DOI:
10.1039/D2AN00283C
(Critical Review)
Analyst, 2022,
147, 2895-2917
Microfluidic flow cytometry for blood-based biomarker analysis
Received
17th February 2022
, Accepted 20th April 2022
First published on 18th May 2022
Abstract
Flow cytometry has proven its capability for rapid and quantitative analysis of individual cells and the separation of targeted biological samples from others. The emerging microfluidics technology makes it possible to develop portable microfluidic diagnostic devices for point-of-care testing (POCT) applications. Microfluidic flow cytometry (MFCM), where flow cytometry and microfluidics are combined to achieve similar or even superior functionalities on microfluidic chips, provides a powerful single-cell characterisation and sorting tool for various biological samples. In recent years, researchers have made great progress in the development of the MFCM including focusing, detecting, and sorting subsystems, and its unique capabilities have been demonstrated in various biological applications. Moreover, liquid biopsy using blood can provide various physiological and pathological information. Thus, biomarkers from blood are regarded as meaningful circulating transporters of signal molecules or particles and have great potential to be used as non (or minimally)-invasive diagnostic tools. In this review, we summarise the recent progress of the key subsystems for MFCM and its achievements in blood-based biomarker analysis. Finally, foresight is offered to highlight the research challenges faced by MFCM in expanding into blood-based POCT applications, potentially yielding commercialisation opportunities.
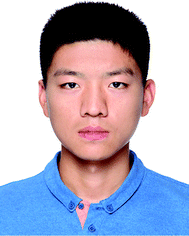 Yuxin Zhang | Yuxin Zhang received his B.Eng (1st class honours) in Mechatronics Engineering from the Beijing Jiaotong University, China and the University of Wollongong, Australia in 2019. He is currently a PhD student at the University of Birmingham, UK. His research interest is microfluidics flow cytometry and modular microfluidics. |
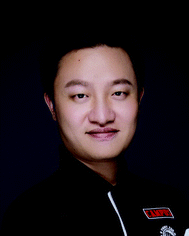 Jinhong Guo | Dr Jinhong Guo received the bachelor's degree in electronic engineering from the University of Electronic Science and Technology of China, Chengdu, China in 2010 and PhD degree in biomedical engineering from the Nanyang Technological University in 2014. Currently, he is a full professor in Shanghai Jiao Tong University, China and executive Dean of Chongqing Medical University, China. After his doctoral studies, he was a postdoctoral fellow in the Pillar of Engineering Design at MIT-SUTD Singapore from 2014 to 2015. He then worked as a Visiting Professor in the School of Mechanical Engineering at University of Michigan, Ann Arbor from January 2016 to July 2016. His current research focuses on Electrochemical Sensor and lab-on-a-chip devices for Point of Care Test toward clinical use. He is a recipient of the China Sichuan Thousand Talents Plan for Scholars Award (2015) and Chengdu Expert in Science and Technology Award (2015). He has published over 100 journal papers. |
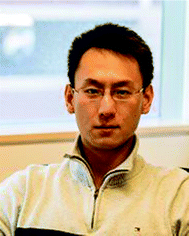 Shi-Yang Tang | Dr Shi-Yang Tang currently is a Lecturer (Assistant Professor) in the Department of Electronic, Electrical and Systems Engineering at the University of Birmingham, UK. He received his BEng (1st class honours) in Electrical Engineering and PhD in Microelectromechanical Systems (MEMS) from the RMIT University, Australia, in 2012 and 2015, respectively. He was the recipient of the Discovery Early Career Researcher Award from the Australian Research Council, and the Vice-Chancellor's Postdoctoral Research Fellow from the University of Wollongong, Australia. Dr Tang's research interests include developing microfluidic platforms for biomedical studies and liquid metal enabled micro-/nanoscale platforms. He has published more than 90 journal papers. |
1 Introduction
Flow cytometry is a powerful single cell analysis instrument which has been widely utilised for disease diagnostics after its innovation in the late 1960s, as a result of its unique characteristics including accurate cell counting, and high-resolution detecting and sorting.1 Most conventional flow cytometry is based on fluorescence or impedance methods to achieve cell detection or sorting by pumping fluid containing samples into narrow flow chambers which are designed for related signal generation and acquisition. When samples pass through the flow chambers, corresponding signals are generated and analysed. Researchers are able to characterise the biological and physical information of samples, for example, micro-organisms, viruses, and individual cells with the assistance of flow cytometry. More importantly, flow cytometry can conduct analysis in a non-destructive and high throughput manner.
As illustrated in the left side of Fig. 1, four essential functional systems are typically assembled in modern flow cytometers: modules for sample focusing, detecting, sorting, and data analysing. Usually, a flow cytometry analysis is performed in the following steps to investigate sample properties individually. First, samples are mixed with buffer to create a solution which is then pumped into a detection chamber. The sample tends to suspend in the solution randomly, therefore, a focusing system is used to narrow the sample solution to a core flow for passing through the detecting module. After acquiring related signals from the detection system, the sorting system is activated to allow for the separation and collection of the target cells. Although flow cytometers have become a basic establishment for the bioassays, normally, they are regarded as complex and costly instruments. To acquire signals in multiple dimensions, an optical-based flow cytometer needs to integrate more than one unit of optical detection setup including bulky laser generators, lenses, filters, and photosensors. Thus, most commercial flow cytometers have a high demand in daily maintenance and operation, which hinders their wide applications in different areas and fields such as diagnostics in low-resource settings and point of care (POC) testing.2
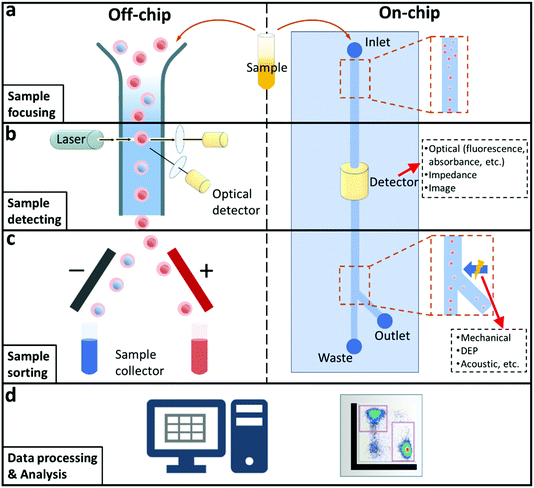 |
| Fig. 1 Schematic illustration of typical off-chip (left side) and on-chip (right side) flow cytometry setup and workflow including (a) Sample focusing. (b) Sample detecting. (c) Sample sorting. (d) Data processing & analysis. | |
The last few decades have seen the emergence and growth of microfluidic technology. Microfluidics is the technology of manipulation of fluid in microchannels with dimensions in the range of tens of micrometres, and it has expanded rapidly into the field of cell biology and many other fields.1,3–5 To overcome the drawbacks of conventional flow cytometers, scientists and engineers applied microfluidic technologies to flow cytometry, resulting in microfluidic flow cytometry. In a microfluidic flow cytometer (MFCM), samples can be confined in micrometre-sized chambers and channels that are compatible with their intrinsic volume, thus significantly reducing the amount of dilution or buffer solution required. MFCM also enables the integration of multiple liquid manipulation processes required in multi-step analytic processes, such as pumping, sampling, dispensing, and sequential loading and washing. The chip format also provides more versatile detecting and sorting methods, for example, multiple detection units can be integrated, and the sorting approach can be varied based on the target sample. These lab-on-a-chip methods have become a potential solution for compact, rapid, and accurate POC diagnostics in underdeveloped settings.3,6–8
A schematic illustration of a microfluidic flow cytometer is shown in Fig. 1 (right side). By applying precise pumping, a sample can be manipulated in the microchannels as desired. The small size of microfluidic chips means a reduced amount of sample and reagent is normally needed for MFCMs. Moreover, the disposable microchip significantly reduces the cost and avoids cross-contamination. In addition, the integrated detection units can be optimised and miniaturised according to the microchip. Therefore, a MFCM usually has a smaller size. With the rapid development of electronics and computer science, data processing has become easier to be miniaturised and integrated into the systems. However, compared to conventional flow cytometers, MFCMs show their own disadvantages. For example, a lower flow rate for bio sample transportation exists in many microfluidic applications. Researchers and engineers are still seeking for solutions to integrate and simplify functional subsystems on microchips.
Biomarkers are commonly found in bodily fluids or tissues and can reflect cellular and molecular variations. As a result, it is instrumental for clinical diagnostic and therapeutic applications that disease-related biomarkers can be detected and quantified.7 Circulating nucleic acids, metabolites, single cells, and viruses existing in living tissues, cells, or bodily fluids constitute all varieties of human biomarkers. Monitoring of conversions, increasing, or decreasing of these biomarkers can be regarded as clinical criteria to prove disease or even forecast disease developments.9–11 Therefore, biomarkers play a significant role in POC diagnostics, medicine monitoring, and therapeutical arrangement.
Blood, as the most important bodily fluid, is capable of indicating a wide range of physiological and pathological properties. Also, various advantages make blood one of the most common choices of liquid biopsy, for example, it is a well-defined material and it is accessible depending on the testing requirements.8 Therefore, blood-based biomarkers are utilised as signal transporters in the circulating system, showing various physiological and pathological conditions and might be applied in non-invasive diagnostic methods. Common biomarkers in blood and diseases that can be diagnosed based on these biomarkers are listed in Table 1.7,12,13
Table 1 List of biomarkers in blood
Biomarker category |
Sub-categories |
Diseases |
Ref. |
Circulating nucleic acids |
• Cell free DNA (cfDNA) |
• Cancer |
14–16
|
• RNA |
• Viral infection |
|
• Hepatitis |
|
• AIDS |
|
• Bacterial infection |
|
• Influenza |
Metabolites |
• Prostate-specific antigen |
• Prostate cancer |
17–19
|
• Matrix metalloproteinase |
• Endometriosis, breast cancer |
• β-Galactosidase |
• Bacterial infection |
• Cellobiohydrolase |
• Bacterial infection |
• Glucose oxidase |
• Diabetes |
• Catalase |
• Infection, inflammation, cancer |
• C-reactive protein |
|
Cells |
• Circular tumour cell (CTC) |
• Cancer |
20–23
|
• Bacteria |
• Bacterial infection |
• Fungi |
• Nosocomial infection |
Viruses |
• Hepatitis viruses |
• Viral infection |
24 and 25 |
• Human immunodeficiency viruses (HIV) |
• Dengue |
• Influenza viruses |
|
In this review, we seek to examine and summarise the recent major developments of MFCMs for blood-based biomarker analysis. We will outline the key enabling technologies (including sample focusing, detecting, and sorting) and recent advances, as well as discuss challenges and future trends as research progresses towards commercial platforms.
2 Focusing systems for microfluidic flow cytometry
In a typical MFCM, to avoid the simultaneous presence of multiple samples in the detection area, proper strategies need to be applied to narrow down the sample suspension stream. By properly focusing the samples, they can be reordered along the central line without contacting each other and the flow cell wall. Eliminating the instability of the sample velocity via proper alignment strategies also ensures the accuracy of acquired signals and the accuracy of the sample sorting process downstream. Therefore, an effective microfluidic focusing system plays a crucial role in a high-performance MFCM. Here, we briefly summarise common strategies adopted for sample focusing, more detailed reviews on this topic can be found elsewhere.26
2.1 Hydrodynamic focusing system
Hydrodynamic based focusing systems are the most common technique to narrow sample flow and have been widely used in conventional flow cytometry applications. The emerging MFCMs also take advantage of this technique. By verifying the microchannel geometries, the focusing performance can be significantly improved and the overall size can be further reduced. Additionally, the simple and straightforward working mechanism makes it possible to easily modify the associated microchannel geometries and achieve mass production at a low cost using standard manufacturing processes such as thermal plastic injection moulding and soft lithography. However, for a planar hydrodynamic focusing technique, the sample core flow can only be limited in the horizontal plane, which hinders its potential to be used in applications with high precision requirements such as image-based detection.
As shown in Fig. 2a, 2D focusing is defined as the horizontal narrowing of samples to the centre plane of a channel, with samples still spread over the microchannel depth. The recent advances and applications of 2D focusing have been comprehensively reviewed in recent publications.27,28 This focusing method is mainly capable of particle sorting applications despite the fact that particle adsorption to the above and below channel walls might be a problem at times. Recent advances such as image-based flow cytometry require high detection sensitivity, and 3D focusing-horizontal and vertical sample focusing, is necessary.26 The 2D hydrodynamic focusing is the most commonly used method in MFCM due to its simple fabrication process, simple control requirement, and high throughput. However, the lack of control of particle position in the vertical direction limits the stability of signals.
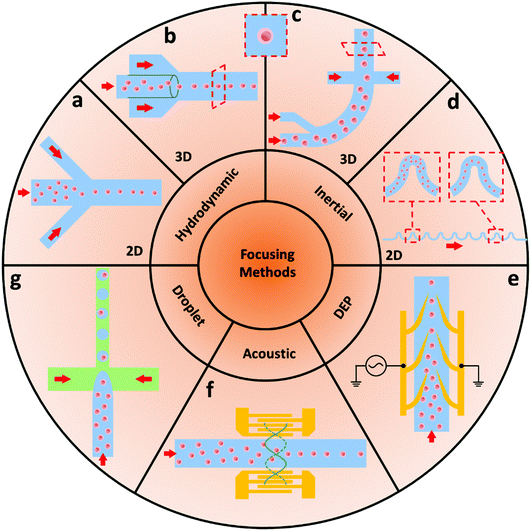 |
| Fig. 2 Schematic of sample focusing methods used in MFCMs. Typical methods are based on (a and b) hydrodynamic, (c and d) inertial, (e) DEP, (f) acoustic, and (g) droplet-based techniques. | |
As shown in Fig. 2b, 3D microfluidic focusing methods limit the core flow both horizontally and vertically, and, therefore, provide more accurate and reliable signals. However, because of the planar fabrication process of microchips, realising on-chip 3D focusing demands more effort than 2D focusing. Normally, multiple-layer microchip designs were used to accomplish 3D hydrodynamic focusing, in which the sample stream is constrained to the centre by sheath flows.26 Alternatively, as an example, a 3D focusing MFCM using hydrodynamic forces by applying a micro-weir structure design at the bottom of the channel was developed by Fu et al.29 The suggested platform effectively separated microbeads with different diameters (5 μm and 10 μm) in the vertical direction. It was also demonstrated that separating the samples caused their sequential passage through the device's interrogation chamber, which increased the detection process’ performance. The same group later employed the Saffman shear lifting force created by a sequence of micro-weirs of gradually decreasing height to focus the sample stream along the centre-line of the microchannel in a 3D focusing sheathless MFCM.30 The newer design achieved a focusing performance of above 99.5% for microbeads with diameters of 5 and 10 μm. The 3D hydrodynamic focusing method largely improves sensitivity level and holds its advantage of high throughput. However, it requires more complex fabrication process and complicated fluid pumping systems.
2.2 Inertial focusing systems
Cross-stream particle motion is used in inertial focusing methods to concentrate particles into one or multiple streams (Fig. 2d). Different channel designs have been investigated for affecting the inertial focusing results, including straight channels,31–33 curved channels,34–36 and type-specific (e.g., groove-structure) channels.37–41 In a channel flow, samples are affected by both shear gradient and wall effect forces, and sample focusing happens whenever these combined forces are balanced. Particles, cells, viruses, and other items have been counted and sorted using inertial focusing microfluidic cytometers.42 Oakey et al.,43 for example, built a staged inertial-based microfluidic system with both straight and curved channel designs to restrict particles into a core flow without the use of a sheath flow. The suggested channel's accuracy was found to be comparable to commercial cytometers with hydrodynamic focusing systems. Furthermore, the device's performance was shown to enhance as the flow rate increased, indicating that it has the capability to be used in high-throughput analytical settings. Tang et al.44 demonstrated an impedance-based MFCM with liquid electrodes and an inertial focusing module. The practicality of the suggested cytometer was proved by examining the size distributions of white blood cells (WBCs) and breast tumour cells (MCF-7) in human whole blood samples. Unlike the hydrodynamic focusing, well-designed microchannel structures ensure its advantages in simple fluidic (due to sheathless) control and throughput. However, the specific geometry and requirement on precise flow rate limit its popularisation in MFCMs.
2.3 Dielectrophoresis based focusing systems
Dielectrophoresis (DEP) is a commonly used method for manipulating cells, particles, DNAs, viruses, and many other items in microfluidic systems.45–47 DEP is also widely employed in a range of bio-sensing devices for trapping, patterning, focusing, separating, transporting, and depositing. The DEP force is affected by particle diameter, dielectric characteristics of the particles, and the fluid through which they are conveyed. Furthermore, the DEP force changes as a function of frequency in an alternating current (AC) field. The DEP response may be adjusted from negative (particles are rejected from high-intensity field zones) to positive (particles are drawn to high-intensity field zones) based on the dielectric characteristics of the fluid and particle. The crossover frequency is the frequency where this change happens. Negative DEP forces were typically used in electrode-based DEP 2D focusing techniques.48 To achieve 3D focusing, combining with other focusing strategies is necessary. For example, Lin et al.49 designed a 3D focusing platform combining hydrodynamic forces and a vertically applied negative DEP force. Polystyrene microbeads (diameters of 10 and 20 μm) and diluted human RBCs were used to illustrate the practicality of the proposed technology. The DEP force increased the consistency of the acquired signal amplitude, which improved the accuracy and consistency of the detection findings. The DEP focusing provides a high level in sensitivity but at the same time sacrifices the throughput. Also, the additional microfabrication process of microelectrodes and the frequency dependent complex control constrain its utilisation in MFCM.
2.4 Acoustic focusing systems
Large forces may be generated on samples in a microfluidic device using acoustic fields. The samples can migrate toward either the pressure nodes or the antinodes as a result of acoustic manipulation, depending on the particle density and deformability, as well as the environment in which they are dispersed, as illustrated in Fig. 2f.50 Moreover, the particles can be driven to migrate within a microfluidic channel by activating a sequence of interdigital transducers (IDTs) on a piezoelectric material at their resonance frequencies, resulting in a sample movement effect.51–53
Numerous approaches for microfluidic manipulation utilising either travelling surface acoustic waves (TSAWs)54,55 or standing surface acoustic waves (SSAWs)56,57 have been reported in various studies. Surface acoustic wave (SAW)-based systems offer a large working frequency range (1 MHz–1 GHz, corresponding to acoustic wavelengths of 4–4000 μm on a piezoelectric lithium niobate substrate) with high biocompatibility for microspheres handling.58 Recent research has revealed that acoustic fields can not only be utilised to transport samples at the microscopic level, but they can also be used to focus samples efficiently. For example, Collins and Ai et al.58–61 applied a group of IDTs to generate narrow acoustic beams with a width of 10–20 μm. Also, a series of hybrid focusing methods combing hydrodynamics and acoustic were developed by Huang's group to enhance the focusing performance and reduce the fabrication process.62–64
Acoustic based focusing systems have been successfully applied on MFCM for a wide range of bio samples. Chen et al.65 developed an SSAW-based MFCM wherein the samples were focused by a 3D focusing field formed by twin IDTs and detected using a laser-based fluorescence approach in the downstream detection chamber. At a rate of ∼1000 particles per s, the suggested system has a coefficient of variation of less than 10%, according to the result acquired using calibrating beads. With a planar electrode Coulter-type impedance spectrometer, Grenvall et al.66 developed an acoustic focusing microchip enabling sheathless focusing of cells and particles, as well as subsequent separating and counting. The system was applied to analyse single and mixed size particle suspensions, and also diluted whole blood samples. The findings were found to be quite comparable to those found using a standard standalone Coulter counter. The acoustic focusing system can maintain high throughput and sensitivity, but the requirement of specific piezoelectric transducers may introduce more cost and complexity.
2.5 Droplet-based focusing systems
In the past decade, droplet-based microfluidics has emerged as a powerful tool for the encapsulation and manipulation of individual cells in a high-throughput manner within pico-litre microdroplets, which has revolutionised the study of single cell analysis.4,67,68 For encapsulating single cells, a cell suspension is dispensed into a single droplet in the oil phase (Fig. 2g). Then the generated microdroplets are reinjected into a customised detection chamber of the MFCM. In the following detection system, instead of detecting labelled individual cells, cell-laden microdroplets are targeted. In this case, the focusing step is simplified since the aimed size is enlarged from individual cells to cell-laden microdroplets, where droplets are narrowed within the interrogation flow channel and driven through the detection unit in order. Then the data is acquired, and the desired sample can be sorted along with its droplet compartment in the sorting unit downstream. Instead of acting on individual cells, the droplet-based focusing method provides an alternative approach, which allows the detecting and sorting system to directly target based on the content of the individual droplets. Baret et al. built a fluorescence-activated droplet sorting (FADS) system that was designed to sort picolitre-sized microdroplets by DEP. In this work, no focusing model is involved and the aqueous droplets flow through the fluorescence-based detecting system in sequence which finally achieved an accurate sorting result. Water-in-oil microdroplets were pumped into a microchip sorter in which samples were spaced out and separated at a Y-shaped structure. Similarly, to identify the cells with high α-amylase expression, Sjostrom et al.69 employed a FADS platform to screen a yeast library with mutations randomly inserted across the genome by UV-irradiation mutagenesis. Over the process of about 2 h, nearly 3 × 106 microdroplets were sorted at a frequency of 323 samples per second. With the aid of the droplet technique, no conventional focusing module is required. Unlike the conventional on-chip process, Cole et al.70 developed printed droplet microfluidics technology, where individual cells and reagents were encapsulated in microdroplets and then a fluorescence-based droplet sorter actively selected and deposited desired samples on an arrayed format substrate. As a new focusing concept to treat droplets as the target, the aimed size is enlarged from individual sample to sample-laden microdroplets, which allows high sensitivity and throughput. However, the droplet size needs to be precisely controlled actively or passively.
3 Detecting systems for microfluidic flow cytometry
The detection capability of MFCMs is dependent on samples being precisely streamwise positioned such that they are able to pass the interrogation chamber in a single line. Optical scattering-based approaches have typically been used to identify and analyse focused samples71–80 (Fig. 3a). However, imaging-based detection81–90 (Fig. 3b) and impedance-based detection91–98 (Fig. 3c) have become progressively universal in the past decade. We will briefly summarise these techniques in the following sections, full details of them have been reviewed elsewhere.95,99–101
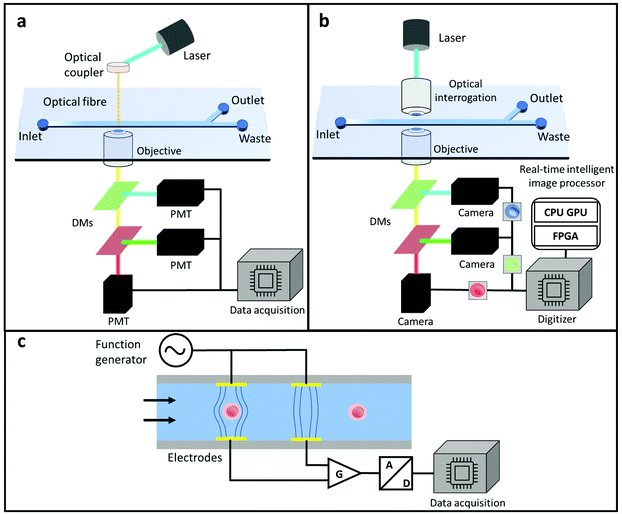 |
| Fig. 3 Schematic of sample detecting system setups in microfluidic flow cytometry. Typical methods are based on (a) optic-based detection, (b) image-based detection, and (c) impedance-based detection. | |
3.1 Optical-based detecting systems
Forward scatter (FSC) and side scatter (SSC) signals, as well as at least a few fluorescence colours signals, are always acquired by traditional flow cytometers.102,103 The FSC and SSC data reveal sample size and internal granularity, whereas the fluorescent data allows immunophenotyping to be used to distinguish between distinct sample types. To realise the miniaturisation of this optical system in MFCMs, recent efforts were made from different perspectives. For example, Fig. 3a shows a typical optical-based detecting module on MFCM. The exciting laser beams were shaped and focused on the sample flow area, and the integrated optical components downstream collected the FSC and SSC information which were detected by photomultiplier tubes (PMTs) with appropriate band pass filters.
3.2 Image-based detecting systems
Image-based analysis detection approaches for MFCMs integrate the high-resolution cell imaging abilities of microscopes with the high-throughput capability of conventional MFCMs. Optical imaging is, without a doubt, the most effective method for visualising live cells with great spatiotemporal resolution. As a result, optical imaging techniques are progressively being used in state-of-the-art cellular assay procedures for the categorization of distinct cell types/stages and dissection of their individual cellular activities. Introducing imaging capabilities to MFCMs, however, limits the throughput to ∼1000 cells per second, which is significantly less than 100
000 cells per second for gold-standard flow cytometers. Normally, the detecting sensors applied in image-based MFCMs can be divided into two types: (1) camera-based imaging sensors such as charge coupled devices (CCD) and complementary metal–oxide-semiconductor (CMOS) devices;81,99,104–106 and (2) photodetector-based imaging devices.107–110 As shown in Fig. 3b, in imaging-based MFCM systems, utilising camera-based devices and a wide-field illumination approach, 2D bio sample imaging could be easily achieved provided that enough photons are detected during a set exposure time. In this case, the dilemma is that of enhancing the image processing speed.
Machine learning (ML), as a winner in processing various image information in recent years, is increasingly bound to microfluidics in the form of intelligent microfluidics thus making detection more automated and intelligent.83,111,112 Image-based detecting MFCM systems, due to their applicability to ML, especially the presently hottest network-based ML, are gaining more attention from researchers. For example, Heo et al.81 built a convolutional neural network (CNN) image recogniser supported image-based detecting system that analyses images captured from CMOS camera for label-free detection of blood cells. Since this system utilised a multi-target tracking algorithm, all cells in a single image can be analysed simultaneously. Therefore, it offered the possibility to improve throughput and achieve real-time analysis. In addition, Isozaki et al.85 achieved high precision and sensitive detection of cells by acquiring virtual-freezing fluorescence images with a CMOS camera, aided by a 6-layers CNN.
3.3 Impedance-based detection systems
Impedance-based MFCM measures the impedance of a single cell in a detection region and therefore directly reflect the cell's dielectric character.113 As shown in Fig. 3c, representatively, this setup features microchips where each sample passing by an integrated detection area is examined individually in an electric field. The dielectric characteristics of the cell within will determine the impedance spectrum of the control volume. The cell membrane provides a significant barrier to current flow at low frequencies, and the impedance amplitude predicts the size of a cell. Membrane polarisation is diminished at intermediate frequencies, and impedance analyses provide information regarding membrane characteristics. The membranes are minimally polarised at high frequencies; therefore, examinations here provide information on intracellular architecture and the cell interior. As a result, analysing the dielectric spectrum in the frequency range from 40 Hz to 1 GHz offers frequency-dependent information on membrane capacitance, cytoplasm conductivity, and cytoplasm permittivity. There are different kinds of electrode configuration designs for impedance-based MFCM, including coplanar electrodes,114,115 parallel electrodes,116 and constriction channels.117 Regardless of the design, the detection principle is similar, where excitations are generated by an electrode and signals are collected by multiple sensing electrodes.
4 Sorting systems for microfluidic flow cytometry
In contemporary flow cytometry, sample sorting is a critical step. The sample sorting in a traditional flow cytometer is accomplished by diverting the charged sample-contained droplets within an electric field. Various on-chip sample sorting methods have been employed in MFCM and they can be generally split into active and passive approaches.118–120 Active sorting relies on external forces (enabled by electric, magnetic, optical, acoustic, or piezoelectric actuation), while passive separation utilises the internal dynamics (such as specifically designed geometric microstructures in microchannels).121,122 In this section, we summarise the main sorting approaches and their characteristics in MFCM. Detailed reviews of the sorting methods in MFCM can be found elsewhere.68,123,124
4.1 Mechanical sorting systems
Mechanical sorting systems usually rely on external actuators connected to the microfluidic chip. Utilising the piezoelectric effect finds a balance between cost and performance when compared to other actuation approaches. In applications, it offers the benefits of flexibility, disposability, and competitive prices. The piezoelectric lead-zirconate-titanate (PZT) actuator has a very short reaction time and may bend according to the polarity of the electric potential. When a PZT actuator is placed onto a microchip and connected to on-chip dual membrane pumps, it can sort cells using a pull-push relay method to generate lateral flows, as shown in Fig. 4a. The volume and response speed of the local flow is controlled by varying the input signal applied to the PZT actuators.125,128 The mechanical sorting system can separate both large and small samples, and the viability of cells can be maintained at a high level. However, the throughput and sorting efficiency are influenced by the upstream focusing and detecting systems.
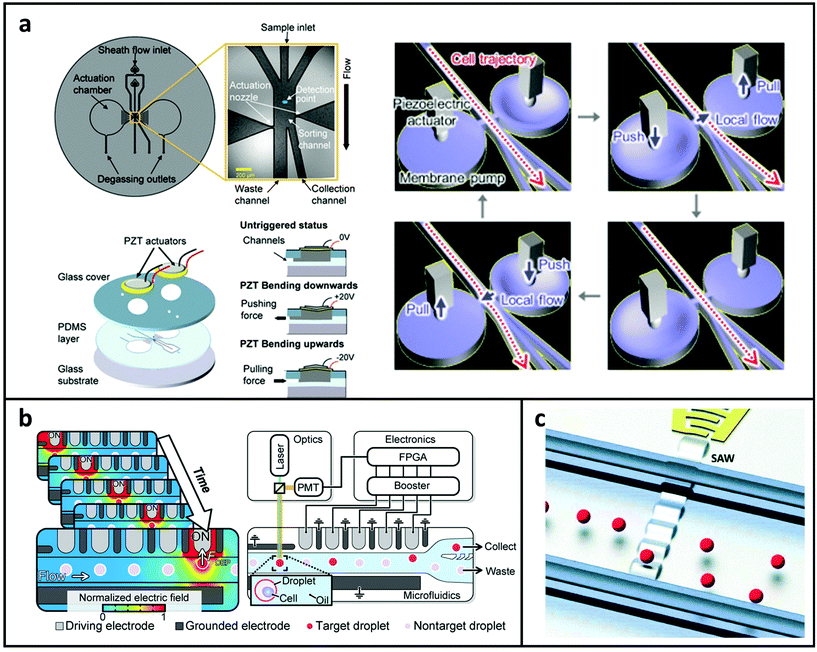 |
| Fig. 4 (a) Illustration of the FACS microchannel based on the mechanical actuation mechanism for sample sorting. (Left) Reproduced from ref. 125 with permission from Springer Nature, copyright 2017. (Right) Reproduced from ref. 86 with permission from Elsevier, copyright 2018. (b) Illustration of the microchannel based on a DEP array for sample sorting. Reproduced from ref. 126 with permission from American Association for the Advancement of Science, copyright 2020. (c) Illustration of the microchannel based on the high-frequency TSAW for sample sorting. Reproduced from ref. 127 with permission from Royal Society of Chemistry, copyright 2016. | |
4.2 DEP based sorting systems
As introduced in the focusing section, DEP can also be utilised to separate particles by generating polarisation forces in a non-uniform electric field.129 A bifurcation configuration is commonly used for sorting targeted samples, such as cell-laden droplets.69,130–132 In this method, in the absence of the electric field, samples flow into the branch with lower hydrodynamic resistance; during the sorting process, the electrode near the bifurcation is energised to steer the required samples into the collecting channel. Recently, this method was further improved with sequentially addressable dielectrophoretic array (SADA).126 As shown in Fig. 4b, the SADA sorter delivers an accumulated DEP force to the target droplet using an array of electrodes that can be sequentially activated and deactivated to synchronise to the droplet's speed and position. The droplet can then be gently pulled in the direction of sorting in a high-speed flow. The SADA sorter can sort microdroplets with a large size, which is challenging to achieve using only a single pair of electrodes as the intensive nonuniform electric field required for sorting can fracture the droplet. The DEP based sorting method is more flexible and programmable. However precise fabrication and control requirements limit its application and integration to MFCM.
4.3 Acoustic sorting systems
The acoustophoretic technique for sample sorting is an appealing and biocompatible option. The viability of cells can be maintained for minutes to hours under a low-intensity acoustic field. Furthermore, it's simple to be incorporated into a microchip-based platform. Acoustic waves are generated mainly by piezoelectric actuators,133 and TSAWs and SSAWs are two main forms of SAWs to achieve the sorting. TSAWs are formed utilising a single pair of interdigital transducers, whereas SSAWs are generated by the interference of two TSAWs.134 As shown in Fig. 4c,127 individual particle displacement were achieved by focused SAW enabled acoustic fields. With the microseconds level pulses, 2 μm and larger samples are able to be moved as required and sorting speed can vary from 1 to 10 kHz. The acoustic system is able to increase the sorting throughput because of its short response time and the large driving force makes it capable of sorting samples with various sizes. However, the complex control instruments may lead to bulky systems.
In summary, we briefly introduced various commonly used techniques adopted by MFCM. Based on the physical and biochemical properties exhibited on different blood-based biomarkers, the choice of specific focusing, detecting, and sorting techniques is summarised in Table 2.
Table 2 Guidance of focusing, detecting, and sorting techniques for blood-based biomarkers
Biomarkers |
Properties |
Focusing |
Detecting |
Sorting |
Circulating nucleic acids |
Small size; amplification is required in most applications |
Droplets |
Optical |
Mechanical |
DEP |
Metabolites |
Various formats; complex sample preparation process |
Droplets |
Optical |
Mechanical |
DEP |
Cells |
Relatively large size; various labelling methods |
Hydrodynamic |
Optical |
Mechanical |
Acoustic |
Image |
Acoustic |
DEP |
Impedance |
|
Inertial |
|
|
Viruses |
Small size; antibody conjugation is required in most applications |
Hydrodynamic (on beads) |
Optical |
Mechanical |
Droplets |
|
Acoustic |
5 Microfluidic flow cytometry for blood-based biomarker analysis
Based on the abovementioned key techniques for focusing, detecting, and sorting biological particles, significant developments of MFCMs for analysing biomarkers in blood, including cells, viruses, circulating nucleic acids, and metabolites have been made in recent years, as summarised in Table 3.
Table 3 Summary of MFCMs applied for blood-based biomarker detection and screening
Biomarker category |
Sub-categories |
Disease |
Separation |
Detection method |
Throughput |
Limit of detection |
Remarks |
Ref. |
Cells |
Tumour cells |
Cancer |
Deterministic lateral displacement (DLD) |
Optical based (fluorescent microscope) |
5 cells per min |
104 cells per mL |
Enzyme assay |
135 and 136 |
Circular tumour cells (CTC) |
Cancer |
N/A |
Optical and photoacoustic based (PMT and ultrasound transducer) |
15 cells per h |
1 cell per 40 mL |
Mouse in vivo analysis |
137 and 138 |
|
Cancer |
Mechanical actuation |
Optical based (PMT) |
3 × 105 cells per min |
10 CTC per mL |
Labelled mouse tumour cells |
139 and 140 |
|
Breast cancer and Nonsmile cell lung cancer |
Inertial |
Optical based (PMT) |
1.2 × 103 particles per h |
1 × 103 particles per mL |
Human tumour samples |
141
|
|
Pancreatic ductal adenocarcinoma |
N/A |
Impedance based (impedance spectroscope) |
∼350 cells per s |
∼2 × 105 cells per mL |
Human tumour samples |
142
|
|
Breast cancer and melanoma |
N/A |
Impedance based (impedance analyser) |
N/A |
N/A |
Human tissue samples |
143–145
|
Bacteria |
Bacterial infection |
N/A |
Optical based (fluorescent microscope) |
N/A |
100 CFU per mL |
E. coli, E. aerogenes, S. aureus
|
23 and 146–148 |
Fungi |
Nosocomial infection |
N/A |
Optical based (digital PCR) |
N/A |
105 CFU per mL |
Candida albicans
|
149
|
Viruses |
Virus particle |
Viral infection |
N/A |
Optical based (PMT) |
1.5 × 109 virus per h |
3 × 107 virus per ml |
Baculovirus |
150
|
|
Viral infection |
N/A |
Impedance based (impedance spectroscope) |
10 mL per min |
Down to 3 ng mL−1 (antigen concentration) |
|
151
|
|
Dengue |
N/A |
Optical based (PMT) |
N/A |
103 PFU per mL |
Dengue virus serotype 2 |
17
|
Circulating nucleic acids |
Cell-free DNA (cfDNA) |
Cancer |
N/A |
Optical based (digital PCR) |
N/A |
20 ng per μL |
PCR |
152–161
|
|
Breast cancer |
N/A |
Optical based (digital PCR) |
N/A |
N/A |
PCR |
162–165
|
|
Colorectal cancer, lung cancer |
N/A |
Optical based (digital PCR) |
N/A |
25 ng per μL |
PCR |
166–169
|
|
Viral infection |
N/A |
Optical based (digital PCR) |
N/A |
10 ng per μL |
PCR |
170–172
|
|
Hepatitis B |
N/A |
Optical based (digital PCR) |
N/A |
N/A |
PCR |
173
|
|
AIDS |
N/A |
Optical based (digital PCR) |
N/A |
20 ng per μL |
PCR |
161 and 174 |
|
Bacterial infection |
N/A |
Optical based (digital PCR) |
N/A |
100 CFU per swab |
PCR |
175 and 176 |
RNA |
Viral infection |
N/A |
Optical based (fluorescent microscope) |
N/A |
N/A |
PCR |
177
|
|
Hepatitis C |
N/A |
Optical based (digital PCR) |
N/A |
0.005% of mutant in the presence of a 20 000-fold excess of the wild-type DNA. |
PCR |
178
|
|
AIDS |
N/A |
Optical based (digital PCR) |
N/A |
N/A |
RT-PCR |
179
|
|
Bacterial infection |
N/A |
Optical based (avalanche photodiode) |
N/A |
10 aM |
Enzyme assay |
180
|
|
Influenza |
N/A |
Optical based (fluorescent microscope) |
N/A |
10 copies per μL |
Loop-mediated isothermal amplification (LAMP) |
181
|
Metabolites |
Prostate-specific antigen |
Prostate cancer |
N/A |
Optical based (fluorescent microscope) |
2 × 105 droplets per chip |
∼100 pg per mL |
Bead-based ELISA |
182
|
Surface protein |
HIV |
N/A |
Optical based (fluorescent microscope) |
200 cell-containing droplets per s |
110 fM |
Enzyme assay |
183
|
Matrix metalloproteinase |
Endometriosis, breast cancer |
N/A |
Optical based (fluorescent detection setup) |
N/A |
5 ng per μL |
Enzyme assay |
184–188
|
β-Galactosidase |
Bacterial infection |
N/A |
Optical based (fluorescent microscope) |
∼960 cells per min |
6.4 × 105 cell per mL |
Enzyme assay |
182, 189 and 190 |
Cellobiohydrolase |
Bacterial infection |
N/A |
Optical based (PMT) |
100 μL per h |
N/A |
Enzyme assay |
191
|
Glucose oxidase |
Diabetes |
N/A |
Electrochemical based (electrochemical workstation) |
3.6 μL per min |
0.05 mM |
Enzyme assay |
192
|
β-Glucosidase, catalase, C-reactive protein |
Infection, inflammation, cancer |
N/A |
Optical (PMT)/electrochemical (electrochemical workstation) based |
500 droplets per s |
0.01 μg per mL |
Enzyme assay, antibody conjugation |
193–195
|
5.1 MFCM for detecting cell-based biomarkers
The introduction of circulating tumour cells (CTC) as a minimally invasive multifunctional biomarker has become one of the most exciting discoveries in modern cancer treatment. CTCs in the peripheral blood come from solid tumours and are implicated in hematogenous metastatic dissemination to distant areas, allowing secondary foci of illness to form. In one millilitre of blood containing ∼1 × 109 blood cells, most individuals with metastatic cancer have less than 10 CTCs. The purpose of CTC technology is to separate and recover individual CTCs or clusters of CTCs in large quantities of whole blood (>5 mL) with excellent purity and low shear stress to avoid cellular damage. Serial evaluations for pharmacodynamic (PD), prognostic, predictive, and intermediate endpoint biomarker research may be performed at numerous life stages during a patient's cancer journey thanks to the development of contemporary CTC technology.20–22
CTCs, like blood cells, carry epithelial surface markers, the most common of which are epithelial cell adhesion molecules (EpCAM). Based on this, the ‘CTC-chip’, the first microfluidic technique to collect CTCs from whole blood, utilised microposts coated with EpCAM antibodies.140 Although other surface epitopes can be used, such as human epidermal grown factor receptor 2 (HER2) or epidermal growth factor receptor (EGFR), the production of cancer surface markers is very variable, even from the same patient.196 Because CTCs have such a wide range of expression, microfluidic methods based on an immunoaffinity technique may overlook a significant percentage of CTC cells. Rather than antibodies, aptamer panels can be employed, although these might lack selectivity in detecting CTCs because they were derived from cancer cell lines.197,198
CTCs sorting can be achieved according to various differences like volume, density, compressibility, deformability, or electrical impedance to address the limits of positive or affinity-based selection.199,200 Volume variances have been used to separate individual samples from blood by applying acoustic radiation forces,199 a mixture of inertial flow and Dean vortex flow,201 or micro vortices.202 Recently, with lateral deterministic displacement technology, clusters containing from 2 to 100 cells can be isolated from whole blood.203,204 While some tumour cells have a larger size compared to leukocytes, a large percentage of CTCs are comparable in size to leukocytes. These approaches, like positive selection, can overlook a significant percentage of CTCs that are roughly comparable to leukocytes.
Hamza et al.139 created an optofluidic real-time cell sorting system that can harvest fluorescently labelled CTCs from a genetically manipulated mouse in a continuous fashion (Fig. 5a). On the back of a mouse, an arteriovenous shunt was inserted, permitting continuous blood extraction from the left carotid artery and return via the right jugular vein. A microchip with one input and two outputs was used to link the circulation. The stream channel was lit by two closely separated laser beamlines for recognising fluorescently expressed CTC and determining cell velocity. The circulation was controlled by two valves downstream of the microchannel, which sent it either back to the mouse body or the CTC collecting tube. This approach allows CTCs sorting with small amounts of blood. Fig. 5b illustrates the tumour cells detecting method enabled by the two excitation laser beams. More recently, as shown in Fig. 5c and d, McGrath et al.142 published a thorough research aimed at phenotyping pancreatic tumour xenografts based on tumorigenicity. Individual cells from primary pancreatic tumours and those from liver metastasis were compared utilising a high-throughput system (>300 cells per sec) and an impedance-based phase-contrast criteria, which relies on impedance phase variations in the low and high frequency ranges. Differences in this measure were discovered to be linked to cell interior electrophysiology and to change consistently as a function of tumorigenicity. Furthermore, DEP was conducted simultaneously, confirming that cancer cells with greater tumorigenicity had lower internal conductivity and higher permittivity. The phenotypic characterisation was further validated by genetic investigation, which revealed that dysregulation of Na+ transportation and Ca2+ removal existed in more tumorigenic cell types.
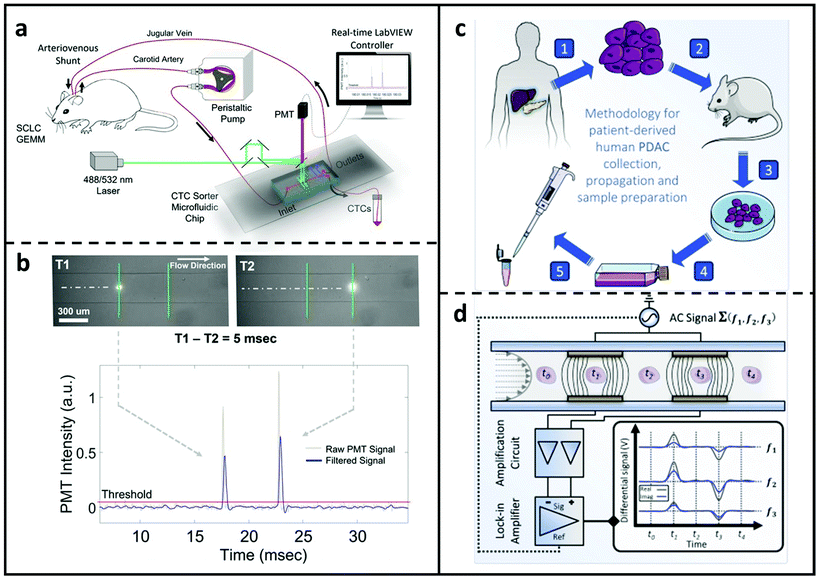 |
| Fig. 5 MFCMs for detecting cancer cells. (a) Microfluidic sorter for CTC studies in genetically engineered mouse models. Peristaltic pump extracts body fluids from a mouse and the blood were pumped into the sorter microchip. A fluorescence-based sorting system was used to collect rare CTC cells, and the processed blood redirected to the vein. (b) Schematic of the CTC detecting module containing two excitation laser beams. Reproduced from ref. 139 with permission from National Academy of Science, copyright 2019. (c) Workflow from Pancreatic ductal adenocarcinoma (PDAC) patient to microchip via the xenograft (PDX) model. (1) Tumour cells collected from patients with PDAC and (2) implanted and propagated in immunocompromised mice as a PDX. (3) After that, PDAC samples were created from surgically removed PDX and (4) cultured in medium at 37 °C. (5) Aspirated PDAC cells were then cleaned and restored in solution used for impedance cytometry. (d) Illustration of the impedance-based detecting process. PDAC tumour cells suspension was driven through the detection channel which includes two pairs of facing microelectrodes. Voltages at three discrete frequencies were applied to the top electrodes and the differential current were amplified and acquired at the bottom electrodes. Reproduced from ref. 142 with permission from Elsevier, copyright 2020. | |
The electrical properties of CTCs were tested with impedance spectroscopy and electrorotation.143–145 As an example, the impedance of four breast cancer cell lines, MCF-7, MCF-10A, MDA-MB-231, and MDA-MB-435S, was examined by Qiao et al.145 These cell lines range from healthy cells to cancer cells in late stages. They used a microfluidic chip with electrodes attached to an impedance analyser to analyse the cell suspensions. The impedance of cell suspensions was used to obtain the electrical characteristics of single cells, including whole cell conductivity, membrane capacitance, cytoplasm conductivity, and relaxation frequency. Researchers discovered that each cell type has unique electrical characteristics that gives it a feature that may be utilised to differentiate cells and determine the stage of cancerous cells.
In addition to the breast cancer cells, the construction of an interdigitated microelectrode array (IDMEA) biosensor using electrochemical impedance spectroscopy and cyclic voltammetry to examine the B16 melanoma cell line was reported by Avram A. et al.144 They measured the adherence and spread of B16 melanoma cells on IDMEA and developed an impedance spectrum for them. Likewise, Avram M. et al.205 proposed a label-free melanoma cell detection approach depending on the melanoma cells’ dielectric characteristics and the gold nanoparticles (AuNPs) within. C57BL/6 mice were injected with 1 × 106 murine melanoma B16 cells dispersed in 0.1 mL PBS. The mice received 200 μL of 200 nM AuNPs suspended in PBS intravenously. The investigators discovered a significant difference in the scattering spectra of AuNPs in melanoma cells and AuNPs in normal tissue during histopathological investigation of the tumour tissue. The B16 melanoma cells containing AuNPs in cytoplasm showed a distinct blue colour under ultraviolet fluorescence, which was associated with the localised surface plasmon resonance (LSPR) principle. This technology might be used to diagnose patients without the usage of labels.
Most MFCMs for single cell-derived biomarker detection rely on immortalised cell lines in controlled buffers or reference bacterial strains in controlled buffers/broth. For a few platforms that process clinical biofluids, each requires varying degrees of off-chip or on-chip sample preparation. Therefore, integrating sample process modules into the platform might be a feasible way to minimise and automate MFCM systems. However, the downsides of such an approach are added assay complexity and difficulty in platform design, fabrication, and control. As such, both the advantages and the disadvantages will have to be carefully evaluated for obtaining optimal platform performance.
5.2 MFCM for detecting bacteria, fungi, and microorganisms
Rapid and reliable identification of low abundance pathogens in biological samples is critical for disease diagnosis and subsequent therapeutic arrangements in infectious diseases. As shown in Fig. 6a, Kaushik et al.23 introduced a fast and integrated method. The researchers used 20 pL microdroplets to encapsulate a single bacterium. Because of the lower background in the smaller droplets, the fluorescence signal formed by bacterial growth could be determined more rapidly. The effect of gentamicin on the development of a susceptible and multidrug resistant strain of E. coli was found after just ∼1 hour, which is comparable to two to three bacterial doubling occurrences. Instead of using droplets, Rho et al.151 demonstrated the utilisation of microbeads, as shown in Fig. 6b, in which an MFCM was used for complex immunoassay using gold microspheres tethered to a filamentous virus. This technique enabled a high number of antibodies to be attached to the microspheres while also reducing non-specific adsorption. The researchers used MFCM with fluorescence and DC-impedance to detect four distinct biomarkers at the same time: cardiac troponin I (cTnI), prostate specific antigen (PSA), creatine kinase MB (CK-MB), and myoglobin. The detection limit of virus-tethered beads was higher than that of virus-free beads.
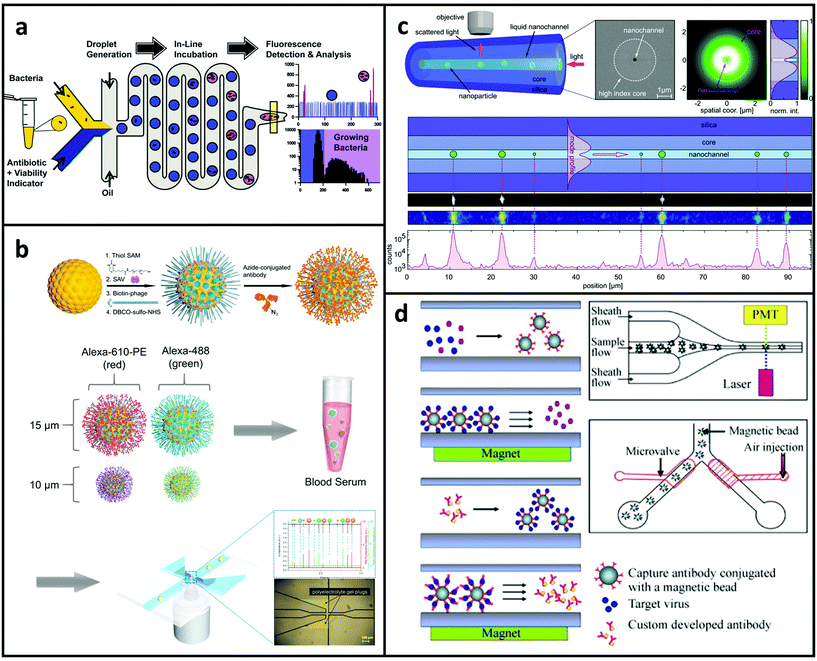 |
| Fig. 6 MFCM analysis for detecting pathogen biomarkers. (a) Illustration of one-step platform for bacterial growth testing and antibiotic susceptibility examination. Reproduced from ref. 23 with permission from Elsevier, copyright 2017. (b) Illustration of the surface modification workflow for bio-inspired filamentous virus tethered on gold-layered beads. Reproduced from ref. 151 with permission from Elsevier, copyright 2018. (c) Detection of dielectric nanoparticles in a nanofluidic fibre. Reproduced from ref. 206 with permission from American Chemical Society, copyright 2015. (d) MFCM for rapid virus detection. Reproduced from ref. 17 with permission from Elsevier, copyright 2008. | |
Faez et al.206 presented a technique that can trace unlabelled dielectric nanoparticles as tiny as 20 nm, at speeds of over 3 kHz for tens of seconds (Fig. 6c). A single-mode, step-index optical fibre with an open nanosised path inside the high-index core is the fundamental component of the technology. The particles were suspended in a solution that fills this channel. The laser was then directed through the centre of the fibre. A part of the optical mode in the fibre overlaps with the nanochannel cross-sectional area since the tube size is smaller than the light wavelength. The directed light scattered off the particles, passed through the transparent fibre cladding, and was captured by a microscope objective positioned vertical to the fibre axis. Although using optical fibres allows detecting nanoparticles without labelling, the rather sophisticated setup makes it difficult to be adapted for other applications. In another example, a MFCM has been developed with functional micro-devices capable of detecting and collecting viral particles.17 The proposed method effectively recognised a dengue virus sample with a concentration of 1000 PFU ml−1 in experimental findings. Fig. 6d illustrates the experimental processes using the MFCM. Surface-coated with streptavidin and conjugated with biotinylated capture antibodies, superparamagnetic beads with a diameter of 6 μm were combined with bio-samples in the incubation system. The targeted viruses can be collected and attached to the antibody-conjugated magnetic microspheres in a specified manner. After an on-chip washing process, the second set of prepared antibodies was mixed with the purified samples and cultivated with fluorescent dye to determine the target viruses. A microfluidic hydrodynamic focusing subsystem was used to drive the refined virus-magnetic-bead compounds. Following that, an optical detection system consisting of a laser generator as well as a photo-multiplier tube was used to stimulate and measure the fluorescent signal coupled to the second antibodies on the specific viruses. Finally, utilising the feedback signals from the optical detector, a sorting module (active microvalves) positioned downstream would sort and harvest virus-bound magnetic beads into the collecting chambers.
Rane et al.207 proposed a method without amplification for detecting pathogen-specific rRNA in individual bacterial samples. For counting E. coli spiked into suspensions, the investigators encapsulated single E. coli cells into 10-pL sized microdroplets and utilised a 16S rRNA-specific peptide nucleic acid (PNA) probe. This method could detect E. coli in low concentrations (equivalent to 5 × 106 CFU mL−1) and could perform continuously for higher throughput and dynamic range. Also, Kang et al.208 employed a DNAzyme sensor to detect E. coli spiked in 10% blood to prove the possibility of direct clinical sample analysis. Individual bacterial cells were enclosed in droplets with a volume of 14 pL and a diameter of 30 μm. The platform might produce a positive/negative result for the existence of the bacteria being examined after 45 minutes of lysis and DNAzyme response. The system was capable of evaluating dilute of E. coli from 1 to 104 CFU mL−1 after 3.5 hours. While fast bacterial detection for E. coli has been achieved using microdroplets with higher sensitivity, additional work is needed to enhance the capabilities of technologies to assess the presence of other pathogenic specimens. Furthermore, whereas the aforementioned systems have already shown pathogen identification with precultured E. coli, a droplet-based method that could instantly analyse culture-positive clinical samples has not been proven.
5.3 MFCM for detecting circulating nucleic acids
Dying cells can release cell-free DNA (cfDNA) and other nucleic acid fragments, possibly by active secretion.210 Tumour cells release mutant cfDNA, also called circulating tumour DNA (ctDNA), which is currently considered a marker and has been utilised as a prognostic diagnostic for several malignancies.14 The short half-life of cfDNA, ranging from 6 min to 2.5 hours, makes it useful for monitoring pharmacological treatment response. cfDNA levels in blood vary from 1–10 ng mL−1 in healthy people, but they can reach 1000 ng mL−1 in patients with cancer, equating to 3000–360
000 mark genes per millilitre of plasma.209,211 High cfDNA levels, on the other hand, can become a sign of inflammation, benign tumours, or tissue damaging.211 While cfDNA has received the greatest attention due to its reliability, cell-free mRNA, microRNA, nucleosomes, and viral DNA are all being explored.210
As shown in Fig. 7a–c, a multiplexed digital PCR platform was introduced by RainDance Technologies for measuring six specific somatic mutations related to colorectal cancer in the Kirsten rat sarcoma virus (KRAS) oncogene at the same time.209 This approach could screen millions of responses from a single sample while consuming less reagent per sample owing to the use of picolitre droplets. Additionally, the researchers were capable of checking each sample for six mutations at the same time by optically coding individual probe microdroplets with different quantities of fluorescent dye and passively combing the sample and the probe microdroplets. Commercial product, RainDrop, from RainDance Technologies has been employed for tracking mutations in KRAS for colorectal cancer167 as well as EGFR for lung cancer,153 B-type Raf kinase (BRAF) for Langerhans cell histiocytosis,152 and a 5-plex genotyping assay for spinal muscular atrophy.166 However, practically all clinical droplet digital PCR solutions have depended on an additional benchtop procedure for nucleic acid extraction and purification before droplets production. Several microfluidic technologies have been introduced to better enhance and incorporate DNA extraction into the microfluidic PCR process.212–214 Despite this, none of these systems has been included in droplet digital PCR procedures. As a result, additional incorporation of sample preparation processes is required to fully realise the sample-in-answer-out potential of microdroplet-enabled systems.
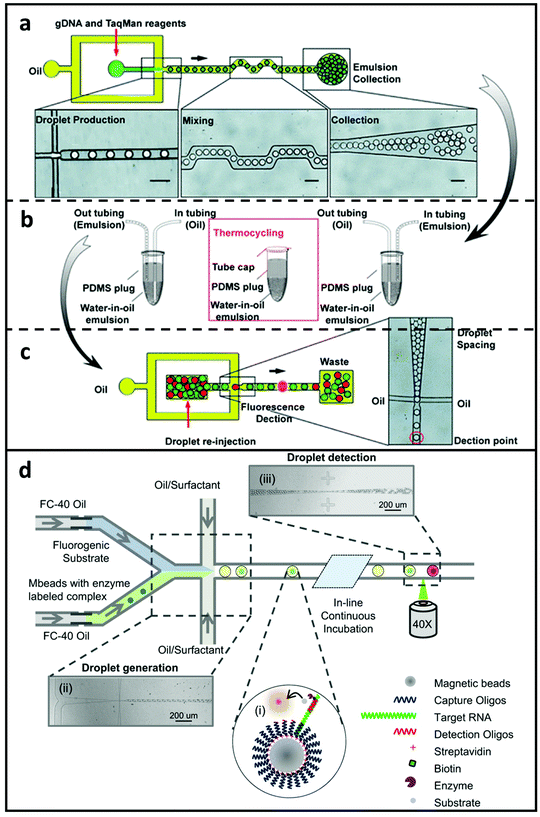 |
| Fig. 7 Droplet-based MFCM for detecting nucleic acid biomarkers. (a–c) Illustrations of a microdroplet digital PCR platform for mutations screening in the KRAS oncogene. TaqMan probes and mutant genes were enclosed in microdroplets with just one haploid genome each. After that, the droplets were thermocycled and re-introduced for fluorescence detecting. This technology was used to identify six different mutations in KRAS at the same time by optically coding droplet groups. Reproduced from ref. 209 with permission from Royal Society of Chemistry, copyright 2011. (d) Co-flowed into droplets with appropriate enzyme substrate forms a sandwiched complex comprising of capture oligo-coated magnetic microspheres hybridised to a single molecule of specific RNA, which is subsequently hybridised to an enzyme-linked detection oligo. Microdroplets containing individual RNA glow brightly after incubation, while microdroplets without the sandwiched complex fluoresce dimly. Reproduced from ref. 180 with permission from Springer Nature, copyright 2015. | |
Custom droplet PCR technologies for detecting viral nucleic acids have also been established, with an emphasis on speed, quantification, and integration. Kiss et al.170 established a combined solution to amplify and measure adenoviral DNA in 65-pL microdroplets. The researchers were able to screen millions of interactions in a continuous manner and identify signals from amplification in as short as 35 minutes enabled by the incorporation of the micro-sized droplets and the optimisation of the workflow procedures within a single platform. Furthermore, the platform's parallel sample interrogation regions enabled the production of real-time PCR fluorescence curves. While this study established quantification of the pAdeasy-1 adenoviral vector, a sensitive RNA quantification system is necessary for additional clinically relevant viral load testing, such as HIV-1 and HCV. To this end, Rački et al.215 designed a rotavirus quantification method that achieved viral RNA extraction, reverse transcription, and on-chip droplet digital PCR to measure viral RNA in wastewater. While custom platforms can be designed to be fully integrated and automated, the relatively small device footprint limits the throughput to roughly 100 droplets per experiment. Guan et al.180 established a more comprehensive platform than the earlier study, which used a platform without amplification for absolute bacterial (N. gonorrhoea) rRNA quantification. An enzyme-linked oligonucleotide hybridization assay (ELOHA) for quantification of RNA was established in this research, which enabled RNA molecules to hybridise onto DNA catching probes which were coupled to magnetic microspheres. The collected RNA was then hybridised with enzyme-labelled detecting probes. After that, the microspheres were enveloped in microdroplets with a fluorogenic substrate for further identification and evaluation. Quantification of nucleic acid-laden microspheres using ELOHA yielded great accuracy (CV 10%) over a rather large dynamic range spanning three orders of magnitude (Fig. 7d). Compared to traditional amplification techniques for detecting nucleic acids, the work utilising droplets presents a unique opportunity to detect nucleic acids without the need for target or signal amplification, which provides an alternative to overcome limits including low amplification efficiency, susceptibility to contamination, and long thermocycling duration.
5.4 MFCM for detecting metabolites
Microfluidic systems have been introduced for low-abundance protein biomarker detection as well as multiplexed protein biomarker testing and analysis. This is then utilised in disease screenings for bacterial infections, cancer, diabetes, and endometriosis, amongst other things.
Droplet systems provide the ability to improve the LoD of enzyme-linked immunosorbent assays (ELISAs), which have become a gold standard for protein detecting at high sensitivity. In an ELISA test, proteins coupled to enzymes are conjugated to antibodies immobilised on the substrate surface or a dispersed microsphere.18 After protein conjugating, a fluorogenic substrate is added into the mixture, and proteins may be identified after processing by enzyme–substrate cleavage. A tagged secondary antibody can also be employed for identification. In order to multiplex analyte detecting, commercial immunoassay technologies rely on microbeads with optically coding or customising microarrays.19 Because of the natural intricacy in the production of microspheres or micro-scale arrays, most of these systems are costly and require a lot of supporting equipment.
The sensitivity of bulk ELISA tests is often restricted to picomolar concentrations or higher. Protein biomarkers might be in significantly lower concentrations for several disorders, such as prostate cancer.216 To meet this demand, Shim et al.182 designed a system using femtoliter droplets for single-molecule counting immunoassays. In this study, antibody-functionalised catch microspheres were combined with the target protein in PBS until each microsphere contained 0 or 1 copy of the target molecule. Next, the trapped proteins were then coupled with a detection antibody, followed by the streptavidin-conjugated β-galactosidase reporter enzyme. 2 × 104 microdroplets were produced that laden bead-analyte-enzyme complex and a fluorogenic substrate. Microspheres carrying the analyte–enzyme combination combined with the fluorogenic substrate began to glow shortly after microdroplet generation. Signals from microdroplets carrying single-molecule can be recognised by imaging utilising a CCD sensor after a quick 10-minute on-chip incubation process (shown in Fig. 8a). The scientists utilised their technology to identify PSA at 46 fM concentrations, which is much lower than bulk ELISA. The scientists also suggest that it is possible to easily scale up the incubating zone and detecting method to enhance dynamic range and perhaps minimise LoD. Droplets can also recognise low-abundance surface proteins. Joensson et al.183 described a technique for screening mammalian cells for the low-abundance surface protein CCR5, which is a biomarker for HIV-1 infection (Fig. 8b). Enzyme-tagged antibodies were combined with fluorogenic substrates inside microdroplets with diameters of 40 μm to mark the cells. The droplets were re-injected and identified after being incubated off-chip, and they demonstrated higher resolution for distinguishing cells expressing CCR5 than typical FACS-based approaches. In the above-mentioned molecular biomarker detecting platforms, the enhanced sensitivity requires mechanisms for rejecting assay inhibitors and sources of false-positive signals. To this end, samples may be prepared prior to encapsulation in droplets, such that the background molecules responsible for assay inhibition or false-positive signals are minimised or even completely removed. Most current droplet-based platforms have achieved high sensitivity by relying on some degree of off-chip sample preparation and/or biomarker purification. Therefore, one important future investigation should be the integration of on-chip sample preparation process to purify the sample or concentrate the target biomarkers prior to droplet generation.
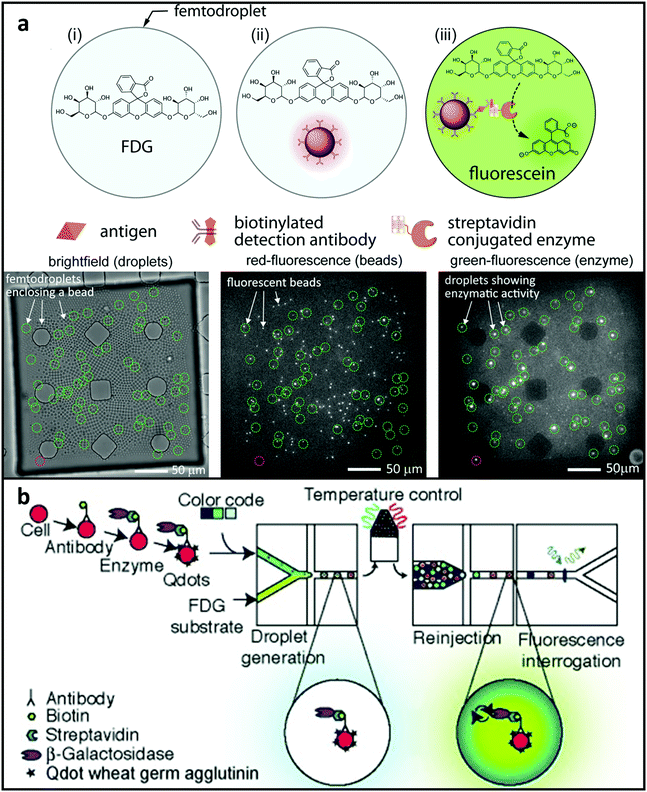 |
| Fig. 8 MFCM for detecting metabolites. (a) Illustration of a platform enabled by microsphere-based sandwich assay for PSA quantifying. The target protein was labelled by a reporter enzyme and hybridised to the microspheres and then modified microspheres and substrate were encapsulated together with microdroplets. Low concentration protein biomarkers can then be detected after incubation. Reproduced from ref. 182 with permission from American Chemical Society, copyright 2015. (b) Illustration of microdroplet MFCM using an enzyme-tagged antibody to screen the low-abundance cell-surface protein biomarker CCR5 in U937 cells. Reproduced from ref. 183 with permission from John Wiley and Sons, copyright 2009. | |
6 Conclusion and perspective
Over the past decade, benefitting from achievements in various technologies such as microfabrication and artificial intelligence, great efforts have been made in the development of MFCM including each subsystem, offering a promising future to make the MFCM achieve better performance in accuracy, cost, and throughput. This review has described the key submodules in a typical MFCM including focusing, detecting, and sorting systems. For each method presented, the characteristics and principles have been described and explained. In addition, the application of MFCM in blood-based biomarkers, such as cell-based and circulating nucleic acids-based has also been reviewed. Overall, this review offers a meaningful summary of the recent advances in microfluidic flow cytometers and their demonstration in blood-based biomarker analysis.
Despite a wide range of promising applications of MFCM, several under-developed and unsolved issues hinder fully unleashing its capabilities. Numerous on-chip platforms rely on bulky, expensive external supporting instruments. For example, the use of sheath flow for hydrodynamic focusing is unable to be achieved without several high-precision syringe pumps; fluorescence-based detecting systems require powerful laser modules and photodetectors. Integrated systems with novel microfluidic techniques such as SAWs or DEP have great potential in focusing and sorting systems without the need for bulky external equipment; however, the performance (e.g., throughput, reliability, repeatability) of such systems needs to be further characterised and improved. Image-based detection systems in MFCM attracts researchers’ attention due to the recent advances in artificial intelligence technology and because they contain high dimensional information, which may be a superior way forward for MFCM; however, powerful computers or field-programmable gate array (FPGA) modules with advanced processing capability and fast memories are needed to handle a significant amount of image data. In addition, existing commercial MFCM has not yet proven significant advantages over existing cytometry systems in terms of size and cost. Hence, more efforts and breakthroughs based on existing technologies are required to reduce the size, cost, and complexity of systems to realise low-cost POCT applications.
Author contributions
S.-Y.T. and J.G. proposed the review idea. Y.Z. and S.-Y.T. structured the review. S.-Y.T. and J.G. supervised the student. Y.Z. and Y. Zhao completed and edited the first draft and revised manuscript. All authors participated in writing the manuscript. All authors have read and agreed to the published version of the manuscript.
Conflicts of interest
The authors declare no conflict of interest.
Acknowledgements
This research received no external funding.
References
- Y. Gong, N. Fan, X. Yang, B. Peng and H. Jiang, Electrophoresis, 2019, 40, 1212–1229 CrossRef CAS PubMed.
- T. Haselgrübler, M. Haider, B. Ji, K. Juhasz, A. Sonnleitner, Z. Balogi and J. Hesse, Anal. Bioanal. Chem., 2014, 406, 3279–3296 CrossRef PubMed.
- G. M. Whitesides, Nature, 2006, 442, 368 CrossRef CAS PubMed.
- M. Li, H. Liu, S. Zhuang and K. Goda, RSC Adv., 2021, 11, 20944–20960 RSC.
- R.-J. Yang, L.-M. Fu and H.-H. Hou, Sens. Actuators, B, 2018, 266, 26–45 CrossRef CAS.
- A. Rajawat and S. Tripathi, Biomed. Eng. Lett., 2020, 10, 241–257 CrossRef PubMed.
- S. Nahavandi, S. Baratchi, R. Soffe, S.-Y. Tang, S. Nahavandi, A. Mitchell and K. Khoshmanesh, Lab Chip, 2014, 14, 1496–1514 RSC.
- J. Luo, C. Chen and Q. Li, Electrophoresis, 2020, 41, 1450–1468 CrossRef CAS PubMed.
- J. L. Garcia-Cordero and S. J. Maerkl, Curr. Opin. Biotechnol., 2020, 65, 37–44 CrossRef CAS PubMed.
- C. D. Chin, T. Laksanasopin, Y. K. Cheung, D. Steinmiller, V. Linder, H. Parsa, J. Wang, H. Moore, R. Rouse, G. Umviligihozo, E. Karita, L. Mwambarangwe, S. L. Braunstein, J. van de Wijgert, R. Sahabo, J. E. Justman, W. El-Sadr and S. K. Sia, Nat. Med., 2011, 17, 1015–1019 CrossRef CAS PubMed.
- H. Chen, K. Liu, Z. Li and P. Wang, Clin. Chim. Acta, 2019, 493, 138–147 CrossRef CAS PubMed.
- M. Sonker, V. Sahore and A. T. Woolley, Anal. Chim. Acta, 2017, 986, 1–11 CrossRef CAS PubMed.
- A. M. Kaushik, K. Hsieh and T.-H. Wang, WIREs Nanomed. Nanobiotechnol., 2018, 10, e1522 Search PubMed.
- C. Bettegowda, M. Sausen, R. J. Leary, I. Kinde, Y. Wang, N. Agrawal, B. R. Bartlett, H. Wang, B. Luber, R. M. Alani, E. S. Antonarakis, N. S. Azad, A. Bardelli, H. Brem, J. L. Cameron, C. C. Lee, L. A. Fecher, G. L. Gallia, P. Gibbs, D. Le, R. L. Giuntoli, M. Goggins, M. D. Hogarty, M. Holdhoff, S.-M. Hong, Y. Jiao, H. H. Juhl, J. J. Kim, G. Siravegna, D. A. Laheru, C. Lauricella, M. Lim, E. J. Lipson, S. K. N. Marie, G. J. Netto, K. S. Oliner, A. Olivi, L. Olsson, G. J. Riggins, A. Sartore-Bianchi, K. Schmidt, L.-M. Shih, S. M. Oba-Shinjo, S. Siena, D. Theodorescu, J. Tie, T. T. Harkins, S. Veronese, T.-L. Wang, J. D. Weingart, C. L. Wolfgang, L. D. Wood, D. Xing, R. H. Hruban, J. Wu, P. J. Allen, C. M. Schmidt, M. A. Choti, V. E. Velculescu, K. W. Kinzler, B. Vogelstein, N. Papadopoulos and L. A. Diaz, Sci. Transl. Med., 2014, 6, 224ra224 Search PubMed.
- G. Wen, T. Zhou and W. Gu, Protein Cell, 2021, 12(12), 911–946 CrossRef CAS PubMed.
- P. S. Mitchell, R. K. Parkin, E. M. Kroh, B. R. Fritz, S. K. Wyman, E. L. Pogosova-Agadjanyan, A. Peterson, J. Noteboom, K. C. O'Briant, A. Allen, D. W. Lin, N. Urban, C. W. Drescher, B. S. Knudsen, D. L. Stirewalt, R. Gentleman, R. L. Vessella, P. S. Nelson, D. B. Martin and M. Tewari, Proc. Natl. Acad. Sci. U. S. A., 2008, 105, 10513–10518 CrossRef CAS PubMed.
- S.-Y. Yang, K.-Y. Lien, K.-J. Huang, H.-Y. Lei and G.-B. Lee, Biosens. Bioelectron., 2008, 24, 855–862 CrossRef CAS PubMed.
- P. J. Tighe, R. R. Ryder, I. Todd and L. C. Fairclough, Proteomics – Clin. Appl., 2015, 9, 406–422 CrossRef CAS PubMed.
- S. X. Leng, J. E. McElhaney, J. D. Walston, D. Xie, N. S. Fedarko and G. A. Kuchel, J. Gerontol., Ser. A, 2008, 63, 879–884 CrossRef PubMed.
- T. A. Yap, D. Lorente, A. Omlin, D. Olmos and J. S. de Bono, Clin. Cancer Res., 2014, 20, 2553–2568 CrossRef CAS PubMed.
- J. S. Ankeny, C. M. Court, S. Hou, Q. Li, M. Song, D. Wu, J. F. Chen, T. Lee, M. Lin, S. Sho, M. M. Rochefort, M. D. Girgis, J. Yao, Z. A. Wainberg, V. R. Muthusamy, R. R. Watson, T. R. Donahue, O. J. Hines, H. A. Reber, T. G. Graeber, H. R. Tseng and J. S. Tomlinson, Br. J. Cancer, 2016, 114, 1367–1375 CrossRef CAS PubMed.
- D. C. Danila, K. Pantel, M. Fleisher and H. I. Scher, Cancer J., 2011, 17, 438–450 CrossRef CAS PubMed.
- A. M. Kaushik, K. Hsieh, L. Chen, D. J. Shin, J. C. Liao and T.-H. Wang, Biosens. Bioelectron., 2017, 97, 260–266 CrossRef CAS PubMed.
- Y.-F. Lee, K.-Y. Lien, H.-Y. Lei and G.-B. Lee, Biosens. Bioelectron., 2009, 25, 745–752 CrossRef CAS PubMed.
- H. Inan, S. Wang, F. Inci, M. Baday, R. Zangar, S. Kesiraju, K. S. Anderson, B. T. Cunningham and U. Demirci, Sci. Rep., 2017, 7, 3322 CrossRef PubMed.
- S. Yan and D. Yuan, Talanta, 2021, 221, 121401 CrossRef CAS PubMed.
- X. Xuan, J. Zhu and C. Church, Microfluid. Nanofluid., 2010, 9, 1–16 CrossRef.
- T. Zhang, Z.-Y. Hong, S.-Y. Tang, W. Li, D. W. Inglis, Y. Hosokawa, Y. Yalikun and M. Li, Lab Chip, 2020, 20, 35–53 RSC.
- L.-M. Fu, C.-H. Tsai and C.-H. Lin, Electrophoresis, 2008, 29, 1874–1880 CrossRef CAS PubMed.
- R.-J. Yang, H.-H. Hou, Y.-N. Wang, C.-H. Lin and L.-M. Fu, Biomicrofluidics, 2012, 6, 034110 CrossRef PubMed.
- J. Kim, J. Lee, C. Wu, S. Nam, D. Di Carlo and W. Lee, Lab Chip, 2016, 16, 992–1001 RSC.
- X. Wang, M. Zandi, C.-C. Ho, N. Kaval and I. Papautsky, Lab Chip, 2015, 15, 1812–1821 RSC.
- J. Zhou, P. V. Giridhar, S. Kasper and I. Papautsky, Lab Chip, 2013, 13, 1919–1929 RSC.
- N. Xiang, X. Zhang, Q. Dai, J. Cheng, K. Chen and Z. Ni, Lab Chip, 2016, 16, 2626–2635 RSC.
- L.-L. Fan, X.-K. He, Y. Han, J. Zhe and L. Zhao, J. Micromech. Microeng., 2015, 25, 035020 CrossRef.
- I. D. Johnston, M. B. McDonnell, C. K. L. Tan, D. K. McCluskey, M. J. Davies and M. C. Tracey, Microfluid. Nanofluid., 2014, 17, 509–518 CrossRef CAS.
- D. Yang, H. Zou, W. Zhong and T. Xu, Microelectron. Eng., 2015, 139, 48–52 CrossRef CAS.
- A. J. Chung, D. R. Gossett and D. Di Carlo, Small, 2013, 9, 685–690 CrossRef CAS PubMed.
- A. J. Chung, D. Pulido, J. C. Oka, H. Amini, M. Masaeli and D. Di Carlo, Lab Chip, 2013, 13, 2942–2949 RSC.
- J.-S. Park, S.-H. Song and H.-I. Jung, Lab Chip, 2009, 9, 939–948 RSC.
- N. Hashemi, J. P. B. Howell, J. S. Erickson, J. P. Golden and F. S. Ligler, Lab Chip, 2010, 10, 1952–1959 RSC.
- J. Zhang, S. Yan, D. Yuan, G. Alici, N.-T. Nguyen, M. E. Warkiani and W. Li, Lab Chip, 2016, 16, 10–34 RSC.
- J. Oakey, R. W. Applegate, E. Arellano, D. D. Carlo, S. W. Graves and M. Toner, Anal. Chem., 2010, 82, 3862–3867 CrossRef CAS PubMed.
- W. Tang, D. Tang, Z. Ni, N. Xiang and H. Yi, Anal. Chem., 2017, 89, 3154–3161 CrossRef CAS PubMed.
- W.-P. Chou, H.-M. Wang, J.-H. Chang, T.-K. Chiu, C.-H. Hsieh, C.-J. Liao and M.-H. Wu, Sens. Actuators, B, 2017, 241, 245–254 CrossRef CAS.
- H. Sadeghian, Y. Hojjat and M. Soleimani, J. Electrost., 2017, 86, 41–49 CrossRef CAS.
- Z. Zhu, X. Xu, L. Fang, D. Pan and Q.-a. Huang, Sens. Actuators, B, 2016, 235, 515–524 CrossRef CAS.
- A. Salari and M. Thompson, Sens. Actuators, B, 2018, 255, 3601–3615 CrossRef CAS.
- C.-H. Lin, G.-B. Lee, L.-M. Fu and B.-H. Hwey, J. Microelectromech. Syst., 2004, 13, 923–932 CrossRef.
- A. Fakhfouri, C. Devendran, T. Albrecht, D. J. Collins, A. Winkler, H. Schmidt and A. Neild, Lab Chip, 2018, 18, 2214–2224 RSC.
- I. Leibacher, S. Schatzer and J. Dual, Lab Chip, 2014, 14, 463–470 RSC.
- I. Leibacher, P. Reichert and J. Dual, Lab Chip, 2015, 15, 2896–2905 RSC.
- B. Ayan, A. Ozcelik, H. Bachman, S.-Y. Tang, Y. Xie, M. Wu, P. Li and T. J. Huang, Lab Chip, 2016, 16, 4366–4372 RSC.
- G. Destgeer, J. H. Jung, J. Park, H. Ahmed, K. Park, R. Ahmad and H. J. Sung, RSC Adv., 2017, 7, 22524–22530 RSC.
- Z. Ma, Y. Zhou, D. J. Collins and Y. Ai, Lab Chip, 2017, 17, 3176–3185 RSC.
- J. Lee, C. Rhyou, B. Kang and H. Lee, J. Phys. D: Appl. Phys., 2017, 50, 165401 CrossRef.
- Z. Mao, Y. Xie, F. Guo, L. Ren, P.-H. Huang, Y. Chen, J. Rufo, F. Costanzo and T. J. Huang, Lab Chip, 2016, 16, 515–524 RSC.
- R. Kishor, Z. Ma, S. Sreejith, Y. P. Seah, H. Wang, Y. Ai, Z. Wang, T.-T. Lim and Y. Zheng, Sens. Actuators, B, 2017, 252, 568–576 CrossRef CAS.
- D. J. Collins, Z. Ma, J. Han and Y. Ai, Lab Chip, 2017, 17, 91–103 RSC.
- Z. Ma, D. J. Collins and Y. Ai, Anal. Chem., 2016, 88, 5316–5323 CrossRef CAS PubMed.
- C. Devendran, K. Choi, J. Han, Y. Ai, A. Neild and D. J. Collins, Lab Chip, 2020, 20, 2674–2688 RSC.
- M. Wu, K. Chen, S. Yang, Z. Wang, P.-H. Huang, J. Mai, Z.-Y. Li and T. J. Huang, Lab Chip, 2018, 18, 3003–3010 RSC.
- A. A. Nawaz, X. Zhang, X. Mao, J. Rufo, S.-C. S. Lin, F. Guo, Y. Zhao, M. Lapsley, P. Li, J. P. McCoy, S. J. Levine and T. J. Huang, Lab Chip, 2014, 14, 415–423 RSC.
- X. Mao, A. A. Nawaz, S.-C. S. Lin, M. I. Lapsley, Y. Zhao, J. P. McCoy, W. S. El-Deiry and T. J. Huang, Biomicrofluidics, 2012, 6, 024113 CrossRef PubMed.
- Y. Chen, A. A. Nawaz, Y. Zhao, P.-H. Huang, J. P. McCoy, S. J. Levine, L. Wang and T. J. Huang, Lab Chip, 2014, 14, 916–923 RSC.
- C. Grenvall, C. Antfolk, C. Z. Bisgaard and T. Laurell, Lab Chip, 2014, 14, 4629–4637 RSC.
- A. Kalantarifard, A. Saateh and C. Elbuken, Chemosensors, 2018, 6, 23 CrossRef.
- H.-D. Xi, H. Zheng, W. Guo, A. M. Gañán-Calvo, Y. Ai, C.-W. Tsao, J. Zhou, W. Li, Y. Huang, N.-T. Nguyen and S. H. Tan, Lab Chip, 2017, 17, 751–771 RSC.
- S. L. Sjostrom, Y. Bai, M. Huang, Z. Liu, J. Nielsen, H. N. Joensson and H. Andersson Svahn, Lab Chip, 2014, 14, 806–813 RSC.
- R. H. Cole, S.-Y. Tang, C. A. Siltanen, P. Shahi, J. Q. Zhang, S. Poust, Z. J. Gartner and A. R. Abate, Proc. Natl. Acad. Sci. U. S. A., 2017, 114, 8728–8733 CrossRef CAS PubMed.
- C. Wu, X. Wei, X. Men, X. Zhang, Y.-L. Yu, Z.-R. Xu, M.-L. Chen and J.-H. Wang, Anal. Chem., 2021, 93(23), 8203–8209 CrossRef CAS PubMed.
- C. Wang, Y. Ma, Z. Chen, Y. Wu, F. Song, J. Qiu, M. Shi and X. Wu, Cytometry, Part A, 2021, 99(10), 987–998 CrossRef CAS PubMed.
- Y.-J. Fan, Y.-C. Hsiao, Y.-L. Weng, Y.-H. Chen, P.-Y. Chiou and H.-J. Sheen, Sens. Actuators, B, 2020, 320, 128255 CrossRef CAS.
- H. Pei, L. Li, Y. Wang, R. Sheng, Y. Wang, S. Xie, L. Shui, H. Si and B. Tang, Anal. Chem., 2019, 91, 11078–11084 CrossRef CAS PubMed.
- J. Wang, Z. Fan, Y. Zhao, Y. Song, H. Chu, W. Song, Y. Song, X. Pan, Y. Sun and D. Li, Sci. Rep., 2016, 6, 23165 CrossRef CAS PubMed.
- B. L. Fiedler, S. Van Buskirk, K. P. Carter, Y. Qin, M. C. Carpenter, A. E. Palmer and R. Jimenez, Anal. Chem., 2017, 89, 711–719 CrossRef CAS PubMed.
- X. Li, B. Fan, S. Cao, D. Chen, X. Zhao, D. Men, W. Yue, J. Wang and J. Chen, Lab Chip, 2017, 17, 3129–3137 RSC.
- T. Sato and R. Miyake, Anal. Methods, 2017, 9, 3992–3997 RSC.
- C. Kunstmann-Olsen, M. M. Hanczyc, J. Hoyland, S. Rasmussen and H.-G. Rubahn, Sens. Actuators, B, 2016, 229, 7–13 CrossRef CAS.
- C.-S. Yan and Y.-N. Wang, Biomed. Opt. Express, 2016, 7, 3585–3595 CrossRef PubMed.
- Y. J. Heo, D. Lee, J. Kang, K. Lee and W. K. Chung, Sci. Rep., 2017, 7, 11651 CrossRef PubMed.
- D. M. D. Siu, K. C. M. Lee, M. C. K. Lo, S. V. Stassen, M. Wang, I. Z. Q. Zhang, H. K. H. So, G. C. F. Chan, K. S. E. Cheah, K. K. Y. Wong, M. K. Y. Hsin, J. C. M. Ho and K. K. Tsia, Lab Chip, 2020, 20, 3696–3708 RSC.
- A. Isozaki, J. Harmon, Y. Zhou, S. Li, Y. Nakagawa, M. Hayashi, H. Mikami, C. Lei and K. Goda, Lab Chip, 2020, 20, 3074–3090 RSC.
- H. Mikami, M. Kawaguchi, C.-J. Huang, H. Matsumura, T. Sugimura, K. Huang, C. Lei, S. Ueno, T. Miura, T. Ito, K. Nagasawa, T. Maeno, H. Watarai, M. Yamagishi, S. Uemura, S. Ohnuki, Y. Ohya, H. Kurokawa, S. Matsusaka, C.-W. Sun, Y. Ozeki and K. Goda, Nat. Commun., 2020, 11, 1162 CrossRef CAS PubMed.
- A. Isozaki, H. Mikami, H. Tezuka, H. Matsumura, K. Huang, M. Akamine, K. Hiramatsu, T. Iino, T. Ito, H. Karakawa, Y. Kasai, Y. Li, Y. Nakagawa, S. Ohnuki, T. Ota, Y. Qian, S. Sakuma, T. Sekiya, Y. Shirasaki, N. Suzuki, E. Tayyabi, T. Wakamiya, M. Xu, M. Yamagishi, H. Yan, Q. Yu, S. Yan, D. Yuan, W. Zhang, Y. Zhao, F. Arai, R. E. Campbell, C. Danelon, D. Di Carlo, K. Hiraki, Y. Hoshino, Y. Hosokawa, M. Inaba, A. Nakagawa, Y. Ohya, M. Oikawa, S. Uemura, Y. Ozeki, T. Sugimura, N. Nitta and K. Goda, Lab Chip, 2020, 20, 2263–2273 RSC.
- N. Nitta, T. Sugimura, A. Isozaki, H. Mikami, K. Hiraki, S. Sakuma, T. Iino, F. Arai, T. Endo, Y. Fujiwaki, H. Fukuzawa, M. Hase, T. Hayakawa, K. Hiramatsu, Y. Hoshino, M. Inaba, T. Ito, H. Karakawa, Y. Kasai, K. Koizumi, S. Lee, C. Lei, M. Li, T. Maeno, S. Matsusaka, D. Murakami, A. Nakagawa, Y. Oguchi, M. Oikawa, T. Ota, K. Shiba, H. Shintaku, Y. Shirasaki, K. Suga, Y. Suzuki, N. Suzuki, Y. Tanaka, H. Tezuka, C. Toyokawa, Y. Yalikun, M. Yamada, M. Yamagishi, T. Yamano, A. Yasumoto, Y. Yatomi, M. Yazawa, D. Di Carlo, Y. Hosokawa, S. Uemura, Y. Ozeki and K. Goda, Cell, 2018, 175, 266–276 CrossRef CAS PubMed.
- Y. Han, R. Tang, Y. Gu, A. C. Zhang, W. Cai, V. Castor, S. H. Cho, W. Alaynick and Y.-H. Lo, Optica, 2019, 6, 1297–1304 CrossRef CAS.
- Y. Gu, A. C. Zhang, Y. Han, J. Li, C. Chen and Y.-H. Lo, Cytometry, Part A, 2019, 95, 499–509 CrossRef PubMed.
- Y. Han, Y. Gu, A. C. Zhang and Y.-H. Lo, Lab Chip, 2016, 16, 4639–4647 RSC.
- M. Ossandon, J. Balsam, H. A. Bruck, K. Kalpakis and A. Rasooly, Analyst, 2017, 142, 641–648 RSC.
- S. Zhu, X. Zhang, Z. Zhou, Y. Han, N. Xiang and Z. Ni, Talanta, 2021, 233, 122571 CrossRef CAS PubMed.
- Q. Zi, W. Ding, C. Sun, S. Li, D. Gao, L. He, J. Liu, L. Xu and B. Qiu, Biosens. Bioelectron., 2020, 148, 111820 CrossRef CAS PubMed.
- T. Tang, X. Liu, R. Kiya, Y. Shen, Y. Yuan, T. Zhang, K. Suzuki, Y. Tanaka, M. Li, Y. Hosokawa and Y. Yalikun, Biosens. Bioelectron., 2021, 193, 113521 CrossRef CAS PubMed.
- L. Gong, C. Petchakup, P. Shi, P. L. Tan, L. P. Tan, C. Y. Tay and H. W. Hou, Small, 2021, 17, 2007500 CrossRef CAS PubMed.
- C. Petchakup, K. H. H. Li and H. W. Hou, Micromachines, 2017, 8, 87 CrossRef.
- J. Chen, C. Xue, Y. Zhao, D. Chen, M.-H. Wu and J. Wang, Int. J. Mol. Sci., 2015, 16, 9804–9830 CrossRef CAS PubMed.
- M. Serhatlioglu, M. Asghari, M. Tahsin Guler and C. Elbuken, Electrophoresis, 2019, 40, 906–913 CrossRef CAS PubMed.
- Y. Feng, L. Huang, P. Zhao, F. Liang and W. Wang, Anal. Chem., 2019, 91, 15204–15212 CrossRef CAS PubMed.
- A. K. S. Lau, H. C. Shum, K. K. Y. Wong and K. K. Tsia, Lab Chip, 2016, 16, 1743–1756 RSC.
- M. Kumar, S. Yadav, A. Kumar, N. N. Sharma, J. Akhtar and K. Singh, Biosens. Bioelectron., 2019, 142, 111526 CrossRef CAS PubMed.
- S. Stavrakis, G. Holzner, J. Choo and A. deMello, Curr. Opin. Biotechnol., 2019, 55, 36–43 CrossRef CAS PubMed.
- P. Simon, M. Frankowski, N. Bock and J. Neukammer, Lab Chip, 2016, 16, 2326–2338 RSC.
- X. Liu, X. Huang, Y. Jiang, H. Xu, J. Guo, H. W. Hou, M. Yan and H. Yu, IEEE Trans. Biomed. Circuits Syst., 2017, 11, 794–803 Search PubMed.
- K. B. Roth, C. D. Eggleton, K. B. Neeves and D. W. M. Marr, Lab Chip, 2013, 13, 1571–1577 RSC.
- Z. Liu, Y. Lee, J. h. Jang, Y. Li, X. Han, K. Yokoi, M. Ferrari, L. Zhou and L. Qin, Sci. Rep., 2015, 5, 14272 CrossRef CAS PubMed.
- T. Sawetzki, C. D. Eggleton, S. A. Desai and D. W. M. Marr, Biophys. J., 2013, 105, 2281–2288 CrossRef CAS PubMed.
- J. Zhang, X. A. Nou, H. Kim and G. Scarcelli, Lab Chip, 2017, 17, 663–670 RSC.
- T. Blasi, H. Hennig, H. D. Summers, F. J. Theis, J. Cerveira, J. O. Patterson, D. Davies, A. Filby, A. E. Carpenter and P. Rees, Nat. Commun., 2016, 7, 10256 CrossRef CAS PubMed.
- K. Goda, A. Ayazi, D. R. Gossett, J. Sadasivam, C. K. Lonappan, E. Sollier, A. M. Fard, S. C. Hur, J. Adam, C. Murray, C. Wang, N. Brackbill, D. Di Carlo and B. Jalali, Proc. Natl. Acad. Sci. U. S. A., 2012, 109, 11630–11635 CrossRef CAS PubMed.
- Y. Jiang, C. Lei, A. Yasumoto, H. Kobayashi, Y. Aisaka, T. Ito, B. Guo, N. Nitta, N. Kutsuna, Y. Ozeki, A. Nakagawa, Y. Yatomi and K. Goda, Lab Chip, 2017, 17, 2426–2434 RSC.
- J. Zheng, T. Cole, Y. Zhang, J. Kim and S.-Y. Tang, Biosens. Bioelectron., 2021, 194, 113666 CrossRef CAS PubMed.
- A. Gupta, P. J. Harrison, H. Wieslander, N. Pielawski, K. Kartasalo, G. Partel, L. Solorzano, A. Suveer, A. H. Klemm, O. Spjuth, I.-M. Sintorn and C. Wählby, Cytometry, Part A, 2019, 95, 366–380 CrossRef PubMed.
- K. C. Cheung, M. Di Berardino, G. Schade-Kampmann, M. Hebeisen, A. Pierzchalski, J. Bocsi, A. Mittag and A. Tárnok, Cytometry, Part A, 2010, 77A, 648–666 CrossRef CAS PubMed.
- F. Caselli and P. Bisegna, Med. Eng. Phys., 2017, 48, 81–89 CrossRef PubMed.
- A. De Ninno, V. Errico, F. R. Bertani, L. Businaro, P. Bisegna and F. Caselli, Lab Chip, 2017, 17, 1158–1166 RSC.
- J. S. McGrath, C. Honrado, D. Spencer, B. Horton, H. L. Bridle and H. Morgan, Sci. Rep., 2017, 7, 2601 CrossRef CAS PubMed.
- Y. Zhao, X. T. Zhao, D. Y. Chen, Y. N. Luo, M. Jiang, C. Wei, R. Long, W. T. Yue, J. B. Wang and J. Chen, Biosens. Bioelectron., 2014, 57, 245–253 CrossRef CAS PubMed.
- C. Wyatt Shields Iv, C. D. Reyes and G. P. López, Lab Chip, 2015, 15, 1230–1249 RSC.
- A. Menachery, N. Kumawat and M. Qasaimeh, TrAC, Trends Anal. Chem., 2017, 89, 1–12 CrossRef CAS.
- C. Liu, Cytometry, Part A, 2018, 93, 589–591 CrossRef PubMed.
- A. Lenshof and T. Laurell, Chem. Soc. Rev., 2010, 39, 1203–1217 RSC.
- A. Reece, B. Xia, Z. Jiang, B. Noren, R. McBride and J. Oakey, Curr. Opin. Biotechnol., 2016, 40, 90–96 CrossRef CAS PubMed.
- M. A. Witek, I. M. Freed and S. A. Soper, Anal. Chem., 2020, 92, 105–131 CrossRef CAS PubMed.
- Y. Shen, Y. Yalikun and Y. Tanaka, Sens. Actuators, B, 2019, 282, 268–281 CrossRef CAS.
- Z. Cheng, X. Wu, J. Cheng and P. Liu, Microfluid. Nanofluid., 2017, 21, 9 CrossRef.
- A. Isozaki, Y. Nakagawa, M. H. Loo, Y. Shibata, N. Tanaka, D. L. Setyaningrum, J.-W. Park, Y. Shirasaki, H. Mikami, D. Huang, H. Tsoi, C. T. Riche, T. Ota, H. Miwa, Y. Kanda, T. Ito, K. Yamada, O. Iwata, K. Suzuki, S. Ohnuki, Y. Ohya, Y. Kato, T. Hasunuma, S. Matsusaka, M. Yamagishi, M. Yazawa, S. Uemura, K. Nagasawa, H. Watarai, D. D. Carlo and K. Goda, Sci. Adv., 2020, 6, eaba6712 CrossRef CAS PubMed.
- D. J. Collins, A. Neild and Y. Ai, Lab Chip, 2016, 16, 471–479 RSC.
- S. Sakuma, Y. Kasai, T. Hayakawa and F. Arai, Lab Chip, 2017, 17, 2760–2767 RSC.
- B. de Wagenaar, S. Dekker, H. L. de Boer, J. G. Bomer, W. Olthuis, A. van den Berg and L. I. Segerink, Lab Chip, 2016, 16, 1514–1522 RSC.
- M. Huang, Y. Bai, S. L. Sjostrom, B. M. Hallström, Z. Liu, D. Petranovic, M. Uhlén, H. N. Joensson, H. Andersson-Svahn and J. Nielsen, Proc. Natl. Acad. Sci. U. S. A., 2015, 112, E4689–E4696 CAS.
- B. E. Debs, R. Utharala, I. V. Balyasnikova, A. D. Griffiths and C. A. Merten, Proc. Natl. Acad. Sci. U. S. A., 2012, 109, 11570–11575 CrossRef PubMed.
- T. Beneyton, F. Coldren, J.-C. Baret, A. D. Griffiths and V. Taly, Analyst, 2014, 139, 3314–3323 RSC.
- B. Watson, J. Friend and L. Yeo, Sens. Actuators, A, 2009, 152, 219–233 CrossRef CAS.
- A. Barani, H. Paktinat, M. Janmaleki, A. Mohammadi, P. Mosaddegh, A. Fadaei-Tehrani and A. Sanati-Nezhad, Biosens. Bioelectron., 2016, 85, 714–725 CrossRef CAS PubMed.
- T. Jing, R. Ramji, M. E. Warkiani, J. Han, C. T. Lim and C.-H. Chen, Biosens. Bioelectron., 2015, 66, 19–23 CrossRef CAS PubMed.
- T. Jing, Z. Lai, L. Wu, J. Han, C. T. Lim and C.-H. Chen, Anal. Chem., 2016, 88, 11750–11757 CrossRef CAS PubMed.
- E. I. Galanzha and V. P. Zharov, Cancers, 2013, 5, 1691–1738 CrossRef PubMed.
- M. A. Juratli, M. Sarimollaoglu, E. R. Siegel, D. A. Nedosekin, E. I. Galanzha, J. Y. Suen and V. P. Zharov, Head Neck, 2014, 36, 1207–1215 CrossRef PubMed.
- B. Hamza, S. R. Ng, S. M. Prakadan, F. F. Delgado, C. R. Chin, E. M. King, L. F. Yang, S. M. Davidson, K. L. DeGouveia, N. Cermak, A. W. Navia, P. S. Winter, R. S. Drake, T. Tammela, C. M.-C. Li, T. Papagiannakopoulos, A. J. Gupta, J. Shaw Bagnall, S. M. Knudsen, M. G. Vander Heiden, S. C. Wasserman, T. Jacks, A. K. Shalek and S. R. Manalis, Proc. Natl. Acad. Sci. U. S. A., 2019, 116, 2232–2236 CrossRef CAS PubMed.
- S. Nagrath, L. V. Sequist, S. Maheswaran, D. W. Bell, D. Irimia, L. Ulkus, M. R. Smith, E. L. Kwak, S. Digumarthy, A. Muzikansky, P. Ryan, U. J. Balis, R. G. Tompkins, D. A. Haber and M. Toner, Nature, 2007, 450, 1235–1239 CrossRef CAS PubMed.
- Q. Li, S. Cui, Y. Xu, Y. Wang, F. Jin, H. Si, L. Li and B. Tang, Anal. Chem., 2019, 91, 14133–14140 CrossRef CAS PubMed.
- J. S. McGrath, C. Honrado, J. H. Moore, S. J. Adair, W. B. Varhue, A. Salahi, V. Farmehini, B. J. Goudreau, S. Nagdas, E. M. Blais, T. W. Bauer and N. S. Swami, Anal. Chim. Acta, 2020, 1101, 90–98 CrossRef CAS PubMed.
- A. Han, L. Yang and A. B. Frazier, Clin. Cancer Res., 2007, 13, 139–143 CrossRef PubMed.
-
A. Avram, C. Marculescu, C. M. Balan, C. Voitincu, C. Pirvulescu, M. Volmer, A. Popescu, M. Mihailescu and M. Avram, Microbiosensor for electrical impedance spectroscopic study of melanoma cells, CAS 2012 (International Semiconductor Conference), 2012, pp. 165–168.
- G. Qiao, W. Duan, C. Chatwin, A. Sinclair and W. Wang, J. Phys.: Conf. Ser., 2010, 224, 012081 CrossRef.
- O. Scheler, N. Pacocha, P. R. Debski, A. Ruszczak, T. S. Kaminski and P. Garstecki, Lab Chip, 2017, 17, 1980–1987 RSC.
- O. Scheler, T. S. Kaminski, A. Ruszczak and P. Garstecki, ACS Appl. Mater. Interfaces, 2016, 8, 11318–11325 CrossRef CAS PubMed.
- J. Q. Boedicker, L. Li, T. R. Kline and R. F. Ismagilov, Lab Chip, 2008, 8, 1265–1272 RSC.
- W. A. Schell, J. L. Benton, P. B. Smith, M. Poore, J. L. Rouse, D. J. Boles, M. D. Johnson, B. D. Alexander, V. K. Pamula, A. E. Eckhardt, M. G. Pollack, D. K. Benjamin, J. R. Perfect and T. G. Mitchell, Eur. J. Clin. Microbiol. Infect. Dis., 2012, 31, 2237–2245 CrossRef CAS PubMed.
- C. L. Stoffel, R. F. Kathy and K. L. Rowlen, Cytometry, Part A, 2005, 65A, 140–147 CrossRef PubMed.
- J. Rho, W. Jang, I. Hwang, D. Lee, C. H. Lee and T. D. Chung, Biosens. Bioelectron., 2018, 102, 121–128 CrossRef CAS PubMed.
- S. Héritier, J.-F. Emile, M.-A. Barkaoui, C. Thomas, S. Fraitag, S. Boudjemaa, F. Renaud, A. Moreau, M. Peuchmaur, C. Chassagne-Clément, F. Dijoud, V. Rigau, D. Moshous, A. Lambilliotte, F. Mazingue, K. Kebaili, J. Miron, E. Jeziorski, G. Plat, N. Aladjidi, A. Ferster, H. Pacquement, C. Galambrun, L. Brugières, G. Leverger, L. Mansuy, C. Paillard, A. Deville, C. Armari-Alla, A. Lutun, M. Gillibert-Yvert, J.-L. Stephan, F. Cohen-Aubart, J. Haroche, I. Pellier, F. Millot, B. Lescoeur, V. Gandemer, C. Bodemer, R. Lacave, Z. Hélias-Rodzewicz, V. Taly, F. Geissmann and J. Donadieu, J. Clin. Oncol., 2016, 34, 3023–3030 CrossRef PubMed.
- G. Zhu, X. Ye, Z. Dong, Y. C. Lu, Y. Sun, Y. Liu, R. McCormack, Y. Gu and X. Liu, J. Mol. Diagn., 2015, 17, 265–272 CrossRef CAS PubMed.
- E. Zonta, F. Garlan, N. Pécuchet, K. Perez-Toralla, O. Caen, C. Milbury, A. Didelot, E. Fabre, H. Blons, P. Laurent-Puig and V. Taly, PLoS One, 2016, 11, e0159094 CrossRef PubMed.
- M. Watanabe, T. Kawaguchi, S.-i. Isa, M. Ando, A. Tamiya, A. Kubo, H. Saka, S. Takeo, H. Adachi, T. Tagawa, S. Kakegawa, M. Yamashita, K. Kataoka, Y. Ichinose, Y. Takeuchi, K. Sakamoto, A. Matsumura and Y. Koh, Clin. Cancer Res, 2015, 21, 3552 CrossRef CAS PubMed.
- T. Takahama, K. Sakai, M. Takeda, K. Azuma, T. Hida, M. Hirabayashi, T. Oguri, H. Tanaka, N. Ebi, T. Sawa, A. Bessho, M. Tachihara, H. Akamatsu, S. Bandoh, D. Himeji, T. Ohira, M. Shimokawa, Y. Nakanishi, K. Nakagawa and K. Nishio, Oncotarget, 2016, 7(36), 58492–58499 CrossRef PubMed.
- M. F. Sanmamed, S. Fernández-Landázuri, C. Rodríguez, R. Zárate, M. D. Lozano, L. Zubiri, J. L. Perez-Gracia, S. Martín-Algarra and A. González, Clin. Chem., 2015, 61, 297–304 CrossRef CAS PubMed.
- A. G. Sacher, C. Paweletz, S. E. Dahlberg, R. S. Alden, A. O'Connell, N. Feeney, S. L. Mach, P. A. Jänne and G. R. Oxnard, JAMA Oncol., 2016, 2, 1014–1022 CrossRef PubMed.
- A. L. Reid, J. B. Freeman, M. Millward, M. Ziman and E. S. Gray, Clin. Biochem., 2015, 48, 999–1002 CrossRef CAS PubMed.
- G. R. Oxnard, C. P. Paweletz, Y. Kuang, S. L. Mach, A. Connell, M. M. Messineo, J. J. Luke, M. Butaney, P. Kirschmeier, D. M. Jackman and P. A. Jänne, Clin. Cancer Res., 2014, 20, 1698 CrossRef CAS PubMed.
- B. J. Hindson, K. D. Ness, D. A. Masquelier, P. Belgrader, N. J. Heredia, A. J. Makarewicz, I. J. Bright, M. Y. Lucero, A. L. Hiddessen, T. C. Legler, T. K. Kitano, M. R. Hodel, J. F. Petersen, P. W. Wyatt, E. R. Steenblock, P. H. Shah, L. J. Bousse, C. B. Troup, J. C. Mellen, D. K. Wittmann, N. G. Erndt, T. H. Cauley, R. T. Koehler, A. P. So, S. Dube, K. A. Rose, L. Montesclaros, S. Wang, D. P. Stumbo, S. P. Hodges, S. Romine, F. P. Milanovich, H. E. White, J. F. Regan, G. A. Karlin-Neumann, C. M. Hindson, S. Saxonov and B. W. Colston, Anal. Chem., 2011, 83, 8604–8610 CrossRef CAS PubMed.
- K. Otsuji, T. Sasaki, A. Tanaka, A. Kunita, M. Ikemura, K. Matsusaka, K. Tada, M. Fukayama and Y. Seto, Breast Cancer Res. Treat., 2017, 162, 11–18 CrossRef CAS PubMed.
- H. Kinugasa, K. Nouso, T. Tanaka, K. Miyahara, Y. Morimoto, C. Dohi, T. Matsubara, H. Okada and K. Yamamoto, Br. J. Cancer, 2015, 112, 1652–1655 CrossRef CAS PubMed.
- N. J. Heredia, P. Belgrader, S. Wang, R. Koehler, J. Regan, A. M. Cosman, S. Saxonov, B. Hindson, S. C. Tanner, A. S. Brown and G. Karlin-Neumann, Methods, 2013, 59, S20–S23 CrossRef CAS PubMed.
- P. Belgrader, S. C. Tanner, J. F. Regan, R. Koehler, B. J. Hindson and A. S. Brown, Clin. Chem., 2013, 59, 991–994 CrossRef CAS PubMed.
- Q. Zhong, S. Bhattacharya, S. Kotsopoulos, J. Olson, V. Taly, A. D. Griffiths, D. R. Link and J. W. Larson, Lab Chip, 2011, 11, 2167–2174 RSC.
- V. Taly, D. Pekin, L. Benhaim, S. K. Kotsopoulos, D. Le Corre, X. Li, I. Atochin, D. R. Link, A. D. Griffiths, K. Pallier, H. Blons, O. Bouché, B. Landi, J. B. Hutchison and P. Laurent-Puig, Clin. Chem., 2013, 59, 1722–1731 CrossRef CAS PubMed.
-
D. Pekin and V. Taly, in Microchip Diagnostics: Methods and Protocols, ed. V. Taly, J.-L. Viovy and S. Descroix, Springer New York, New York, NY, 2017, pp. 143–164, DOI:10.1007/978-1-4939-6734-6_12.
- P. Laurent-Puig, D. Pekin, C. Normand, S. K. Kotsopoulos, P. Nizard, K. Perez-Toralla, R. Rowell, J. Olson, P. Srinivasan, D. Le Corre, T. Hor, Z. El Harrak, X. Li, D. R. Link, O. Bouché, J.-F. Emile, B. Landi, V. Boige, J. B. Hutchison and V. Taly, Clin. Cancer Res., 2015, 21, 1087 CrossRef CAS PubMed.
- M. M. Kiss, L. Ortoleva-Donnelly, N. R. Beer, J. Warner, C. G. Bailey, B. W. Colston, J. M. Rothberg, D. R. Link and J. H. Leamon, Anal. Chem., 2008, 80, 8975–8981 CrossRef CAS PubMed.
- G. S. Brunetto, R. Massoud, E. C. Leibovitch, B. Caruso, K. Johnson, J. Ohayon, K. Fenton, I. Cortese and S. Jacobson, J. NeuroVirol., 2014, 20, 341–351 CrossRef CAS PubMed.
- J. Pavšič, J. Žel and M. Milavec, Anal. Bioanal. Chem., 2016, 408, 67–75 CrossRef PubMed.
- J.-T. Huang, Y.-J. Liu, J. Wang, Z.-G. Xu, Y. Yang, F. Shen, X.-h. Liu, X. Zhou and S.-M. Liu, Clin. Chem., 2015, 61, 290–296 CrossRef CAS PubMed.
- M. C. Strain, S. M. Lada, T. Luong, S. E. Rought, S. Gianella, V. H. Terry, C. A. Spina, C. H. Woelk and D. D. Richman, PLoS One, 2013, 8, e55943 CrossRef CAS PubMed.
- K. Kelley, A. Cosman, P. Belgrader, B. Chapman and D. C. Sullivan, J. Clin. Microbiol., 2013, 51, 2033–2039 CrossRef PubMed.
- J. Luo, J. Li, H. Yang, J. Yu, H. Wei and N. A. Ledeboer, J. Clin. Microbiol., 2017, 55, 2946–2955 CrossRef CAS PubMed.
- N. R. Beer, E. K. Wheeler, L. Lee-Houghton, N. Watkins, S. Nasarabadi, N. Hebert, P. Leung, D. W. Arnold, C. G. Bailey and B. W. Colston, Anal. Chem., 2008, 80, 1854–1858 CrossRef CAS PubMed.
- M. Mukaide, M. Sugiyama, M. Korenaga, K. Murata, T. Kanto, N. Masaki and M. Mizokami, J. Virol. Methods, 2014, 207, 169–177 CrossRef CAS PubMed.
- M. Kiselinova, A. O. Pasternak, W. De Spiegelaere, D. Vogelaers, B. Berkhout and L. Vandekerckhove, PLoS One, 2014, 9, e85999 CrossRef PubMed.
- W. Guan, L. Chen, T. D. Rane and T.-H. Wang, Sci. Rep., 2015, 5, 13795 CrossRef PubMed.
- Y. Hu, P. Xu, J. Luo, H. He and W. Du, Anal. Chem., 2017, 89, 745–750 CrossRef CAS PubMed.
- J.-u. Shim, R. T. Ranasinghe, C. A. Smith, S. M. Ibrahim, F. Hollfelder, W. T. S. Huck, D. Klenerman and C. Abell, ACS Nano, 2013, 7, 5955–5964 CrossRef CAS PubMed.
- H. N. Joensson, M. L. Samuels, E. R. Brouzes, M. Medkova, M. Uhlén, D. R. Link and H. Andersson-Svahn, Angew. Chem., Int. Ed., 2009, 48, 2518–2521 CrossRef CAS PubMed.
- E. X. Ng, M. A. Miller, T. Jing, D. A. Lauffenburger and C.-H. Chen, Lab Chip, 2015, 15, 1153–1159 RSC.
- C.-H. Chen, M. A. Miller, A. Sarkar, M. T. Beste, K. B. Isaacson, D. A. Lauffenburger, L. G. Griffith and J. Han, J. Am. Chem. Soc., 2013, 135, 1645–1648 CrossRef CAS PubMed.
- C.-H. Chen, A. Sarkar, Y.-A. Song, M. A. Miller, S. J. Kim, L. G. Griffith, D. A. Lauffenburger and J. Han, J. Am. Chem. Soc., 2011, 133, 10368–10371 CrossRef CAS PubMed.
- T. D. Rane, H. C. Zec and T.-H. Wang, Anal. Chem., 2015, 87, 1950–1956 CrossRef CAS PubMed.
- S. Jambovane, D. J. Kim, E. C. Duin, S.-K. Kim and J. W. Hong, Anal. Chem., 2011, 83, 3358–3364 CrossRef CAS PubMed.
- J. Lim, J. Vrignon, P. Gruner, C. S. Karamitros, M. Konrad and J.-C. Baret, Appl. Phys. Lett., 2013, 103, 203704 CrossRef PubMed.
- Z. Li, A. M. Leshansky, L. M. Pismen and P. Tabeling, Lab Chip, 2015, 15, 1023–1031 RSC.
- M. Najah, E. Mayot, I. P. Mahendra-Wijaya, A. D. Griffiths, S. Ladame and A. Drevelle, Anal. Chem., 2013, 85, 9807–9814 CrossRef CAS PubMed.
- S. Gu, Y. Lu, Y. Ding, L. Li, H. Song, J. Wang and Q. Wu, Biosens. Bioelectron., 2014, 55, 106–112 CrossRef CAS PubMed.
- M. Y. H. Tang and H. C. Shum, Lab Chip, 2016, 16, 4359–4365 RSC.
- Z. Han, W. Li, Y. Huang and B. Zheng, Anal. Chem., 2009, 81, 5840–5845 CrossRef CAS PubMed.
- R. Arayanarakool, L. Shui, S. W. M. Kengen, A. van den Berg and J. C. T. Eijkel, Lab Chip, 2013, 13, 1955–1962 RSC.
- F. Fachin, P. Spuhler, J. M. Martel-Foley, J. F. Edd, T. A. Barber, J. Walsh, M. Karabacak, V. Pai, M. Yu, K. Smith, H. Hwang, J. Yang, S. Shah, R. Yarmush, L. V. Sequist, S. L. Stott, S. Maheswaran, D. A. Haber, R. Kapur and M. Toner, Sci. Rep., 2017, 7, 10936 CrossRef PubMed.
- L. Zhao, C. Tang, L. Xu, Z. Zhang, X. Li, H. Hu, S. Cheng, W. Zhou, M. Huang, A. Fong, B. Liu, H.-R. Tseng, H. Gao, Y. Liu and X. Fang, Small, 2016, 12, 1072–1081 CrossRef CAS PubMed.
- W. Sheng, T. Chen, R. Kamath, X. Xiong, W. Tan and Z. H. Fan, Anal. Chem., 2012, 84, 4199–4206 CrossRef CAS PubMed.
- P. Li, Z. Mao, Z. Peng, L. Zhou, Y. Chen, P.-H. Huang, C. I. Truica, J. J. Drabick, W. S. El-Deiry, M. Dao, S. Suresh and T. J. Huang, Proc. Natl. Acad. Sci. U. S. A., 2015, 112, 4970–4975 CrossRef CAS PubMed.
- E. S. Park, C. Jin, Q. Guo, R. R. Ang, S. P. Duffy, K. Matthews, A. Azad, H. Abdi, T. Todenhöfer, J. Bazov, K. N. Chi, P. C. Black and H. Ma, Small, 2016, 12, 1909–1919 CrossRef CAS PubMed.
- M. E. Warkiani, G. Guan, K. B. Luan, W. C. Lee, A. A. S. Bhagat, P. Kant Chaudhuri, D. S.-W. Tan, W. T. Lim, S. C. Lee, P. C. Y. Chen, C. T. Lim and J. Han, Lab Chip, 2014, 14, 128–137 RSC.
- E. Sollier, D. E. Go, J. Che, D. R. Gossett, S. O'Byrne, W. M. Weaver, N. Kummer, M. Rettig, J. Goldman, N. Nickols, S. McCloskey, R. P. Kulkarni and D. Di Carlo, Lab Chip, 2014, 14, 63–77 RSC.
- S. H. Au, J. Edd, A. E. Stoddard, K. H. K. Wong, F. Fachin, S. Maheswaran, D. A. Haber, S. L. Stott, R. Kapur and M. Toner, Sci. Rep., 2017, 7, 2433 CrossRef PubMed.
- A. F. Sarioglu, N. Aceto, N. Kojic, M. C. Donaldson, M. Zeinali, B. Hamza, A. Engstrom, H. Zhu, T. K. Sundaresan, D. T. Miyamoto, X. Luo, A. Bardia, B. S. Wittner, S. Ramaswamy, T. Shioda, D. T. Ting, S. L. Stott, R. Kapur, S. Maheswaran, D. A. Haber and M. Toner, Nat. Methods, 2015, 12, 685–691 CrossRef CAS PubMed.
- M. Avram, C. M. Bălan, I. Petrescu, V. Schiopu, C. Mărculescu and A. Avram, Plasmonics, 2012, 7, 717–724 CrossRef CAS.
- S. Faez, Y. Lahini, S. Weidlich, R. F. Garmann, K. Wondraczek, M. Zeisberger, M. A. Schmidt, M. Orrit and V. N. Manoharan, ACS Nano, 2015, 9, 12349–12357 CrossRef CAS PubMed.
- T. D. Rane, H. C. Zec, C. Puleo, A. P. Lee and T.-H. Wang, Lab Chip, 2012, 12, 3341–3347 RSC.
- D.-K. Kang, M. M. Ali, K. Zhang, S. S. Huang, E. Peterson, M. A. Digman, E. Gratton and W. Zhao, Nat. Commun., 2014, 5, 5427 CrossRef CAS PubMed.
- D. Pekin, Y. Skhiri, J.-C. Baret, D. Le Corre, L. Mazutis, C. Ben Salem, F. Millot, A. El Harrak, J. B. Hutchison, J. W. Larson, D. R. Link, P. Laurent-Puig, A. D. Griffiths and V. Taly, Lab Chip, 2011, 11, 2156–2166 RSC.
- S. Mader and K. Pantel, Oncol. Res. Treat., 2017, 40, 404–408 CrossRef CAS PubMed.
- H. Schwarzenbach, D. S. B. Hoon and K. Pantel, Nat. Rev. Cancer, 2011, 11, 426–437 CrossRef CAS PubMed.
- D. J. Shin, Y. Zhang and T.-H. Wang, Microfluid. Nanofluid., 2014, 17, 425–430 CrossRef CAS PubMed.
- Y. Zhang, S. Park, S. Yang and T.-H. Wang, Biomed. Microdevices, 2010, 12, 1043–1049 CrossRef CAS PubMed.
- Q. Wu, W. Jin, C. Zhou, S. Han, W. Yang, Q. Zhu, Q. Jin and Y. Mu, Anal. Chem., 2011, 83, 3336–3342 CrossRef CAS PubMed.
- N. Rački, D. Morisset, I. Gutierrez-Aguirre and M. Ravnikar, Anal. Bioanal. Chem., 2014, 406, 661–667 CrossRef PubMed.
- S. M. Hanash, C. S. Baik and O. Kallioniemi, Nat. Rev. Clin. Oncol., 2011, 8, 142–150 CrossRef PubMed.
Footnote |
† These authors contributed equally to this work. |
|
This journal is © The Royal Society of Chemistry 2022 |
Click here to see how this site uses Cookies. View our privacy policy here.