DOI:
10.1039/D3AN01466E
(Critical Review)
Analyst, 2024,
149, 46-58
Surface-enhanced Raman spectroscopy as a powerful method for the analysis of Chinese herbal medicines
Received
25th August 2023
, Accepted 1st November 2023
First published on 2nd November 2023
Abstract
Chinese herbal medicines (CHMs) derived from nature have received increasing attention and become more popular. Due to their diverse production processes, complex ingredients, and different storage conditions, it is highly desirable to develop simple, rapid, efficient and trace detection methods to ensure the drug quality. Surface-enhanced Raman spectroscopy has the advantages of being time-saving, non-destructive, usable in aqueous environments, and highly compatible with various biomolecular samples, providing a promising analytical method for CHM. In this review, we outline the major advances in the application of SERS to the identification of raw materials, detection of bioactive constituents, characterization of adulterants, and detection of contaminants. This clearly shows that SERS has strong potential in the quality control of CHM, which greatly promotes the modernization of CHM.
1. Introduction
Chinese herbal medicines (CHMs), extracted from natural plants, minerals and animal substances, are gaining increasing attention worldwide.1–3 Driven by domestic and international demands, WHO is also developing benchmarking documents for training and practice of CHMs, to support their development.4 In the past two decades, the field of CHMs has made great progress in modern research such as clinical trial evaluation,5 pharmaco-logical mechanisms,6 and new drug discovery.7 However, there are still great challenges in translating the experience-based CHM to evidence-based medicine, promoting its modernization and worldwide acceptance. Due to the diverse ingredients, rich varieties, and complex sources of CHMs, it is highly desirable to develop a fast and effective modern analysis technique to identify its constituents and detect bioactive constituents for the quality control and fundamental understanding of pharmacology/pharmaceutics.
At present, a large number of techniques are being used for the detection of drugs including CHMs, such as nuclear magnetic resonance (NMR), mass spectrometry (MS), combined gas chromatography/mass spectrometry (GC-MS), liquid chromatography,8,9 gas chromatography10,11 and high-performance liquid chromatography.12,13 They provide fingerprint information of the constituents and active pharmaceutical ingredients with high reliability and accuracy. Despite their high molecular specificity, the aforementioned analytical methods are often centralized and require complicated pretreatment, extensive sample preparation, trained personnel, and time-consuming processes, which greatly limit their practical application in on-site and in situ drug testing or routine use in small publicly funded institutions.
Alternatively, vibrational spectroscopy can simply, quickly, and non-invasively provide rich information about the molecular structure, conformation, intermolecular interactions, and chemical bonding. Among them, Raman spectroscopy has the advantages of ultra-high surface sensitivity, no interference from water, no requirement of staining and labeling,14,15etc. It provides an effective quality control method in the pharmaceutical industry. So far, different types of Raman spectroscopic techniques, including dispersive Raman spectroscopy,16,17 resonance Raman spectroscopy,18 Fourier transform Raman spectroscopy,19,20 and confocal/microscopic Raman spectroscopy,21,22 have been used to detect the chemical constituents of herbal medicines effectively and accurately without the complicated separation and extraction procedures. However, the cross-section of Raman scattering is as low as ca. 10−31–10−29 cm2 per molecule. This heavily limits the application of conventional Raman spectroscopy in the detection and identification of many drugs. For example, fentanyl and its analogues are typically present at less than 5.0 wt% of the total sample and therefore cannot be detected by conventional Raman spectroscopy.23 This shortcoming can be overcome with SERS, which can greatly improve the sensitivity of Raman spectroscopy to the single-molecule level and overcome the strong fluorescent background of chromophores in plant samples,24 which is crucial in the analysis of herbal medicines.
Recently, SERS have been proven to be a promising technique for a simple, rapid, low-cost, and nondestructive analysis in the quality control and safety assessment of CHMs.25,26 In this review, we first briefly introduce the working principle of SERS. Next, we discuss the use of SERS to analyse CHMs from the following aspects: raw material identification; bioactive constituent detection; adulterant characterization; and contamination detection. Finally, we briefly summarize the challenges and prospects in this field.
2. Working principles of surface enhanced Raman spectroscopy
Surface-enhanced Raman spectroscopy (SERS) is a nondestructive and ultrasensitive method that has become a powerful analysis method since its discovery in the 1970s.27–29 It has been extensively used to gather rich structural information on molecules adsorbed on SERS-active substrates under various conditions. Typically, the SERS-active substrates are coinage-metal nanostructures, such as roughened Au and Ag electrodes, colloidal aggregates, and microlithographic particles. As schematically illustrated in Fig. 1A, the free electrons in these metal nanomaterials can be coherently excited by incident light to oscillate collectively at metal/dielectric interfaces, which is called surface plasmon resonance (SPR) and can greatly enhance the electromagnetic field strength near the surface.29 Benefiting from the rapid development of nanoscience, great progress has been made in the field of SERS based on highly sensitive nanoparticles, enabling SERS to realize single-molecule detection.29–32
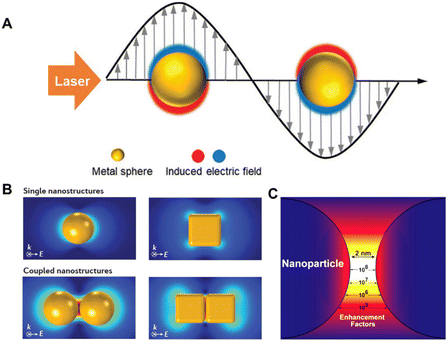 |
| Fig. 1 (A) Schematic diagram of localized surface plasmon resonance (LSPR) of metal nanoparticles irradiated with a laser. (B) Finite-element simulations of SERS-enhancement distribution in typical Au nanoparticles. (C) The calculated and quantitative SERS enhancement factor distribution in the nanogap between dimer nanoparticles. Reprinted with permission from ref. 29–31. Copyright 2021 Wiley and 2016 Nature publishing group and 2015 Elsevier. | |
The local SPR response and local electromagnetic field distribution depend not only on the shape and size of these plasmonic nanostructures but also on the interparticle gap.30,31Fig. 1B shows the finite element simulation of the SERS enhancement distribution in 60 nm Au nanospheres and cubes.30 Typically, the SERS enhancement of coupled dimer nanoparticles is two to four orders of magnitude greater than that of monomers. It is worth pointing out that the size of the SERS hotspot generated by coupled nanostructures is very small, about 1–5 nm, and the Raman signal of the probe molecule located at the hotspot contributes more than 50% of the total Raman signal (Fig. 1C). These pose a great challenge for the quantitative detection of SERS. For example, the SERS intensities can vary by several orders of magnitude, ca. from 2.8 × 104 to >1 × 1010 reported on a silver nanosphere array.32
In order to solve the above problems, the past decades have focused on improving the sensitivity and reproducibility of SERS detection, such as fabricating uniform substrates with abundant hotspots, designing nanostructure assemblies, and using SERS active cores with internal standards.33–35 This enables excellent reproducibility and high sensitivity for quantitative SERS detection.
3. Raw material identification
Typically, CHMs are obtained from a wide range of natural plants, minerals and animal substances. Throughout their long history, qualitative identification of these raw materials has traditionally been based on morphological characteristics such as colour, shape, or smell. To realize the modernization of CHMs, the raw materials must first be certified, which requires a high-throughput screening method to identify and collect their chemical information.36–39 Raman spectroscopy has the characteristics of high chemical specificity and rapid analysis. So far, various Raman techniques have been successfully applied in the qualitative and quantitative analyses of CHM raw materials to establish the corresponding Raman spectrum database, such as distinguishing geographical origins of Ginseng,16Lycium barbarum40 and Radix astragali,41 probing the protein structures of various yam species20 and collecting the chemical fingerprint information of Gynostemma pentaphyllum,42Radix sanguisorbae43 and Aconiti kusnezoffii and cinnabar.44 In particular, the machine learning algorithms like principal component analysis followed by linear discriminant analysis (PCA-LDA) greatly improve the analytical performance of Raman spectrum classification in CHM analysis.
Compared with normal Raman spectroscopy, SERS has great advantages of ultrahigh sensitivity and overcoming the fluorescent background. For example, Chen et al. compared the Ag nanoparticle-based SERS spectrum of the Atractylodis Macrocephalae Rhizoma’ piece (AMRP) to its normal spectrum.45 As shown in Fig. 2A, the SERS spectrum of AMRP can show clearly not only the main Raman bands similar to the weak normal Raman spectra, but also intense bands at 619, 733, 958, 1324, 1395 and 1469 cm−1. In general, the characteristic peaks at 555, 619, and 1632 cm−1 belong to proteins, while the band at 1112 cm−1 corresponds to glucose. Therefore, SERS spectroscopy for the chemical composition analysis of CHMs has become a novel, fast, accurate and non-destructive method.
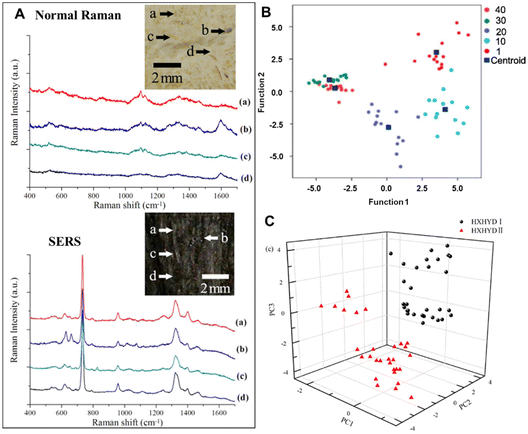 |
| Fig. 2 (A) The regular Raman spectra and SERS spectra (a)–(d) of the AMRP; the inset bright-field image indicates the locations of the AMRP employed for measurements. (B) A scatter plot of PCA-LDA scores for the PCR samples in different age groups. (C) A 3D mapping of the PCA results for HXHYD I (black circle) and HXHYD II (red triangle). Reprinted with permission from ref. 45, 49 and 53. Copyright 2013 IOP Publishing, 2023 Elsevier and 2015 IOP Publishing. | |
Another important indicator of some CHM raw materials is its age. Typically, clinical quality improves with age or storage time, with higher quality meaning higher prices,46–48 because the development and growth of many medicinal parts of plants and animals take time, and the active ingredients accumulate over time. However, determining the age of these herbal raw materials is challenging, especially for inexperienced pharmacists. Recently, studies have shown such feasibility of age determination using SERS. For example, Dai et al. have investigated aged Pericarpium Citri Reticulatae (PCR) using SERS.49 In conjunction with PCA-LDA, interestingly, the peak intensity at 1607 cm−1 was negatively correlated with PCR age (1, 10, 20 and 30 years). Based on such SERS spectral analysis, the classification accuracy can exceed 90% (Fig. 2B). This suggests that SERS can be used as a new method to identify the age of CHM products.
In addition to the direct detection of raw materials, SERS has a capacity to distinguish the principal constituents of the same CHM with different weight ratios of raw materials in their original formulation.50–52 For example, Huang et al. used Ag colloid-based SERS technology to study the chemical constituents of two Huoxue Huayu decoctions.53 The two decoctions were made from 12 same herbs with different weight ratios. Their corresponding difference in the SERS spectrum could be clearly observed in the band intensities of 523, 538, 622, 647, 732, 977, 1003, 1048, 1326, 1402, 1456 and 1605 cm−1, which arises from the different percentages of biochemical constituents in the two decoctions. Further linear discriminant analysis can achieve a diagnostic accuracy of 100% for identifying the two decoctions (Fig. 2C).
4. Bioactive constituent detection
Fully identifying the active constituents to ensure clinical effectiveness and safety is the primary issue in the quality control of CHM and its modernization research.54–56 This faces challenges from three aspects of CHMs: (1) low concentration of active ingredients in the herb extract; (2) interference from lots of other substances in the herb extract; and (3) time-consuming separation that might result in permanent loss of active ingredients. Although people have made many attempts and developed methods to solve these problems, such as high-performance liquid chromatography, high-speed counter current chromatography, macroreticular resin chromatography, etc., these chromatographic techniques still rely on solving two fundamental problems: limited sensitivity or specificity for target analytes and incomplete separation of compounds with similar structures or polarity. Recently, SERS combined with other techniques has been proven to have great potential in solving these problems with promising prospects in the rapid analysis and discovery of herbal bioactive compounds,45,57–59 due to its ultrahigh sensitivity at the single-molecule level and good capability of various analyte configurations with unique spectral fingerprints.
To show the direct analysis of active constituents without the separation and extraction procedures, Zhao et al. used SERS to probe the main active constituent of berberine in raw Coptis chinensis and Phellodendron amurense.57 The SERS spectra of CHM sample dilutions with silver colloid clearly show the characteristic peaks of berberine for both Coptis chinensis and Phellodendron amurense but not for the counterfeit of Radix of Platycodon grandiflorus, which highlights the ability of SERS to directly detect the active constituents of raw TCMs. Furthermore, quantitative analysis found a good linear relationship between the Raman band intensity at 727 cm−1 and berberine concentration below 5 μM (Fig. 3A), although the liquid chromatography-mass spectrometry analysis revealed that the decoction sample extract contains at least four other constituents.
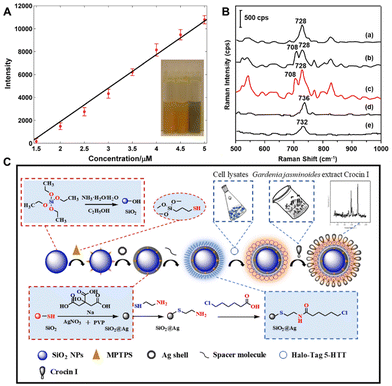 |
| Fig. 3 (A) Fitting result of the relationship of intensity and berberine concentration. (B) The corresponding SERS spectra of five target analytes: (a) BER standard, (b) COP standard, (c) mixture of the four standards, (d) PAL standard and (e) JAT standard. (C) Schematic illustration of direct screening of potential active ingredients in Gardenia jasminoides extract by using a bioconjugated dual-functional SERS probe. Reprinted with permission from ref. 57–59. Copyright 2014 Wiley, 2018 Elsevier and 2023 Elsevier. | |
SERS is vibrational spectroscopy with the ability to distinguish molecules of similar structures, which provides the possibility to detect similar active constituents in many herbal medicines. For example, many herbal medicines mainly contain protoberberine analogues, which have the same mother core structure, called protoberberine, such as berberine (BER), coptisine (COP), palmatine (PAL) and lanthazine (JAT).60 While there are one or two dioxolane rings in BER and COP, the dioxolane ring is broken and replaced by methoxyl and hydroxyl in PAL and JAT. To identify these bioactive ingredients in CHMs, Du et al. have employed a promising method combined with thin layer chromatography (TLC) and ratiometric SERS.58 It is significant that PAT and JAL are distinctly separated from the other two protoberberines, but BER and COP are extremely difficult to distinguish due to the poor resolution. After the optimal volume of Ag colloid was dropped on five spots of the TLC plate after the development of five target analytes, the corresponding SERS spectra are shown in Fig. 3B. It is obvious that the four protoberberines can be identified by the characteristic peaks at 728, 708, 736 and 732 cm−1, and the identification of BER and COP can be especially discriminated by ratiometric Raman intensity (I708/I728).
In order to improve specificity in SERS detection, functionalized probes are usually developed and used to modify the substrate.61–63 Although the affinity screening method based on immobilized proteins has demonstrated dramatic advances in screening bioactive compounds from natural products,64–66 it is still challenged by long screening time, binder loss and low contents in samples. To address the urgent needs, Zhao et al. have employed a dual-functional SERS probe to screen and detect bioactive ingredients binding to the target protein from Gardenia jasminoides extract.59 As shown in Fig. 3C, through a bioorthogonal dehalogenation reaction between the fusion protein tag and the halogenated alkanes, the Halo-tagged serotonin transporter (5-HTT) can be immobilized onto SiO2@Ag core–shell nanoparticles which have been modified by 6-chlorohexanoic acid. The SERS spectra of Gardenia jasminoides extract mixed with four drugs clearly show that immobilized 5-HTT nanoparticles can specifically recognize and screen the bioactive constituent crocin I of Gardenia jasminoides. Besides, the intensity of the characteristic peaks at 629 cm−1 and 718 cm−1 changes a little in 3 days, indicating that the immobilized 5-HTT nanoparticles have sufficient stability to screen bioactive compounds from Chinese herbal medicine extracts within minutes. Furthermore, according to the signal-to-noise ratio of the Raman peak at 1543 cm−1, the detection limit of immobilized 5-HTT nanoparticles is 10 ng mL−1, indicating that it has sufficient sensitivity in the trace detection of Gardenia jasminoides. Finally, the immobilized 5-HTT nanoparticles have good repeatability, and their RSD is 3.57%. Therefore, this approach was recognized as a promising method for the identification and screening of bioactive compounds from natural herbal medicines with desirable specificity, stability, sensitivity, and repeatability.
5. Adulterant characterization
With the increase in demand and price of CHMs, the problem of illegal addition of chemical synthetic drugs and counterfeit herbal raw materials has become increasingly prominent. Thus, the adulteration detection of CHMs is critically important to ensure patient safety. However, the complexity of CHM constituents makes this work challenging.
To improve the efficacy of CHMs in a short time, nifedipine, phenformin, metformin and rosiglitazone are often added to a hypoglycemic drug;67 sildenafil and tadalafil are added to some healthcare products; and nicardipine hydrochloride, doxazosin mesylate, propranolol hydrochloride and hydrochlorothiazide are added into hypotensors.68 Besides, pharmacologically similar chemicals are often found to be used for illegal adulteration of herbs,69,70 such as diphenhydramine hydrochloride (DIP), benproperine phosphate (BEN) and chlorphenamine maleate (CHL), which could cause huge threats to human health. As shown in Fig. 4A, Yang et al. have employed TLC combined with dynamic surface enhanced Raman spectroscopy (DSERS) for on-site detection of antitussive and antiasthmatic drugs with adulterants in herbs.71 It is found that 50% glycerol silver colloid leads to the most intense bands and the peak signals could remain for a rather long period of time at a rather high level. The simulated positive sample can be separated into three spots representing the reference chemicals DIP, BEN, and CHL, while a simulated negative sample did not contain the abovementioned three adulterants in herbs. In addition, the SERS spectra of the three separated spots of the simulated positive sample are shown in Fig. 4B with red lines, and black lines indicate the SERS spectra of the three different adulterants, illustrating that TL-DSERS was able to specifically identify adulterants.
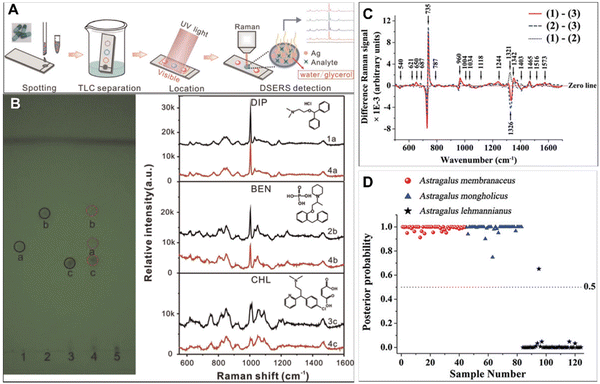 |
| Fig. 4 (A) Schematic illustration of TLC–DSERS for on-site detection of antitussive and antiasthmatic drugs adulterated in BDS. (B) Results from TLC analysis and the highest SERS spectra of reference chemicals (black lines) and corresponding three separated spots of simulated positive samples (red lines). (C) Difference spectra calculated from the mean Raman spectra. (D) Scatter plot of the posterior probability for the classification of authentic Astragali Radix samples and adulterant samples. Reprinted with permission from ref. 71 and 75. Copyright 2015 Elsevier and 2018 Informa UK Limited. | |
Since the plants used as raw materials for CHMs have other plants in similar families and genera, it is sometimes difficult to distinguish them purely from their appearance. The fingerprint information in Raman spectra might solve this problem well. A prime example of counterfeits Astragali Radix is effective in boosting the vitality and immunity of the human system.72–74 Since the appearances of adulterants are similar to that of authentic Astragali Radix, the quality identified only from the morphology easily causes misidentification. In general, the roots of Astragalus membranaceus and Astragalus mongholicus are authentic Astragali Radix, but there are still some adulterants on the commercial market, such as Astragalus lehmannianus. Yu et al. have employed SERS technology to detect the percentage of active constituents for adulterant identification of Astragali Radix.75 As shown in Fig. 4C, the Raman peaks at 735, 960, 1244, 1342, 1465, and 1573 cm−1 are more intense for authentic Astragali Radix than for the adulterant, while the Raman peaks at 1321 and 1403 cm−1 are greater in the adulterant. Besides, the PCA-LDA statistical approach for the identification of authentic Astragali Radix samples from the adulterant samples shows a high diagnostic sensitivity (100%) and specificity (97.6%) (Fig. 4D). These results suggest that there are significant changes in the percentage of chemical constituents in different sample groups, indicating the potential of SERS spectroscopy for adulterant identification.
In addition, a dye can change the colour of unregulated herbs or the ingredients without pharmacodynamic effects as an adulterant,76–78 which may cause the risk of carcinoma and genetic damage. For example, Sudan dyes, as a fat-soluble compound, are often doped in various CHMs and tonics due to their low cost and color fastness. However, the specific detection of dyes in the extremely complex composition of CHMs is still a significant challenge. For rapid direct identification of dye adulterants, Zhao et al. have developed defective Cu2O hollow nanocubes as SERS substrates to detect the trace Sudan dye adulteration in a herbal medicine matrix.79 As shown in Fig. 5A, the strong coordination between the hydroxyl/azo groups and exposed Cu sites can lead to great chemical enhancement which enables selective detection of SR3 from multivariate specimens with a detection limit of 10−6 M (Fig. 5B). Furthermore, this approach can directly detect SR3 adulteration in various herbal medicines even at an extremely low concentration of 0.3 mg kg−1. Thus, the direct and selective detection of dyes by semiconducting SERS could be an attractive method for the adulterant identification of CHMs, without requiring time-consuming pretreatments.
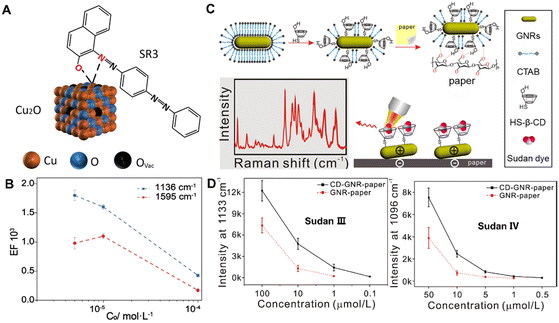 |
| Fig. 5 (A) Schematic illustration of the interaction between the SR3 molecule and the Cu2O surface. (B) The calculated EF plotted as a function of SR3 concentration on the logarithmic scale, with the error bars representing the statistical analysis over 20 spots per sample. (C) Schematic representation of the preparation progress of CD-GNR-paper and the detection of Sudan molecules. (D) Intensity plots of the represented bands at 1133 cm−1 for Sudan III and 1097 cm−1 for Sudan IV with different concentrations using CD-GNR-paper (solid line) and GNR-paper (dotted line). Reprinted with permission from ref. 79 and 83. Copyright 2021 Wiley and 2018 Elsevier. | |
Generally, Sudan dyes, water-insoluble molecules, have little affinity to hydrophilic SERS substrates, resulting in reduced detection sensitivity and selectivity. To date, various methods and techniques have been developed to improve the affinity of target molecules toward SERS substrates, including the use of probe molecules80,81 and graphene oxide.82 For example, Lu et al. have developed a functional paper-based SERS substrate for rapid and sensitive detection of Sudan dyes in herbal medicine.83 As shown in Fig. 5C, gold nanorods are functionalized with mono-6-thio-cyclodextrin (HS-β-CD) to form CD-GNR through strong chemical interactions of Au–S bonds. Then, the CD-GNR can adsorb on the surface of the paper through electrostatic interactions and hydrogen bonding to form CD-GNR-paper. Finally, the GNR functionalized with HS-β-CD enable the capture of the Sudan molecules through non-covalent interactions. As shown in Fig. 5D, CD-GNR-paper enables rapid and sensitive detection with the LOD value for Sudan III being 0.1 μM and that of Sudan IV being 0.5 μM. Besides, CD-GNR-paper can effectively collect the Sudan dyes illegally adulterated into samples of Resina draconis with a simple operation, opening up new exciting opportunities for SERS detection of more compounds illegally added with high sensitivity and fast signal responses.
6. Contaminant detection
With the artificial cultivation of raw materials and environmental pollution, pesticide residues,84,85 heavy metal/metalloids,86 and mycotoxins87 have become the main contaminant sources of CHMs, posing a huge threat to human health. To ensure the quality of CHMs, it is necessary to probe these residual contaminants of CHMs, which requires efficient and convenient detection methods. To date, many studies have adopted SERS to detect pollutants in CHMs, including deltamethrin, thiram, mercury (Hg2+), cadmium (Cd2+), lead (Pb2+), arsenic (As3+), mycotoxins etc.
The complex composition of CHMs poses a huge challenge to the effective detection of the contamination of trace pesticide residues by SERS. One of the effective strategies is the removal of the interfering constituents.88,89 For example, He et al. used carbon nanotubes for pre-adsorption treatment, and then used SERS to realize the quantitative detection of deltamethrin in Corydalis herbs.90 As schematically shown in Fig. 6A, the amount of multi-walled carbon nanotubes (MWCNTs) was optimized to purify the interfering substances in the pretreatment process. The intensity of two characteristic peaks at 559 cm−1 and 999 cm−1 increased with the increase in the concentration of deltamethrin. In the partial least squares regression (PLSR) model, which is based on the characteristic peak intensity of a combination of two peak bands, the correlation coefficient of determination (R2), root mean square error for prediction (RMSEP) and residual predictive deviation (RPD) were 0.9306, 0.710 and 3.046, respectively (Fig. 6B). In the deltamethrin quantitative analysis model, the PLSR model combined two peak bands showing the best prediction and the LOD was 0.484 mg L−1.
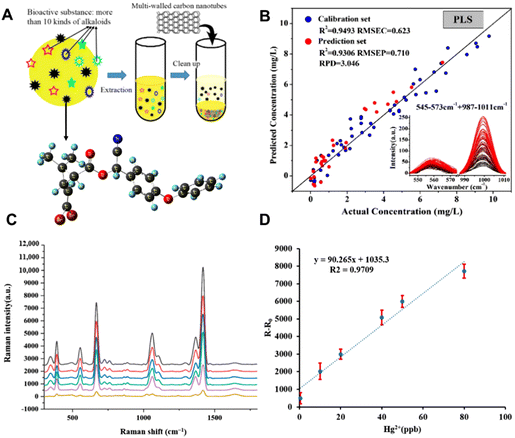 |
| Fig. 6 (A) Schematic diagram of purification by multi-walled carbon nanotubes (MWCNTs). (B) Linear regression equation between peak intensity and deltamethrin content in Corydalis. (C) Raman spectra of the reaction between different concentrations of Hg2+ and Fe3O4@SiO2@AuNPs-DMcT SERS probes. (D) Linear relationship between Hg2+ concentration and SERS peak intensity at 1418 cm−1 for DMcT. Reprinted with permission from ref. 90 and 93. Copyright 2020 MDPI and 2022 MDPI. | |
In contaminant detection of harmful elements such as heavy metals, it is usually necessary to allow their interaction with SERS probe molecules and ions to achieve specific and efficient detection.91,92 For example, Zhang et al. have proposed the use of functional Fe3O4@SiO2@AuNPs as a Raman sign amplifier, combined with a probe molecule DMcT for the detection of Hg2+ in the complex system of licorice.93 The Fe3O4 nanoparticles coated with a SiO2 protective shell appeared slightly stable and dispersible, and could be aminated and connected with AuNPs to obtain the magnetic properties and Raman-enhanced properties. As shown in Fig. 6C, the SERS intensity of DMcT increased with the increase in the concentration of Hg2+. Among them, the characteristic Raman peak at 1418 cm−1 was assigned to the C
N vibration in DMcT which is a good marker for the detection of Hg2+. Besides, the relative Raman intensity at 1418 cm−1 showed an excellent linear relationship with the concentration of Hg2+ (0.5–80 ng mL−1). Fig. 6D shows its good linear relationship with an R2 of 0.9709.
In addition, CHM raw materials are susceptible to mycotoxin contamination during drying and storage,94,95 such as aflatoxin B1 (AFB1), zearalenone (ZEN) and deoxynivalenol (DON). Although a variety of SERS active substrates, such as Au nanobipyramids,96 silica-encapsulated hollow Au,97 MXenes@Au dimers,98 Au@Ag nanorods,99 Au@Ag nanotriangles,100 Au nanostar core-silver nanoparticle satellite assemblies,101 and graphene oxide-Au@Ag,102 have been widely used in the detection of mycotoxins, the sensitivity, repeatability and stability of the detection are still challenging. Recently, gold magnetic nanoparticles have become a promising SERS active substrate,103–105 which can not only provide magnetic field regulation to improve repeatability, stability and sensitivity, but also provide aptamer modification sites to improve detection specificity. For example, Wang et al. have built controllable hot spots by the combination of Fe3O4@Au NPs and Ag NPs using the aflatoxin B1 aptamer and cDNA for rapid detection of AFB1 in coix seed.106 As shown in Fig. 7A, satellite structured Fe3O4@Au nanocomposites modified with the AFB1 aptamer can offer rapid separation via applying a magnetic field, and also possess SERS signal enrichment effects, and the Ag NPs modified with DTNB and cDNA can form through DNA hybridization with Fe3O4@Au nanocomposites as aptasensors. The logarithm of Raman intensity at 1331 cm−1 had a good linear correlation with the logarithm of AFB1 concentration in the range of 0.01 and 100 ng mL−1. As shown in Fig. 7B, the correlation coefficient R2 was 0.9948 with the calculated LOD = 0.0060 ng mL−1, which is significantly lower than the maximum allowable limits in China. The proposed SERS aptasensor enables quantitative detection of AFB1 in actual samples directly, which shows the promising potential of this aptasensor in rapid and effective detection of AFB1 in CHMs.
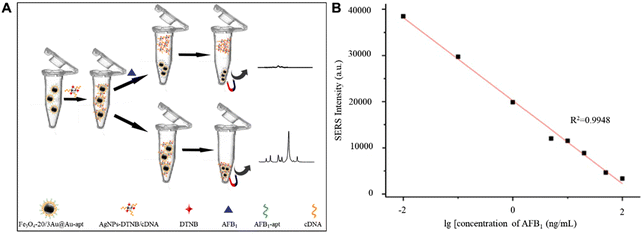 |
| Fig. 7 (A) Schematic illustration of the proposed SERS aptasensor for AFB1 detection. (B) Calibration curve of a SERS based aptasensor for AFB1 at different concentrations. Reprinted with permission from ref. 106. Copyright 2022 Elsevier. | |
7. Conclusions and outlook
In summary, the importance of SERS spectroscopy in CHM analysis has become increasingly prominent in the past two decades. The technique can identify and screen raw materials based on their chemical “fingerprints” with ultra-high sensitivity. Furthermore, SERS spectroscopy can be used for rapid online inspection and analysis, such as identification of active ingredients, identification of adulterants, and trace detection of pollutants. In particular, aptamer-modified SERS-active nanocomposites can specifically and quantitatively detect the concentration of mycotoxins in CHMs. Thus, SERS techniques can provide a promising platform for simple, rapid, low-cost and nondestructive detection for safety assessment and quality control of CHMs.
Despite all the advancements, the use of SERS to detect the CHMs is still in its preliminary stage and many challenges still exist. First, due to the wide variety of materials used for CHMs, a comprehensive SERS database has not yet been established. Then, uniform and reproducible SERS signals depend heavily on the optimization and stabilization of the substrates. Currently, most studies are based on the uncontrollable distribution of hotspots in the SERS active substrates, which have intrinsic limitations for quantitative detection. Moreover, enhanced detection performance of SERS requires the proximity of molecules on the surface of plasmonic nanoparticles. The active ingredients in CHM samples often exist in small quantities and face competitive adsorption from other components. In addition, the strong fluorescence of components in most biological samples in the visible light region can also significantly decrease the SERS detection sensitivity.
In order to overcome these shortcomings, current efforts to fabricate SERS substrates with high reproducibility and good stability, especially functionalizing the substrates to selectively capture target analytes, are a step in the right direction. On the other hand, combining SERS with other separation techniques such as HPLC, thin layer chromatography, chemical and physical extraction, and phase extraction can greatly facilitate its practical application in the quality control of CHMs. Furthermore, the recent advances in deep learning and artificial intelligence data processing for extracting chemical information from Raman experiments have had a significant impact on the development of SERS sensors in many fields.107–109 These greatly improve the analytical performance of Raman spectrum classification in food and beverage, forensics, bacteria and viruses, medical diagnostics, etc., providing an important reference for the analysis of the complex components of CHMs. Finally, the performance of handheld Raman spectrometers establishes the great potential of SERS to serve not only as a standard laboratory method but also as a mobile platform for drug detection including CHMs.
Author contributions
Conceptualization: Y. H. W. and P. S.; writing – original preparation: C. X. X. and Z. Y.; revisions: P. S. and Y. H. W. Both authors have read and agreed to the published version of the manuscript.
Conflicts of interest
The authors declare no conflict of interest.
Acknowledgements
We are thankful for the financial support from the National Natural Science Foundation of China (No. 22102150 and 22204143), the Zhejiang Provincial Natural Science Foundation of China (No. LQ21B030010 and LQ22B050006) and China Postdoctoral Science Foundation (2022M722827).
References
- D. Normile, The new face of traditional Chinese medicine, Science, 2003, 299, 188–190 CrossRef CAS
.
- F. Cheung, TCM: Made in China, Nature, 2011, 480, S82–S83 CrossRef CAS
.
- U. Eckstein-Ludwig, R. J. Webb, I. D. A. van Goethem, J. M. East, A. G. Lee, M. Kimura, P. M. O'Neill, P. G. Bray, S. A. Ward and S. Krishna, Artemisinins target the SERCA of Plasmodium falciparum, Nature, 2003, 424, 957–961 CrossRef CAS
.
- C.-C. Huang, Applications of Raman spectroscopy in herbal medicine, Appl. Spectrosc. Rev., 2016, 51, 1–11 CrossRef CAS
.
- T. Efferth, P. C. H. Li, V. S. B. Konkimalla and B. Kaina, From traditional Chinese medicine to rational cancer therapy, Trends Mol. Med., 2007, 13, 353–361 CrossRef CAS
.
- R. Hoessel, S. Leclerc, J. A. Endicott, M. E. M. Nobel, A. Lawrie, P. Tunnah, M. Leost, E. Damiens, D. Marie, D. Marko, E. Niederberger, W. Tang, G. Eisenbrand and L. Meijer, Indirubin, the active constituent of a Chinese antileukaemia medicine, inhibits cyclin-dependent kinases, Nat. Cell Biol., 1999, 1, 60–67 CrossRef CAS PubMed
.
- T. K. Au, T. L. Lam, T. B. Ng, W. P. Fong and D. C. C. Wan, A comparison of HIV-1 integrase inhibition by aqueous and methanol extracts of Chinese medicinal herbs, Life Sci., 2001, 68, 1687–1694 CrossRef CAS PubMed
.
- M. Moloney, S. Tuck, A. Ramkumar, A. Furey and M. Danaher, Determination of pyrethrin and pyrethroid residues in animal fat using liquid chromatography coupled to tandem mass spectrometry, J. Chromatogr. B, 2018, 1077–1078, 60–70 CrossRef CAS
.
- J. M. Montiel-León, S. V. Duy, G. Munoz, M.-A. Verner, M. Y. Hendawi, H. Moya, M. Amyot and S. Sauvé, Occurrence of pesticides in fruits and vegetables from organic and conventional agriculture by QuEChERS extraction liquid chromatography tandem mass spectrometry, Food Control, 2019, 104, 74–82 CrossRef
.
- X. Gao, H. Guo, J. Wang and Q. Zhao, Sensitive and rapid determination of pyrethroids in human blood by gas chromatography–tandem mass spectrometry with ultrasound-assisted dispersive liquid-liquid microextraction, Drug Test. Anal., 2018, 10, 1131–1138 CrossRef CAS PubMed
.
- X. Cao, Q. Xie, S. Zhang, H. Xu, J. Su, J. Zhang, C. Deng and G. Song, Fabrication of functionalized magnetic microspheres based on monodispersed polystyrene for quantitation of allyl-benzodioxoles coupled with gas chromatography and mass spectrometry, J. Chromatogr., A, 2019, 1607, 460402 CrossRef CAS
.
- A. P. Grollman, S. Shibutani, M. Moriya, F. Miller, L. Wu, U. Moll, N. Suzuki, A. Fernandes, T. Rosenquist, Z. Medverec, K. Jakovina, B. Brdar, N. Slade, R. J. Turesky, A. K. Goodenough, R. Rieger, M. Vukelić and B. Jelaković, Aristolochic acid and the etiology of endemic (Balkan) nephropathy, Proc. Natl. Acad. Sci. U. S. A., 2007, 104, 12129–12134 CrossRef CAS PubMed
.
- A. Kaňa, M. Sadowska, J. Kvíčala and O. Mestek, Simultaneous determination of oxo- and thio-arsenic species using HPLC-ICP-MS, J. Food Compos. Anal., 2020, 92, 103562 CrossRef
.
- Z. Movasaghi, S. Rehman and I. U. Rehman, Raman spectroscopy of biological tissues, Appl. Spectrosc. Rev., 2007, 42, 493–541 CrossRef CAS
.
- A. C. S. Talari, Z. Movasaghi, S. Rehman and I. U. Rehman, Raman Spectroscopy of Biological Tissues, Appl. Spectrosc. Rev., 2015, 50, 46–111 CrossRef CAS
.
- H. G. M. Edwards, T. Munshi and K. Page, Analytical discrimination between sources of ginseng using Raman spectroscopy, Anal. Bioanal. Chem., 2007, 389, 2203–2215 CrossRef CAS
.
- S. Li, Y. Shan, X. Zhu, X. Zhang and G. Ling, Detection of honey adulteration by high fructose corn syrup and maltose syrup using Raman spectroscopy, J. Food Compos. Anal., 2012, 28, 69–74 CrossRef CAS
.
- M. Macernis, D. Galzerano, J. Sulskus, E. Kish, Y.-H. Kim, S. Koo, L. Valkunas and B. Robert, Resonance Raman spectra of carotenoid molecules: Influence of Methyl Substitutions, J. Phys. Chem. A, 2015, 119, 56–66 CrossRef CAS
.
- J. S. Rooney, A. McDowell, C. J. Strachan and K. C. Gordon, Evaluation of vibrational spectroscopic methods to identify and quantify multiple adulterants in herbal medicines, Talanta, 2015, 138, 77–85 CrossRef CAS PubMed
.
- Y.-H. Liao, C.-H. Wang, C.-Y. Tseng, H.-L. Chen, L.-L. Lin and W. Chen, Compositional and conformational analysis of Yam proteins by near infrared Fourier Transform Raman spectroscopy, J. Agric. Food Chem., 2004, 52, 8190–8196 CrossRef CAS
.
- P. Rösch, J. Popp and W. Kiefer, Raman and surface enhanced Raman spectroscopic investigation on Lamiaceae plants, J. Mol. Struct., 1999, 480–481, 121–124 CrossRef
.
- J.-B. Chen, S.-Q. Sun, F. Ma and Q. Zhou, Vibrational microspectroscopic identification of powdered traditional medicines: chemical micromorphology of poria observed by infrared and Raman microspectroscopy, Spectrochim. Acta, Part A, 2014, 128, 629–637 CrossRef CAS PubMed
.
- S. Azimi and A. Docoslis, Recent advances in the use of surface-enhanced Raman scattering for illicit drug detection, Sensors, 2022, 22, 3877 CrossRef CAS PubMed
.
- X. M. Qian and S. M. Nie, Single-molecule and single-nanoparticle SERS: from fundamental mechanisms to biomedical applications, Chem. Soc. Rev., 2008, 37, 912–920 RSC
.
- L. Zheng, H. Mao, L. Zhang, Y. Jin, Y. Zhou, Y. Peng and S. Du, Aminopyrine Raman spectral features characterised by experimental and theoretical methods: toward rapid SERS detection of synthetic antipyretic–analgesic drug in traditional Chinese medicine, Anal. Methods, 2014, 6, 5925–5933 RSC
.
- D. Li, Q. Zhu, D. Lv, B. Zheng, Y. Liu, Y. Chai and F. Lu, Silver-nanoparticle-based surface-enhanced Raman scattering wiper for the detection of dye adulteration of medicinal herbs, Anal. Bioanal. Chem., 2015, 407, 6031–6039 CrossRef CAS
.
- M. Fleischmann, P. J. Hendra and A. J. McQuillan, Raman spectra of pyridine adsorbed at a silver electrode, Chem. Phys. Lett., 1974, 26, 163–166 CrossRef CAS
.
- M. D. Sonntag, J. M. Klingsporn, A. B. Zrimsek, B. Sharma, L. K. Ruvuna and R. P. Van Duyne, Molecular plasmonics for nanoscale spectroscopy, Chem. Soc. Rev., 2014, 43, 1230–1247 RSC
.
- Y.-J. Zhang, P. M. Radjenovic, X.-S. Zhou, H. Zhang, J.-L. Yao and J.-F. Li, Plasmonic core–shell nanomaterials and their applications in spectroscopies, Adv. Mater., 2021, 33, 2005900 CrossRef CAS
.
- S.-Y. Ding, J. Yi, J.-F. Li, B. Ren, D.-Y. Wu, R. Panneerselvam and Z.-Q. Tian, Nanostructure-based plasmon-enhanced Raman spectroscopy for surface analysis of materials, Nat. Rev. Mater., 2016, 1, 16021 CrossRef CAS
.
- A. Hakonen, P. O. Andersson, M. S. Schmidt, T. Rindzevicius and M. Käll, Explosive and chemical threat detection by surface-enhanced Raman scattering: A review, Anal. Chim. Acta, 2015, 893, 1–13 CrossRef CAS PubMed
.
- Y. Fang, N.-H. Seong and D. D. Dlott, Measurement of the distribution of site enhancements in surface-enhanced Raman scattering, Science, 2008, 321, 388–392 CrossRef CAS
.
- C. Li, Y. Huang, X. Li, Y. Zhang, Q. Chen, Z. Ye, Z. Alqarni, S. E. J. Bell and Y. Xu, Towards practical and sustainable SERS: a review of recent developments in the construction of multifunctional enhancing substrates, J. Mater. Chem. C, 2021, 9, 11517–11552 RSC
.
- W. Ji, X. Zhang, J. Zhao, Y. Gao, W. Song and Y. Ozaki, In situ formation of SERS hot spots by a bis-quaternized perylene dye: a simple strategy for highly sensitive detection of heparin over a wide concentration range, Analyst, 2018, 143, 1899–1905 RSC
.
- L. Zhang, L.-Z. Su, Z. Yu, L.-Y. Su, Y. Zheng, P. Song, Y.-H. Wang, Y.-B. Pan and B. Zheng, Quantitative detection of creatinine in human serum by SERS with evaporation-induced optimal hotspots on Au Nanocubes, ACS Appl. Nano Mater., 2022, 5, 4841–4847 CrossRef CAS
.
- C. Y. Zhang, D. L. Chang and S. L. Chen, Simultaneous determination of five nonsteroidal anti-inflammatory drugs and two glucocorticoids in adulterated traditional herbal medicines for the treatment of rheumatism, Anal. Lett., 2011, 44, 1769–1782 CrossRef CAS
.
- H. Huang, M. Liu and P. Chen, Recent advances in ultra-high performance liquid chromatography for the analysis of traditional Chinese medicine, Anal. Lett., 2014, 47, 1835–1851 CrossRef CAS
.
- Y. Q. Chang, S. N. Tan, J. W. H. Yong and L. Ge, Determination of flavonoids in costus speciosus and etlingera elatior by liquid chromatography-mass spectrometry, Anal. Lett., 2012, 45, 345–355 CrossRef CAS
.
- J. Ye, Application of gas chromatography-mass spectrometry in research of traditional Chinese medicine, Chem. Pap., 2009, 63, 506–511 CAS
.
- L. Shi, Y. Li, Q. Wang, C. Xue and S. Wang, Synthesis of one-dimensional ZnO nanorods by oxidating zinc films deposited with magnetron sputtering, Chin. J. Semicond., 2004, 25, 1211–1214 CAS
.
- W. Huang, L. Chen, B. Zhang, M. Park and M. Toborek, PPAR agonist-mediated protection against HIV tat-induced cerebrovascular toxicity is enhanced in MMP-9-deficient mice, J. Cereb. Blood Flow Metab., 2014, 34, 646–653 CrossRef CAS
.
- P. Guo, Y. L. Yuan and P. Xiong, Analysis of gynostemma pentaphyllum (Thunb) makino for Chinese crude drug by FTIR and FT-Raman, Spectrosc. Spectral Anal., 2004, 24, 1210 CAS
.
-
I. L. Lin, Y. S. Yen and A. Chang, In A Study on Digital Forensics Standard Operation Procedure for Wireless Cybercrime, 2011 Fifth International Conference on Innovative Mobile and Internet Services in Ubiquitous Computing, 30 June-2 July 2011; 2011; pp. 543–548.
- P. Xiong, P. Guo and Q. Zhou, The analysis of radix aconiti kusnezoffii by FT-Raman spectra, Spectrosc. Spectral Anal., 2002, 22, 417–418 CAS
.
- H. Huang, H. Shi, S. Feng, J. Lin, W. Chen, Y. Yu, D. Lin, Q. Xu and R. Chen, Quick detection of traditional Chinese medicine ‘Atractylodis Macrocephalae Rhizoma’ pieces by surface-enhanced Raman spectroscopy, Laser Phys., 2013, 23, 015601 CrossRef CAS
.
- Q. Shi, T. Guo, T. Yin, Z. Wang, C. Li, X. Sun, Y. Guo and W. Yuan, Classification of pericarpium citri reticulatae of different ages by using a voltammetric electronic tongue system, Int. J. Electrochem. Sci., 2018, 13, 11359–11374 CrossRef CAS
.
- M. Luo, H. Luo, P. Hu, Y. Yang, B. Wu and G. Zheng, Evaluation of chemical components in Citri Reticulatae Pericarpium of different cultivars collected from different regions by GC–MS and HPLC, Food Sci. Nutr., 2018, 6, 400–416 CrossRef CAS
.
- S.-Z. Li, X.-M. Guan, Z. Gao, H.-C. Lan, Q. Yin, C. Chu, D.-P. Yang, E. H. Liu and P. Zhou, A simple method to discriminate Guangchenpi and Chenpi by high-performance thin-layer chromatography and high-performance
liquid chromatography based on analysis of dimethyl anthranilate, J. Chromatogr. B, 2019, 1126–1127, 121736 CrossRef CAS
.
- G. Dai, L. Wu, J. Zhao, Q. Guan, H. Zeng, M. Zong, M. Fu and C. Du, Classification of Pericarpium Citri Reticulatae (Chenpi) age using surface-enhanced Raman spectroscopy, Food Chem., 2023, 408, 135210 CrossRef CAS PubMed
.
- J. Hou, C. Yao, Y. Li, L. Yang, X. Chen, M. Nie, H. Qu, S. Ji and D.-A. Guo, A MS-feature-based medicinal plant database-driven strategy for ingredient identification of Chinese medicine prescriptions, J. Pharm. Biomed. Anal., 2023, 234, 115482 CrossRef CAS PubMed
.
- C. Y. Ung, H. Li, C. Y. Kong, J. F. Wang and Y. Z. Chen, Usefulness of traditionally defined herbal properties for distinguishing prescriptions of traditional Chinese medicine from non-prescription recipes, J. Ethnopharmacol., 2007, 109, 21–28 CrossRef CAS PubMed
.
- D.-Y. Lin, W.-T. Huang, Y.-C. Lin, H.-H. Hung, S.-C. Ou, C.-W. Chang, H.-E. Lin, T.-Y. Lin, C.-W. Chang, H.-C. Hung and S.-T. Huang, Prescription system to calculate precise doses of Chinese herbal medicine to avoid toxic effects, Heliyon, 2023, 9, e16612 CrossRef CAS PubMed
.
- W. Chen, J. Lin, R. Chen, S. Feng, Y. Yu, D. Lin, M. Huang, H. Shi and H. Huang, Detection and identification of Huo–Xue–Hua–Yu decoction (HXHYD) using surface-enhanced Raman scattering (SERS) spectroscopy and multivariate analysis, Laser Phys. Lett., 2015, 12, 045602 CrossRef
.
- W.-F. Li, J.-G. Jiang and J. Chen, Chinese medicine and its modernization demands, Arch. Med. Res., 2008, 39, 246–251 CrossRef
.
- R. Graziose, M. A. Lila and I. Raskin, Merging traditional Chinese medicine with modern drug discovery technologies to find novel drugs and functional foods, Curr. Drug Discovery Technol., 2010, 7, 2–12 CrossRef CAS PubMed
.
- D.-A. Guo, A. Lu and L. Liu, Modernization of traditional Chinese medicine, J. Ethnopharmacol., 2012, 141, 547–548 CrossRef PubMed
.
- J. Zhao, Y. Liu, A. M. Fales, J. Register, H. Yuan and T. Vo-Dinh, Direct analysis of traditional Chinese medicines using surface-enhanced raman scattering (SERS), Drug Test. Anal., 2014, 6, 1063–1068 CrossRef CAS
.
- X. Gu, Y. Jin, F. Dong, Y. Cai, Z. You, J. You, L. Zhang and S. Du, Toward rapid analysis, forecast and discovery of bioactive compounds from herbs by jointly using thin layer chromatography and ratiometric surface-enhanced Raman spectroscopy technique, J. Pharm. Biomed. Anal., 2018, 153, 9–15 CrossRef CAS PubMed
.
- D. Zhang, J. Ma, X. Zheng, Z. Zhang, X. Lian, X. Zhao and X. Zhao, Fabrication of a bioconjugated dual-functional SERS probe for facile compound screening and detection, Biosens. Bioelectron., 2023, 234, 115369 CrossRef CAS PubMed
.
- C. Sun, J. Li, X. Wang, W. Duan, T. Zhang and Y. Ito, Preparative separation of quaternary ammonium alkaloids from Coptis chinensis Franch by pH-zone-refining counter-current chromatography, J. Chromatogr., A, 2014, 1370, 156–161 CrossRef CAS
.
- M.-M. Gu, P.-C. Guan, S.-S. Xu, H.-M. Li, Y.-C. Kou, X.-D. Lin, M. Kathiresan, Y. Song, Y.-J. Zhang, S.-Z. Jin and J.-F. Li, Ultrasensitive detection of SARS-CoV-2 S protein with aptamers biosensor based on surface-enhanced Raman scattering, J. Chem. Phys., 2023, 158, 024203 CrossRef CAS PubMed
.
- P.-C. Guan, H. Zhang, Z.-Y. Li, S.-S. Xu, M. Sun, X.-M. Tian, Z. Ma, J.-S. Lin, M.-M. Gu, H. Wen, F.-L. Zhang, Y.-J. Zhang, G.-J. Yu, C. Yang, Z.-X. Wang, Y. Song and J.-F. Li, Rapid point-of-care assay by SERS detection of SARS-CoV-2 virus and its variants, Anal. Chem., 2022, 94, 17795–17802 CrossRef CAS PubMed
.
- Y. Luo, M. Zhou, C. Fan, Y. Song, L. Wang, T. Xu and X. Zhang, Active enrichment of nanoparticles for ultra-trace point-of-care COVID-19 detection, Anal. Chem., 2023, 95, 5316–5322 CrossRef CAS
.
- R. Prudent, D. A. Annis, P. J. Dandliker, J.-Y. Ortholand and D. Roche, Exploring new targets and chemical space with affinity selection-mass spectrometry, Nat. Rev. Chem., 2021, 5, 62–71 CrossRef CAS PubMed
.
- J. Wang, Y. Wang, J. Liu, Q. Li, G. Yin, Y. Zhang, C. Xiao, T. Fan, X. Zhao and X. Zheng, Site-specific immobilization of β2-AR using O6-benzylguanine derivative-functionalized supporter for high-throughput receptor-targeting lead discovery, Anal. Chem., 2019, 91, 7385–7393 CrossRef CAS PubMed
.
- R. Tian, J. Yin, Q. Yao, T. Wang, J. Chen, Q. Liang, Q. Li and X. Zhao, Development of an allostery responsive chromatographic method for screening potential allosteric modulator of Beta2-adrenoceptor from a natural product-derived DNA-encoded chemical library, Anal. Chem., 2022, 94, 9048–9057 CrossRef CAS PubMed
.
- X. Yang, X. Wang and C. B. Ching, In situ monitoring of solid-state transition of p-aminobenzoic acid polymorphs using Raman spectroscopy, J. Raman Spectrosc., 2009, 40, 870–875 CrossRef CAS
.
- W. Pang, H. Yang, Z. Wu, M. Huang and J. Hu, LC-MS,–MS in MRM Mode for Detection and structural identification of synthetic hypoglycemic drugs added illegally to ‘Natural’ anti-diabetic herbal products, Chromatographia, 2009, 70, 1353–1359 CrossRef CAS
.
- S. A. Jordan, D. G. Cunningham and R. J. Marles, Assessment of herbal medicinal products: Challenges, and opportunities to increase the knowledge base for safety assessment, Toxicol. Appl. Pharmacol., 2010, 243, 198–216 CrossRef CAS PubMed
.
- T. C. Goosen, D. Cillié, D. G. Bailey, C. Yu, K. He, P. F. Hollenberg, P. M. Woster, L. Cohen, J. A. Williams, M. Rheeders and H. P. Dijkstra, Bergamottin contribution to the grapefruit juice–felodipine interaction and disposition in humans, Clin. Pharmacol. Ther., 2004, 76, 607–617 CrossRef CAS PubMed
.
- F. Fang, Y. Qi, F. Lu and L. Yang, Highly sensitive on-site detection of drugs adulterated in botanical dietary supplements using thin layer chromatography combined with dynamic surface enhanced Raman spectroscopy, Talanta, 2016, 146, 351–357 CrossRef CAS PubMed
.
- M. Angell and J. P. Kassirer, Alternative medicine—The risks of untested and unregulated remedies, N. Engl. J. Med., 1998, 339, 839–841 CrossRef CAS PubMed
.
- K. K. Adom and R. H. Liu, Antioxidant activity of grains, J. Agric. Food Chem., 2002, 50, 6182–6187 CrossRef CAS PubMed
.
- M. Chen, B. H. May, I. W. Zhou, C. C. L. Xue and A. L. Zhang, Meta-analysis of oxaliplatin-based chemotherapy combined with traditional medicines for colorectal cancer: contributions of specific plants to tumor response, Integr. Cancer Ther., 2015, 15, 40–59 CrossRef PubMed
.
- H. Lin, Y. Chen, S. Feng, W. Chen, D. Lin, H. Huang, J. Lin, R. Chen, G. Chen and Y. Yu, Surface-enhanced Raman on gold nanoparticles for the identification of the most common adulterant of Astragali Radix, Spectrosc. Lett., 2018, 51, 389–394 CrossRef CAS
.
- S. He, W. Xie, W. Zhang, L. Zhang, Y. Wang, X. Liu, Y. Liu and C. Du, Multivariate qualitative analysis of banned additives in food safety using surface enhanced Raman scattering spectroscopy, Spectrochim. Acta, Part A, 2015, 137, 1092–1099 CrossRef CAS
.
- L. He, Y. Su, B. Fang, X. Shen, Z. Zeng and Y. Liu, Determination of Sudan dye residues in eggs by liquid chromatography and gas chromatography–mass spectrometry, Anal. Chim. Acta, 2007, 594, 139–146 CrossRef CAS PubMed
.
- C. Villa, J. Costa, M. B. P. P. Oliveira and I. Mafra, Novel quantitative real-time PCR approach to determine safflower (Carthamus tinctorius) adulteration in saffron (Crocus sativus), Food Chem., 2017, 229, 680–687 CrossRef CAS PubMed
.
- Y. Jiang, S. Cong, G. Song, H. Sun, W. Zhang, W. Yao and Z. Zhao, Defective cuprous oxide as a selective surface-enhanced Raman scattering sensor of dye adulteration in Chinese herbal medicines, J. Raman Spectrosc., 2021, 52, 1265–1274 CrossRef CAS
.
- J. Ko, S. Lee, E. K. Lee, S. I. Chang, L. Chen, S. Y. Yoon and J. Choo, SERS-based immunoassay of tumor marker VEGF using DNA aptamers and silica-encapsulated hollow gold nanospheres, Phys. Chem. Chem. Phys., 2013, 15, 5379–5385 RSC
.
- H. L. Marks, M. V. Pishko, G. W. Jackson and G. L. Coté, Rational design of a bisphenol a aptamer selective surface-enhanced Raman scattering nanoprobe, Anal. Chem., 2014, 86, 11614–11619 CrossRef CAS PubMed
.
- G. Lu, H. Li, C. Liusman, Z. Yin, S. Wu and H. Zhang, Surface enhanced Raman scattering of Ag or Au nanoparticle-decorated reduced graphene oxide for detection of aromatic molecules, Chem. Sci., 2011, 2, 1817–1821 RSC
.
- M. Wu, P. Li, Q. Zhu, M. Wu, H. Li and F. Lu, Functional paper-based SERS substrate for rapid and sensitive detection of Sudan dyes in herbal medicine, Spectrochim. Acta, Part A, 2018, 196, 110–116 CrossRef CAS PubMed
.
- E. S. J. Harris, S. Cao, B. A. Littlefield, J. A. Craycroft, R. Scholten, T. Kaptchuk, Y. Fu, W. Wang, Y. Liu, H. Chen, Z. Zhao, J. Clardy, A. D. Woolf and D. M. Eisenberg, Heavy metal and pesticide content in commonly prescribed individual raw Chinese herbal medicines, Sci. Total Environ., 2011, 409, 4297–4305 CrossRef CAS PubMed
.
- L. Chen, F. Song, Z. Liu, Z. Zheng, J. Xing and S. Liu, Multi-residue method for fast determination of pesticide residues in plants used in traditional Chinese medicine by ultra-high-performance liquid chromatography coupled to tandem mass spectrometry, J. Chromatogr., A, 2012, 1225, 132–140 CrossRef CAS PubMed
.
- Z. Jiang, N. Xu, B. Liu, L. Zhou, J. Wang, C. Wang, B. Dai and W. Xiong, Metal concentrations and risk assessment in water, sediment and economic fish species with various habitat preferences and trophic guilds from Lake Caizi, Southeast China, Ecotoxicol. Environ. Saf., 2018, 157, 1–8 CrossRef CAS PubMed
.
- M.-T. Chen, Y.-H. Hsu, T.-S. Wang and S.-W. Chien, Mycotoxin monitoring for commercial foodstuffs in Taiwan, J. Food Drug Anal., 2016, 24, 147–156 CrossRef CAS PubMed
.
- S. Fan, P. Zhao, C. Yu, C. Pan and X. Li, Simultaneous determination of 36 pesticide residues in spinach and cauliflower by LC-MS/MS using multi-walled carbon nanotubes-based dispersive solid-phase clean-up, Food Addit. Contam.: Part A, 2014, 31, 73–82 CrossRef CAS PubMed
.
- L. Liu, Y. Jiang, L. Yong, B. Hu, L. Zhu, Y. Wang, C. Li, Z. Feng, D. Zhou and C. Sun, MWCNTs-solid phase extraction combined with ultra-high performance liquid chromatography-tandem mass spectrometry for the determination of eleven organophosphorus pesticides in river water, Int. J. Environ. Anal. Chem., 2018, 98, 743–757 CrossRef CAS
.
- H. Zhang, P. Nie, Z. Xia, X. Feng, X. Liu and Y. He, Rapid quantitative detection of deltamethrin in Corydalis yanhusuo by SERS coupled with Multi-Walled Carbon Nanotubes, Molecules, 2020, 25, 4081 CrossRef CAS PubMed
.
- C. Song, B. Yang, Y. Yang and L. Wang, SERS-based mercury ion detections: principles, strategies and recent advances, Sci. China: Chem., 2016, 59, 16–29 CrossRef CAS
.
- S. S. R. Dasary, Y. K. Jones, S. L. Barnes, P. C. Ray and A. K. Singh, Alizarin dye based ultrasensitive plasmonic SERS probe for trace level cadmium detection in drinking water, Sens. Actuators, B, 2016, 224, 65–72 CrossRef CAS PubMed
.
- J. Zhu, B. Wang, P. Yang, J. Li, G. Xiao, J. Yao, X. Gong, J. Yan and H. Zhang, The functional Fe3O4@SiO2@AuNPs SERS nanomaterials for rapid enrichment and detection of mercury ions in Licorice, Chemosensors, 2022, 10, 403 CrossRef CAS
.
- J. W. Bennett and M. Klich, Mycotoxins, Clin. Microbiol. Rev., 2003, 16, 497–516 CrossRef CAS PubMed
.
- S. Bräse, A. Encinas, J. Keck and C. F. Nising, Chemistry and biology of mycotoxins and related fungal metabolites, Chem. Rev., 2009, 109, 3903–3990 CrossRef
.
- B. Lin, P. Kannan, B. Qiu, Z. Lin and L. Guo, On-spot surface enhanced Raman scattering detection of aflatoxin B1 in peanut extracts using gold nanobipyramids evenly trapped into the AAO nanoholes, Food Chem., 2020, 307, 125528 CrossRef CAS PubMed
.
- J. Ko, C. Lee and J. Choo, Highly sensitive SERS-based immunoassay of Aflatoxin B1 using silica-encapsulated hollow gold nanoparticles, J. Hazard. Mater., 2015, 285, 11–17 CrossRef CAS PubMed
.
- Z. Wu, D.-W. Sun, H. Pu, Q. Wei and X. Lin, Ti3C2Tx MXenes loaded with Au nanoparticle dimers as a surface-enhanced Raman scattering aptasensor for AFB1 detection, Food Chem., 2022, 372, 131293 CrossRef CAS PubMed
.
- Q. Chen, M. Yang, X. Yang, H. Li, Z. Guo and M. H. Rahma, A large Raman scattering cross-section molecular embedded SERS aptasensor for ultrasensitive Aflatoxin B1 detection using CS-Fe3O4 for signal enrichment, Spectrochim. Acta, Part A, 2018, 189, 147–153 CrossRef CAS PubMed
.
- M. Yang, G. Liu, H. M. Mehedi, Q. Ouyang and Q. Chen, A universal SERS aptasensor based on DTNB labeled GNTs/Ag core-shell nanotriangle and CS-Fe3O4 magnetic-bead trace detection of Aflatoxin B1, Anal. Chim. Acta, 2017, 986, 122–130 CrossRef CAS
.
- A. Li, L. Tang, D. Song, S. Song, W. Ma, L. Xu, H. Kuang, X. Wu, L. Liu, X. Chen and C. Xu, A SERS-active sensor based on heterogeneous gold nanostar core–silver nanoparticle satellite assemblies for ultrasensitive detection of aflatoxinB1, Nanoscale, 2016, 8, 1873–1878 RSC
.
- P. Chen, C. Li, X. Ma, Z. Wang and Y. Zhang, A surface-enhanced Raman scattering aptasensor for ratiometric detection of aflatoxin B1 based on graphene oxide-Au@Ag core-shell nanoparticles complex, Food Control, 2022, 134, 108748 CrossRef CAS
.
- H. He, D.-W. Sun, H. Pu and L. Huang, Bridging Fe3O4@Au nanoflowers and Au@Ag nanospheres with aptamer for ultrasensitive SERS detection of aflatoxin B1, Food Chem., 2020, 324, 126832 CrossRef CAS PubMed
.
- Y. Han, S.-L. Lei, J.-H. Lu, Y. He, Z.-W. Chen, L. Ren and X. Zhou, Potential use of SERS-assisted theranostic strategy based on Fe3O4/Au cluster/shell nanocomposites for bio-detection, MRI, and magnetic hyperthermia, Mater. Sci. Eng., C, 2016, 64, 199–207 CrossRef CAS PubMed
.
-
Z.-H. Sun, Coronary CT Angiography in the Quantitative Analysis of Coronary Plaques, World Scientific, 2015, pp. 7–35 Search PubMed
.
- C. Han, W. Zhai, Y. Wang, J. Cao and M. Wang, A SERS aptasensor for rapid detection of Aflatoxin B1 in coix seed using satellite structured Fe3O4@Au nanocomposites, Food Control, 2022, 142, 109228 CrossRef CAS
.
- H. Shi, H. Wang, X. Meng, R. Chen, Y. Zhang, Y. Su and Y. He, Setting up a surface-enhanced Raman scattering database for artificial-intelligence-based label-free discrimination of tumor suppressor genes, Anal. Chem., 2018, 90, 14216–14221 CrossRef CAS PubMed
.
- F. Lussier, V. Thibault, B. Charron, G. Q. Wallace and J.-F. Masson, Deep learning and artificial intelligence methods for Raman and surface-enhanced Raman scattering, TrAC, Trends Anal. Chem., 2020, 124, 115796 CrossRef CAS
.
- N. Kim, M. R. Thomas, M. S. Bergholt, I. J. Pence, H. Seong, P. Charchar, N. Todorova, A. Nagelkerke, A. Belessiotis-Richards, D. J. Payne, A. Gelmi, I. Yarovsky and M. M. Stevens, Surface enhanced Raman scattering artificial nose for high dimensionality fingerprinting, Nat. Commun., 2020, 11, 207 CrossRef CAS PubMed
.
|
This journal is © The Royal Society of Chemistry 2024 |