, C. L. Kling and J. S. Shapiro, The low but uncertain measured benefits of US water quality policy, Proc. Natl. Acad. Sci. U. S. A., 2019, 116, 5262–5269 CrossRef CAS PubMed.
- J. Rockström, W. Steffen, K. Noone, Å. Persson, F. S. Chapin, E. F. Lambin, T. M. Lenton, M. Scheffer, C. Folke, H. J. Schellnhuber, B. Nykvist, C. A. de Wit, T. Hughes, S. van der Leeuw, H. Rodhe, S. Sörlin, P. K. Snyder, R. Costanza, U. Svedin, M. Falkenmark, L. Karlberg, R. W. Corell, V. J. Fabry, J. Hansen, B. Walker, D. Liverman, K. Richardson, P. Crutzen and J. A. Foley, A safe operating space for humanity, Nature, 2009, 461, 472–475 CrossRef PubMed.
- A. Y. Hoekstra and T. O. Wiedmann, Humanity's unsustainable environmental footprint, Science, 2014, 344, 1114–1117 CrossRef CAS PubMed.
- W. Steffen, K. Richardson, J. Rockström, S. E. Cornell, I. Fetzer, E. M. Bennett, R. Biggs, S. R. Carpenter, W. de Vries, C. A. de Wit, C. Folke, D. Gerten, J. Heinke, G. M. Mace, L. M. Persson, V. Ramanathan, B. Reyers and S. Sörlin, Planetary boundaries: Guiding human development on a changing planet, Science, 2015, 347, 1259855 CrossRef PubMed.
- D. W. O'Neill, A. L. Fanning, W. F. Lamb and J. K. Steinberger, A good life for all within planetary boundaries, Nat. Sustain., 2018, 1, 88–95 CrossRef.
-
International Organization for Standardization, Environmental management — Eco-efficiency assessment of product systems — Principles, requirements and guidelines, International Organization for Standardization (ISO), 2012 Search PubMed.
-
International Organization for Standardization, Environmental management — Environmental performance evaluation — Guidelines, International Organization for Standardization (ISO), 2021 Search PubMed.
-
I. V. Muralikrishna and V. Manickam, in Environmental Management, ed. I. V. Muralikrishna and V. Manickam, Butterworth-Heinemann, 2017, pp. 57–75 Search PubMed.
-
Carnegie Mellon University, Approaches to LCA - Economic Input-Output Life Cycle Assessment, https://www.eiolca.net/Method/LCAapproaches.html, (accessed 11 April 2021).
- M. Wakeel, B. Chen, T. Hayat, A. Alsaedi and B. Ahmad, Energy consumption for water use cycles in different countries: A review, Appl. Energy, 2016, 178, 868–885 CrossRef.
-
R. Azari, in Sustainable Construction Technologies, ed. V. W. Y. Tam and K. N. Le, Butterworth-Heinemann, 2019, pp. 123–144 Search PubMed.
- P. Lamers, A. F. T. Avelino, Y. Zhang, E. C. D. Tan, B. Young, J. Vendries and H. Chum, Potential Socioeconomic and Environmental Effects of an Expanding U.S. Bioeconomy: An Assessment of Near-Commercial Cellulosic Biofuel Pathways, Environ. Sci. Technol., 2021, 55, 5496–5505 CrossRef CAS.
- T. Ekvall, A. Azapagic, G. Finnveden, T. Rydberg, B. P. Weidema and A. Zamagni, Attributional and consequential LCA in the ILCD handbook, Int. J. Life Cycle Assess., 2016, 21, 293–296 CrossRef.
- L. B. Brentner, M. J. Eckelman and J. B. Zimmerman, Combinatorial Life Cycle Assessment to Inform Process
Design of Industrial Production of Algal Biodiesel, Environ. Sci. Technol., 2011, 45, 7060–7067 CrossRef CAS PubMed.
- S. Cucurachi, C. van der Giesen and J. Guinée, Ex-ante LCA of Emerging Technologies, Procedia CIRP, 2018, 69, 463–468 CrossRef.
- M. Buyle, A. Audenaert, P. Billen, K. Boonen and S. Van Passel, The Future of Ex-Ante LCA? Lessons Learned and Practical Recommendations, Sustainability, 2019, 11, 5456 CrossRef.
- C. van der Giesen, S. Cucurachi, J. Guinée, G. J. Kramer and A. Tukker, A critical view on the current application of LCA for new technologies and recommendations for improved practice, J. Cleaner Prod., 2020, 259, 120904 CrossRef.
- N. Thonemann, A. Schulte and D. Maga, How to Conduct Prospective Life Cycle Assessment for Emerging Technologies? A Systematic Review and Methodological Guidance, Sustainability, 2020, 12, 1192 CrossRef.
-
M. Goedkoop, R. Heijungs, M. Huijbregts, A. De Schryver, J. Struijs and R. van Zelm, ReCiPe 2008, 2009 Search PubMed.
- M. A. J. Huijbregts, Z. J. N. Steinmann, P. M. F. Elshout, G. Stam, V. Verones, M. Vieira, M. Zijp, A. Hollander and R. van Zelm, ReCiPe2016: A harmonized life cycle impact assessment method at midpoint and endpoint level, Int. J. Life Cycle Assess., 2017, 22, 138–147 CrossRef.
- J. Bare, G. Norris, D. Pennington and T. McKone, TRACI—The Tool for the Reduction and Assessment of Chemical and Other Environmental Impacts, J. Ind. Ecol., 2002, 6, 49–78 CrossRef.
- J. Bare, TRACI 2.0: the tool for the reduction and assessment of chemical and other environmental impacts 2.0, Clean Technol. Environ. Policy, 2011, 13, 687–696 CrossRef CAS.
-
J. Bare, Tool for the Reduction and Assessment of Chemical and Other Environmental Impacts (TRACI), Version 2.1 - User's Manual Search PubMed.
- J. Bare, P. Hofstetter, D. Pennington and H. Haes, Midpoints Versus Endpoints: The Sacrifices and Benefits, Int. J. Life Cycle Assess., 2012, 5, 319–326 CrossRef.
- R. K. Rosenbaum, T. M. Bachmann, L. S. Gold, M. A. J. Huijbregts, O. Jolliet, R. Juraske, A. Koehler, H. F. Larsen, M. MacLeod, M. Margni, T. E. McKone, J. Payet, M. Schuhmacher, D. van de Meent and M. Z. Hauschild, USEtox—the UNEP-SETAC toxicity model: recommended characterisation factors for human toxicity and freshwater ecotoxicity in life cycle impact assessment, Int. J. Life Cycle Assess., 2008, 13, 532 CrossRef CAS.
- B. Cantoni, L. Penserini, D. Vries, M. M. L. Dingemans, B. G. H. Bokkers, A. Turolla, P. W. M. H. Smeets and M. Antonelli, Development of a quantitative chemical risk assessment (QCRA) procedure for contaminants of emerging concern in drinking water supply, Water Res., 2021, 116911 CrossRef CAS PubMed.
- F. Tong, A. Jenn, D. Wolfson, C. D. Scown and M. Auffhammer, Health and Climate Impacts from Long-Haul Truck Electrification, Environ. Sci. Technol., 2021, 55, 8514–8523 CrossRef CAS.
-
F. Ackerman and L. Heinzerling, Priceless: On Knowing the Price of Everything and the Value of Nothing, New Press, 2004 Search PubMed.
-
W. K. Viscusi, Policy Challenges of the Heterogeneity of the Value of Statistical Life, MIC, 2011, vol. 6, pp. 99–172 Search PubMed.
-
J. Colmer, What is the meaning of (statistical) life? Benefit–cost analysis in the time of COVID-19, Oxford Review of Economic Policy, 2020, p. graa022 Search PubMed.
-
C. N. Haas, J. B. Rose and C. P. Gerba, Quantitative microbial risk assessment, John Wiley & Sons, 2014 Search PubMed.
-
C. N. Haas, J. B. Rose and C. P. Gerba, Quantitative Microbial Risk Assessment: Second Edition, Wiley-Blackwell, 2014 Search PubMed.
- A. W. Bivins, T. Sumner, E. Kumpel, G. Howard, O. Cumming, I. Ross, K. Nelson and J. Brown, Estimating Infection Risks and the Global Burden of Diarrheal Disease Attributable to Intermittent Water Supply Using QMRA, Environ. Sci. Technol., 2017, 51, 7542–7551 CrossRef CAS PubMed.
- J. G. Elliott, L. Taylor-Edmonds and R. C. Andrews, Quantitative microbial risk assessments for drinking water facilities: evaluation of a range of treatment strategies, Environ. Sci.: Water Res. Technol., 2019, 5, 1943–1955 RSC.
- D. M. Byrne, K. A. Hamilton, S. A. Houser, M. Mubasira, D. Katende, H. A. C. Lohman, J. T. Trimmer, N. Banadda, A. Zerai and J. S. Guest, Navigating Data Uncertainty and Modeling Assumptions in Quantitative Microbial Risk Assessment in an Informal Settlement in Kampala, Uganda, Environ. Sci. Technol., 2021, 55, 5463–5474 CrossRef CAS PubMed.
- K. D. Orner, E. M. Symonds, H. Madrigal-Solís, R. A. Orozco-Montoya, A. Fonseca-Sánchez, M. E. Verbyla and M. R. Cairns, Holistically Managing Pathogens and Nutrients in Urbanizing Tropical Towns: Can Sanitation Technologies Create Safer Conditions for Beach Recreation?, ACS ES&T Water, 2021, 1, 1184–1197 Search PubMed.
- R. Harder, S. Heimersson, M. Svanström and G. M. Peters, Including Pathogen Risk in Life Cycle Assessment of Wastewater Management. 1. Estimating the Burden of Disease Associated with Pathogens, Environ. Sci. Technol., 2014, 48, 9438–9445 CrossRef CAS PubMed.
- S. Heimersson, R. Harder, G. M. Peters and M. Svanström, Including Pathogen Risk in Life Cycle Assessment of Wastewater Management. 2. Quantitative Comparison of Pathogen Risk to Other Impacts on Human Health, Environ. Sci. Technol., 2014, 48, 9446–9453 CrossRef CAS PubMed.
- A. Anastasopoulou, A. Kolios, T. Somorin, A. Sowale, Y. Jiang, B. Fidalgo, A. Parker, L. Williams, M. Collins, E. McAdam and S. Tyrrel, Conceptual environmental impact assessment of a novel self-sustained sanitation system incorporating a quantitative microbial risk assessment approach, Sci. Total Environ., 2018, 639, 657–672 CrossRef CAS.
- Y. Kobayashi, G. M. Peters, N. J. Ashbolt, S. Heimersson, M. Svanström and S. J. Khan, Global and local health burden trade-off through the hybridisation of quantitative microbial risk assessment and life cycle assessment to aid water management, Water Res., 2015, 79, 26–38 CrossRef CAS PubMed.
- M. E. Schoen, X. Xue, A. Wood, T. R. Hawkins, J. Garland and N. J. Ashbolt, Cost, energy, global warming, eutrophication and local human health impacts of community water and sanitation service options, Water Res., 2017, 109, 186–195 CrossRef CAS PubMed.
-
D. A. Vallero, in Waste, ed. T. M. Letcher and D. A. Vallero, Academic Press, 2nd edn, 2019, pp. 693–740 Search PubMed.
- H. Zind, L. Mondamert, Q. B. Remaury, A. Cleon, N. K. V. Leitner and J. Labanowski, Occurrence of carbamazepine, diclofenac, and their related metabolites and transformation products in a French aquatic environment and preliminary risk assessment, Water Res., 2021, 117052 CrossRef CAS PubMed.
- D. Awfa, M. Ateia, D. Mendoza and C. Yoshimura, Application of Quantitative Structure–Property Relationship Predictive Models to Water Treatment: A Critical Review, ACS ES&T Water, 2021, 1, 498–517 Search PubMed.
- F. Melnikov, J. Kostal, A. Voutchkova-Kostal, J. B. Zimmerman and P. T. Anastas, Assessment of predictive models for estimating the acute aquatic toxicity of organic chemicals, Green Chem., 2016, 18, 4432–4445 RSC.
- D. Prat, A. Wells, J. Hayler, H. Sneddon, C. R. McElroy, S. Abou-Shehada and P. J. Dunn, CHEM21 selection guide of classical- and less classical-solvents, Green Chem., 2015, 18, 288–296 RSC.
- A. J. Doolin, R. G. Charles, C. S. P. D. Castro, R. G. Rodriguez, E. V. Péan, R. Patidar, T. Dunlop, C. Charbonneau, T. Watson and M. L. Davies, Sustainable solvent selection for the manufacture of methylammonium lead triiodide (MAPbI3) perovskite solar cells, Green Chem., 2021, 23, 2471–2486 RSC.
- J. A. Kaminsky and A. N. Javernick-Will, The Internal Social Sustainability of Sanitation Infrastructure, Environ. Sci. Technol., 2014, 48, 10028–10035 CrossRef CAS.
- S. Van Schoubroeck, M. Van Dael, S. Van Passel and R. Malina, A review of sustainability indicators for biobased chemicals, Renewable Sustainable Energy Rev., 2018, 94, 115–126 CrossRef CAS.
- S. Vallance, H. C. Perkins and J. E. Dixon, What is social sustainability? A clarification of concepts, Geoforum, 2011, 42, 342–348 CrossRef.
- R. H. W. Boyer, N. D. Peterson, P. Arora and K. Caldwell, Five Approaches to Social Sustainability and an Integrated Way Forward, Sustainability, 2016, 8, 878 CrossRef.
-
C. Benoît-Norris, M. Traverso, S. Neugebauer, E. Ekener, T. Schaubroeck, S. Russo Garrido, M. Berger, S. Valdivia, A. Lehmann, M. Finkbeiner and G. Arcese, Guidelines for Social Life Cycle Assessment (S-LCA) - Life Cycle Initiative, United Nations Environment Programme (UNEP), 2020 Search PubMed.
-
C. Benoît-Norris, M. Traverso, S. Valdivia, G. Vickery-Niederman, J. Franze, L. Azuero, A. Ciroth, B. Mazijn and D. Aulisio, The methodological sheets for subcategories in social life cycle assessment (S-LCA), United Nations Environment Programme (UNEP), 2013 Search PubMed.
- C. Benoît, G. A. Norris, S. Valdivia, A. Ciroth, A. Moberg, U. Bos, S. Prakash, C. Ugaya and T. Beck, The guidelines for social life cycle assessment of products: just in time!, Int. J. Life Cycle Assess., 2010, 15, 156–163 CrossRef.
- C. Benoît-Norris, G. Vickery-Niederman, S. Valdivia, J. Franze, M. Traverso, A. Ciroth and B. Mazijn, Introducing the UNEP/SETAC methodological sheets for subcategories of social LCA, Int. J. Life Cycle Assess., 2011, 16, 682–690 CrossRef.
- D. M. Byrne, H. A. C. Lohman, S. M. Cook, G. M. Peters and J. S. Guest, Life cycle assessment (LCA) of urban water infrastructure: emerging approaches to balance objectives and inform comprehensive decision-making, Environ. Sci.: Water Res. Technol., 2017, 3, 1002–1014 RSC.
- S. Van Schoubroeck, G. Thomassen, S. Van Passel, R. Malina, J. Springael, S. Lizin, R. A. Venditti, Y. Yao and M. V. Dael, An integrated techno-sustainability assessment (TSA) framework for emerging technologies, Green Chem., 2021, 23, 1700–1715 RSC.
- M. Garfì and L. Ferrer-Martí, Decision-making criteria and indicators for water and sanitation projects in developing countries, Water Sci. Technol., 2011, 64, 83–101 CrossRef PubMed.
- F. Salisbury, C. Brouckaert, D. Still and C. Buckley, Multiple criteria decision analysis for sanitation selection in South African municipalities, Water SA, 2018, 44, 448–458 CrossRef.
-
SWRR, Sustainable Water Resources Roundtable, Preliminary Report, 2005 Search PubMed.
-
K. G. Chambers, Ph.D. Thesis, University of Colorado Boulder, 2021 Search PubMed.
-
United Nations, Indicators of Sustainable Development: Guidelines and Methodologies, Department of Economic and Social Affairs, United Nations, New York, 2007 Search PubMed.
- A. Davis, A. Javernick-Will and S. M. Cook, A comparison of interviews, focus groups, and photovoice to identify sanitation priorities and increase success of community-based sanitation systems, Environ. Sci.: Water Res. Technol., 2018, 4, 1451–1463 RSC.
- A. Davis, A. Javernick-Will and S. M. Cook, Priority Addressment Protocol: Understanding the Ability and Potential of Sanitation Systems to Address Priorities, Environ. Sci. Technol., 2019, 53, 401–411 CrossRef CAS PubMed.
- A. Flores, C. Buckley and R. Fenner, Selecting sanitation systems for sustainability in developing countries, Water Sci. Technol., 2009, 60, 2973–2982 CrossRef CAS PubMed.
- H. E. Muga and J. R. Mihelcic, Sustainability of wastewater treatment technologies, J. Environ. Manage., 2008, 88, 437–447 CrossRef CAS PubMed.
-
M. Lennartsson, E. Kvarnström, T. Lundberg, J. Buenfil and R. Sawyer, Comparing Sanitation Systems Using Sustainability Criteria, 2009 Search PubMed.
- M. A. Iribarnegaray, F. R. Copa, M. L. Gatto D'Andrea, M. F. Arredondo, J. D. Cabral, J. J. Correa, V. I. Liberal and L. Seghezzo, A comprehensive index to assess the sustainability of water and sanitation management systems, J. Water, Sanit. Hyg. Dev., 2012, 2, 205 CrossRef.
- A. Padilla-Rivera and L. P. Güereca, A proposal metric for sustainability evaluations of wastewater treatment systems (SEWATS), Ecol. Indic., 2019, 103, 22–33 CrossRef.
- F. Salisbury, C. Brouckaert, D. Still and C. Buckley, Multiple criteria decision analysis for sanitation selection in South African municipalities, Water SA, 2018, 44, 448–458 CrossRef.
- A. C. del Munguía-López, A. F. de Sánchez-Bautista, M. M. El-Halwagi and J. M. Ponce-Ortega, Strategic Planning of an Integrated Fuel Production System with a Fair-Sustainable Approach, ACS Sustainable Chem. Eng., 2021, 9, 5116–5127 CrossRef.
- A. C. del Munguía-López, A. F. de Sánchez-Bautista, M. M. El-Halwagi and J. M. Ponce-Ortega, Strategic Planning of an Integrated Fuel Production System with a Fair-Sustainable Approach, ACS Sustainable Chem. Eng., 2021, 9, 5116–5127 CrossRef.
-
M. Lennartsson, E. Kvarnström, T. Lundberg, J. Buenfil and R. Sawyer, Comparing Sanitation Systems Using Sustainability Criteria, Stockholm Environment Institute, 2009 Search PubMed.
- M. A. Iribarnegaray, F. R. Copa, M. L. Gatto D'Andrea, M. F. Arredondo, J. D. Cabral, J. J. Correa, V. I. Liberal and L. Seghezzo, A comprehensive index to assess the sustainability of water and sanitation management systems, J. Water, Sanit. Hyg. Dev., 2012, 2, 205–222 CrossRef.
- S. L. Young, G. O. Boateng, Z. Jamaluddine, J. D. Miller, E. A. Frongillo, T. B. Neilands, S. M. Collins, A. Wutich, W. E. Jepson and J. Stoler, The Household Water InSecurity Experiences (HWISE) Scale: development and validation of a household water insecurity measure for low-income and middle-income countries, BMJ Glob. Health, 2019, 4, e001750 CrossRef PubMed.
- J. Kaminsky and A. Javernick-Will, Theorizing the Internal Social Sustainability of Sanitation Organizations, J. Constr. Eng. Manag., 2015, 141, 04014071 CrossRef.
- A. Davis, A. Javernick-Will and S. M. Cook, Analyzing Sanitation Sustainability Assessment Frameworks for Resource-Limited Communities, Environ. Sci. Technol., 2019, 53, 13535–13545 CrossRef CAS PubMed.
- E. Grubert, The Need for a Preference-Based Multicriteria Prioritization Framework in Life Cycle Sustainability Assessment, J. Ind. Ecol., 2017, 21, 1522–1535 CrossRef CAS.
- A. Davis, A. Javernick-Will and S. M. Cook, Priority Addressment Protocol: Understanding the Ability and Potential of Sanitation Systems to Address Priorities, Environ. Sci. Technol., 2019, 53, 401–411 CrossRef CAS PubMed.
- C. H. Jones, J. Meyer, P. K. Cornejo, W. Hogrewe, C. J. Seidel and S. M. Cook, A new framework for small drinking water plant sustainability support and decision-making, Sci. Total Environ., 2019, 695, 133899 CrossRef CAS PubMed.
- D. Stokes and R. Bergin, Methodology or ‘methodolatry’? An evaluation of focus groups and depth interviews, Qual. Mark. Res., 2006, 9, 26–37 CrossRef.
-
D. W. Stewart and P. N. Shamdasani, Focus Groups: Theory and Practice, SAGE Publications, Thousand Oaks, 2nd edn, 2007 Search PubMed.
- A. Bakchan, M. E. Hacker and K. M. Faust, Resilient Water and Wastewater Infrastructure Systems through Integrated Humanitarian-Development Processes: The Case of Lebanon's Protracted Refugee Crisis, Environ. Sci. Technol., 2021, 55, 6407–6420 CrossRef CAS PubMed.
- A. Khatri-Chhetri, P. K. Aggarwal, P. K. Joshi and S. Vyas, Farmers' prioritization of climate-smart agriculture (CSA) technologies, Agric. Syst., 2017, 151, 184–191 CrossRef.
- K. Bakker, M. Kooy, N. E. Shofiani and E. J. Martijn, Governance Failure: Rethinking the Institutional Dimensions of Urban Water Supply to Poor Households, World Dev., 2008, 36, 1891–1915 CrossRef.
- H. M. Breunig, T. Huntington, L. Jin, A. Robinson and C. D. Scown, Dynamic Geospatial Modeling of the Building Stock To Project Urban Energy Demand, Environ. Sci. Technol., 2018, 52, 7604–7613 CrossRef CAS PubMed.
- D. Spuhler, A. Scheidegger and M. Maurer, Generation of sanitation system options for urban planning considering novel technologies, Water Res., 2018, 145, 259–278 CrossRef CAS PubMed.
- Y. Cortés-Peña, D. Kumar, V. Singh and J. S. Guest, BioSTEAM: A Fast and Flexible Platform for the Design, Simulation, and Techno-Economic Analysis of Biorefineries under Uncertainty, ACS Sustainable Chem. Eng., 2020, 8, 3302–3310 CrossRef.
- S. Leow, B. D. Shoener, Y. Li, J. L. DeBellis, J. Markham, R. Davis, L. M. L. Laurens, P. T. Pienkos, S. M. Cook, T. J. Strathmann and J. S. Guest, A Unified Modeling Framework to Advance Biofuel Production from Microalgae, Environ. Sci. Technol., 2018, 52, 13591–13599 CrossRef CAS PubMed.
- Y. Li, S. Leow, T. Dong, N. J. Nagle, E. P. Knoshaug, L. M. L. Laurens, P. T. Pienkos, J. S. Guest and T. J. Strathmann, Demonstration and Evaluation of Hybrid Microalgae Aqueous Conversion Systems for Biofuel Production, ACS Sustainable Chem. Eng., 2019, 7, 5835–5844 CrossRef CAS.
- A. Bhat and A. Kumar, Application of the Crystal Ball® software for uncertainty and sensitivity analyses for predicted concentration and risk levels, Environ. Prog., 2008, 27, 289–294 CrossRef CAS.
- S. P. Hilton, G. A. Keoleian, G. T. Daigger, B. Zhou and N. G. Love, Life Cycle Assessment of Urine Diversion and Conversion to Fertilizer Products at the City Scale, Environ. Sci. Technol., 2021, 55, 593–603 CrossRef CAS PubMed.
-
Hydromantis, GPS-X, https://www.hydromantis.com/GPSX.html, (accessed 12 April 2021).
-
EnviroSim, BioWin, https://envirosim.com/products, (accessed 10 August 2022).
-
AspenTech, Aspen Plus, https://www.aspentech.com/en/products/engineering/aspen-plus, (accessed 12 April 2021).
-
Intelligen, Inc., SuperPro Designer, https://www.intelligen.com/products/superpro-overview/, (accessed 12 April 2021).
-
The AnyLogic Company, AnyLogic, https://www.anylogic.com/transportation/, (accessed 22 May 2021).
- J. Rashidi, G. Rhee, M. Kim, K. Nam, S. Heo, C. Yoo and A. Karbassi, Life Cycle and Economic Assessments of Key Emerging Energy Efficient Wastewater Treatment Processes for Climate Change Adaptation, Int. J. Environ. Res., 2018, 12, 815–827 CrossRef CAS.
- M. Nowrouzi and H. Abyar, A framework for the design and optimization of integrated fixed-film activated sludge-membrane bioreactor configuration by focusing on cost-coupled life cycle assessment, J. Cleaner Prod., 2021, 296, 126557 CrossRef CAS.
- S. C. D'Angelo, A. Dall'Ara, C. Mondelli, J. Pérez-Ramírez and S. Papadokonstantakis, Techno-Economic Analysis of a Glycerol Biorefinery, ACS Sustainable Chem. Eng., 2018, 6, 16563–16572 CrossRef.
- P. H. Chen and J. C. Quinn, Microalgae to biofuels through hydrothermal liquefaction: Open-source techno-economic analysis and life cycle assessment, Appl. Energy, 2021, 289, 116613 CrossRef CAS.
- G. Zang, P. Sun, A. Elgowainy, A. Bafana and M. Wang, Life Cycle Analysis of Electrofuels: Fischer–Tropsch Fuel Production from Hydrogen and Corn Ethanol Byproduct CO2, Environ. Sci. Technol., 2021, 55, 3888–3897 CrossRef CAS PubMed.
- National Alliance for Water Innovation (NAWI), Water-TAP3, https://www.nawihub.org/watertap, (accessed 12 April 2021).
- Y. Li, X. Zhang, V. L. Morgan, H. A. C. Lohman, L. S. Rowles, S. Mittal, A. Kogler, R. D. Cusick, W. A. Tarpeh and J. S. Guest, QSDsan: an integrated platform for quantitative sustainable design of sanitation and resource recovery systems, Environ. Sci.: Water Res. Technol., 2022 10.1039/D2EW00455K , Advance Article.
-
NREL, WESyS, https://github.com/NREL/WESyS-Model, (accessed 12 April 2021).
- Y. Wang, J. W. Levis and M. A. Barlaz, Development of Streamlined Life-Cycle Assessment for the Solid Waste Management System, Environ. Sci. Technol., 2021, 55, 5475–5484 CrossRef CAS.
-
D. W. O. de Medeiros, DWSIM, https://dwsim.inforside.com.br/new/, (accessed 12 April 2021).
-
FOSSEE, OMChemSim, https://github.com/FOSSEE/OMChemSim, (accessed 12 April 2021).
-
D. C. Miller, J. D. Siirola, D. Agarwal, A. P. Burgard, A. Lee, J. C. Eslick, B. Nicholson, C. Laird, L. T. Biegler, D. Bhattacharyya, N. V. Sahinidis, I. E. Grossmann, C. E. Gounaris and D. Gunter, in Computer Aided Chemical Engineering, ed. M. R. Eden, M. G. Ierapetritou and G. P. Towler, Elsevier, 2018, vol. 44, pp. 2209–2214 Search PubMed.
-
K. Koster and M. Cohen, Practical Approaches to Sustainability: iSUSTAIN® Tool for Green Chemistry Case Study, Treatise on Sustainability Science and Engineering, 2013, pp. 81–108 Search PubMed.
-
American Chemical Society, Research Tools, https://www.acs.org/content/acs/en/greenchemistry/research-innovation/tools-for-green-chemistry.html, (accessed 12 April 2021).
- R. Sacchi, C. Bauer and B. L. Cox, Does Size Matter? The Influence of Size, Load Factor, Range Autonomy, and Application Type on the Life Cycle Assessment of Current and Future Medium- and Heavy-Duty Vehicles, Environ. Sci. Technol., 2021, 55, 5224–5235 CrossRef CAS PubMed.
-
GitHub, https://github.com, (accessed 30 May 2021).
-
GitLab, https://about.gitlab.com/, (accessed 30 May 2021).
- Technical University of Denmark and Lund University, Wastewater Treatment Models, https://github.com/wwtmodels, (accessed 12 April 2021).
- R. Frischknecht, N. Jungbluth, H.-J. Althaus, G. Doka, R. Dones, T. Heck, S. Hellweg, R. Hischier, T. Nemecek, G. Rebitzer and M. Spielmann, The ecoinvent Database: Overview and Methodological Framework (7 pp), Int. J. Life Cycle Assess., 2005, 10, 3–9 CrossRef CAS.
-
PRé Sustainability, SimaPro, https://simapro.com/, (accessed 12 April 2021).
-
Sphera Solutions GmbH, GaBi, https://www.gabi-software.com/america/index/, (accessed 12 April 2021).
-
GreenDelta, openLCA, https://www.openlca.org/, (accessed 12 April 2021).
-
Chris Mutel, Brightway2, https://brightway.dev/, (accessed 12 April 2021).
-
Argonne National Laboratory, The Greenhouse gases, Regulated Emissions, and Energy use in Transportation (GREET) Model, https://greet.es.anl.gov/, (accessed 25 August 2020).
- Center for Advancing Microbial Risk Assessment, QMRAwiki, https://qmrawiki.org/, (accessed 10 May 2021).
- I. Schmidt, M. Meurer, P. Saling, A. Kicherer, W. Reuter and C.-O. Gensch, SEEbalance®: Managing sustainability of products and processes with the socio-eco-efficiency analysis by BASF, Greener Manag. Int., 2004, 79–94 Search PubMed.
- D. Kolsch, P. Saling, A. Kicherer, A. G. Sommer and I. Schmidt, How to measure social impacts? A socio-eco-efficiency analysis by the SEEBALANCE® method, Int. J. Sustain. Dev., 2008, 11, 1 CrossRef.
-
SHDB, Social Hotspots Database, https://www.socialhotspot.org/, (accessed 28 May 2021).
- C. Benoit-Norris, D. A. Cavan and G. Norris, Identifying social impacts in product supply chains: Overview and application of the social hotspot database, Sustainability, 2012, 4, 1946–1965 CrossRef.
- R. Mahmud, S. M. Moni, K. High and M. Carbajales-Dale, Integration of techno-economic analysis and life cycle assessment for sustainable process design – A review, J. Cleaner Prod., 2021, 317, 128247 CrossRef.
- R. Davis, A. Aden and P. T. Pienkos, Techno-economic analysis of autotrophic microalgae for fuel production, Appl. Energy, 2011, 88, 3524–3531 CrossRef.
- J. Sun, L. Yang, S. Xiao, H. Chu, S. Jiang, Z. Yu, X. Zhou and Y. Zhang, A promising microalgal wastewater cyclic cultivation technology: Dynamic simulations, economic viability, and environmental suitability, Water Res., 2022, 217, 118411 CrossRef CAS PubMed.
- M. B. Beck, Principles of Modelling, Water Sci. Technol., 1991, 24, 1–8 CrossRef CAS.
- J. C. Helton, J. D. Johnson and W. L. Oberkampf, An exploration of alternative approaches to the representation of uncertainty in model predictions, Reliab. Eng. Syst, 2004, 85, 39–71 CrossRef.
- R. L. Winkler, Uncertainty in probabilistic risk assessment, Reliab. Eng. Syst, 1996, 54, 127–132 CrossRef.
- E. Hofer, When to separate uncertainties and when not to separate, Reliab. Eng. Syst, 1996, 54, 113–118 CrossRef.
- W. L. Oberkampf, J. C. Helton, C. A. Joslyn, S. F. Wojtkiewicz and S. Ferson, Challenge problems: uncertainty in system response given uncertain parameters, Reliab. Eng. Syst, 2004, 85, 11–19 CrossRef.
- G. Li, Z. Lu, L. Li and B. Ren, Aleatory and epistemic uncertainties analysis based on non-probabilistic reliability and its kriging solution, Appl. Math. Model., 2016, 40, 5703–5716 CrossRef.
- N. R. Baral, O. Kavvada, D. Mendez-Perez, A. Mukhopadhyay, T. Soon Lee, B. A. Simmons and C. D. Scown, Techno-economic analysis and life-cycle greenhouse gas mitigation cost of five routes to bio-jet fuel blendstocks, Energy Environ. Sci., 2019, 12, 807–824 RSC.
- S. S. Bhagwat, Y. Li, Y. R. Cortés-Peña, E. C. Brace, T. A. Martin, H. Zhao and J. S. Guest, Sustainable Production of Acrylic Acid via 3-Hydroxypropionic Acid from Lignocellulosic Biomass, ACS Sustainable Chem. Eng., 2021, 9, 16659–16669 CrossRef CAS.
- B. P. Weidema and M. S. Wesnæs, Data quality management for life cycle inventories—an example of using data quality indicators, J. Cleaner Prod., 1996, 4, 167–174 CrossRef.
- B. P. Weidema, Multi-user test of the data quality matrix for product life cycle inventory data, Int. J. Life Cycle Assess., 1998, 3, 259–265 CrossRef.
- A. Ciroth, Cost data quality considerations for eco-efficiency measures, Ecol. Econ., 2009, 68, 1583–1590 CrossRef.
- N. C. Do and S. Razavi, Correlation Effects? A Major but Often Neglected Component in Sensitivity and Uncertainty Analysis, Water Resour. Res., 2020, 56, e2019WR025436 Search PubMed.
- I. M. Sobol, On the distribution of points in a cube and the approximate evaluation of integrals, USSR Comput. Math. Math. Phys., 1967, 7, 86–112 CrossRef.
-
R. L. Winkler and W. L. Hays, Statistics: Probability, Inference, and Decision, Holt, Rinehart and Winston, 1975 Search PubMed.
- J. K. Choe, A. M. Bergquist, S. Jeong, J. S. Guest, C. J. Werth and T. J. Strathmann, Performance and life cycle environmental benefits of recycling spent ion exchange brines by catalytic treatment of nitrate, Water Res., 2015, 80, 267–280 CrossRef CAS PubMed.
- M. Yang, N. R. Baral, A. Anastasopoulou, H. M. Breunig and C. D. Scown, Cost and Life-Cycle Greenhouse Gas Implications of Integrating Biogas Upgrading and Carbon Capture Technologies in Cellulosic Biorefineries, Environ. Sci. Technol., 2020, 54, 12810–12819 CrossRef CAS PubMed.
- J. L. Field, T. L. Richard, E. A. H. Smithwick, H. Cai, M. S. Laser, D. S. LeBauer, S. P. Long, K. Paustian, Z. Qin, J. J. Sheehan, P. Smith, M. Q. Wang and L. R. Lynd, Robust paths to net greenhouse gas mitigation and negative emissions via advanced biofuels, Proc. Natl. Acad. Sci. U. S. A., 2020, 117, 21968–21977 CrossRef CAS PubMed.
- Y. Li, S. S. Bhagwat, Y. R. Cortés-Peña, D. Ki, C. V. Rao, Y.-S. Jin and J. S. Guest, Sustainable Lactic Acid Production from Lignocellulosic Biomass, ACS Sustainable Chem. Eng., 2021, 9, 1341–1351 CrossRef CAS.
- J. L. Field, T. L. Richard, E. A. H. Smithwick, H. Cai, M. S. Laser, D. S. LeBauer, S. P. Long, K. Paustian, Z. Qin, J. J. Sheehan, P. Smith, M. Q. Wang and L. R. Lynd, Robust paths to net greenhouse gas mitigation and negative emissions via advanced biofuels, Proc. Natl. Acad. Sci. U. S. A., 2020, 117, 21968–21977 CrossRef CAS.
-
S. B. Jones, Y. Zhu, D. B. Anderson, R. T. Hallen, D. C. Elliott, A. J. Schmidt, K. O. Albrecht, T. R. Hart, M. G. Butcher, C. Drennan, L. J. Snowden-Swan, R. Davis and C. Kinchin, Process Design and Economics for the Conversion of Algal Biomass to Hydrocarbons: Whole Algae Hydrothermal Liquefaction and Upgrading, Pacific Northwest National Lab. (PNNL), Richland, WA (United States), 2014 Search PubMed.
-
R. Davis, M. Wiatrowski, C. Kinchin and D. Humbird, Conceptual Basis and Techno-Economic Modeling for Integrated Algal Biorefinery Conversion of Microalgae to Fuels and Products. 2019 NREL TEA Update: Highlighting Paths to Future Cost Goals via a New Pathway for Combined Algal Processing, National Renewable Energy Lab. (NREL), Golden, CO (United States), 2020 Search PubMed.
-
A. Saltelli, S. Tarantola, F. Campolongo and M. Ratto, Sensitivity Analysis in Practice: A Guide to Assessing Scientific Models, Wiley, 2004 Search PubMed.
-
X. Zhou and H. Lin, in Encyclopedia of GIS, ed. S. Shekhar and H. Xiong, Springer US, Boston, MA, 2008, pp. 616–616 Search PubMed.
-
X. Zhou, H. Lin and H. Lin, in Encyclopedia of GIS, ed. S. Shekhar and H. Xiong, Springer US, Boston, MA, 2008, pp. 408–409 Search PubMed.
- Y. Li, S. A. Slouka, S. M. Henkanatte-Gedera, N. Nirmalakhandan and T. J. Strathmann, Seasonal treatment and economic evaluation of an algal wastewater system for energy and nutrient recovery, Environ. Sci.: Water Res. Technol., 2019, 5, 1545–1557 RSC.
- D. E. Giammar, D. M. Greene, A. Mishrra, N. Rao, J. B. Sperling, M. Talmadge, A. Miara, K. A. Sitterley, A. Wilson, S. Akar, P. Kurup, J. R. Stokes-Draut and K. Coughlin, Cost and Energy Metrics for Municipal Water Reuse, ACS ES&T Eng., 2022, 2, 489–507 Search PubMed.
- J. Morio, Global and local sensitivity analysis methods for a physical system, Eur. J. Phys., 2011, 32, 1577–1583 CrossRef.
- M. Gómez-Delgado and S. Tarantola, GLOBAL sensitivity analysis, GIS and multi-criteria evaluation for a sustainable planning of a hazardous waste disposal site in Spain, Int. J. Geogr. Inf., 2006, 20, 449–466 CrossRef.
- P. Heiselberg, H. Brohus, A. Hesselholt, H. Rasmussen, E. Seinre and S. Thomas, Application of sensitivity analysis in design of sustainable buildings, Renewable Energy, 2009, 34, 2030–2036 CrossRef.
- G. Sin, K. V. Gernaey, M. B. Neumann, M. C. M. van Loosdrecht and W. Gujer, Global sensitivity analysis in wastewater treatment plant model applications: Prioritizing sources of uncertainty, Water Res., 2011, 45, 639–651 CrossRef CAS PubMed.
- D. L. Johnsen, H. Emamipour, J. S. Guest and M. J. Rood, Environmental and Economic Assessment of Electrothermal Swing Adsorption of Air Emissions from Sheet-Foam Production Compared to Conventional Abatement Techniques, Environ. Sci. Technol., 2016, 50, 1465–1472 CrossRef CAS PubMed.
- J. M. Hutchison, J. S. Guest and J. L. Zilles, Evaluating the Development of Biocatalytic Technology for the Targeted Removal of Perchlorate from Drinking Water, Environ. Sci. Technol., 2017, 51, 7178–7186 CrossRef CAS.
- A. Rezaei, K. B. Nakshatrala, F. Siddiqui, B. Dindoruk and M. Soliman, A Global Sensitivity Analysis and Reduced-Order Models for Hydraulically Fractured Horizontal Wells, Comput. Geosci., 2020, 24, 995–1029 CrossRef.
- J. T. Trimmer, H. A. C. Lohman, D. M. Byrne, S. A. Houser, F. Jjuuko, D. Katende, N. Banadda, A. Zerai, D. C. Miller and J. S. Guest, Navigating Multidimensional Social–Ecological System Trade-Offs across Sanitation Alternatives in an Urban Informal Settlement, Environ. Sci. Technol., 2020, 54, 12641–12653 CrossRef CAS PubMed.
-
S. Kokoska and D. Zwillinger, CRC Standard Probability and Statistics Tables and Formulae, CRC Press, Boca Raton, Fla, 2000 Search PubMed.
- M. D. Morris, Factorial Sampling Plans for Preliminary Computational Experiments, Technometrics, 1991, 33, 161–174 CrossRef.
- D. M. King and B. J. C. Perera, Morris method of sensitivity analysis applied to assess the importance of input variables on urban water supply yield – A case study, J. Hydrol., 2013, 477, 17–32 CrossRef.
- I. M. Sobol', Sensitivity Estimates for Nonlinear Mathematical Models, Mathematical Modelling and Computational Experiments, 1993, 1, 407–414 Search PubMed.
- R. I. Cukier, H. B. Levine and K. E. Shuler, Nonlinear sensitivity analysis of multiparameter model systems, J. Comput. Phys., 1978, 26, 1–42 CrossRef.
- M. J. Spaniol and N. J. Rowland, Defining scenario, Futures Foresight Sci., 2019, 1, e3 CrossRef.
- D. B. Gingerich and M. S. Mauter, Air Emissions Damages from Municipal Drinking Water Treatment Under Current and Proposed Regulatory Standards, Environ. Sci. Technol., 2017, 51, 10299–10306 CrossRef CAS PubMed.
- A. K. Vuppaladadiyam, P. Prinsen, A. Raheem, R. Luque and M. Zhao, Sustainability Analysis of Microalgae Production Systems: A Review on Resource with Unexploited High-Value Reserves, Environ. Sci. Technol., 2018, 52, 14031–14049 CrossRef CAS PubMed.
- N. R. Baral, J. Dahlberg, D. Putnam, J. C. Mortimer and C. D. Scown, Supply Cost and Life-Cycle Greenhouse Gas Footprint of Dry and Ensiled Biomass Sorghum for Biofuel Production, ACS Sustainable Chem. Eng., 2020, 8, 15855–15864 CrossRef CAS.
- A. Mendoza Beltran, B. Cox, C. Mutel, D. P. van Vuuren, D. Font Vivanco, S. Deetman, O. Y. Edelenbosch, J. Guinée and A. Tukker, When the Background Matters: Using Scenarios from Integrated Assessment Models in Prospective Life Cycle Assessment, J. Ind. Ecol., 2020, 24, 64–79 CrossRef CAS.
-
K. Stock and H. Guesgen, in Automating Open Source Intelligence, ed. R. Layton and P. A. Watters, Syngress, Boston, 2016, pp. 171–204 Search PubMed.
- J. T. Trimmer, A. J. Margenot, R. D. Cusick and J. S. Guest, Aligning Product Chemistry and Soil Context for Agronomic Reuse of Human-Derived Resources, Environ. Sci. Technol., 2019, 53, 6501–6510 CrossRef CAS PubMed.
- L. Chen, E. Blanc-Betes, T. W. Hudiburg, D. Hellerstein, S. Wallander, E. H. DeLucia and M. Khanna, Assessing the Returns to Land and Greenhouse Gas Savings from Producing Energy Crops on Conservation Reserve Program Land, Environ. Sci. Technol., 2021, 55, 1301–1309 CrossRef CAS PubMed.
- J.
T. Trimmer and J. S. Guest, Recirculation of human-derived nutrients from cities to agriculture across six continents, Nat. Sustain., 2018, 1, 427–435 CrossRef.
- S. Ankathi, D. Watkins, P. Sreedhara, J. Zuhlke and D. R. Shonnard, GIS-Integrated Optimization for Locating Food Waste and Manure Anaerobic Co-digestion Facilities, ACS Sustainable Chem. Eng., 2021, 9, 4024–4032 CrossRef CAS.
- D. Burns and E. Grubert, Attribution of production-stage methane emissions to assess spatial variability in the climate intensity of US natural gas consumption, Environ. Res. Lett., 2021, 16, 044059 CrossRef CAS.
- F. Pomponi and A. Stephan, Water, energy, and carbon dioxide footprints of the construction sector: A case study on developed and developing economies, Water Res., 2021, 194, 116935 CrossRef CAS PubMed.
- J. Tovar-Facio, L. S. Guerras, J. M. Ponce-Ortega and M. Martín, Sustainable Energy Transition Considering the Water–Energy Nexus: A Multiobjective Optimization Framework, ACS Sustainable Chem. Eng., 2021, 9, 3768–3780 CrossRef CAS.
- X. Liu, G. Shen, L. Chen, Z. Qian, N. Zhang, Y. Chen, Y. Chen, J. Cao, H. Cheng, W. Du, B. Li, G. Li, Y. Li, X. Liang, M. Liu, H. Lu, Z. Luo, Y. Ren, Y. Zhang, D. Zhu and S. Tao, Spatially Resolved Emission Factors to Reduce Uncertainties in Air Pollutant Emission Estimates from the Residential Sector, Environ. Sci. Technol., 2021, 55, 4483–4493 CrossRef CAS PubMed.
- A. Parchomenko and S. Borsky, Identifying phosphorus hot spots: A spatial analysis of the phosphorus balance as a result of manure application, J. Environ. Manage., 2018, 214, 137–148 CrossRef CAS PubMed.
- R. Wielemaker, C. Wilken, W.-S. Chen, O. Oenema and J. Weijma, Resource Dynamo: A GIS model to match urban nutrient supply with agricultural demand, J. Cleaner Prod., 2020, 258, 120789 CrossRef CAS.
- U. Akram, N.-H. Quttineh, U. Wennergren, K. Tonderski and G. S. Metson, Optimizing Nutrient Recycling From Excreta in Sweden and Pakistan: Higher Spatial Resolution Makes Transportation More Attractive, Front. Sustain. Food Syst., 2019, 3, Article 50 CrossRef.
-
L. Li, H. Ban, S. P. Wechsler and B. Xu, in Comprehensive Geographic Information Systems, ed. B. Huang, Elsevier, Oxford, 2018, pp. 313–340 Search PubMed.
- A. Maertens, E. Golden and T. Hartung, Avoiding Regrettable Substitutions: Green Toxicology for Sustainable Chemistry, ACS Sustainable Chem. Eng., 2021, 9, 7749–7758 CrossRef CAS PubMed.
- M. Curran, L. de Baan, A. M. De Schryver, R. van Zelm, S. Hellweg, T. Koellner, G. Sonnemann and M. A. J. Huijbregts, Toward Meaningful End Points of Biodiversity in Life Cycle Assessment, Environ. Sci. Technol., 2011, 45, 70–79 CrossRef CAS PubMed.
- L. Winter, A. Lehmann, N. Finogenova and M. Finkbeiner, Including biodiversity in life cycle assessment – State of the art, gaps and research needs, Environ. Impact Assess. Rev., 2017, 67, 88–100 CrossRef.
- A. A. Farouk, M. M. El-Halwagi, D. C. Y. Foo and I. M. L. Chew, Development of a C–H–O Symbiosis Network during Conceptual Design via Economic, Sustainability, and Safety Metrics, ACS Sustainable Chem. Eng., 2021, 9, 3735–3749 CrossRef CAS.
- M. Cinelli, M. Spada, W. Kim, Y. Zhang and P. Burgherr, MCDA Index Tool: an interactive software to develop indices and rankings, Environ. Syst. Decis., 2021, 41, 82–109 CrossRef PubMed.
- S. Van Schoubroeck, J. Springael, M. Van Dael, R. Malina and S. Van Passel, Sustainability indicators for biobased chemicals: A Delphi study using Multi-Criteria Decision Analysis, Resour., Conserv. Recycl., 2019, 144, 198–208 CrossRef.
- R. W. Saaty, The analytic hierarchy process—what
it is and how it is used, Mathematical Modelling, 1987, 9, 161–176 CrossRef.
- M. Behzadian, R. B. Kazemzadeh, A. Albadvi and M. Aghdasi, PROMETHEE: A comprehensive literature review on methodologies and applications, Eur. J. Oper. Res., 2010, 200, 198–215 CrossRef.
- Q. Chen and I. E. Grossmann, Recent Developments and Challenges in Optimization-Based Process Synthesis, Annu. Rev. Chem. Biomol. Eng., 2017, 8, 249–283 CrossRef PubMed.
- J. S. Guest, S. J. Skerlos, G. T. Daigger, J. R. E. Corbett and N. G. Love, The use of qualitative system dynamics to identify sustainability characteristics of decentralized wastewater management alternatives, Water Sci. Technol., 2010, 61, 1637–1644 CrossRef CAS.
- D. M. Byrne, M. K. Grabowski, A. C. B. Benitez, A. R. Schmidt and J. S. Guest, Evaluation of Life Cycle Assessment (LCA) for Roadway Drainage Systems, Environ. Sci. Technol., 2017, 51, 9261–9270 CrossRef CAS PubMed.
Footnotes |
† Electronic supplementary information (ESI) available: Table S1. Additional literature examples illustrating concepts, steps, and applications of QSD. See DOI: https://doi.org/10.1039/d2ew00431c |
‡ Current address: Carollo Engineers, Inc., 14755 Preston Road, Suite 500, Dallas, Texas 75254, USA. |
|
This journal is © The Royal Society of Chemistry 2022 |
Click here to see how this site uses Cookies. View our privacy policy here.