DOI:
10.1039/D1EA00046B
(Critical Review)
Environ. Sci.: Atmos., 2022,
2, 9-23
Methane detection and quantification in the upstream oil and gas sector: the role of satellites in emissions detection, reconciling and reporting†
Received
18th June 2021
, Accepted 3rd November 2021
First published on 5th November 2021
Abstract
Oil and gas activities are a major source of methane and in recent years multiple companies have made pledges to cut their emissions of this potent greenhouse gas. Satellites are a promising technology, but their relevance to emissions reconciliation and reporting has not yet been independently established. In this review paper, we assess the capabilities of satellites to determine their role in emissions detection, reconciling and reporting in the upstream section of the oil and gas value chain. In reconciling, satellites have a role in verifying emissions estimated by other technologies, as well as in determining what is causing discrepancies in emission estimates. There are many limitations to satellite usage which need to be addressed before their widescale or routine use by the sector, particularly relating to where they can be used, and high uncertainty associated with their emission estimates. However, where limitations are overcome, satellites could potentially transform the way emissions are reconciled and reported through long-term monitoring, building emission profiles, and tracking whether emission targets are being met. Satellites are valuable tools, not just to the oil and gas sector but to international governments and organisations, as abating methane is crucial for achieving Paris Agreement ambitions.
Environmental significance
Methane is the second most important greenhouse gas and companies in the oil and gas sector are actively seeking solutions to detect, quantify and abate their emissions. However, there is high uncertainty in the emissions reported and therefore it is unclear how effective abatement strategies are and whether the sector is making progress towards reducing their emissions. Satellites are a technology which could improve the confidence in emissions reporting. In this work, by investigating the characteristics of how satellites detect and measure methane, we demonstrate that current technology is not capable of tracking emissions but could be suitable for large scale emissions verification. We identify where satellites can benefit the sector and where alternative technology should be used.
|
1. Introduction
Methane is a potent greenhouse gas (GHG),1 and large quantities are emitted by the oil and gas sector every year through venting, fugitives and incomplete combustion.2,3 Tackling methane has become a primary objective for oil and gas companies and many have either joined consortiums, which aim to reduce methane emissions through collaborative actions e.g., oil and gas methane partnership (OGMP), global methane initiative (GMI), oil and gas climate initiative (OGCI), or have set company specific targets.4–10 As emission targets for methane become tighter, it is imperative that methane emission estimates become more accurate and robust. In the oil and gas sector, reporting requirements are evolving to include more detail on emissions (source, type and method used to quantify emissions) and reconciling emissions has emerged as a key component of emission reporting frameworks. For example, in the OGMP 2.0 framework, reconciling emissions estimated between source-level (bottom-up) estimates and site-level (top-down) estimates is necessary for achieving the highest level and standard in emissions reporting under this framework.11
In this review paper, we assess the role of satellites in the upstream oil and gas value chain, with the aim of determining how they can be used to detect emissions, reconcile emission measurements, and challenge emissions reporting. There have been many studies carried out to detect and measure methane emissions in the oil and gas sector12–21 and these have largely focussed on determining the magnitude of emissions, as well as deriving emissions rates for the different activities, components, stages etc. in the oil and gas value chain. Previous studies have also compared technologies for their suitability in fugitive emissions detection and quantification.22,23 However, none of these answer the questions: how can satellites be used to reconcile emission measurements and how they could challenge reconciliation and emissions reporting?
To address these objectives, we analyse the factors which affect satellite usage to determine the state of the technology at the time of writing. From this, we determine the role they play in emissions detection, reconciling and reporting. This review paper will begin with an overview of how methane emissions are quantified in the upstream oil and gas value chain. We then outline how satellites measure methane, the factors which impede their use, how they compare to other technologies and how they have been used, as reported in the literature, so far. From this we discuss their role in emissions reconciliation and how they could challenge emissions reporting and reconciliation. We end the article with suggestions of areas for future work and our conclusions drawn. This review paper is of use to anyone with an interest in emissions reconciliation, as well as anyone with an interest in how satellites quantify methane emissions.
2. Methane detection and quantification-how emissions are estimated
In the oil and gas sector, as well as other sectors where methane is emitted, methane emissions can be quantified through one of three methods:24–27 engineering calculations, emission factors and direct measurements.
2.1. Engineering calculations
Emissions from venting, fugitives and incomplete combustions can be calculated through the use of engineering calculations.24,25 This encompasses a large variety of methods ranging from equipment design specification, such as flaring efficiency, to complex process simulations using software like Aspen HYSYS or Aspen Plus.24,28 As there is such a variety in the methods that can be used, engineering calculations are suitable for estimating emissions from many emission sources, ranging from point source to whole facility/site. However, the accuracy of the emission estimates is highly dependent on the data inputs and assumptions made in the calculations or models. Therefore, improving data is key to improving the accuracy of this method.28 The emissions estimated are best used in combination with direct measurement. Not only to verify the emission estimates but also to verify the data inputs and assumptions made in the calculations/model.29 Engineering calculations are beneficial over both direct measurement and emission factors as they can be used to model and predict emissions, as has been done for other methane sources, such as reservoirs and dairy farms,30,31 but the accuracy of any predictions is dependent on the accuracy of the input data and assumptions. This method can only provide emission estimates for known emissions sources and is unable to identify new emission sources. They are also only suitable for estimating emissions under the conditions specified in the model input, so super-emitters (see the ESI,† for definition) are difficult to quantify because of their irregular and uncertain nature.
2.2. Emission factors
The emissions estimated (venting, fugitives and incomplete combustion) using emission factors are similar to those estimated via engineering calculations but can be less detailed and lower in granularity.29 Emission factors are an emission intensity rate (e.g., mass per unit time, mass per unit/process per time, mass per unit energy) which allow for emissions to be estimated relatively quickly as only activity factor (throughput, energy consumption, hours equipment is operating, number of sources etc.) data are needed.24,29 Emission factors vary in their level of detail, from component (types of pumps and valves), process (gas production and gas processing) and facility (gas processing plant and refinery) specific which makes them versatile.13,26,32,33 The granularity of their emission estimates can be lower than engineering calculations and direct measurement, if they are generic i.e. IPCC Tier 1 emission factors.29,32 When using emission factors, emissions are estimated assuming all emission sources are accounted for in the activity data and consequentially they are unable to account for unidentified emission sources. Additionally, their accuracy is highly dependent on how representative the emission factor is.24,28,29 Consequentially, they cannot account for super-emitters because of their uncertain nature. Like engineering calculations, emission factors are best used in combination with direct measurement estimates for validating emission estimates, as well as calibrating emission factors to increase their representativeness and accuracy.
2.3. Direct measurement
In the direct measurement method, emissions (venting, fugitives and incomplete combustion) are quantified at the source.24,28,29 A combination of technologies (see Table S4 in the ESI† for examples) are typically applied, with one used to detect the presence of methane (e.g., parts per billion) and another to measure a flowrate or flux, from which an emissions rate is determined.25,27,29 There are many technologies which are used to either detect or quantify methane emissions in the oil and gas sector, which can be classified as bottom-up or top-down (Fig. 1).
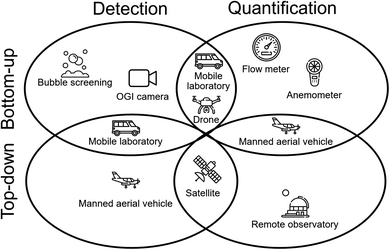 |
| Fig. 1 Illustration of the categorisation of technologies used to detect and quantify methane emissions in the oil and gas sector. The technologies shown are examples and a larger list of technologies can be found in the ESI.† The figure is adapted from one developed by National Academies of Sciences and Medicine.24 Please note that the figure gives examples of what kinds of technologies are top-down/bottom-up and detection/quantification and is not definitive. The classification of some technologies will vary depending on how it is used e.g., an OGI camera can be a quantification technology depending on whether or not a quantitative system is used;41 mobile labs can be a quantification technology if the measurement campaign intends to use the data collected to estimate emission rates. | |
2.3.1. Bottom-up approach and technologies.
In the bottom-up approach, emissions are measured at the source e.g. valve, pump, wellhead.24,25 The emission estimates are then multiplied by the number of components to estimate total facility or basin emissions. In this aspect, they are similar to emission factors. There is a wide range of bottom-up technologies, including point source, enclosed chambers and external tracers (Fig. 1).25,26,29
2.3.2. Top-down approach and technologies.
The top-down approach estimates emissions by measuring the atmospheric concentration of methane, over a given area, and then disaggregates this to derive the emission rate per component or facility.24,34 Essentially, it is the opposite to bottom-up. Remote sensing technologies (aircrafts, satellites, observation towers etc. (Fig. 1) are typical examples of top-down technologies.24,26
2.3.3. Comparing bottom-up to top-down and direct measurement to engineering calculations and emission factors.
There is no clear distinction between bottom-up and top-down technologies as the main difference is the method used to estimate emissions; extrapolated up or disaggregated.24 Therefore, for some technologies the mode in which they are used can shift it between the two and consequentially, some technologies can be both bottom-up and top-down. When comparing the direct measurement method to engineering calculations and emission factors, the direct measurement method is more accurate, as the level of detail and granularity in the emissions data are typically much greater.11,32
While considered to be more accurate, the emission estimates are subject to uncertainties. In addition to instrument uncertainty, in the bottom-up approach assumptions are made on the representativeness of the measurement sample when extrapolating.25 Similarly, in the top-down approach assumptions are made in the modelling to convert the methane concentration into a flow rate, as well as in disaggregating emissions.24 Therefore, it can be argued that direct measurement is not necessarily more accurate than engineering calculations and emission factors. However, this is the only method which can verify emission estimates and the data collected from measurement studies are crucial for updating emission factors and data used in engineering calculations. This method is also the only one out of the three that can identify previously unknown emission sources and account for super-emitters.
An important limitation in the accuracy of direct measurement emission estimates is the reconciling of emissions estimates.34 Numerous studies have compared bottom-up emission estimates to top-down estimates and have found significant differences. Alvarez, et al.13 found that emissions reported by the USA's Environmental Protection Agency (EPA) could be underreporting emissions by 60% compared to emissions measured in independent field studies. Similarly Chan, et al.35 found Canadian emissions could be underreported in Government inventories by a factor of two. Also, Kayrros, using data from the Sentinel-5P satellite, found emission events from Russian gas pipelines in 2020 were 40% higher than in 2019, despite the impacts on gas exports due to COVID-19.36 In general, top-down estimates tend to overestimate while bottom-up estimates tend to underestimate.37
In recent years, satellites have emerged as a promising technology which could aid the sector by providing site-level emission estimates.21,38–40 They have numerous benefits over other technologies but for them to benefit the sector, verifying the accuracy and usability of their data and derived results are imperative. Therefore, the questions raised regarding reconciling emissions estimated through top-down and bottom-up methods are important for establishing how useful satellites are and how they can be used to aid the sector in its emissions reporting.
3. Satellite overview-how they work, their capabilities and limitations and how they compare to other technologies
3.1. How satellites detect and measure methane
Satellites measure the atmospheric concentration of methane, typically the column-average dry mole fraction (XCH4). This is converted into a flux through atmospheric inversion modelling,42 from which emission sources can be detected. This is most commonly achieved through measuring the backscattering of sunlight; when sunlight is reflected from the Earth's surface into a spectrometer onboard a satellite.43 As sunlight travels through the atmosphere, it is absorbed and re-emitted by any gases it encounters, with certain gases emitting energy at specific wavelengths. The spectrometer analyses the incoming light for the relevant wavelengths, in this case those which are diagnostic of methane. Because sunlight is often impeded by particles, complementary measurements (e.g., of cloud coverage), taken by either the same or a different satellite, are used to determine the level of impediment. When obstructions are minimal, correction factors can be used to ensure measurements are accurate. However, at high impediment, data are discarded. This occurs either when no sunlight reaches the satellite or past a cut-off point in impediment where errors are considered too significant.
Inverse modelling is utilised to quantify emissions as tracking back methane in time from raw satellite measurements incurs high uncertainties. Consequentially, details in flux measurements are lost;44 inverse modelling lowers these uncertainties. The inverse modelling process uses atmospheric transport models to simulate the journey of methane molecules, dependant on observed emissions and a prior input data to determine its source. It requires meteorological, inventory and satellite data as inputs to solve the mass continuity equations. The two main types of models are Lagrangian particle dispersion models and Eulerian models.43 Inverse models have been well established for decades and are continuously improving.45 Examples of such models are Transport model version 5, (TM5)46 and Goddard Earth Observing System (GEOS-Chem).47 The resolution of these models has been shown to alter the final emissions estimate,48 highlighting a key uncertainty in their use. Many models are open source and have detailed user guides. In recent years the surge in satellites for methane quantification interest has launched the commercialisation of methane satellites data/products e.g., Kayrros, GHGSat and Bluefield. Further details on inverse modelling can be found in the ESI.†
The measurements taken by a satellite are a snapshot of the atmospheric concentration at a given moment,49 for given pixels (squares or circles within a grid).50 Therefore, any intra-daily variations e.g., intermittent or episodic emissions, are challenging to accurately measure because they are more difficult to profile than those which are constant. For example, if a source emits 90% of its emissions two hours after a satellite overpass, by the next overpass the methane could have been carried away and may not be detected. Conversely, if 90% of emissions are emitted in the hours before an overpass, it may be incorrectly attributed higher emissions if the emission rate is assumed to be constant. Repeat measurements help to reduce this effect, but it is not currently possible to vary the time at which a satellite takes measurements.
At the time of writing, 22 satellites capable of measuring methane have been launched (more information in the ESI†) and these broadly fall into one of two types based on their capabilities, which we refer to as Category 1 (C1) and Category 2 (C2) (Table 1). Satellites in general are not intended for the identification and quantification of individual emitting (point) sources, but rather long-term coverage of large areas, such as whole facilities or basins, as well as monitoring the global methane budget. Satellites are in most circumstances a detection technology because they measure the concentration per pixel, but have been used as a quantification tool under specific circumstances, for example unusually high emission events e.g. Aliso Canyon51 and XTO's Ohio well blowout.52 To further the field of oil and gas value chain emission analysis, open access satellite data are fundamental. Therefore, it is encouraging that MethaneSAT, a C2 satellite, will be providing its data to the public domain.53 There are two key satellite features that will be explored below:
Table 1 The two satellite categories and their characteristics. C2 satellites are dedicated microsatellites and are only equipped with a spectrometer for measuring methane and carbon dioxide. C1 satellites are not dedicated to methane and are equipped with equipment for other proposes e.g., aerosols, other greenhouse gases, ozone. Details on specific satellites can be found in Tables S1 and S2 in the ESI
Characteristic |
Category 1 |
Category 2 |
Category 1 satellite data is typically freely available to registered users while Category 2 satellite data typically must be purchased, though there are exceptions.
|
Sensitivity to methane |
High total column concentration |
Low total column concentration, differentiated from background |
Spatial resolution |
km2 |
m2 |
Pixel size |
5 to 60 km (diameter or width) |
10 to 40 m (width) |
Coverage |
Global or regional |
Targeted (oil and gas facilities) |
Return time |
Short (daily) |
Long (15 days) |
Data accessa |
Free |
Premium |
Example |
Sentinel-5P |
GHGSat |
GOSAT-2 |
• where measurements can be taken; and
• what their capabilities are?
These are interconnected and improving one can often improve the other.
3.2. Where satellites can take measurements-impeding factors
We examined the following factors that impede satellite usage: cloud coverage, surface albedo, wind and missing pixels. These are considered as they were determined to be the most important factors based on our review of the literature. Aerosols, latitude and surface terrain also effect satellite usability but are less impactful. These are not discussed here but information on their effect can be found in the ESI.†
3.2.1. Clouds.
Clouds are the most important limiting factor because they can prevent sunlight reaching the Earth's surface by either altering or blocking sunlight. Measurements can be taken over low cloud coverage e.g., Cirrus. However, refraction may occur and lead to inaccurate measurements. For thicker and denser clouds e.g., Nimbostratus, Stratus and Cumulonimbus, no detection can occur. The cloud fraction is the fraction of a pixel that is covered by clouds and is used to quantify the impact on measurement capabilities. The threshold at which no detection occurs, or data are rejected, varies by spectrometer e.g. 8 to 15% for TROPOMI onboard Sentinel-5P54 but for most satellites this information is not in the public domain at the time of writing. Examining cloud coverage worldwide, we find that large areas are unsuitable for daily monitoring with C1 satellites (Fig. 2), see the ESI† for more details. Increasing the number of C2 satellites in complementary orbits would increase the probability of acquiring viable data, as it would increase the likelihood of imaging through clouds (Section 3.3.1). For C1 satellites, when the cloud fraction threshold increases, more regions become suitable for daily measurement (see the ESI†). Also, when alternate days, weekly or monthly monitoring is permissible, more regions become suitable though it should be noted that periods of high cloud coverage are often seasonal which may impede the frequency of measurements taken (Fig. S2 and S3 in the ESI†).
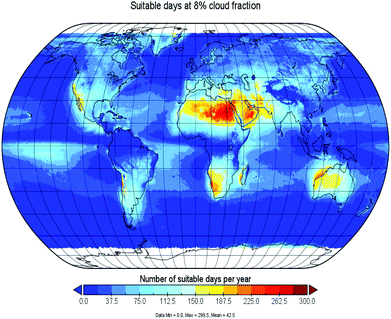 |
| Fig. 2 Impact of cloud coverage on suitability for measurement. Map is based on 8% cloud fraction using data collected from multiple ESA and NASA satellites. Areas in white indicate no data available. Suitability changes little between 8% and 15% cloud fraction (Fig. S2 in the ESI†). | |
3.2.2. Albedo.
Albedo is a measure of the amount of solar radiation reflected off the Earth's surface without being absorbed. Lighter, or brighter, surfaces have high albedo (high diffuse reflection of radiation) while dark surfaces have low albedo. This has an impact on satellite capabilities by impacting backscattering and radiation re-emittance, with low albedo regions not reflecting enough light to allow for measurements. The cut-off albedo for measurement is 0.02 in the shortwave infrared (SWIR) band for satellites such as Sentinel-5P54 and moreover, Schneising, et al.55 found that for albedo >0.03 the precision error is <1%. However, this cut-off affects only waterbodies, which are often pre-filtered out by satellites, though sunglint (reflection of sunlight over water, see ESI† for more information) has been positioned to overcome this.56 Other low albedo areas such as forests, which have albedos >0.1,57 should not be affected. Recently, Lorente, et al.58 analysed two years of TROPOMI data which showed an underestimation of methane at low albedo and an overestimation at high albedo. A bias correction factor was developed to account for this which removed most of the inaccuracies, but some remain at very high and low albedos such as snow.
3.2.3. Wind.
Satellite measurements are typically snapshots, with one measurement taken per day. Therefore, wind can impact the mixing of methane with other atmospheric constituents. Wind speed is linearly proportional to the detection limit of a satellite,43 thus without knowledge of this, emissions may go undetected. This can be troublesome if satellite data are being used for emission point-source identification. Wind effects the calculation of flux as wind speed and turbulence induce errors in the calculations. In general, large errors can be induced across all wind speeds because of unknown turbulence and variability in wind speed and direction at various altitudes. Depending on the method used to covert XCH4 into a flux, low wind speeds (∼2 m s−1) are suitable for detection but result in larger uncertainties (∼50%), which are bad for quantification.59 Improving data on wind speed and turbulence could help to overcome this.
3.2.4. The impact of missing pixels.
The above (Sections 3.2.1 to 3.2.3) have demonstrated the causes of missing pixels in satellite data. In general, this is a common feature of satellite data. Should satellites be used in regions near the sea, a body of water or mountains, coverage will be very low year-round, while in other areas it will be better but can still be patchy. To attempt to quantify the effect of missing data on the final methane enhancement detected by a satellite, TROPOMI data were collected for five countries (Australia, Canada, Iran, Saudi Arabia and the USA) over areas 2 degrees (latitude and longitude) in size. By withholding varying quantities of data and assuming an average methane enhancement of 5 ppb, we calculated the percentage error. Repeating this 10
000 times allowed for the variation between each level of data removal to be calculated.
As can be seen in Fig. 3, the uncertainty in the final methane enhancement rapidly increases over 70% of missing data. An interesting feature is that the uncertainty is largely dependent on the variation in methane levels in the area examined. Regions where background methane is relatively stable result in low uncertainty even when large quantities of data have been removed. Whereas areas where background levels varied had much higher uncertainties when smaller quantities of data were removed. Oil and gas regions of interest are likely to have high levels of variation in background methane levels due to the sporadic nature of their emission sources. This means low levels of missing data when quantifying emissions across large areas are vitally important.
 |
| Fig. 3 Uncertainty in final methane enhancement as a function of missing pixels. Calculated based on data for Australia, Canada, Iran, Saudi Arabia and the USA, using data collected by Sentinel-5P. Bounds display one standard deviation. | |
3.3. Satellite detection capabilities-resolution, detection limits, data processing, usage and comparison to other technologies
3.3.1. Resolution.
The spatial resolution of methane measuring satellites has greatly improved over the last 20 years, from 1800 km2 (60 × 30 km, Envisat) down to 38.5–49 km2 (5.5 × 7 km to 7 × 7 km, Sentinel-5P) and there is no reason to believe this trend will not continue. C2 satellites have pushed this even further proving that much higher resolutions (2.5 × 10−3 km2, 50 × 50 m) are possible. As resolution improves, the effect of clouds become less important, as there is a higher probability of imaging through gaps in clouds. For example, in a 10 km2 area, a satellite with a 10 km2 resolution would be impeded if 10% of this area is covered by clouds. However, if the satellite's resolution is 2.5 × 10−3 km2 (10 km2 area split into 4000 2.5 × 10−3 km2 pixels), then clouds would need to be present in every pixel for null measurements in the 10 km2 area. Therefore, as spatial resolution improves satellite measurements will be applicable to more areas.
3.3.2. Minimum detection limit (MDL).
The MDL restricts detection to specific emission rate thresholds, as shown in Table 2. As many of these correspond to very high emissions rates, most satellite studies have quantified emissions from large emission sources only.21,52,60,61 MDLs are calculated using the precision of the instrument (a quantification of the random errors associated with measurement) and the accuracy of the instrument (the systematic errors). The accuracy of the instrument cannot be improved with repeated measurements but may be accounted for with correction factors. The precision, on the other hand, can be improved over repeated measurements, thus lowering the MDL. This is because increasing the number of measurements increases confidence levels in the recorded values. For example, the MDL of Sentinel-5P could reach 500 kg CH4 per h per pixel assuming a 17% measurement success rate and 5 km h−1 wind speed over a year.43 Therefore, decreasing the effects of cloud coverage would effectively decrease the MDL over a prolonged measurement campaign as more days can be measured.
Table 2 Minimum detection limits (per pixel) of satellites.40,43,63,64 EnviSat, GOSAT-1/2 and Sentinel-5P are C1 satellites and GHGSat-C1/D and MethaneSAT are C2 satellites
Satellite |
MDLa (kg per CH4 per h) |
Mt CO2eq. per yearb |
MDL is sensitive to conditions during measurement. Less ideal conditions (cloud coverage and windy) will increase the MDL.
Assuming GWP of 29.8 (ref. 65) and continuous emissions i.e., 24 hours a day for 365 days. For contextual comparisons, 1241 Mt CO2 was emitted from US coal electricity and 546 Mt CO2 from natural gas electricity in 2016.66
GHGSat-C1 detection limit is anticipated MDL. At the time of writing, GHGSat-C1 is still undergoing testing to determine its detection and measurement capabilities.
MethaneSAT MDL is theoretical. It is scheduled to be launched in 2021/2022.
Detection limits are for a single measurement, increasing the number of measurements would decrease this.
|
EnviSat |
68 000 |
17.8 |
GHGSat-C1c (Iris) |
100 |
0.03 |
GHGSat-D (Claire) |
250–1000 |
0.1–0.3 |
GOSAT-1 |
7100 |
1.9 |
GOSAT-2 |
4000 |
1.0 |
MethaneSATd |
100–1800 |
0.03–0.5 |
Sentinel-5Pe |
3600–4200 |
0.9–1.1 |
However, satellites could be suitable for detecting and quantifying super-emitters, which we define as the 5% of sources responsible for approximately 50% of all oil and gas methane emissions.62 Satellites, despite having a high MDL, are capable of scanning large areas quickly and therefore can identify any high emitters (provided the emission rate is above the MDL) in a given area. However, it should be noted that satellites cannot be used to identify and account for all super-emitters. This is because a super-emitter is by definition a statistical outlier – the heavy tail in the distribution. An emission source with an emission rate much lower than the MDL of satellites, but consistently emits over time, can still be a super-emitter.
3.3.3. Data processing and calibration.
Data processing and calibration are features which play vital roles in assessing satellite suitability for methane quantification. A satellite must undergo calibration to ensure its measurements are correct. This often happens in the months after their launch,67 which often means their detection limits and precision are not known until they have been in use for some time. If this calibration is incorrect, all measurements will be incorrect. Satellites, such as Suomi-NPP are constantly improving calibration while in operation, with new models being developed to ensure accurate measurements.68 These will lead to increased use, greater agreement between satellites and ultimately more accurate results. The time needed and difficulty of data processing are other key factors in satellite use. Currently, only specialist companies and academic researchers attempt to quantify emissions using satellites, and these can take significant amounts of time. As methods are refined it is hoped that quantification will become more accessible and commonplace, especially as inverse modelling software is often open source. Within data processing, there are other limitations within the data and assumptions used to convert XCH4 into flux, notably: meteorological data and a prior data.
3.3.3.1. Uncertainty in meteorological data.
As satellite resolutions improve, limiting factors in their global use may become dependent on the resolution of meteorological grids. The data that are currently available give wind speeds at specific intervals but because of this, small-scale changes which occur in the real world are not captured. There is some literature on the uncertainty in meteorological data and the effect this could have on inverse modelling.69,70 However, these do not correlate the variation in spatial and temporal resolutions to uncertainties in specific locations. We found that changes in wind speed were normally distributed with an average change of 25% (see Section 4.2 in the ESI†). The wind speed also changes as we move through the atmosphere, with the lowest levels having the greatest change. When tracking a particle as it moves between grids, the uncertainties arising from the grid size also have a significant effect on the final particle location. This shows that when attributing emissions to individual pixels, the meteorological domain size could potentially affect emission estimates in individual pixel. However, if satellites trade granularity for lower uncertainty and merged pixels, such as when basins are examined, the effect of meteorological grid size becomes less important.
The uncertainties arising from meteorological data demonstrate that for individual facilities high resolution meteorological data are of paramount importance. On site measurements of local wind conditions could provide additional insight to the short-term changes in wind direction that are not captured by the large meteorological datasets.
3.3.3.2. A prior data.
A key component of inversion modelling is the inventory data used to allocate emissions to specific sources. However, within these inventories there are significant uncertainties. Fig. 4 shows an estimate of the uncertainty from EDGAR and WetCHARTS globally, adapted from Solazzo, et al.71 and Bloom, et al.72 (note this value will alter throughout the year). This gives further impetus to the suggestion that satellites are only suitable for certain locations, and for large methane sources.
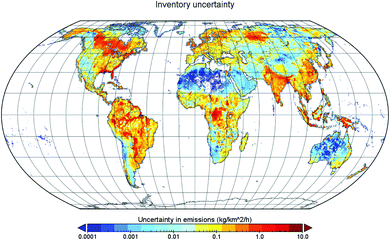 |
| Fig. 4 Uncertainties in inventory data; based on EDGAR and WetCHARTS, adapted from Solazzo, et al.71 and Bloom, et al.72 Blue indicates low uncertainty while red indicates high uncertainty. | |
3.3.4. Total uncertainties in satellite data and derived emission estimates.
There are numerous sources of uncertainty in the emission estimates derived from satellites and these are synthesised in Table 3. Overall, it is the satellite itself (hardware) that is the largest source of uncertainty, while uncertainties associated with the inversion model used is the second largest source. Uncertainties in a prior inventory and meteorological data are smaller sources but are still significant. It is not possible to combine the uncertainties to derive a single uncertainty value as they are independent from one another or are included in the inversion modelling e.g., uncertainties in meteorological data are included in the emission estimates when running an inversion model. Also, the uncertainties vary depending on which satellite data are collected and the quality of these data, which inversion method is used and what assumptions are made in the inversion modelling. However, it is evident that the emissions estimated carry high uncertainties, which are much higher than other technologies, see Table S4 in ESI,† and these should be taken into account when attempting to reconcile emission estimates.
Table 3 Sources of data uncertainty in satellite and inversion modelling
|
Uncertainty upper (increase from central value) |
Uncertainty lower (decrease from central value) |
Notes |
Satellite (instrument and measurements)
|
Precision |
+370% |
−370% |
The uncertainty due to the satellite instrumentation varies by pixel. The stated literature error value averages out to 1%. However, this 1% uncertainty refers to 1% of the measured column methane which usually ranges from 1700–1900 ppb, meaning ±17–19 ppb uncertainty. The relative% uncertainty of this will therefore change with the level of background methane in the study region |
Systematic |
+370% |
−370% |
The value stated here refers to an individual pixel and single measurement if a 5 ppb enhancement was detected against an 1850 ppb background. This would be viewed as being below the MDL of the satellite. As the size of the measured source, and the number of measurements taken increases, this uncertainty decreases. The lower uncertainty is above 100% in this scenario, meaning negative emission estimates are possible |
Missing data |
+11% |
−11% |
When 25% of data are missing (see Fig. 4) |
![[thin space (1/6-em)]](https://www.rsc.org/images/entities/char_2009.gif) |
Modelling
|
Inverse modelling |
+90% |
−90% |
When two models were used to measure the same emission source.73 |
![[thin space (1/6-em)]](https://www.rsc.org/images/entities/char_2009.gif) |
Uncertainty from within a prior inventory estimates of emissions
|
Energy |
+94.2% |
−60.4% |
EDGAR model.71 |
Industrial processes and product use (IPPU) |
+35.4% |
−53.4% |
EDGAR model.71 |
Agriculture |
+37.5% |
−30.6% |
EDGAR model.71 |
Waste |
+78.8% |
−77.7% |
EDGAR model.71 |
Other |
+117.3% |
−117.3% |
EDGAR model.71 |
Wetlands globally |
+25% |
−25% |
Global methane budget.3 |
Wetlands regionally |
+7800% |
−7800% |
WetCHARTS model,72 regionally differences are significant, it can reach up to a min max ratio of 156 in the tropics |
![[thin space (1/6-em)]](https://www.rsc.org/images/entities/char_2009.gif) |
Meteorological
|
Compared to real world data |
+10% |
−10% |
Varies depending on the dataset that is being used |
Grid size and temporal resolution |
+4.5% |
−4.5% |
Varies pixel by pixel |
3.4. The usage of satellites for methane detection and quantification-the literature
In the literature up to the time of writing, satellites are relatively new in comparison to other technologies. Therefore, there are fewer studies conducted which have used them for oil and gas methane detection and quantification. At the time of writing, as far as the authors are aware, there are six peer reviewed papers which have utilised satellites for oil and gas methane quantification (14 papers in total which use satellite to study oil and gas methane and largely focus on detection). In the non-peer reviewed literature, satellites have been used in campaigns by the Environmental Defence Fund (EDF) and in European studies, such as the Copernicus Programme and Kayrros.36,74–78 However, reports on their findings were not always publicly available.
In the peer reviewed literature, satellites have mostly been used to estimate emissions across whole basins, regions and countries. The studies are long term, ranging from one month to nine years in data collecting. Satellites have also been used to quantify large emission events: the TXO Ohio well blow out and Aliso Canyon gas leak.51,52 These usages are largely due to the measurement capabilities (resolution and detection limit) of satellites currently in orbit being much poorer than other technologies (Fig. 5). Despite this, satellites have been proven to produce intriguing results. For example, recent satellite studies have identified large methane anomalies such as in Florida in May 2020 (ref. 79) and Bangladesh in April 2021 (ref. 80).
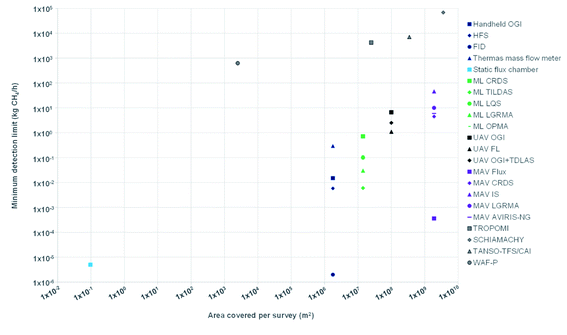 |
| Fig. 5 Comparison of methane emission quantification technologies based on what is reported in the peer reviewed literature. Please see Table S4 in the ESI† for limits in the MDL of the technologies listed. OGI: optical gas imaging camera; HFS: high flow sampler; FID: flame ionisation detector; ML: mobile laboratory; CRDS: cavity ring-down spectrometer; TILDAS: tuneable infrared laser differential absorption spectroscopy; LQS: line quantum sensor; LGRMA: los gatos research methane analyser; UAV: unmanned aerial vehicle; FL: feedback laser; TDLAS: tuneable diode laser absorption spectroscopy; MAV: manned aerial vehicle; IS: infrared imaging spectrometer; AVIRIS-NG: airborne visible infrared imaging spectrometer-next generation; TROPOMI: TROPOspheric monitoring instrument (device onboard Sentinel-5P satellite); SCHIAMACHY: scanning imaging absorption spectrometer for atmospheric CartograpHY (device onboard EnviSat satellite); TANSO-TFS/CAI: therman and near infrared sensor for carbon observation-fourier transport spectrometer/cloud and aerosol imager; WAF-P: wide-angle fabry-perot imaging spectrometer. | |
The emission estimates derived from satellite measurements have also been used in revising estimates, such as by the International Energy Agency (IEA) in their Methane Tracker.2 This highlights a potential common use of satellite data; the EU Methane Strategy, published in 2020, outlines the intention of establishing an international methane emissions observatory to monitor oil and gas emissions, and identifies satellites as a key technology for emissions data verification in the development of this observatory.38
3.5. Comparison to other technologies
The detection and measurement capabilities of satellites are, at the time of writing, poorer than other technologies as shown in Fig. 5. This is because the detection limit is much higher than other technologies and they take measurements per pixel rather than per component or facility (lower granularity). The technologies with the lowest detection limit and spatial coverage are point source technologies (e.g., flame ionisation detectors, optical gas imaging cameras, high flow samplers) and enclosed chambers, while technologies such as cavity ring-down spectrometers, methane analysers and lasers when equipped on vehicles (road or aerial) are intermediates between the point source technologies and satellites. Technologies such as spectrometers, chromatography analysis and laser spectroscopy when used in remote observatories and towers can produce similar data to satellites and have lower detection limits. However, they are only capable of estimating emissions over large areas and cannot distinguish between different emission sources (within anthropogenic and biogenic sources). All of these technologies are compatible with satellite data and can be directly compared and reconciled with emission estimates produced from satellite data, provided the emissions being compared are comparable.
As was inferred in previous sections, there are emissions sources satellites are unable to detect and will likely never be able to accurately detect: offshore emissions and subterranean seepage. Where satellites can be used, a challenge they face is the accuracy of their measurements. Satellite emission estimates have a high degree of uncertainty (Tables 3 and S4 in the ESI†). Therefore, their measurements should be used with caution if they are used in emission management decisions, as potential adverse impacts could result if the satellite overestimates or underestimates emissions; if overestimated more efforts and resources would be put into abating emissions than would otherwise be needed and emission management strategies appearing less (or not) effective. As was discussed in Section 3.3.2, the MDL of a satellite lowers with repeat measurements. Likewise, the accuracy of their emissions estimates (not to be confused with accuracy of the instrument as stated in Section 3.3.2) increases with repeat measurements. This is reflected in the peer reviewed literature as all the satellite studies were carried out over a minimum of one month. Similarly, the accuracy of other technologies also improves with repeat measurements, which is also reflected in the literature as multiple samples or surveys were carried out in the same area. From Fig. 5, it can be seen that there are overlaps in the measurement capabilities of the different technologies. Therefore, for accurate measurements the choice of technology used is less important, provided the technology is appropriate and properly calibrated for the emission source being measured, and the number of measurements taken is the more important factor.
Repeat measurements are also important for establishing inter-daily and intra-daily variations in emissions. As discussed in Section 3.1, this can significantly impede the accuracy of satellite measurements if the emission source is not constant and continuous. Intra-daily and inter-daily variation can be significant; differences in emission rates recorded for an abandoned well can vary by a factor of 18 over a day81 and similarly for whole oil and gas fields.82 All measurement technologies will fall victim to these types of variation, with some well suited to capturing intra-daily variation (enclosed chamber, perimeter facility, micrometeorological, remote observatories and towers). But without significant investment of time and money few are capable of accounting for inter-daily variation.
This is important for satellites as intra-daily variation cannot be explicitly measured due to long return times. Without knowledge of this variation in emissions, estimates are likely to be inaccurate, and have been shown to be a source of overestimation in top-down studies.83 Satellites could account for this variation via knowledge of facility operations and use. In the future, a fleet of satellites could measure at differing times to offset this weakness. Satellites are far more well suited to measuring inter-daily variation as they can measure continuously year-round with no added investment for the raw data. A time investment is required to turn the satellite data into a flux, but once models have been built and an area's methane sources mapped out, adding additional measurement days comes with little added cost. However, satellites must also be mindful of the variation in emissions from alternative methane sources. Inventories may account for monthly changes in estimates, but the daily variations will not be captured. Increasing the days examined will also aid in reducing these errors.
4. The role of satellites in emissions detection, reconciling and reporting
The detection of emissions and reconciling of emission estimates is important for improving the confidence, comprehensiveness and rigor of emissions reporting. This is one of the reasons why in the OGMP 2.0 framework, the highest level of reporting requires emissions reconciling between site and source level emissions,11 and why methane strategies, such as the EU methane strategy cite satellites as the technology for monitoring and tracking emissions.38 At the time of writing, there is a large data gap in oil and gas emissions accounting, especially in the international and national reporting2 as many countries rely on basic emission factors, developed by the IPCC for reporting in conjunction with the Kyoto Protocol,32 to estimate their oil and gas methane emissions. An allure of satellites, as was highlighted in Section 3, is their ability to take measurements over large areas quickly, while other technologies need more in terms of capital and person hours. Therefore, the need for satellites in improving emissions detection and reporting is one of the driving forces for their promoted use. However, their use in emissions detection, quantification and reporting is new compared to other technologies and consequentially there are still many factors either unknown or not well established on how the technology should and can be used.
At the time of writing, satellites are being used to detect and quantify methane emissions from the oil and gas sector (and other sectors). Based on our assessment of current satellite capabilities: their limiting factors, their use as reported in the literature and how they compare to other detection and measurement technologies, they can play an active role in emissions reconciling and reporting through the verification of emission estimates. For both C1 and C2 satellites, because of their high MDL and ability to scan large areas quickly, their measurements can be useful as an upper limit for checking whether extrapolated emission estimates and government inventory estimates are valid; identifying emission sources not accounted for by other methods and technologies; detecting the presence of super-emitters (Section 3.3.2). The verification of national GHG inventories is an area where satellites could have a particular prominent role; in North America national inventories reported by governments have been found to be under reporting by as much as a factor of two when compared with large independent field studies.13,35,84 However, the independent field studies took years to measure, collect and process the data. Satellites could significantly reduce the time (and resources) required to measure and collect data.
Their detection abilities offer the potential for improved identification of emission sources, specifically super-emitters. Given good conditions for measurements, all emission sources can be accounted for. Despite this, the accuracy of emission estimates compared to other technologies is, for the time being, not well established. In the literature, the authors are unaware of any studies which attempted to directly reconcile measurements taken using other methods to satellite measurements. While it is common to calibrate satellites through controlled release experiments, the direct comparison to other technologies is lacking. In 2020, ExxonMobil launched a large test study to assess the capabilities and scalability of numerous methane detection and quantification technologies, including satellites.85 While no results of this study have been made publicly available (at the time of writing), it is likely that direct comparisons and reconciling between satellites and other technologies would have been made. Because of this paucity in the literature, the authors cannot speculate on how accurate satellites are in comparison to other technologies. However, if the accuracy of satellite measurements relative to other technologies can be established, they would be a relatively cheap technology to use for establishing preliminary emission estimates (as field studies are expensive in terms of person-hours), from which targeted measurement studies, such as leak detection and repair (LDAR), can be carried out, or for creating country specific emission factors used in national and international reporting.
However, as was evident from our assessment of their measurement capabilities, their emission estimates hold large uncertainties, and it is only possible to use measurements derived from their data under certain circumstances-both C1 and C2. To be able to reconcile emission estimates to satellite derived measurements, it is important that the measurements are compatible, otherwise any attempts at reconciling will be invalid. For emission measurements taken by alternative technologies to be compatible, factors such as the timing of measurements taken, the duration of the survey and the quality of the data need to be considered. This is because satellites can only take one measurement per day and there are numerous factors (as discussed in Section 3) that can hinder their ability to take measurements. Ideally measurements taken by other technologies should coincide with the time of overpass of a satellite and the measurement campaign should be equal in duration to the number of days of satellite data used for comparison/reconciling i.e., if using one year of satellite data, the comparison technology/technologies must be used in a yearlong measurement campaign. Furthermore, because of the high unlikeliness of gathering good quality day-to-day data from satellites, they are better suited for long term emissions monitoring/measuring and thus should be reconciled with similar estimates.
When reconciling emission estimates, the high uncertainty in satellite estimates needs to be factored in, in addition to their inability to account for intra-daily variations in emissions. The authors are of the opinion that provided the (alternative technology) emission estimate is within the uncertainty bounds of the satellite estimate, then this can be considered a successful reconciliation (Fig. 6), given the numerous uncertainties and assumptions which need to be made in both satellite derived estimates and other methods. However, as there have been no reconciliation studies conducted, the authors are unable to advise on what specific actions should be taken if reconciling is unsuccessful. Based on our assessment of satellite capabilities and our knowledge of the capabilities of other methods, actions that could be taken to resolve failures in reconciling include:
 |
| Fig. 6 Illustration of reconciling between satellite emission estimate and bottom-up (BU) estimate, showing when action would be needed and when reconciliation can be considered successful. Note: not based on any measurement data and is for purely illustrative purposes only. | |
• further surveying the study area if the satellite measurements identified emission sources not accounted for in the other method;
• using the emissions data reported by the alternative method to run forward mode atmospheric transport models and compare this to the satellite measurement to determine discrepancy causes;
• recalculate the satellite derived emissions using alternative inversion models and assumptions; and
• identify any potential methane anomalies which could have impacted either the satellite or alternative method e.g., well blowout, large release of methane from nearby sources (e.g. landfills, wetlands, coal mines).
These are suggestions based on our assessment of satellites and other methane detection and quantification technologies and are not intended to be taken explicitly. If successful reconciliations can be made then satellites will significantly improve the confidence in emissions reporting, which will in turn improve emissions monitoring and tracking and consequentially, reporting.
In addition to emissions reporting and monitoring, satellites could play a key role in ensuring the effectiveness of policy tools such as the EU Methane Supply Index-an inventory which allows gas buyers to choose where to purchase natural gas from based on the methane intensity of the supply chain,38 or guarantee of origin schemes similar to those for green gas and electricity.86,87 While satellites are not capable of estimating the emissions intensities of the various activities in the gas value chain, they can monitor the methane concentration over production, processing and liquefaction facilities to determine whether there are any spikes. These uses of satellites could also be applied to other important sources of methane: agriculture, waste management and bioenergy and could help improve the understanding of the global methane budget, through better accounting of sources.
Satellites could also play a key role in long-term emissions monitoring on a global, regional or local scale. Their data could be combined with data collected from remote observatories or towers and daily or even hourly emission data could be generated, allowing for more accurate emissions record keeping and tracking. If these data are combined with satellite imaging data, a heat map of emissions could be generated to view the changes in emissions/methane concentration in an area with time, similar to tools that have already been developed by the National Aeronautics and Space Administration (NASA) and GHGSat.88,89 However, due to limitations in data processing, a tool such as this is highly unlikely to allow for real-time emissions reporting. Also, due to limitations in resolution (technological as well as legal/privacy), resolutions are likely to be limited to the facility level.
Overall, it is the authors' view that satellites could provide much needed confidence and rigor to emissions reporting. Satellites could also be a valuable tool in advancing methane detection, monitoring and tracking, either in aiding policy tools or in developing new mapping tools, as well in improving the understanding of the global methane budget. However, there is a large gap in the literature around reconciling measurements and consequentially the authors are unable to speculate on aspects on satellite use in emissions reporting and reconciling, including the accuracy of satellite derived measurements and more specific actions to take when addressing failures in reconciling.
5. Recommendations for future work and how to further the usage of satellites in emissions detection and quantification
From our analysis of the factors which impact satellite measurement capabilities, we suggest the following as areas of particular interest/importance:
• further modelling to determine the impact of wind speed and turbulence on flux estimation and the impact of gas mixing in the column;
• combining or comparing data from multiple satellites to see if there is any variation in emission quantification; and.
• further measurement campaigns, including controlled release experiments, using a mixture of technologies, including satellites.
As there were no studies which attempted to directly reconcile satellite measurements with other technologies, filling in this knowledge gap would greatly contribute towards a better understanding of how to reconcile measurements. Therefore, we suggest future studies should attempt to reconcile satellite measurements with measurements taken using other top-down technologies, as well as bottom-up technologies.
6. Conclusions
There are several technologies available to the oil and gas sector for detecting and quantifying their methane emissions, of which satellites offer superior scale and speed. However, because of limitations to where and when measurements can be taken and because of high uncertainties in their derived emission estimates, they are unsuitable for quantifying all oil and gas methane emissions. Despite this, we find that satellites are a valuable tool for emissions reconciling as they could allow for more robust and confident emissions detection and reporting.
When using satellites, care should be taken when selecting the study area as they can become highly restricted in where and when emissions can be detected and measured because of factors which impact their capabilities: clouds, albedo, quality of a prior data etc. Thus any emissions estimated carry much higher uncertainties than other methods. Also, because of limitations to their measurement abilities (one measurement per day which is a snapshot), reconciliation can only truly be carried out if the estimates being compared to are compatible.
At the time of writing, satellites are being used to quantify emissions and have been identified as a key technology for emissions monitoring and tracking. In emissions reconciling, their data can be used to establish an upper limit in emissions over an area or facility/site, identifying any previously unknown emission sources or detecting super-emitters. There was limited literature found that focused on directly reconciling satellite derived emission estimates with other methods and thus the authors were unable to deduce their accuracy in comparison to other detection and quantification technologies. As the accuracy and reliability of satellite derived emission estimates could not be determined, it is uncertain to what degree satellites over or underestimate emissions. Repeat measurements can improve the accuracy and reliability of emission estimates, in particular for asserting what inter-daily variations occur which would contribute towards improved characterisation of emission profiles.
In emissions reporting, satellites could allow for increased confidence in reporting, as well as aiding in the development of emission factors for countries or regions where there are large data gaps in emissions data. They are also a valuable tool for tracking and monitoring methane, both on a global and local scale. Their data can be used to monitor the atmospheric methane concentration in areas of oil and gas activity, from which spikes or dips in concentration can be determined i.e., to assess the effectiveness of abatement measures. If their data are combined with emission data collected from other technologies, such as observatories, methane mapping tools could be developed, which could transform emissions reporting tools and products.
Overall, at the time of writing, there are many barriers to satellite usage in emissions reporting and reconciling but if these can be overcome, then they would significantly aid the oil and gas sector in their emissions reporting through more robust and confident reporting and methodological rigour. There are currently many data gaps in the satellite for methane detection and quantification literature, particularly on reconciling with other emission estimates and how different factors (meteorological data, inversion modelling assumptions etc.) affect the derived results. Hence, more work is needed to better understand the abilities of this technology, as well as how far it can be relied upon for emissions reporting, tracking and monitoring.
Author contributions
Jasmin Cooper: conceptualization, methodology, formal analysis, investigation, writing – original draft, writing – review & editing, visualization, project administration. Luke Dubey: methodology, software, formal analysis, investigation, writing – original draft, writing – review & editing. Adam Hawkes: conceptualization, methodology, writing – review & editing.
Conflicts of interest
There are no conflicts to declare.
Acknowledgements
The authors would like to acknowledge the funding from the Sustainable Gas Institute, founded by Imperial College London and BG Group (now part of Royal Dutch Shell). Funding for the Sustainable Gas Institute is gratefully received from Royal Dutch Shell. Note, the funding bodies had no influence on the findings and conclusions drawn in this study.
References
-
G. Myhre, D. Shindell, F.-M. BréOn, W. Collins, J. Fuglestvedt and J. Huang, et al., Anthropogenic and Natural Radiative Forcing. In: Climate Change 2013: The Physical Science Basis, Contribution of Working Group I to the Fifth Assessment Report of the Intergovernmental Panel on Climate Change, ed. T. F. Stocker, D. Qin, G.-K. Plattner, M. Tignor, S. K. Allen and J. Boschung, et al., Cambridge University Press, Cambridge, United Kingdom and New York, NY, USA, 2013 Search PubMed.
-
Iea, Methane Tracker 2021, International Energy Agency (IEA), Paris, FR, 2021, available, https://www.iea.org/reports/methane-tracker-2021 Search PubMed.
- M. Saunois, A. R. Stavert, B. Poulter, P. Bousquet, J. G. Canadell and R. B. Jackson,
et al., The Global Methane Budget 2000–2017, Earth System Science Data, 2020, 12(3), 1561–1623 CrossRef.
-
Z. Hausfather, UNEP: Net-zero pledges provide an ‘opening’ to close growing emissions ‘gap’, Carbon Brief, 2020, https://www.carbonbrief.org/unep-net-zero-pledges-provide-an-opening-to-close-growing-emissions-gap Search PubMed.
-
UK Oil and Gas Authority, New net zero requirement for UK oil and gas industry, UK Oil and Gas Authority (OGA), London, UK, 2020, press release Search PubMed.
-
British Petroleum (BP), BP sets ambition for net zero by 2050, fundamentally changing organisation to deliver, British Petroleum (BP), London, UK, 2020, press Search PubMed.
-
Shell, Shell accelerates drive for net-zero emissions with customer-first strategy, Royal Dutch Shell, The Hague, NL, 2021, cited 2021 11th February, available from, https://www.shell.com/media/news-and-media-releases/2021/shell-accelerates-drive-for-net-zero-emissions-with-customer-first-strategy.html Search PubMed.
-
R. Bousso and M. Green, BP unveils measures to battle methane leaks, Reuters, 2019, https://uk.reuters.com/article/uk-bp-methane/bp-unveils-measures-to-battle-methane-leaks-idUKKCN1VV199 Search PubMed.
-
Gmi, UNEP: Oil and Gas Methane Partnership Initiative to Manage Methane Emissions from Upstream Oil and Gas Operations, Global Methane Initiative (GMI), 2020, available from, https://globalmethane.org/challenge/ogmp.html Search PubMed.
-
M. Lammey, BP invests $5m as part of crusade against methane leaks Energy Voice, 2020 Search PubMed.
-
Ccac, Oil and Gas Methane Partnership (OGMP) 2.0 Framework, Climate and Clean Air Coalition (CCAC), Paris, FR, 2020, available, https://www.ccacoalition.org/en/resources/oil-and-gas-methane-partnership-ogmp-20-framework Search PubMed.
- D. T. Allen, A. P. Pacsi, D. W. Sullivan, D. Zavala-Araiza, M. Harrison and K. Keen,
et al., Methane Emissions from Process Equipment at Natural Gas Production Sites in the United States: Pneumatic Controllers, Environ. Sci. Technol., 2015, 49(1), 633–640 CrossRef CAS PubMed.
- R. A. Alvarez, D. Zavala-Araiza, D. R. Lyon, D. T. Allen, Z. R. Barkley and A. R. Brandt,
et al., Assessment of methane emissions from the U.S. oil and gas supply chain, Science, 2018, 361(6398), 186 CAS.
- Y. Y. Cui, J. Brioude, S. A. Mckeen, W. M. Angevine, S.-W. Kim and G. J. Frost,
et al., Top-down estimate of methane emissions in California using a mesoscale inverse modeling technique: The South Coast Air Basin. Journal of Geophysical Research:, Atmospheres, 2015, 120(13), 6698–6711 CAS.
- C. S. Foster, E. T. Crosman, L. Holland, D. V. Mallia, B. Fasoli and R. Bares,
et al., Confirmation of Elevated Methane Emissions in Utah's Uintah Basin With Ground-Based Observations and a High-Resolution Transport Model, J. Geophys. Res.: Atmos., 2017, 122(23), 13026–13044 CAS.
- S. V. Jagovkina, I. L. Karol, V. A. Zubov, V. E. Lagun, A. I. Reshetnikov and E. V. Rozanov, Reconstruction of the methane fluxes from the west Siberia gas fields by the 3D regional chemical transport model, Water, Air, Soil Pollut.: Focus, 2000, 34(29–30), 5319–5328 CAS.
- A. Karion, C. Sweeney, E. A. Kort, P. B. Shepson, A. Brewer and M. Cambaliza,
et al., Aircraft-Based Estimate of Total Methane Emissions from the Barnett Shale Region, Environ. Sci. Technol., 2015, 49(13), 8124–8131 CrossRef CAS PubMed.
- J. Peischl, T. B. Ryerson, K. C. Aikin, J. A. De Gouw, J. B. Gilman and J. S. Holloway,
et al., Quantifying atmospheric methane emissions from the Haynesville, Fayetteville, and northeastern Marcellus shale gas production regions, J. Geophys. Res.: Atmos., 2015, 120(5), 2119–2139 CAS.
- A. Townsend-Small, D. Disbennett, J. M. Fernandez, R. W. Ransohoff, R. Mackay and R. A. Bourbonniere, Quantifying emissions of methane derived from anaerobic organic matter respiration and natural gas extraction in Lake Erie, Limnol. Oceanogr., 2016, 61, S356–S366 CrossRef.
- T. I. Yacovitch, C. Daube and S. C. Herndon, Methane Emissions from Offshore Oil and Gas Platforms in the Gulf of Mexico, Environ. Sci. Technol., 2020, 54(6), 3530–3538 CrossRef CAS PubMed.
- Y. Zhang, R. Gautam, S. Pandey, M. Omara, J. D. Maasakkers and P. Sadavarte,
et al., Quantifying methane emissions from the largest oil-producing basin in the United States from space, Sci. Adv., 2020, 6(17), eaaz5120 CrossRef CAS PubMed.
- T. A. Fox, T. E. Barchyn, D. Risk, A. P. Ravikumar and C. H. Hugenholtz, A review of close-range and screening technologies for mitigating fugitive methane emissions in upstream oil and gas, Environ. Res. Lett., 2019, 14(5), 053002 CrossRef CAS.
- A. R. Ravikumar, S. Sreedhara, J. F. Wang, J. Englander, D. Roda-Stuart and C. Bell,
et al., Single-blind inter-comparison of methane detection technologies - results from the Stanford/EDF Mobile Monitoring Challenge, Elementa: Science of the Anthropocene, 2019, 7, 16 Search PubMed.
-
National Academies of Sciences E. and Medicine, Improving Characterization of Anthropogenic Methane Emissions in the United States, The National Academies Press, Washington, DC, 2018, p. 250 Search PubMed.
-
Methane Guiding Principles, Reducing Methane Emissions: Best Practice Guide Identification, Detection, Measurement and Quantification, Methane Guiding Principles, 2020, available, https://methaneguidingprinciples.org/best-practice-guides/ Search PubMed.
-
P. F. Brown, S. Saunier, M. Simon and B. H. Cruz, Overview of methane detection and measurement technologies for offshore applications, Carbon Limits AS for the Norwegian Oil and Gas Association, Oslo, NO, 2020, available, https://www.carbonlimits.no/wp-content/uploads/2020/08/Methane-measurement-technologies-offshore_for-website.pdf Search PubMed.
-
Marcogaz, Guidance for using the MARCOGAZ methane emissions reporting template, MARCOGAZ, Brussels, BE, 2020, available, https://www.marcogaz.org/wp-content/uploads/2021/04/WG_ME-710.pdf Search PubMed.
-
G. Heath
E. Warner
D. Steinberg and A. Brandt, Estimating U.S. Methane Emissions from the Natural Gas Supply Chain, Approaches, Uncertainties, Current Estimates and Future Studies. Golden, CO, USA, The Joint Institute for Strategic Energy Analysis, the U.S. Department of Energy’s National Renewable Energy Laboratory, the University of Colorado-Boulder, the Colorado School of Mines, the Colorado State University, the Massachusetts Institute of Technology, and Stanford University; 2015, available, https://www.nrel.gov/docs/fy16osti/62820.pdf.
-
T. Haugland, Best Practice Guidance for Effective Methane Management in the Oil and Gas Sector: Monitoring, Reporting and Verification (MRV) and Mitigation, Carbon Limits for the United Nations Economic Commission for Europe (UNECE), Geneva, CH, 2019, available, https://unece.org/sustainable-energymethane-management/best-practice-guidance-effective-methane-management-oil-and Search PubMed.
- G. Li, M. Yang, Y. Zhang, J. Grace, C. Lu and Q. Zeng,
et al., Comparison model learning methods for methane emission prediction of reservoirs on a regional field scale: Performance and adaptation of methods with different experimental datasets, Ecological Engineering, 2020, 157, 105990 CrossRef.
- B. Nowakowicz-Dębek, S. A. Wlazło Ł., M. Ossowski, M. Kasela and A. Chmielowiec-Korzeniowska,
et al., Estimating Methane Emissions from a Dairy Farm Using a Computer Program, Atmosphere, 2020, 11(8), 803 CrossRef.
-
Ipcc, 2006 IPCC Guidelines for National Greenhouse Gas Inventories, Prepared by the National Greenhouse Gas Inventories Programme, Kanagawa, JP, 2006, available, https://www.ipcc-nggip.iges.or.jp/public/2006gl/ Search PubMed.
- B. K. Lamb, S. L. Edburg, T. W. Ferrara, T. Howard, M. R. Harrison and C. E. Kolb,
et al., Direct Measurements
Show Decreasing Methane Emissions from Natural Gas Local Distribution Systems in the United States, Environ. Sci. Technol., 2015, 49(8), 5161–5169 CrossRef CAS PubMed.
- D. T. Allen, Methane emissions from natural gas production and use: Reconciling bottom-up and top-down measurements, Curr. Opin. Chem. Eng., 2014, 5, 78–83 CrossRef.
- E. Chan, D. E. J. Worthy, D. Chan, M. Ishizawa, M. D. Moran and A. Delcloo,
et al., Eight-Year Estimates of Methane Emissions from Oil and Gas Operations in Western Canada Are Nearly Twice Those Reported in Inventories, Environ. Sci. Technol., 2020, 54(23), 14899–14909 CrossRef CAS PubMed.
-
P. Neill, Methane emissions events in Russia increased by 40% in 2020, Environment Journal, 2021, https://environmentjournal.online/articles/methane-emissions-events-in-russia-increased-by-40/ Search PubMed.
- D. Allen, Attributing Atmospheric Methane to Anthropogenic Emission Sources, Acc. Chem. Res., 2016, 49(7), 1344–1350 CrossRef CAS.
-
European Commission, EU Methane Strategy, European Union (EU), Brussels, BE, 2020, available, https://ec.europa.eu/energy/sites/ener/files/eu_methane_strategy.pdf Search PubMed.
- Y. Zhang, R. Gautam, D. Zavala-Araiza, D. J. Jacob, R. Zhang and L. Zhu,
et al., Satellite-Observed Changes in Mexico's Offshore Gas Flaring Activity Linked to Oil/Gas Regulations, Geophys. Res. Lett., 2019, 46(3), 1879–1888 CrossRef.
-
J. Elkind, E. Blanton, H. D. V. D. Gon, R. L. Kleinberg and A. Leemhus, Nowhere to hide: the implications of satellite-based methane detection for policy, industry, and finance, Columbia University SIPA and Netherlands Organisation for Applied Scientific Research (TNO), New York City, NY, USA, 2020, available, https://www.energypolicy.columbia.edu/research/commentary/nowhere-hide-implications-policy-industry-and-finance-satellite-based-methane-detection Search PubMed.
-
Teledyne Flir, FLIR QL320 Wilsonville, OR, USA, Teledyne FLIR, 2021, available from, https://www.flir.co.uk/products/flir-ql320/.
- S. Houweling, P. Bergamaschi, F. Chevallier, M. Heimann, T. Kaminski and M. Krol,
et al., Global inverse modeling of CH4 sources and sinks: an overview of methods, Atmos. Chem. Phys., 2017, 17(1), 235–256 CrossRef CAS.
- D. J. Jacob, A. J. Turner, J. D. Maasakkers, J. Sheng, K. Sun and X. Liu,
et al., Satellite observations of atmospheric methane and their value for quantifying methane emissions, Atmos. Chem. Phys., 2016, 16(22), 14371–14396 CrossRef CAS.
-
R. Blayo, M. Bocquet, E. Cosme and L. F. Cugliandolo, Advanced data assimilation for geosciences: Ecole de Physics des Houches, Oxford University Press, Oxford, UK, 2015 Search PubMed.
-
Geos-Chem, Narrative description, GEOS-Chem, 2021, available from, http://acmg.seas.harvard.edu/geos/geos_chem_narrative.html Search PubMed.
- P. Bergamaschi, M. Krol, F. Dentener, A. Vermeulen, F. Meinhardt and R. Graul,
et al., Inverse modelling of national and European CH4 emissions using the atmospheric zoom model TM5, Atmos. Chem. Phys., 2005, 5(9), 2431–2460 CrossRef CAS.
- I. Bey, D. J. Jacob, R. M. Yantosca, J. A. Logan, B. D. Field and A. M. Fiore,
et al., Global modeling of tropospheric chemistry with assimilated meteorology: Model description and evaluation, J. Geophys. Res.: Atmos., 2001, 106(D19), 23073–23095 CrossRef CAS.
- I. Stanevich, D. B. A. Jones, K. Strong, R. J. Parker, H. Boesch and D. Wunch,
et al., Characterizing model errors in chemical transport modeling of methane: impact of model resolution in versions v9-02 of GEOS-Chem and v35j of its adjoint model, Geosci. Model Dev., 2020, 13(9), 3839–3862 CrossRef CAS.
-
Esa, Orbit Prediction Tool, European Space Agency (ESA), 2021, available from, https://evdc.esa.int/orbit/ Search PubMed.
-
Esa, Sentinel-5P TROPOMI User Guide, European Space Agency (ESA), Sentinel, 2021, available from, https://sentinel.esa.int/web/sentinel/user-guides/sentinel-5p-tropomi Search PubMed.
- D. R. Thompson, A. K. Thorpe, C. Frankenberg, R. O. Green, R. Duren and L. Guanter,
et al., Space-based remote imaging spectroscopy of the Aliso Canyon CH4 superemitter, Geophys. Res. Lett., 2016, 43(12), 6571–6578 CrossRef CAS.
- S. Pandey, R. Gautam, S. Houweling, H. D. Van Der Gon, P. Sadavarte and T. Borsdorff,
et al., Satellite observations reveal extreme methane leakage from a natural gas well blowout, Proc. Natl. Acad. Sci. U. S. A., 2019, 116(52), 26376–26381 CrossRef CAS PubMed.
-
J. Coifman, MethaneSAT, 2020, cited 2020, available from, https://www.methanesat.org/2020/02/12/software-engineer-tom-melendez-joins-methanesat-will-head-development-of-missions-groundbreaking-data-platform/ Search PubMed.
-
O. Hasekamp, A. Lorente, H. Hu, A. Butz, J. a. D. Brugh and J. Landgraf, Algorithm Theoretical Baseline Document for Sentinel-5 Precursor Methane Retrieval, Netherlands Institute for Space REsearch (SROM), Utrecht, NL, 2019, available, https://sentinel.esa.int/documents/247904/2476257/Sentinel-5P-TROPOMI-ATBD-Methane-retrieval Search PubMed.
- O. Schneising, M. Buchwitz, M. Reuter, H. Bovensmann, J. P. Burrows and T. Borsdorff,
et al., A scientific algorithm to simultaneously retrieve carbon monoxide and methane from TROPOMI onboard Sentinel-5 Precursor, Atmos. Meas. Tech., 2019, 12(12), 6771–6802 CrossRef CAS.
- N. Larsen and K. Stamnes, Methane detection from space: use of sunglint, Opt. Eng., 2006, 45(1), 016202 CrossRef.
- B. G. B. Forests and C. Change, Forcings, Feedbacks, and the Climate Benefits of Forests, Science, 2008, 320(5882), 1444–1449 CrossRef PubMed.
- A. Lorente, T. Borsdorff, A. Butz, O. Hasekamp, J. Aan De Brugh and A. Schneider,
et al., Methane retrieved from TROPOMI: improvement of the data product and validation of the first 2 years of measurements, Atmos. Meas. Tech., 2021, 14(1), 665–684 CrossRef CAS.
- D. J. Varon, D. J. Jacob, J. Mckeever, D. Jervis, B. O. A. Durak and Y. Xia,
et al., Quantifying methane point sources from fine-scale satellite observations of atmospheric methane plumes, Atmos. Meas. Tech., 2018, 11(10), 5673–5686 CrossRef CAS.
- J. A. De Gouw, J. P. Veefkind, E. Roosenbrand, B. Dix, J. C. Lin and J. Landgraf,
et al., Daily Satellite Observations of Methane from Oil and Gas Production Regions in the United States, Sci. Rep., 2020, 10(1), 1379 CrossRef CAS.
- D. J. Varon, J. Mckeever, D. Jervis, J. D. Maasakkers, S. Pandey and S. Houweling,
et al., Satellite Discovery of Anomalously Large Methane Point Sources From Oil/Gas Production, Geophys. Res. Lett., 2019, 46(22), 13507–13516 CrossRef CAS.
- A. R. Brandt, G. A. Heath and D. Cooley, Methane Leaks from Natural Gas Systems Follow Extreme Distributions, Environ. Sci. Technol., 2016, 50(22), 12512–12520 CrossRef CAS.
-
Methanesat, MethaneSAT: Putting the Brakes on Climate Change, Environmental Defence Fund (EDF), New York, NY, USA, 2019, available, https://www.edf.org/sites/default/files/MethaneSAT%20Technical%20considerations-May%202019.pdf Search PubMed.
-
Rmi, What is methane and why should you care?, Rocky Mountin Institute (RMI) and SYSTEMIQ, Basalt, CO, USA, 2019, available, https://rmi.org/wp-content/uploads/2019/09/What-is-Methane-and-Why-Should-You-Care.pdf Search PubMed.
-
Ipcc, AR6 Climate Change 2021: The Physical Science Basis, Intergovernmental Panel on Climate Change (IPCC), Geneva, CH, 2021, available, https://www.ipcc.ch/report/ar6/wg1/ Search PubMed.
-
Epa, Inventory of U.S. Greenhouse Gas Emissions and SInks: 1990-2016, United States Environmental Protection Agency (EPA), Washington D.C., USA, 2018, available, https://www.epa.gov/sites/production/files/2018-01/documents/<?pdb_no 2018?>2018<?pdb END?>_complete_report.pdf Search PubMed.
-
Esa, GHGSat-D - Satellite Missions - eoPortal Directory, 2021, available from, https://directory.eoportal.org/web/eoportal/satellite-missions/g/ghgsat-d Search PubMed.
- Q. Zheng, Q. Weng and K. Wang, Developing a new cross-sensor calibration model for DMSP-OLS and Suomi-NPP VIIRS night-light imageries, ISPRS Journal of Photogrammetry and Remote Sensing, 2019, 153, 36–47 CrossRef.
- A. Ershadi, M. F. Mccabe, J. P. Evans, G. Mariethoz and D. A. Kavetski, Bayesian analysis of sensible heat flux estimation: Quantifying uncertainty in meteorological forcing to improve model prediction, Water Resour. Res., 2013, 49(5), 2343–2358 CrossRef.
-
W. Pendergrass, Evaluation of NOAA/NCEP's North America Mesoscale (NAM) 12-km and 4-km High-Resolution Nest (NAM4) Forecast for a typical Southern Temperate Deciduous Forest, 2017, conference proceedings Search PubMed.
- E. Solazzo, M. Crippa, D. Guizzardi, M. Muntean, M. Choulga and G. Janssens-Maenhout, Uncertainties in the Emissions Database for Global Atmospheric Research (EDGAR) emission inventory of greenhouse gases, Atmos. Chem. Phys., 2021, 21(7), 5655–5683 CrossRef CAS.
-
A. A. Bloom, K. W. Bowman, M. Lee, A. J. Turner, R. Schroeder, J. R. Worden, et al., CMS: Global 0.5-deg Wetland Methane Emissions and Uncertainty (WetCHARTs v1.0), ORNL Distributed Active Archive Center, 2017 Search PubMed.
- R. R. Thiruvenkatachari, V. Carranza, F. Ahangar, A. Marklein, F. Hopkins and A. Venkatram, Uncertainty in using dispersion models to estimate methane emissions from manure lagoons in dairies, Agricultural and Forest Meteorology, 2020, 290, 108011 CrossRef.
-
C. Leyden, Energy Exchange, Environmental Defence Fund (EDF), New York, NY, USA, 2019, cited 2021, available from, http://blogs.edf.org/energyexchange/2019/01/24/satellite-data-confirms-permian-gas-flaring-is-double-what-companies-report/ Search PubMed.
-
Esa, Sentinel-5P, The European Space Agency (ESA), 2020, cited 2021], available from, https://www.esa.int/Applications/Observing_the_Earth/Copernicus/Sentinel-5P/Mapping_methane_emissions_on_a_global_scale Search PubMed.
-
A. Clark, Methane Plumes Spotted Near Central Asia Pipes to China, Russia, Bloomberg Green, 2021, https://www.bloomberg.com/news/articles/2021-09-16/methane-plumes-spotted-near-turkmenistan-pipes-to-china-russia Search PubMed.
-
A. Rathi and K. A. Ansary, Large methane cloud in Iraq coincided with gas pipeline leak, Bloomberg Green, 2021, https://www.bloomberg.com/news/articles/2021-08-16/satellite-detected-methane-near-iraq-gas-pipeline-leak Search PubMed.
-
A. Rathi and N. Bisenov, Huge methane cloud spotted near gas pipeline that supplies China, Bloomberg Green, 2021, https://www.bloomberg.com/news/articles/2021-08-02/methane-leak-detected-near-gas-pipeline-that-supplies-china Search PubMed.
-
Bluefield takes the lead in methane detection: the Florida leak, New York City, NY, USA: Bluefield, 2020, press release, https://bluefield.co/press-release-florida-methane-0720/ Search PubMed.
-
A. Clark, J. Wu and A. Devnath, Mysterious Plumes of Methane Gas Appear Over Bangladesh, Bloomberg Quint, 2021, https://www.bloombergquint.com/politics/mysterious-plumes-of-methane-gas-appear-over-bangladesh Search PubMed.
- S. N. Riddick, D. L. Mauzerall, M. A. Celia, M. Kang and K. Bandilla, Variability observed over time in methane emissions from abandoned oil and gas wells, Int. J. Greenhouse Gas Control, 2020, 100, 103116 CrossRef CAS.
- O. Schneising, M. Buchwitz, M. Reuter, S. Vanselow, H. Bovensmann and J. P. Burrows, Remote sensing of methane leakage from natural gas and petroleum systems revisited, Atmos. Chem. Phys., 2020, 20(15), 9169–9182 CrossRef CAS.
- T. L. Vaughn, C. S. Bell, C. K. Pickering, S. Schwietzke, G. A. Heath and G. Pétron,
et al., Temporal variability largely explains top-down/bottom-up difference in methane emission estimates from a natural gas production region, Proc. Natl. Acad. Sci. U. S. A., 2018, 115(46), 11712–11717 CrossRef CAS PubMed.
- K. Mackay, M. Lavoie, E. Bourlon, E. Atherton, E. O’connell and J. Baillie,
et al., Methane emissions from upstream oil and gas production in Canada are underestimated, Sci. Rep., 2021, 11(1), 8041 CrossRef CAS.
-
S. Singh, ExxonMobil tests new technologies to slash methane emissions, Reuters, 2020, https://uk.reuters.com/article/us-exxon-mobil-methane/exxonmobil-tests-new-technologies-to-slash-methane-emissions-idUKKCN21R27F Search PubMed.
-
Ecohz, Guarantees of Origin (GOs), ECOHZ, Oslo, NO, 2021, available from, https://www.ecohz.com/renewable-energy-solutions/guarantees-of-origin/ Search PubMed.
-
Entsog, Certification of green gases, European Network of Transmission System Operators for Gas (ENTSOG), Brussels, BE, 2021, available from, https://www.entsog.eu/certification-green-gases Search PubMed.
-
Nasa, New 3D View of Methane Tracks Sources and Movement around the Globe, National Aeronautics and Space Administration (NASA), Washington D.C., USA, 2020, updated 23rd March 2020, available from, https://www.nasa.gov/feature/goddard/2020/new-3d-view-of-methane-tracks-sources-and-movement-around-the-globe Search PubMed.
-
Ghgsat, PULSE GHGSat Montreal, GHGSat, QC, CA, 2020, available from, https://www.ghgsat.com/pulse/ Search PubMed.
Footnote |
† Electronic supplementary information (ESI) available. See DOI: 10.1039/d1ea00046b |
|
This journal is © The Royal Society of Chemistry 2022 |
Click here to see how this site uses Cookies. View our privacy policy here.