Heterogeneous endotoxin detection bioassay using drug–nanoparticle bioconjugates: an optimization study†
Received
24th May 2017
, Accepted 25th July 2017
First published on 25th July 2017
Abstract
We present a detailed study of an endotoxin detection bioassay performed in a sandwich format on solid substrates. Self-assembled monolayers (SAMs) of C-18 alkyl silanes were immobilized on glass to heterogeneously entrap lipopolysaccharides (LPS) from human serum. The captured endotoxin was then tagged with polymyxin B sulfate–gold nanoparticle (GNP) conjugates followed by silver enhancement. The intensity of the obtained color spots were quantified using a mobile phone camera and the assay performance was optimized by studying the effects of various experimental parameters like silane concentration, temperature, humidity and incubation time. The assay gave a linear dynamic range of 104 with a lower limit of detection (LOD) at 1 pg mL−1. This LOD was further improved by an LPS pre-enrichment step prior to the assay. Rudimentary thermodynamic and kinetic models fitted to our experimental data suggest that LPS binding is an entropic process and LPS/GNP binding is mainly adsorption-controlled.
Design, System, Application
We have developed a bioassay for the detection of endotoxins or lipopolysaccharides (LPS) in human serum using a solid phase. For this, the molecular structure of LPS has been taken into consideration in order to capture the molecule from its tail end. This molecular orientation not only helps in immobilizing the molecules using simple hydrophobic interactions but also provides advantages in terms of better availability of epitopes for more efficient targeting. The entire assay has been optimized empirically in order to obtain the most suitable conditions for its best performance. We have also estimated how the free energy of interaction between the molecules depends on the length of the carbon chains in the lipid chain. Further, a simple kinetic model has been developed which shows that the binding process is mainly adsorption-controlled. This is not surprising as the LPS molecules are large (∼15 kDa) and bind in a specific orientation. The assay has great utility for the medical diagnosis of septicemia where the LPS concentration levels in blood are low (subnanomolar) especially at the onset of infection.
|
Introduction
LPS or endotoxins are components of the Gram-negative bacterial cell wall.1 They are readily shed as the cells undergo active growth or death. Each cell contains several million copies of the LPS molecule resulting in their ubiquitous presence all around our milieu, including in laboratory utensils, potable water and food.2 Our intestine also carries copious amounts of endotoxins. It is now well known that LPS molecules can enter the bloodstream through open wounds, systemic infection or intestinal leakage and cause serious inflammatory response including sepsis.3 Given the high mortality rates associated with this medical condition, the Pharmacopoeia imposes stringent limits (<0.25 EU mL−1, where 1 EU–100 pg for E. coli. LPS) on the levels of endotoxins present in certified pharmaceutical products. Similarly, international guidelines for severe sepsis and septic shock management (SSCM) highlight an unmet need for developing new technologies for efficient detection and removal of endotoxins from biofluids.4
Presently, the most widely used endotoxin detection or quantification assays (LAL tests) are based on amebocyte lysate from Limulus polyphemus or the rare horseshoe crab. Many formats of these assays exist, namely, the gel clot, turbidimetric and chromogenic, which work on the principle of liquid to solid phase transition (of the hemolymph), endogenous substrate cleavage-induced turbidity variation and a synthetic peptide–chromogen complex cleavage-induced color change, respectively.5–7 All the assays are FDA approved to measure endotoxin levels in parenteral drugs, biologicals and medical devices. However, they cannot be directly applied to biological samples because of the presence of circulating inhibitors of the coagulation/gel clot reaction. In addition, other microbial products, notably fungi, can also activate the Limulus reaction, so the assay is not specific for endotoxin. Therefore, designing an endotoxin assay that works efficiently in a complex matrix such as serum still remains a challenge.
One way to overcome the problem of serum complexity is to selectively capture and remove LPS from biological fluids using a solid phase. This approach requires an understanding of the LPS molecular structure such that site-specific binding receptors may be appropriately designed or selected for LPS targeting. For instance, one end of the LPS molecule, known as the outer O-antigen domain, consists of a polysaccharide chain that varies from species to species. This portion is the exposed region in a bacterial cell wall and thus, can be specifically targeted using antibodies. Many commercial ELISAs (enzyme linked immunosorbent assays) use this principle to immobilize LPS on their assay plates.8,9 Similarly, the middle oligosaccharide core region comprises heptose and 2-keto 3-deoxy-D-manno-octolusonic acid (KDO) sugars with phosphate moieties that can be targeted via electrostatic ligand interactions. The third portion, called the lipid A region, is the conserved domain that makes LPS highly toxic and responsible for biological activation inside a host.10,11 It comprises phosphorylated glucosamine disaccharides joined together with multiple fatty acid chains that are each 12 to 18 carbon atoms long. Therefore, lipid bilayer membranes of synthetic phospholipids mimicking the outer wall of the Gram-negative bacteria can serve as suitable supports for asymmetric capture of LPS. The many receptor–ligand based strategies reported in the literature for specific LPS binding are summarized in Table 1.
Table 1 Compilation of the various LPS–ligand binding interactions reported in the literature
Receptor |
LPS binding region |
Mode of interaction |
Ref. |
Note: DOPC: 1,2-dioleoyl-sn-glycero-3-phosphocholine, POPC: 1-palmitoyl-2-oleoyl-sn-glycero-3-phosphocholine and DSPC: 1,2-distearoyl-sn-glycero-3-phosphocholine.
|
Mono- and polyclonal antibodies |
Core (R1, R2, R3, R4 and K12) |
Biological |
8, 9, 12–14 |
|
gp9 protein on p22 bacteriophage |
O-antigen |
Biological |
15–19
|
|
Lipid binding proteins (LBPs) and mannose binding lectins (MBLs) |
(O-antigen) N-acetyl-D-glucosamine, mannose and N-acetyl-mannosamine |
Biological |
20–23
|
|
Aptamers |
Phosphate moieties (lipid A) |
Electrostatic |
24, 25 |
|
Antibiotics (PMB, bacitracin) |
Phosphate moieties (lipid A) |
Electrostatic and hydrophobic |
26–28
|
|
Antimicrobial peptides (AMPs) (cathelicidins, indolicidine, sushi tachyplesin) |
Phosphate moieties (lipid A) |
Electrostatic |
29–32
|
|
Phospholipids (DOPC, POPC, DSPC etc.)a |
Lipid A tail |
Hydrophobic |
33–35
|
|
C-18 alkyl chains |
Lipid A tail |
Hydrophobic |
36, 37 |
Our group recently demonstrated a facile strategy for entrapping endotoxins from human sera using C-18 alkyl silane SAMs on glass substrates (Fig. 1).38 The captured LPS molecules were labeled with GNPs conjugated to an antibiotic drug called polymyxin B sulfate (PMB) enabling ultrasensitive detection of endotoxins down to sub-pM levels with the naked eye within 2.5 h. PMB is known to interact electrostatically with the two negatively charged phosphate moieties in the lipid-A region of LPS via its three positively charged amine groups in the cyclic hepta-peptide ring.39,40 Its use in the GNP detection probe as a tag (say, in place of an antibody) allows improving the cost and thermostability of the assay. In the current work, we present a detailed optimization study of the various experimental parameters involved in this detection process with the aim of developing a better understanding of their role in the signal response and to create a more robust assay that can work efficiently under any clinical setting. We also report a significant improvement in the signal collection and data analysis procedure that further improves the speed of the assay. In addition, kinetic and thermodynamic models have been applied to our experimental system which have not been reported earlier. With the optimized conditions, the variability in the results has been reduced and the sensitivity enhanced.
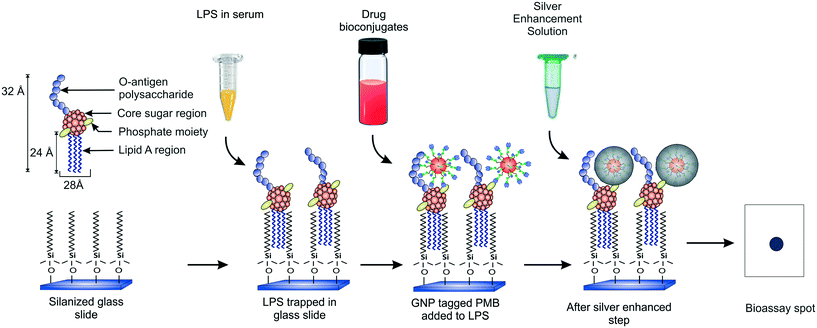 |
| Fig. 1 Schematic of the protocol used for endotoxin detection. | |
Experimental section
Materials
Gold(III) chloride trihydrate (HAuCl4·3H2O), sodium citrate dihydrate (C6H5Na3O7·2H2O), tannic acid (C76H62O48), PMB (M.W. 1385.61 Da), trichloro (octadecyl) silane (OTS), toluene, glutaraldehyde (GLA), hydrogen peroxide (H2O2), chloroform, phenol, acetone, ammonia, purified LPS (M.W. ∼15 kDa) extract from E. coli 055:B5, and silver enhancement kit (Sigma Aldrich, India); dithiol alkane aromatic PEG6 hydrazide (DTH) (Sensopath Technologies, USA); microscope glass slides (Blue Star, India); pyrogen-free water (Hyglos GmbH, Germany); ultrapure deionized (DI) water of resistivity ∼18 MΩ cm (Millipore, India). The DI water was further autoclaved at 15 psi for 30 min and subsequently filtered through 0.22 μm PVDF membrane filters (Millipore, India) prior to all experiments. All chemicals used were of analytical grade.
Preparation of PMB-conjugated GNPs
Citrate-stabilized GNPs of size 16 ± 4 nm (Fig. S1†) were prepared by diluting 10 mL of 1% (w/v) HAuCl4 solution with 800 mL of ultrapure DI water.41 Another solution containing 40 mL of 1% (w/v) sodium citrate and 100 μL of 0.1% (w/v) tannic acid was diluted to 200 mL. Both solutions were heated to 60 °C and then mixed under continuous stirring for 4 h. The mixture was taken off heat after the first appearance of a wine red color and immediately quenched in an ice bath. 10 mL of this 2 nM GNP suspension was incubated with 10
000× molar excess of DTH for 6 h at room temperature (∼25 °C) with constant stirring in a shaker incubator (Orbitek, India). The particles were then washed thrice with 40 mM HEPES at pH 7.4 at 8690, 11
175 and 14
900 rcf for 20 min each, respectively, to remove excess DTH. The final pellet was resuspended in 20 mL of 2.5% (v/v) GLA solution in HEPES buffer and incubated overnight with constant stirring at room temperature. The amine-reactive NP conjugates were centrifuged the next day using the settings mentioned above and the final pellet was resuspended in 8 mL of 20 nM PMB in HEPES followed by 16 h of incubation with constant stirring. The unreacted aldehyde groups were passivated with 0.05 mL of 20 mM glycine in HEPES to minimize nonspecific binding. The final conjugate was washed and resuspended in 6 mL of 40 mM HEPES buffer at pH 7.4.38 The particles were stored at 4 °C until further use.
Preparation of silanized glass slides
Regular glass microscope slides were cleaned with acetone and dipped in a basic piranha solution of 1
:
1
:
5
:
H2O2
:
NH3
:
H2O (v/v) for 1 h at 70 °C. The slides were then washed extensively with DI water and dried under a purge of nitrogen. The dried glass slides were then dipped in 10 mM OTS solution prepared in toluene and incubated at 60 °C for at least 14 h.42 The slides were finally washed with excess toluene to remove any unbound OTS and stored in a desiccator until further use. The entire silanization protocol was performed in a clean and dry environment in the presence of nitrogen gas to avoid silane self-polymerization.42,43
Preparation of LPS samples
1 mg of lyophilized LPS extract was reconstituted in 1 mL of pyrogen-free DI water inside pyrogen-free borosilicate glass vials. This stock was vortexed vigorously for 30 min followed by 15 min of sonication and then diluted to lower concentrations in pyrogen-free DI water. 10 μL from each of these dilutions was spiked into 90 μL of endotoxin-free serum to obtain the final analyte solutions. Strict safety guidelines were followed at all times while handling LPS.
Bioassay procedure
10 μL of analyte was drop cast on a silanized glass slide using a micropipette and kept inside a closed water bath system (Equitron) at 37 °C to provide a humid environment during the immobilization step. The slide was washed with DI water and flooded with a suspension of 20 nM PMB–GNP conjugates and again kept inside the humid chamber. The unbound NPs were washed with DI water and the slide was set aside at room temperature for 10 min to remove moisture. Finally, a silver enhancement step was carried out wherein two freshly prepared solutions, one containing a silver salt and another a reducing agent, were mixed as instructed in the kit and smeared over the LPS spot for ∼30 s. The slides were then washed with copious amounts of ultrapure DI water to obtain dark metallic silver spots wherever the LPS was immobilized.
Signal quantification
The slides were illuminated in the dark from the top (∼1 ft) using a 40 μW soft white LED and simultaneously imaged using a 4S iPhone camera in no flash, manual mode. The angle, distance and intensity of the light source were empirically set to eliminate backscatter and to obtain uniform images. The acquired images were then cropped in MS-Paint/Photoshop-CS6 and analyzed with an image processing code written in MATLAB (R2015b). The final signals were displayed as normalized intensities ± 1 standard deviation (SD) from twelve different data points obtained from three different glass slides containing four spots each (see the ESI† for details).
Chemical pre-concentration protocol
LPS was enriched using a hot phenol–chloroform extraction protocol reported in the literature.44,45 For this, 300 μL of 100 fg mL−1 LPS in serum was mixed with an equal volume of 1
:
1 v/v chloroform–phenol mixture inside an eppendorf tube. The tube was vigorously vortexed for 20 s followed by 5 min of incubation at 65 °C. The sample was centrifuged at 1660 rcf for 10 min and the aqueous supernatant (∼300 μL) was pipetted out carefully into three equal fractions (top: SN1, middle: SN2 and lower: SN3) avoiding the inclusion of the white precipitate and chloroform layer at the bottom of the tube.
Results and discussion
The endotoxin bioassay was performed in three main steps (Fig. 1). First, the glass slide was functionalized with OTS in order to capture LPS from the analyte. Next, the captured LPS was tagged with PMB-conjugated GNPs. Finally, the GNPs were silver enhanced for further signal amplification. The average number of PMB molecules was estimated to be around ten per particle.38 All the negative and positive controls performed are summarized in Table S1.†
Thermodynamic analysis of LPS capture using SAMs
We believe that the LPS-alkane chain interaction is an entropically-driven process in which the excluded volume is minimized by maximizing the surface area overlap between the lipid A tails and the C-18 carbon chains. This is supported by the fact that several other untreated surfaces like Teflon, polyethylene, polypropylene, Parafilm® M and polydimethylsiloxane (PDMS) with similar macroscopic hydrophobicities (contact angles) failed to show any LPS binding response. Also, gentle washing seemed to play a key role in obtaining reproducible results as hydrophobic interactions are known to be weak in nature. The overall Gibbs free energy of the system was estimated using eqn (1) which states that the free energy required for transferring a single molecule from the bulk to the surface is opposed by the loss of its translational and rotational entropy due to association.46 Considering that the receptor molecules are fixed in our case (see Fig. 1), we only included the contributions from the analyte.
| ΔGT = (ΔGF) × (A) × (n) + (kBT) × ln(Vf/Vb). | (1) |
Here, ΔGF is the transfer free energy per unit area of the hydrocarbon, A is the area of one C-18 chain, n is the number of chains per LPS molecule, kB is the Boltzmann constant, T is the temperature, and Vb and Vf are the bound and free volumes, respectively. Now, A = π(r2 + 2rl), where r is the axial cross-sectional radius of the carbon chain (∼0.26 nm) and l is length of the carbon chain interacting with one alkyl silane chain. Further, l = 0.154 + 0.1265m, where m is the number of C atoms per LPS chain. Taking Vf = 10 μL (analyte volume taken for the assay), Vb = 1.71 × 10−15 m3 (i.e., disk volume with height l = 2.4 nm × base area of the micro droplet with 1 mm diameter), T = 298 K, ΔGF = −41 mJ m−2, A = 4.17 nm2 and n = 4, ΔGT was computed to be −6.2 × 10−19 J (per LPS molecule). In other words, LPS binding is a thermodynamically feasible process. Interestingly, ΔGT increases with the decrease in carbon chain length and becomes zero below C-6. This trend was also observed experimentally using C-12 alkyl silanes (data not shown). Thus, this simple thermodynamic model suggests that the surface area overlap between the hydrophobic surface and the endotoxin molecules is crucial and leads to an orientational binding of LPS molecules over the surface. This feature additionally allows targeting of the phosphate groups in the conserved region and thus, a common detection strategy for all LPS molecules across different bacterial strains.
Effect of experimental conditions
Since the above analysis shows that the surface plays a crucial role in our bioassay, one of the first parameters we studied was the effect of the receptor site concentration on LPS entrapment. For this, the substrates were functionalized with different bulk OTS concentrations (varying over six orders of magnitude) and experiments were performed using varying amounts of the analyte. All other parameters including the analyte incubation time (∼20 min) were kept fixed throughout these experiments. The results obtained showed that for 10 nM silane, the signal remained more or less constant across the different LPS concentrations used but as the silane concentration was increased to 10 μM and 10 mM, the spots progressively became darker and this happened in an LPS-concentration dependent manner (Fig. 2a).
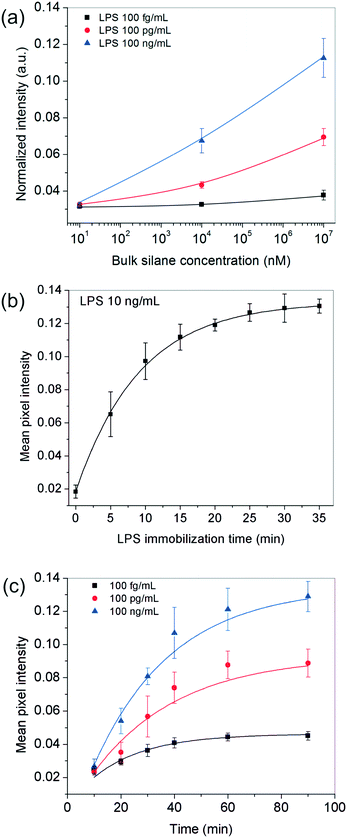 |
| Fig. 2 Optimization of bioassay parameters. (a) Effect of silane concentration: darker spots were obtained as a function of higher silane and LPS concentrations. The results are depicted at 85% relative humidity and 20 min of LPS and 40 min of GNP incubation times. (b) Effect of LPS incubation time: the surface of 10 mM silane reached saturation after 20 min when a 10 ng mL−1 LPS solution was used. (c) Effect of GNP incubation time: the surface saturation time depended on the LPS concentration used and was at least 40 min. The LPS incubation time was fixed to 20 min in all cases. | |
These results suggested that at higher silane concentrations, the process was either operating away from equilibrium as in a kinetically-controlled process (i.e., the total time given for LPS immobilization was insufficient) or there were not enough LPS molecules present in the bulk to saturate all the silane receptor sites. To confirm this, we performed another set of experiments in which the incubation time of LPS was systematically varied up to 35 min for a fixed silane and LPS concentration in the range of interest. The results showed that an LPS incubation time of 20 min was sufficient (>90% of the saturation value or 2τ) to get a reasonably stable spot intensity (Fig. 2b), further implying that it was the silane receptors that were in excess and the LPS binding time was not a limiting factor.
Next, we looked into the effect of GNP incubation time. As the contact time for NPs was increased, the final spot intensities came out darker and eventually saturated (Fig. 2c). The saturation time increased with the number of underlying receptor sites for gold (LPS in this case), suggesting that this was most likely an adsorption-controlled process. For instance, 40 min were sufficient to saturate 100 fg mL−1 of LPS, whereas this time was found to be >60 min in the case of 100 ng ml−1 of LPS and more. Since the sensitivity of the assay generally comes at the cost of its running time, one may choose the operating conditions appropriately depending on their process requirement.
In addition to the above experimental conditions, temperature and humidity were also expected to impact the assay performance significantly. Higher temperatures were anticipated to speed up the process kinetics, whereas a higher relative humidity was expected to minimize the rate of evaporation of the sessile serum droplet in which the reaction took place. Minimizing solvent loss was important for many reasons – (i) to avoid change in “reactor” volume (ii) to prevent generation of convective currents that would make the process unsteady and difficult to model and, (iii) to preclude any changes in viscosity and ultimately cake formation by the proteins present in serum. Since in general, temperature and relative humidity vary inversely for a fixed water content in the air, all our experiments were performed inside a humidifying chamber maintained at a high relative humidity of 85% at 37 °C. When the relative humidity was reduced to ∼46% by carrying out the incubation steps inside a 37 °C incubator instead, the bioassay indeed showed a discernibly poor performance, especially at higher LPS concentrations (Fig. 3b). Similarly, when the bioassays were performed below 37 °C, the spot intensities also decreased as expected (Fig. 3a).
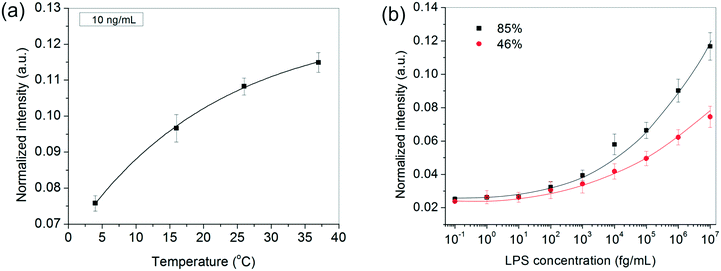 |
| Fig. 3 The effect of (a) temperature and (b) humidity on the bioassay performance. The results in (a) are depicted for 10 ng mL−1 LPS and the curve is fitted using the Arrhenius equation. In (b), the lines are drawn as a guide to the eye. In both (a) and (b), the GNP concentration was fixed at 1 nM and the LPS and GNP incubation times were 20 and 40 min, respectively. | |
After developing a fairly good understanding of the process parameters, finally a standard calibration curve was prepared for the LPS concentrations using the optimized experimental conditions (Fig. 4a). The dynamic range of the assay was seen to vary over four orders of magnitude and 1 pg mL−1 was found to be the lowest LOD. These values lie right in the clinically relevant range for correlating to the severity of sepsis.47 The assay also gave an equally good performance in aqueous samples (data not shown).
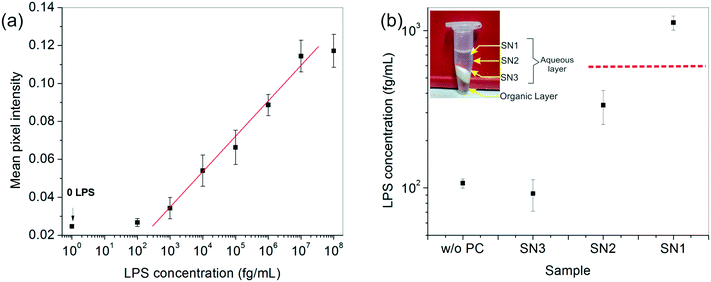 |
| Fig. 4 (a) The calibration curve obtained for LPS detection under the best operating assay conditions, resulting in a linear dynamic range of 1 pg mL−1 to 10 ng mL−1. The ‘0’ LPS point corresponds to plain serum without any spiked LPS (negative control). (b) Chemical enrichment helped improve the lower LOD of the assay by an order of magnitude allowing undetectable LPS (data point marked w/o PC) to be easily detected (data point marked SN1). Here, ( ) indicates the lower LOD of the assay obtained from the graph in (a). The inset shows the different fractions obtained after centrifugation. | |
Chemical enrichment of LPS
To enhance the LOD of our assay even further, we undertook additional experiments to enrich the LPS in the spiked serum prior to performing the assay. Among the many options available, a chemical treatment method, particularly the hot phenol–chloroform extraction technique, was found to be the most suitable due to its simplicity of operation. Upon centrifugation, the ternary phenol–chloroform–serum mixture separated, yielding an upper aqueous phase containing the LPS, a middle white dense layer containing a mixture of cell debris, proteins and other cellular components, and a lower clear chloroform–phenol organic phase (Fig. 4b). The top aqueous layer was further divided into three fractions: SN1, SN2 and SN3, and the bioassay was carried out on each of these portions separately.
The results showed an interesting trend. A clear upward concentration gradient was observed as we moved away from the water–debris interface towards air (i.e., from SN3 to SN1) (Fig. 4b). This was not entirely unexpected given the amphiphilic disposition of the LPS molecules to stay near the hydrophobic–hydrophilic interface. Consequently, SN3 was slightly depleted of LPS as compared to the original sample. Something, however, that could not be explained by mass conservation was the slight increase in the overall LPS amount in the system (obtained by averaging the concentrations of the three fractions). We attributed this variation to the change in the matrix composition and viscosity upon removal of the proteins from the serum which may lead to faster binding kinetics and hence, a higher signal.
The absolute amount of LPS in SN1 was estimated to be ∼1 pg mL−1 from the calibration curve, which was an order of magnitude higher than the original sample, whereas the SN2 and SN3 fractions lay below the detectable limit. Although this pre-enrichment approach adds an additional step to our bioassay, it may be used as a valuable option in cases where the LPS concentration in clinical samples poses a limitation to its diagnostic utility.
Kinetic analysis of molecular binding
The binding of molecules on a surface can be mainly divided into two steps – (i) their molecular diffusion from the bulk to the surface and (ii) their adsorption on the surface receptor sites. At steady state, the rates of these two steps are equal, i.e., radsoption = rdiffusion but either may be rate-limiting. The rate of diffusion is given by Fick's 1st law (eqn (2)), | 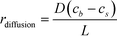 | (2) |
where D (cm2 s−1) is the molecular diffusivity in solution, L (cm) is the average distance traveled by the molecule from the bulk to the surface, and cb (molecules cm−3) and cs (molecules cm−3) are the bulk and surface molecular concentrations, respectively. Similarly, the rate of adsorption can be written as (eqn (3))where k (cm3 molecules−1 s−1) is the adsorption rate constant and cv (molecules cm−3) is the surface concentration of vacant sites.
For the analysis of GNP binding, we assumed the GNP
:
LPS binding stoichiometry to be 1
:
1 and the spot intensity I to vary linearly with the bound GNP concentration at any time.48 This gave us the relation (eqn (4))
|  | (4) |
Here, I0 is the highest intensity (maximum darkness) achieved and ct is the total number of binding sites at the beginning. In the case of the adsorption-limited process, the concentration of molecules near the surface matches that of the bulk (cs = cb) as the molecules cannot get adsorbed that quickly and get accumulated. In other words (eqn (5)),
| I = I0(1 − exp(−k*cb*t)). | (5) |
Conversely, if the process is diffusion-controlled, the molecules near the surface bind instantaneously (cs = 0) giving the final form of the equation as (eqn (6))
| 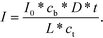 | (6) |
When we modeled the data in Fig. 2b using both eqn (5) and (6), the data fitted better using the adsorption-controlled process (coefficient of determination with the regression value R2 = 0.99) giving a k value of 0.21 nM−1 min−1. Similarly, the data in Fig. 2c also fitted more effectively assuming the rate to be adsorption-limited (R2 > 0.96 for all the 3 cases), but it was not possible to fit all the experimental data with a single rate constant. The value of k decreased from 0.040 nM−1 min−1 to 0.028 nM−1 min−1 to 0.026 nM−1 min−1 as the LPS concentration was raised from 100 fg mL−1 to 100 pg mL−1 to 100 ng mL−1. This lowering of effective rate constants was linked to the steric hindrance produced at a higher receptor packing density, due to which GNPs take more time to orient and find a more favorable configuration for binding.
Based on the literature, the values of the rate constants obtained for LPS and GNP binding seemed fairly reasonable but to further confirm that our model was indeed a true fit, we looked into the effect of temperature on our rate constants. The results showed an exponential dependence of k on temperature (see Fig. 3a), as predicted by the Arrhenius law which confirmed that our model was correct and the binding was dominated by surface reaction kinetics. On the other hand, the dependence of signal intensity on temperature assuming the process to be diffusion-controlled did not show any correlation at all (see the ESI†), thus supporting our claims. The relation between diffusion rate and temperature was obtained from the Stokes–Einstein equation which states that
, where μ is the viscosity of the solution and r is the molecular radius.49 Since viscosity follows the relation μ = μ0*10b/T, D varies directly proportional to T*10−b/T.
Conclusions
We have demonstrated a detailed optimization study of a surface endotoxin bioassay. Developing a simple assay for the detection of LPS in biological fluids has been a challenge so far for various reasons, the most important among these being the inhibition observed with biological samples. An antibiotic, too, has never been used as an affinity ligand for diagnostic applications. We have illustrated for the first time that LPS can be detected with a very high level of sensitivity and reliability in biological samples like the human serum using a simple PMB–GNP drug bioconjugate as an affinity ligand. The kinetics of the LPS and gold molecular binding events were found to be adsorption-rate limited. The lowest LPS detected was 1 pg mL−1 and this limit could be enhanced 10× by including a pre-treatment step at the beginning of the assay. The dynamic range of the signal varied linearly over four orders of magnitude and the performance of the assay improved with higher silane loading, humidity and temperature in the range of LPS concentrations studied. These performance characteristics of the technique make it a viable option for sepsis management in a clinical setting. Other potential applications include pharmaceutical product testing, quality control of foods and environmental water checks.
Funding sources
Biotechnology Innovations and Research Activity Council (BIRAC) and Council of Scientific and Industrial Research (CSIR), New Delhi.
Notes
No competing financial interest.
Acknowledgements
This work was supported by the Biotech Ignition Grant (BIG) from Biotechnology Innovations and Research Activity Council (BIRAC). PK acknowledges a Senior Research Fellowship provided by the Council of Scientific and Industrial Research (CSIR), New Delhi. SG thanks Gaurav Goel (IITD) for useful discussions.
References
- Y. Rosenfeld, N. Papo and Y. Shai, J. Biol. Chem., 2006, 281, 1636–1643 CrossRef CAS PubMed
.
- M. B. Gorbet and M. V. Sefton, Biomaterials, 2005, 26, 6811–6817 CrossRef CAS PubMed
.
- J. Cohen, Nature, 2002, 420, 885–891 CrossRef CAS PubMed
.
- M. Singer, C. S. Deutschman, C. W. Seymour, M. Shankar-Hari, D. Annane, M. Bauer, R. Bellomo, G. R. Bernard, J.-D. Chiche, C. M. Coopersmith, R. S. Hotchkiss, M. M. Levy, J. C. Marshall, G. S. Martin, S. M. Opal, G. D. Rubenfeld, T. van der Poll, J. Vincent and D. C. Angus, JAMA, 2016, 315, 801–810 CrossRef CAS PubMed
.
- A. P. Das, P. S. Kumar and S. Swain, Biosens. Bioelectron., 2014, 51, 62–75 CrossRef CAS PubMed
.
- Bacterial Endotoxins/Pyrogens. U.S. Food drug adminstration 2015, https://www.fda.gov/iceci/inspections/inspectionguides/inspectiontechnicalguides/ucm072918.htm (Accessed May 1, 2017)
.
- D. W. Bates, J. Parsonnet, P. A. Ketchum, E. B. Miller, T. J. Novitsky, K. Sands, P. L. Hibberd, P. S. Graman, P. N. Lanken, J. S. Schwartz, K. Kahn, D. R. Snydman, R. Moore and E. Black, Clin. Infect. Dis., 1998, 27, 582–591 CrossRef CAS PubMed
.
- D. Nelson, W. Neill and I. R. Poxton, J. Immunol. Methods, 1990, 133, 227–233 CrossRef CAS PubMed
.
- N. C. Gagliardi, J. P. Nolan, D. M. Feind and M. Delissio, J. Immunol. Methods, 1986, 91, 243–247 CrossRef CAS PubMed
.
- K. Takayamasb, N. Qureshis, P. Mascagnilii, L. Andersonll, C. R. H. Raetzliss, M. Wisconsin and C. R. H. J. B. Chern, J. Biol. Chem., 1983, 258, 14245–14252 Search PubMed
.
- P. Li, M. Sun, T. Wohland, B. Ho and J. L. Ding, Cell. Mol. Immunol., 2006, 3, 21–28 CAS
.
- J. J. Kroll, M. A. Eichmeyer, M. L. Schaeffer, S. McOrist, D. L. Harris and M. B. Roof, Clin. Diagn. Lab. Immunol., 2005, 12, 693–699 CAS
.
- R. J. Gibbs, J. Stewart and I. R. Poxton, J. Med. Microbiol., 2004, 53, 959–964 CrossRef CAS PubMed
.
- W. C. Bogard Jr., D. L. Dunn, K. Abernethy, C. Kilgarriff and P. C. Kung, Infect. Immun., 1987, 55, 899–908 CAS
.
- H. Handa, S. Gurczynski, M. P. Jackson and G. Mao, Langmuir, 2010, 26, 12095–12103 CrossRef CAS PubMed
.
- F. Yu, H. Yamada and S. Mizushima, J. Bacteriol., 1981, 148, 712–715 CAS
.
- D. V. Rakhuba, E. I. Kolomiets, E. Szwajcer Dey and G. I. Novik, Pol. J. Microbiol., 2010, 59, 145–155 CAS
.
- H. Shin, J. H. Lee, H. Kim, Y. Choi, S. Heu and S. Ryu, PLoS One, 2012, 7, 1–11 Search PubMed
.
- A. Washizaki, T. Yonesaki and Y. Otsuka, Microbiology, 2016, 5, 1003–1015 CAS
.
- J. H. Kang, M. Super, C. W. Yung, R. M. Cooper, K. Domansky, A. R. Graveline, T. Mammoto, J. B. Berthet, H. Tobin, M. J. Cartwright, A. L. Watters, M. Rottman, A. Waterhouse, A. Mammoto, N. Gamini, M. J. Rodas, A. Kole, A. Jiang, T. M. Valentin, A. Diaz, K. Takahashi and D. E. Ingber, Nat. Med., 2014, 20, 1211–1216 CrossRef CAS PubMed
.
- G. A. Esparza, A. Teghanemt, D. Zhang, T. L. Gioannini and J. P. Weiss, Innate Immun., 2012, 18, 478–491 CrossRef CAS PubMed
.
- M. I. Lassenius, P. J. Pussinen, C. Forsblom, P. H. Groop and M. Lehto, Diabetes Care, 2011, 34, 1809–1815 CrossRef CAS PubMed
.
- M. D. L. Oliveira, C. A. S. Andrade, M. T. S. Correia, L. C. B. B. Coelho, P. R. Singh and X. Zeng, J. Colloid Interface Sci., 2011, 362, 194–201 CrossRef CAS PubMed
.
- W. Su, M. Lin, H. Lee, M. Cho, W. S. Choe and Y. Lee, Biosens. Bioelectron., 2012, 32, 32–36 CrossRef CAS PubMed
.
- S. E. Kim, W. Su, M. Cho, Y. Lee and W. S. Choe, Anal. Biochem., 2012, 424, 12–20 CrossRef CAS PubMed
.
- D. C. Morrison and D. M. Jacobs, Immunochemistry, 1976, 13, 813–818 CrossRef CAS PubMed
.
- J. Mares, S. Kumaran, M. Gobbo and O. Zerbe, J. Biol. Chem., 2009, 284, 11498–11506 CrossRef CAS PubMed
.
- S. Srimal, N. Surolia, S. Balasubramanian and A. Surolia, Biochem. J., 1996, 315, 679–686 CrossRef CAS PubMed
.
- Y. Sun and D. Shang, Mediators Inflammation, 2015, 2015, 1–8 CrossRef PubMed
.
- D. Pulido, M. V. Nogús, E. Boix and M. Torrent, J. Innate Immun., 2012, 4, 327–336 CrossRef CAS PubMed
.
- A. Datta, A. Ghosh, C. Airoldi, P. Sperandeo, K. H. Mroue, J. Jiménez-Barbero, P. Kundu, A. Ramamoorthy and A. Bhunia, Sci. Rep., 2015, 5, 11951 CrossRef PubMed
.
- S. Bera, A. Ghosh, S. Sharma, T. Debnath, B. Giri and A. Bhunia, J. Colloid Interface Sci., 2015, 452, 148–159 CrossRef CAS PubMed
.
- L. A. Clifton, M. W. Skoda, E. L. Daulton, A. V. Hughes, A. P. Le Brun, J. H. Lakey and S. A. Holt, J. R. Soc., Interface, 2013, 10, 20130810 CrossRef PubMed
.
- P. G. Adams, K. L. Swingle, W. F. Paxton, J. J. Nogan, L. R. Stromberg, M. A. Firestone, H. Mukundan and G. A. Montaño, Sci. Rep., 2015, 5, 10331 CrossRef PubMed
.
- C. Y. Hsia, L. Chen, R. R. Singh, M. P. DeLisa and S. Daniel, Sci. Rep., 2016, 6, 32715 CrossRef CAS PubMed
.
- M. M. Domingues, R. G. Inácio, J. M. Raimundo, M. Martins, M. A. R. B. Castanho and N. C. Santos, Biopolymers, 2012, 98, 338–344 CrossRef CAS PubMed
.
- M. Piazza, M. Colombo, I. Zanoni, F. Granucci, P. Tortora, J. Weiss, T. Gioannini, D. Prosperi and F. Peri, Angew. Chem., Int. Ed., 2011, 50, 622–626 CrossRef CAS PubMed
.
- P. Kalita, A. Dasgupta, V. Sritharan and S. Gupta, Anal. Chem., 2015, 87, 11007–11012 CrossRef CAS PubMed
.
- Y. Sun and D. Shang, Mediators Inflammation, 2015, 2015, 1–8 CrossRef PubMed
.
- C. J. Thomas, J. Biol. Chem., 1999, 274, 29624–29627 CrossRef CAS PubMed
.
- H. J. Slot and J. W. Geuze, Eur. J. Cell Biol., 1985, 10, 5–6 Search PubMed
.
- M. E. Mcgovern, K. M. R. Kallury and M. Thompson, Langmuir, 1994, 3607–3614 CrossRef CAS
.
- A. Ulman, Chem. Rev., 1996, 96, 1533–1554 CrossRef CAS PubMed
.
- A. Tirsoaga, A. Novikov, M. Adib-Conquy, C. Werts, C. Fitting, J. M. Cavaillon and M. Caroff, Appl. Environ. Microbiol., 2007, 73, 1803–1808 CrossRef CAS PubMed
.
- S. Rezania, N. Amirmozaffari, B. Tabarraei, M. Jeddi-Tehrani, O. Zarei, R. Alizadeh, F. Masjedian and A. H. Zarnani, Avicenna J. Med. Biotechnol., 2011, 3, 3–9 CAS
.
- N. Ben-Tal, B. Honig, C. K. Bagdassarian and A. Ben-Shaul, Biophys. J., 2000, 79, 1180–1187 CrossRef CAS PubMed
.
- P. Kalita, L. M. Chaturvedulla, V. Sritharan and S. Gupta, Nanomedicine, 2017, 13, 1–8 CrossRef PubMed
.
- S. Gupta, S. Huda, P. K. Kilpatrick and O. D. Velev, Anal. Chem., 2012, 79, 3810–3820 CrossRef PubMed
.
- M. E. Young, Biotechnol. Bioeng., 1980, XXII, 947–955 CrossRef
.
Footnote |
† Electronic supplementary information (ESI) available. See DOI: 10.1039/c7me00037e |
|
This journal is © The Royal Society of Chemistry 2017 |
Click here to see how this site uses Cookies. View our privacy policy here.