DOI:
10.1039/D1AN01163D
(Paper)
Analyst, 2021,
146, 6496-6505
SERS and MALDI-TOF MS based plasma exosome profiling for rapid detection of osteosarcoma†
Received
30th June 2021
, Accepted 23rd August 2021
First published on 7th September 2021
Abstract
Osteosarcoma is the most frequent primary bone cancer, particularly among children and adolescents. The early diagnosis of osteosarcoma is significant for timely clinical treatment to reduce the mortality of patients. Exosomes play a significant role in intercellular communication and serve as promising biomarkers in liquid biopsy for the diagnosis and monitoring of tumors. Herein, we report the utility of surface-enhanced Raman scattering (SERS) and matrix-assisted laser desorption/ionization time-of-flight mass spectrometry (MALDI-TOF MS) for rapid identification of osteosarcoma. We firstly profiled the intrinsic SERS signals and MALDI-TOF mass fingerprints of different subgroups of extracellular vesicles (EVs) and the corresponding cells, demonstrating that the SERS signals and MALDI-TOF mass spectra of exosomes from different types of cells were more discriminative compared to those of large and medium EVs and the cells themselves. Then, we characterized plasma-derived exosomes of 15 osteosarcoma patients and 15 healthy volunteers using SERS and MALDI-TOF MS, revealing distinctive biochemical differences in the spectra. We further utilized a data fusion approach to combine the two types of spectroscopic techniques, differentiating osteosarcoma patients from healthy controls with higher precision than either technique. The results reveal that the non-invasive liquid biopsy method using SERS and MALDI-TOF MS fingerprinting of exosomes has great potential for rapid diagnosis of osteosarcoma.
Introduction
Osteosarcoma is the most prevalent primary type of bone cancer, particularly among children and adolescents.1 The current clinical treatment of osteosarcoma is mainly surgery combining neoadjuvant and adjuvant chemotherapy, with which the 5-year survival rate of osteosarcoma patients, especially those at the early stage, has been significantly increased, underlining the importance of early diagnosis of osteosarcoma.2 The clinical diagnosis of osteosarcoma relies on medical history review, physical examination, and plain radiography, together with histological variation.3,4 Nevertheless, histological biopsy is normally invasive, can induce the spread of tumor cells, and suffers from false negative results due to mis-sampling.5,6 Therefore, it is important to develop novel non-invasive and label-free tools for the detection of osteosarcoma.
Liquid biopsy is an emerging diagnostic method for cancer detection and monitoring with the advantages of high efficiency, high sensitivity and low-invasive merits.7 Recently, exosomes have been considered as potential biomarkers in liquid biopsies for disease diagnosis.8 Exosomes are extracellular vesicles (EVs) with the size ranging from 30 to 150 nm and carry proteins, nucleic acids, lipids, etc.9 They can be shed by various cell types10 and are found in different body fluids, such as plasma, urine, saliva, etc.,11,12 playing a significant role in intercellular communication and transfer of biochemical substances.13 It has been reported that exosomes from osteosarcoma can induce immune escape of osteosarcoma cells, promote angiogenesis, growth and invasion of osteosarcoma, and serve as potential biomarkers and are closely related to tumor progression.14–17 To date, various techniques have been developed for exosome analysis, including flow cytometry,18,19 western blotting,20 enzyme-linked immunosorbent assay (ELISA),21etc. These methods can provide subgroup-level characterization of exosomes, but suffer from limitations, such as expensive equipment, long processing time, complicated labeling procedures, and so on. Furthermore, the biomarker-based characterization methods perform targeted analysis that can miss unknown and new biomarkers. In this regard, it is important to develop untargeted profiling methods for exosome characterization.
Surface enhanced Raman spectroscopy (SERS) is one of the most widely employed non-targeted spectroscopic methods with the characteristics of label-free, non-destructive and non-invasive analysis, small volume of sample consumption, and rapid analysis.22–26 It has been widely used for the identification of cancer cells,27,28 cancer biomarkers,29 and pathogens.30 Recently, SERS has been further applied to detect and identify exosomes derived from different cells.22,31,32 Yan et al. developed a SERS-based platform to discriminate exosomes from different biological sources.33 Shin et al. proved that the deep learning-based SERS signal analysis of plasma-derived exosomes can be used for the diagnosis of early-stage lung cancer.34
Matrix-assisted laser desorption/ionization time-of-flight mass spectrometry (MALDI-TOF MS) is another high throughput and non-targeted analysis method, which can provide mass fingerprints of complex samples.35–37 It has been used for the screening and diagnosis of periodontitis,38 COVID-19,39,40 Alzheimer's disease,41 and cancer.42 Recently, MALDI-TOF MS has been further used for exosome profiling to diagnose cancer and monitor cancer progression.43 Zhu et al. reported that MALDI-TOF MS based exosome characterization can be applied for fast detection of melanoma and monitoring of the disease state.43 Nguyen et al. proved platelet factor 4 as a novel exosome biomarker by MALDI-TOF MS analysis of serum-derived exosomes.44 Choi et al. demonstrated EV-derived CD5L identified by MALDI-TOF MS as a potential biomarker for the diagnosis of lung cancer.45
Herein, we demonstrate that SERS and MALDI-TOF MS based profiling of plasma-derived exosomes can be used to detect osteosarcoma (Scheme 1), and we applied, for the first time, a data fusion approach to combine the two types of spectroscopic techniques to differentiate osteosarcoma patients from healthy controls with high precision. We firstly profiled the intrinsic SERS signals and MALDI-TOF mass fingerprints of different subgroups of EVs and the corresponding cells, demonstrating that the SERS signals and MALDI-TOF mass fingerprints of exosomes can better differentiate different types of cells compared to those of large EVs (LEVs), medium EVs (MEVs) and the cells themselves. Then, SERS signals and MALDI-TOF spectra of plasma-derived exosomes from osteosarcoma patients (n = 15) and healthy volunteers (n = 15) were collected. The osteosarcoma patients can be discriminated from the healthy controls by multivariate statistical analyses of the SERS and MALDI-TOF data separately. Combined analyses of SERS signals and MALDI-TOF spectra by the data fusion approach led to more accurate identification of osteosarcoma. The results reveal that SERS and MALDI-TOF fingerprints of exosomes have great potential for clinical diagnosis of osteosarcoma.
 |
| Scheme 1 Schematic illustration of the SERS and MALDI-TOF MS profiling of plasma-derived exosomes for the identification of osteosarcomas. | |
Experimental section
Chemicals
Phosphate buffered saline (1× PBS, pH 7.2–7.4, 0.01 M) and Dulbecco's Modified Eagle's Medium (DMEM) were obtained from Solarbio Science and Technology Co., Ltd (Beijing, China). Fetal bovine serum (FBS) was obtained from Zhejiang Tianhang Biotechnology Co., Ltd (Hangzhou, China). Hydrogen tetrachloroaurate(III) trihydrate (HAuCl4·3H2O, ≥99.9%) was purchased from Sigma-Aldrich (Saint Louis, MO, USA). Phosphotungstic acid (≥99%), sodium citrate (>98%), and trifluoroacetic acid (TFA, ≥99%) were obtained from Adamas Reagent Co., Ltd (Shanghai, China). Cytochrome c from equine heart (≥95%) and myoglobin from equine skeletal muscle (95%–100%) were obtained from Sigma-Aldrich (Saint Louis, MO, USA). Sinapic acid (SA, ≥99%) was purchased from Bruker (Bremen, Germany). Acetonitrile (ACN, ≥99.9%) was obtained from Merck (Darmstadt, Germany). All aqueous solutions were prepared with deionized (DI) water (18.2 MΩ cm) obtained from a Smart-Q DI water system (Hitech pure water technology, Shanghai, China).
Cell culture
HeLa Kyoto EGFP-H2B cells (CLS cell line service, Eppelheim, Germany) and MCF-7 breast cancer cells (ATCC, Manassas, VA) were maintained in DMEM with 10% heat-inactivated FBS and 1% penicillin–streptomycin at 37 °C under a humid atmosphere with 5% CO2. The cells were cultured in a cell culture flask with a confluency of 80%–90% for at least 48 h, carefully washed three times with 1× PBS, and then cultured in serum-free media for another 48 h.
Human plasma sample collection
Blood was collected using a disposable vacuum blood vessel collection tube, kept for 10 min at room temperature, and then subjected to 10 min of centrifugation at 2000g and 10 min at 3500g. Finally, the plasma samples were aliquoted and frozen at −80 °C for further usage.
Isolation of exosomes by ultracentrifugation
Exosomes were separated from the culture medium supernatant using a reported method based on ultracentrifugation (UC),46 as shown in Fig. S1.† In brief, 45 mL of culture supernatant of approximately 4.5 × 107 cells was centrifuged at 800g for 30 min to collect large EVs (LEVs) at 4 °C. Then, the supernatant was centrifuged at 16
000g for 45 min to collect medium EVs (MEVs) at 4 °C. Subsequently, the supernatant was centrifuged for 2 h at 110
000g to collect exosomes. The collected LEVs, MEVs and exosomes were washed with 1× PBS, respectively, and then resuspended in 50 μL of DI water and kept at −80 °C. For each plasma sample, 8 mL of 8× diluted plasma by 1× PBS was centrifuged at 10
000g for 30 min, and then processed by UC at 110
000g twice for exosome isolation as described above. The collected exosomes were resuspended in 100 μL of DI water and frozen at −80 °C.
Characterization of exosomes
A Tecnai G2 20 TWIN transmission electron microscope (TEM) (FEI, Hillsboro, USA) was used to characterize the size and morphology of the collected exosomes. One microliter of LEVs, MEVs or exosomes was deposited on a 200-mesh carbon-coated copper grid, dried for 20 min, negatively stained with 2% phosphotungstic acid for 10 min, dried, and then observed at 200 kV. Exosomes were also characterized using a ZetaView® BASIC Nanoparticle Tracking Video Microscope PMX-120 (Particle Metrix, Meerbusch, Germany) for size distribution and concentration characterization.
Synthesis of AuNPs
One milliliter of 1% HAuCl4 was added to 99 mL of DI water, followed by stirring and heating to boiling. Once the gold solution was refluxing, 1 mL of 1% sodium citrate solution was quickly added. After refluxing for 15 min, the mixture was cooled down to room temperature. The synthesized AuNPs were characterized using a Tecnai G2 20 TWIN TEM (FEI, Hillsboro, USA), a Gemini SEM500 field emission scanning electron microscope (FESEM) (Carl Zeiss AG, Oberkochen, Germany), and a PerkinElmer Lambda750 UV/Vis spectrophotometer (Waltham, Massachusetts, USA).
SERS analysis
The hydrophobic treatment of a silicon wafer was carried out according to reported methods.47,48 Briefly, the silicon wafer was first cleaned and immersed in a piranha solution, then washed with DI water and ethanol, and dried with nitrogen. Subsequently, the silicon wafer was directly immersed in a triethoxy-1H,1H,2H,2H-tridecafluoro-n-octylsilane solution (40 mM) for at least 12 h, followed by washing with ethanol and drying with nitrogen. One milliliter of the synthesized AuNPs was centrifuged at 12
000g for 6 min, and then 995 μL of supernatant was removed. Five microliters of the remaining pellets were re-dispersed under sonication. One microliter of the concentrated AuNPs was dropped onto the silicon wafer, and then the analyte solution was coated on the dried AuNPs.
The SERS signals were recorded using an XploRA Raman spectrometer (HORIBA JobinYvon, Paris, France) at room temperature with an excitation wavelength of 638 nm and a laser power of 2.5 mW. The laser was focused via a 50× objective. The parameters for collecting SERS signals in 530–2000 cm−1 were as follows: an acquisition time of 15 s, 400 μm entrance slit, confocal mode (300 μm pinhole), and a diffraction grating with a groove density of 1200 grooves per mm. The Raman shift was manually calibrated using the LabSpec 6 software (HORIBA) with the Si Raman spectrum as the reference.
MALDI-TOF MS analysis
MALDI-TOF MS analyses were performed with a Microflex LRF MALDI-TOF mass spectrometer (Bruker, Bremen, Germany) equipped with a pulsed nitrogen laser (λ = 337 nm). The SA matrix solution (20 mg mL−1) was prepared in 50% ACN/49.9% water/0.1% TFA v/v/v. One microliter of exosomes was spotted on a MALDI steel plate (Bruker, Bremen, Germany) and dried under ambient conditions followed by the addition of 1 μL of SA matrix. After the matrix was dried at room temperature, MALDI-TOF MS analysis was performed in the positive linear mode. The optimized parameters were as follows: 70% laser intensity, laser attenuator with 35% offset and 40% range, accumulation of 500 laser shots, 10.3× detector gain, and 150 ns delayed extraction time. External mass calibration was performed using the standard calibration mixture of cytochrome c (2 mg mL−1) and myoglobin (2 mg mL−1).
Statistical analysis of SERS and MALDI-TOF MS data
All the SERS raw spectra were processed with baseline correction and Savitsky–Golay smoothing using the NGSLabSpec software (HORIBA JobinYvon, Paris, France). Second derivative spectra from 2000 to 530 cm−1 were calculated using OriginPro 2018 (OriginLab, Northampton, MA, USA).
The raw data of MALDI-TOF spectra were converted to text files (.txt) using Bruker Daltonics flexAnalysis (Bruker, Bremen, Germany) and subsequently preprocessed using the R packages MALDIquant and MALDIquantForeign.49 The mass range was 2–20 kDa. Then, statistics-sensitive non-linear iterative peak-clipping (SNIP) baseline correction was applied, followed by Savitzky–Golay smoothing and square root transformation. The warpMassSpectra function was used for the mass value alignment. The signal-to-noise ratio of peak detection was set to 3 and the half window size was set to 20. Peaks were removed with the binPeaks function with a tolerance of 0.002. Finally, a peak intensity matrix with the m/z values and intensity of peaks normalized by the sum intensity of the corresponding mass spectrum was obtained.
Multivariate statistical analyses, including data transformation (log
2 transformation and autoscaling), principal component analysis (PCA), partial least-squares discriminant analysis (PLS-DA), hierarchical clustering, receiver operating characteristic (ROC) curve analysis, and ROC curve based model evaluation (Tester), were performed using MetaboAnalyst 5.0 (McGill University, Montreal, Canada, https://www.metaboanalyst.ca/).50 The cosine correlation51 was calculated using R packages and plotted using GraphPad Prism 6 (GraphPad Software, California, USA). The confusion matrix was generated and plotted using Python 3.9.6 (Python Software Foundation, Delaware, USA) according to actual values and the predicted values from model evaluation.
Ethical statement
All experiments were performed in compliance with the guideline “Ethical Review of Biomedical Research Involving Humans” as the China national guideline, and approved by the ethics committee at Ruijin Hospital affiliated to Shanghai Jiao Tong University School of Medicine (Reference Number: 2017-LLS-No.13). The subjects gave their informed consent for using the biological material for research purpose.
Results and discussion
SERS and MALDI-TOF profiling of exosomes to discriminate different cells
To test the feasibility of SERS and MALDI-TOF profiling of exosomes for cancer detection, exosomes from different types of cells were firstly analyzed. HeLa is a cell line of cervical cancer cells, and MCF-7 is a cell line of breast cancer cells. LEVs, MEVs and exosomes were isolated from HeLa and MCF-7 cell culture supernatants by differential centrifugation52 and then characterized by TEM. As shown in Fig. 1A, TEM micrographs showed that the EVs were characteristic cup-shaped vesicles and the size distribution of EV subpopulations can be estimated. The LEVs presented a diameter of ∼500 nm; the MEVs presented a diameter of ∼200 nm; while the exosomes presented a diameter of ∼100 nm. Fig. S2† shows the NTA measurement results of the HeLa and MCF-7 exosomes. The particle sizes of HeLa and MCF-7 exosomes were 141.1 ± 59.8 (mean ± standard deviation, similarly hereinafter) and 137.2 ± 46.0 nm, respectively, and the mean concentrations were (1.41 ± 0.01) × 1011 and (8.23 ± 0.06) × 1010 particles per mL, respectively. By characterizing the concentration of total proteins of the purified exosomes by the BCA method,53 the purities of the isolated HeLa and MCF-7 exosomes were found to be (9.14 ± 0.06) × 1011 and (3.30 ± 0.02) × 1011 particles per milligram protein, respectively.54,55 The above results indicated that the EV subpopulations were successfully isolated from the cell supernatant.
 |
| Fig. 1 TEM images, SERS signals and MALDI-TOF mass spectra of different EV subpopulations derived from HeLa and MCF-7 cells. (A) TEM images of LEVs, MEVs and exosomes. Representative SERS signals of LEVs (purple curve), MEVs (blue curve) and exosomes (green curve) derived from (B) HeLa cells, (C) MCF-7 cells, and the cells themselves (orange curve). Representative MALDI-TOF spectra of LEVs (purple curve), MEVs (blue curve) and exosomes (green curve) derived from (D) HeLa cells, (E) MCF-7 cells, and the cells themselves (orange curve). | |
To further analyze EV subpopulations, an aggregated AuNP substrate was prepared for SERS detection. As shown in Fig. S3A, S3C and S3D,† the SEM image, TEM image and particle size distribution evidenced that the average diameter of AuNPs was ∼50 nm. The UV-vis absorption spectrum of AuNPs showed the surface plasmon resonance (SPR) of the AuNPs, and a sharp peak at 529 nm was obtained (Fig. S3B†). The aggregated AuNPs can generate enhanced SERS hotspots in the nanogap between AuNPs to enhance SERS signals. The EV subpopulation sample was dropped on the AuNPs, and then dried under ambient conditions for SERS analysis as illustrated in Fig. S4.† The characteristic SERS fingerprints of different EV subpopulations derived from HeLa and MCF-7 cells are shown in Fig. 1B and C, presenting different SERS features compared to cells as controls. Further control experiments were also performed on the cell supernatants, culture medium and bare substrate of AuNPs as shown in Fig. S5,† demonstrating that the SERS features were not from the cell culture agents nor the substrates. The characteristic MALDI-TOF mass spectra of different EV subpopulations derived from HeLa and MCF-7 cells as well as the cells themselves were also collected, in the mass range of m/z 2000–20
000, as shown in Fig. 1D and E. It is obvious that the mass spectral features among different EV subpopulations and the cells were also different.
The SERS signals and MALDI-TOF spectra of the same EV subpopulation derived from HeLa and MCF-7 culture supernatants and the cells were analyzed. It was found that the SERS and MALDI-TOF features of HeLa cells and HeLa exosomes were clearly different from those of MCF-7 cells and MCF-7 exosomes, as shown in Fig. 1B, C, D, and E. However, the SERS and MALDI-TOF features of HeLa LEVs and HeLa MEVs were very similar to those of MCF-7 LEVs and MCF-7 MEVs, respectively, as shown in Fig. 1B, C, D, and E. Subsequently, the SERS signals (second derivative) and MALDI-TOF mass spectra of cells and different EV subpopulations were analyzed by PCA. As shown in Fig. 2A, D, E, and H, the SERS signals and MALDI-TOF spectra of different exosomes and cells can be grouped into two sets and distinguished by PCA. In contrast, the SERS signals and MALDI-TOF spectra of LEVs and MEVs derived from different cells cannot be well distinguished, as shown in Fig. 2B, C, F, and G. All the results showed that SERS and MALDI-TOF profiling of exosomes can well discriminate different cell origins, better than those of MEVs, LEVs and the cells themselves.
 |
| Fig. 2 PCA of SERS data of (A) exosomes, (B) MEVs, (C) LEVs, and (D) cells from HeLa cells and MCF-7 cells visualized in a score plot. PCA of MALDI-TOF data of (E) exosomes, (F) MEVs, (G) LEVs, and (H) cells from HeLa cells and MCF-7 cells visualized in a score plot. | |
Identification of osteosarcoma by SERS profiling of exosomes
Motivated by the successful discrimination of different cell types, SERS profiling of exosomes was further applied to clinical samples. Plasma-derived exosomes were isolated from 15 healthy volunteers and 15 osteosarcoma patients, and then characterized by TEM and NTA. The TEM micrographs in Fig. 3A and B show that the exosomes were typical cup-shaped in accordance with the previous report.56 As shown in Fig. S6A and S6B,† the exosomes derived from the healthy controls and the osteosarcoma patients had a uniform size distribution with an average diameter of around 149.0 ± 4.1 nm and 139.3 ± 0.8 nm, respectively. NTA results revealed that the amounts of exosomes derived from the healthy control and the osteosarcoma patient per milliliter plasma were (7.26 ± 0.55) × 109 particles and (1.50 ± 0.10) × 1010 particles, respectively, as shown in Fig. S7A.† By characterizing the concentration of total proteins of the purified exosomes by the BCA method,53 the purities of exosomes isolated from the healthy control and the osteosarcoma patient were found to be (1.59 ± 0.12) × 1010 and (6.24 ± 0.42) × 109 particles per milligram protein, respectively, as shown in Fig. S7B.†
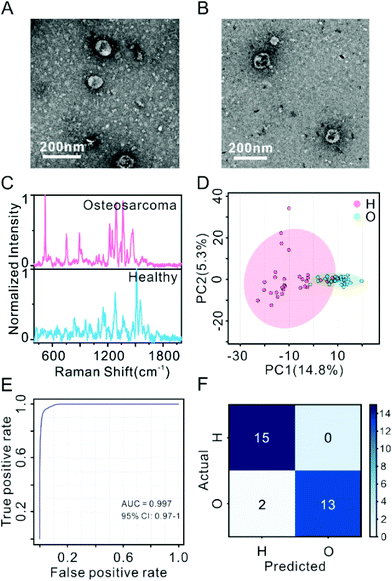 |
| Fig. 3 TEM micrographs of exosomes derived from (A) an osteosarcoma patient and (B) a healthy control. (C) SERS signals of plasma-derived exosomes in the range of 530–2000 cm−1 from an osteosarcoma patient and a healthy control. (D) PCA score plot of SERS data of plasma-derived exosomes from 10 healthy controls (H) and 10 osteosarcoma patients (O) in the training dataset, with three replicates for each sample. (E) ROC analysis with cross-validation on the training data using classification models built by PLS-DA. (F) Confusion matrix of the classification results of another 5 healthy controls (H) and 5 osteosarcoma patients (O) with three replicates for each sample. The classification model was built by PLS-DA using all the training data. | |
Fig. 3C shows the representative SERS fingerprints of plasma-derived exosomes (1.5 × 1010 particles) from an osteosarcoma patient and a healthy control. The SERS fingerprints of the exosomes from the healthy control and the osteosarcoma patient showed strong identical and differential Raman peaks in the range of 530 to 2000 cm−1, originating from proteins (amide I 1600–1690 cm−1), DNA, and RNA (720–820 cm−1).57,58 The assignments of the major SERS peaks are shown in Table S1.† The identical peaks that appeared in all clinical samples were located at 668 cm−1 (T, G, in nucleic acids), 1004 cm−1 (phenylalanine), 1101 cm−1 (phosphodioxy PO2−sym-stretching, in nucleic acids), 1219 cm−1 (U, C ring, sugar puckering, in nucleic acids), 1244 cm−1 (amide III β-sheet, in proteins), 1336 cm−1 (CH2, CH3 twisting, in proteins), 1362 cm−1 (pyrimidine and imidazole rings, in nucleic acids), 1402 cm−1 (deformation CH3asym; COO− stretching), 1465 cm−1 (C–H deformation), and 1655 cm−1 (amide I). Control experiments were performed on EV-free plasma and the bare substrate of AuNPs as shown in Fig. S8.†
The 30 clinical samples were randomly divided into a training dataset (10 patients + 10 controls) and a validation dataset (5 + 5). Three replicated SERS fingerprints were collected from each sample. Thirty SERS fingerprints of exosomes from 10 osteosarcoma patients are shown in Fig. S9A.† Fig. S9B† shows the cosine correlation between SERS fingerprints from the technique replicates, and the cosine correlation between fingerprints from different clinical samples but in the same group (osteosarcoma patients or healthy controls). The results demonstrated the good reproducibility of the SERS analyses. The second derivative of SERS data of the exosomes was subjected to multivariate statistical analyses. Firstly, the SERS data of the training set were analyzed by PCA and hierarchical clustering. As shown in Fig. 3D, the SERS signals of exosomes from osteosarcoma patients cannot be completely distinguished from the healthy controls. For hierarchical clustering analysis, the data of one osteosarcoma patient were wrongly clustered, as shown in Fig. S10.†
PLS-DA was performed on the SERS data of plasma-derived exosomes from the 10 osteosarcoma patients and the 10 healthy controls in the training dataset, as shown in Fig. S11A.† Fig. S11B† shows the variable importance for the projection (VIP) values of the top 15 features. There were 133 features with the VIP value >1.5. Receiver operating characteristic (ROC) analysis with 10 times cross-validation was performed on the training data (80% for modeling and 20% for validation) to demonstrate the feasibility of using the method to identify osteosarcoma patients. The area under the curve (AUC) value of the ROC analysis was 0.997 (Fig. 3E). Then, a classification model was built by PLS-DA based on all the training data with the significant features (VIP > 1.5) and applied to the validation data. As shown in the confusion matrix in Fig. 3F, two samples in the validation dataset were misclassified. The sensitivity, specificity, and accuracy in the detection of osteosarcoma were 86%, 100% and 93%, respectively. The result demonstrated that SERS based plasma-derived exosome profiling is promising as a method in the diagnosis of osteosarcoma.
Identification of osteosarcoma by MALDI-TOF profiling of exosomes
MALDI-TOF MS profiling of exosomes (1.5 × 1010 particles) was further applied to the identification of osteosarcoma. The representative MALDI-TOF spectra of plasma-derived exosomes from an osteosarcoma patient and a healthy control are shown in Fig. 4A. They were significantly different in the mass range of m/z 2000 to 20
000. Same as the SERS analysis, three replicated MALDI-TOF spectra were collected from each sample. Thirty MALDI-TOF spectra of exosomes from 10 osteosarcoma patients are shown in Fig. S12A.† Fig. S12B† shows the cosine correlation between MALDI-TOF spectra from the technique replicates and the cosine correlation between spectra from different clinical samples but in the same group. The results also demonstrated good reproducibility of the MALDI-TOF analyses. The MALDI-TOF mass spectra of plasma-derived exosomes were then subjected to multivariate statistical analyses. By PCA, the mass spectra of plasma-derived exosomes from the 10 osteosarcoma patients and the 10 healthy controls in the training dataset can be separated into two groups, as shown in Fig. 4B. The hierarchical clustering result in Fig. S13† evidenced that the osteosarcoma patients can be discriminated from the healthy controls based on the MALDI-TOF mass spectra.
 |
| Fig. 4 (A) MALDI-TOF mass spectra of plasma-derived exosomes in the mass range of m/z 2000–20 000 from an osteosarcoma patient and a healthy control. (B) PCA score plot of the MALDI-TOF data of plasma-derived exosomes from 10 healthy controls (H) and 10 osteosarcoma patients (O) in the training dataset, with three replicates for each sample. (C) ROC analysis with cross-validation on the training data using classification models built by PLS-DA. (D) Confusion matrix of the classification results of another 5 healthy controls (H) and 5 osteosarcoma patients (O) with three replicates for each sample. The classification model was built by PLS-DA using all the training data. | |
PLS-DA was performed on the MALDI-TOF MS data of the training set, as shown in Fig. S14A.† Fig. S14B† shows the VIP values of the top 15 features. There were 35 features with the VIP value >1.5. The ROC analysis with 10 times cross-validation on the training data (80% for modeling and 20% for validation) showed an AUC value of 1.0 (Fig. 4C). With the classification model built from all the training data by PLS-DA (VIP > 1.5), all samples in the validation dataset were correctly classified, as shown in the confusion matrix in Fig. 4D. The sensitivity, specificity, and accuracy in the detection of osteosarcoma were all 100% with the validation dataset. The results strongly demonstrated the potential of MALDI-TOF MS profiling of plasma-derived exosomes for the diagnosis of osteosarcoma.
Identification of osteosarcoma by combining SERS and MALDI-TOF profiling of exosomes
To increase the information content of plasma-derived exosomes, a data fusion approach was utilized to combine the SERS data with the MALDI-TOF MS data. First, the data of SERS and MALDI-TOF were preprocessed and normalized to the total spectral intensity, separately. Then the data obtained by the two methods were concatenated for a joint analysis with the workflow shown in Fig. 5A. By PCA, the spectral data in the training set can be separated into two groups as shown in Fig. 5B. Then, a classification model was built by PLS-DA based on all the training data with the significant features (VIP > 1.5). All samples in the test dataset were correctly classified as shown in the confusion matrix in Fig. 5C. The sensitivity, specificity, and accuracy in the detection of osteosarcoma were all 100% with the validation dataset. The PCs account for 20.1% for the PCA on SERS spectra, 33.4% for the PCA on MALDI-TOF spectra, and 55.5% on the combined spectra (Fig. 3D, 4B and 5B). The results show that the data fusion approach is more concrete than either technique at profiling plasma-derived exosomes for the identification of osteosarcoma.
 |
| Fig. 5 (A) Analysis workflow combining SERS and MALDI-TOF data by a data fusion approach. (B) PCA score plot of the fusion data of plasma-derived exosomes from 10 healthy controls (H) and 10 osteosarcoma patients (O). Three replicates were performed for each sample. (C) Confusion matrix of the classification results of another 5 healthy controls (H) and 5 osteosarcoma patients (O) with three replicates for each sample. The classification model was built by PLS-DA using all the training data. | |
SERS and MALDI-TOF MS can provide molecular information of exosomes from different aspects. MALDI-TOF MS is used for profiling the mass fingerprints of proteins, metabolites, and lipids. Because the exosomal lipids are normally present in the mass range of m/z 400–2000 (ref. 59 and 60) and the metabolites in the mass range of <m/z 1000,61 the peaks on MALDI-TOF mass spectra have mainly originated from small proteins and protein fragments. Each peak represents a protein/protein fragment or a group of proteins/protein fragments with closer molecular weights. In contrast, SERS is a non-destructive spectroscopic method, and used for clinical sample analysis to prevent damage.62 The features on SERS have originated from the vibrational modes of functional groups on molecules, including nucleic acids, lipids, amino acids, and other components. By combining the two techniques, a more comprehensive view of the exosomes can be obtained, leading to a higher precision in the identification of osteosarcoma.
Compared to existing methods in the characterization of exosomes, the two fingerprinting methods have the advantages of rapid assay, easy operation, simple sample preparation, label-free process, and low sample consumption; therefore, they are especially promising to be applied to clinical diagnosis. In addition, the SERS substrate is used to enhance the Raman signals of analytes, and MALDI-TOF MS uses the matrix to assist the ionization of analytes. The SERS substrates and matrices are crucial for the profiling of targets. By developing substrates suitable for both SERS and MALDI-TOF MS analysis, the two fingerprinting methods can be successively performed on the same sample to avoid heterogeneity among samples in different analyses and improve the repeatability and reliability of detection.
Conclusions
In summary, we demonstrate that SERS and MALDI-TOF signals of exosomes are different from those of other EV subpopulations derived from the same cells, and different among exosomes from different types of cells. SERS and MALDI-TOF MS can be used to characterize exosomes derived from clinical plasma samples to identify osteosarcoma patients in a label-free manner. By combining the two types of spectroscopic analysis techniques using a data fusion approach, more comprehensive characterization of exosomes can be obtained, resulting in more accurate identification of osteosarcoma patients. Based on a similar principle, it is possible to extend the method to other cancer types in the future.
For future development, optimization of the label-free SERS conditions, e.g. SERS substrate, needs further investigation to ensure desirable robustness and reproducibility in exosome characterization from clinical samples. In addition, MALDI-TOF MS fingerprinting can also be supplemented by proteomics experiments to support peak annotation, protein identification, and biomarker discovery.
Author contributions
Zhenzhen Han: conceptualization, methodology, investigation, and writing – original draft. Jia Yi, Yi Yang, and Dandan Li: investigation and formal analysis. Cheng Peng, Shuping Long and Yuhui Shen: resources. Xinyan Peng: investigation. Baohong Liu: conceptualization and funding acquisition. Liang Qiao: conceptualization, methodology, writing – review & editing, supervision, and funding acquisition.
Conflicts of interest
There are no conflicts to declare.
Acknowledgements
This work was supported by the National Natural Science Foundation of China (22022401, 21934001, 22074022, and 81773298), the Science and Technology Commission of Shanghai Municipality (18441901000 and 17411951900), the Shanghai Municipal Commission of Health and Family Planning (201740139) and the Clinical Research Plan of SHDC (No. SHDC2020CR3078B).
Notes and references
- Y. Wang, J. Wang, H. Hao, M. Cai, S. Wang, J. Ma, Y. Li, C. Mao and S. Zhang, ACS Nano, 2016, 10, 9927–9937 CrossRef CAS PubMed.
- G. Tiram, E. Segal, A. Krivitsky, R. Shreberk-Hassidim, S. Ferber, P. Ofek, T. Udagawa, L. Edry, N. Shomron, M. Roniger, B. Kerem, Y. Shaked, S. Aviel-Ronen, I. Barshack, M. Calderon, R. Haag and R. Satchi-Fainaro, ACS Nano, 2016, 10, 2028–2045 CrossRef CAS PubMed.
- J. Ritter and S. S. Bielack, Ann. Oncol., 2010, 21, vii320–vii325 CrossRef PubMed.
- J. S. Meyer, H. R. Nadel, N. Marina, R. B. Womer, K. L. Brown, J. F. Eary, R. Gorlick, H. E. Grier, R. L. Randall, E. R. Lawlor, S. L. Lessnick, P. J. Schomberg and M. D. Kailo, Pediatr. Blood Cancer, 2008, 51, 163–170 CrossRef PubMed.
- J. Zhang, J. Shi, H. Zhang, Y. Zhu, W. Liu, K. Zhang and Z. Zhang, J. Extracell. Vesicles, 2020, 10, e12025 CAS.
- M. Panzica, U. Luke, P. Mommsen and C. Krettek, Unfallchirurg, 2014, 117, 501–509 CrossRef CAS PubMed.
- B. Martin-Gracia, A. Martin-Barreiro, C. Cuestas-Ayllon, V. Grazu, A. Line, A. Llorente, J. M. Fuente and M. Moros, J. Mater. Chem. B, 2020, 8, 6710–6738 RSC.
- K. R. Chi, Nature, 2016, 532, 269–271 CrossRef CAS PubMed.
- M. Chang, Q. Wang, W. Qin, X. Shi and G. Xu, Anal. Chem., 2020, 92, 15497–15505 CrossRef CAS PubMed.
- D. J. Clark, W. E. Fondrie, Z. Liao, P. I. Hanson, A. Fulton, L. Mao and A. J. Yang, Anal. Chem., 2015, 87, 10462–10469 CrossRef CAS PubMed.
- Q. Yang, L. Cheng, L. Hu, D. Lou, T. Zhang, J. Li, Q. Zhu and F. Liu, Biosens. Bioelectron., 2020, 163, 112290 CrossRef CAS PubMed.
- C. Lasser, V. S. Alikhani, K. Ekstrom, M. Eldh, P. T. Paredes, A. Bossios, M. Sjostrand, S. Gabrielsson, J. Lotvall and H. Valadi, J. Transl. Med., 2011, 9, 9 CrossRef PubMed.
- J. Dai, Y. Su, S. Zhong, L. Cong, B. Liu, J. Yang, Y. Tao, Z. He, C. Chen and Y. Jiang, Signal Transduction Targeted Ther., 2020, 5, 145 CrossRef CAS PubMed.
- E. Torreggiani, L. Roncuzzi, F. Perut, N. Zini and N. Baldini, Int. J. Oncol., 2016, 49, 189–196 CrossRef CAS PubMed.
- X. Mu, R. Agarwal, D. March, A. Rothenberg, C. Voigt, J. Tebbets, J. Huard and K. Weiss, Sarcoma, 2016, 2016, 3758162 CrossRef PubMed.
- M. Chicon-Bosch and O. M. Tirado, Cells, 2020, 9, 241 CrossRef CAS PubMed.
- A. Galardi, M. Colletti, V. Di Paolo, P. Vitullo, L. Antonetti, I. Russo and A. Di Giannatale, Int. J. Mol. Sci., 2019, 20, 4600 CrossRef CAS PubMed.
- D. Choi, L. Montermini, H. Jeong, S. Sharma, B. Meehan and J. Rak, ACS Nano, 2019, 13, 10499–10511 CrossRef CAS PubMed.
- W. Shen, K. Guo, G. B. Adkins, Q. Jiang, Y. Liu, S. Sedano, Y. Duan, W. Yan, S. E. Wang, K. Bergersen, D. Worth, E. H. Wilson and W. Zhong, Angew. Chem., Int. Ed., 2018, 57, 15675–15680 CrossRef CAS PubMed.
- J. Ren, L. Ding, D. Zhang, G. Shi, Q. Xu, S. Shen, Y. Wang, T. Wang and Y. Hou, Theranostics, 2018, 8, 3932–3948 CrossRef CAS PubMed.
- K. Boriachek, M. K. Masud, C. Palma, H.-P. Phan, Y. Yamauchi, M. S. A. Hossain, N.-T. Nguyen, C. Salomon and M. J. A. Shiddiky, Anal. Chem., 2019, 91, 3827–3834 CrossRef CAS PubMed.
- J. Park, M. Hwang, B. Choi, H. Jeong, J. H. Jung, H. K. Kim, S. Hong, J. H. Park and Y. Choi, Anal. Chem., 2017, 89, 6695–6701 CrossRef CAS PubMed.
- Z. Han, H. Liu, J. Meng, L. Yang, J. Liu and J. Liu, Anal. Chem., 2015, 87, 9500–9506 CrossRef CAS PubMed.
- J. Liu, C. Cai, Y. Wang, Y. Liu, L. Huang, T. Tian, Y. Yao, J. Wei, R. Chen, K. Zhang, B. Liu and K. Qian, Adv. Sci., 2020, 7, 1903730 CrossRef CAS PubMed.
- W. Xu, L. Wang, R. Zhang, X. Sun, L. Huang, H. Su, X. Wei, C. C. Chen, J. Lou, H. Dai and K. Qian, Nat. Commun., 2020, 11, 1654 CrossRef CAS PubMed.
- H. Tan, H. Hu, L. Huang and K. Qian, Analyst, 2020, 145, 5699–5712 RSC.
- J. Song, J. Zhou and H. Duan, J. Am. Chem. Soc., 2012, 134, 13458–13469 CrossRef CAS PubMed.
- W. Nam, X. Ren, S. A. S. Tali, P. Ghassemi, I. Kim, M. Agah and W. Zhou, Nano Lett., 2019, 19, 7273–7281 CrossRef CAS PubMed.
- X. X. Zhang, D. Xu, D. Guo, H. X. Han, D. W. Li and W. Ma, Chem. Commun., 2020, 56, 2933–2936 RSC.
- J. Yi, X. Wang, Y. Dai, L. Qiao and B. Liu, Anal. Chem., 2019, 91, 14220–14225 CrossRef CAS PubMed.
- S. Stremersch, M. Marro, B. E. Pinchasik, P. Baatsen, A. Hendrix, S. C. De Smedt, P. Loza-Alvarez, A. G. Skirtach, K. Raemdonck and K. Braeckmans, Small, 2016, 12, 3292–3301 CrossRef CAS PubMed.
- H. Shin, H. Jeong, J. Park, S. Hong and Y. Choi, ACS Sens., 2018, 3, 2637–2643 CrossRef CAS PubMed.
- Z. Yan, S. Dutta, Z. Liu, X. Yu, N. Mesgarzadeh, F. Ji, G. Bitan and Y. H. Xie, ACS Sens., 2019, 4, 488–497 CrossRef CAS PubMed.
- H. Shin, S. Oh, S. Hong, M. Kang, D. Kang, Y. G. Ji, B. H. Choi, K. W. Kang, H. Jeong, Y. Park, S. Hong, H. K. Kim and Y. Choi, ACS Nano, 2020, 14, 5435–5444 CrossRef CAS PubMed.
- W. Xu, J. Lin, M. Gao, Y. Chen, J. Cao, J. Pu, L. Huang, J. Zhao and K. Qian, Adv. Sci., 2020, 7, 2002021 CrossRef CAS PubMed.
- V. Vedarethinam, L. Huang, M. Zhang, H. Su, H. Hu, H. Xia, Y. Liu, B. Wu, X. Wan, J. Shen, L. Xu, W. Liu, J. Ma and K. Qian, Adv. Funct. Mater., 2020, 30, 2002791 CrossRef CAS.
- L. Z. Samarah and A. Vertes, View, 2020, 1, 20200063 CrossRef.
- J. Yi, Y. Shen, Y. Yang, C. Shen, B. Liu, L. Qiao and Y. Wang, Talanta, 2021, 225, 121956 CrossRef CAS PubMed.
- L. Yan, J. Yi, C. Huang, J. Zhang, S. Fu, Z. Li, Q. Lyu, Y. Xu, K. Wang, H. Yang, Q. Ma, X. Cui, L. Qiao, W. Sun and P. Liao, Anal. Chem., 2021, 93, 4782–4787 CrossRef CAS PubMed.
- F. M. Nachtigall, A. Pereira, O. S. Trofymchuk and L. S. Santos, Nat. Biotechnol., 2020, 38, 1168–1173 CrossRef CAS PubMed.
- A. Nakamura, N. Kaneko, V. L. Villemagne, T. Kato, J. Doecke, V. Dore, C. Fowler, Q. X. Li, R. Martins, C. Rowe, T. Tomita, K. Matsuzaki, K. Ishii, K. Ishii, Y. Arahata, S. Iwamoto, K. Ito, K. Tanaka, C. L. Masters and K. Yanagisawa, Nature, 2018, 554, 249–254 CrossRef CAS PubMed.
- K. Li, Y. Pei, Y. Wu, Y. Guo and W. Cui, J. Ovarian Res., 2020, 13, 6 CrossRef PubMed.
- Y. Zhu, H. Pick, N. Gasilova, X. Li, T.-E. Lin, H. P. Laeubli, A. Zippelius, P.-C. Ho and H. H. Girault, Chem, 2019, 5, 1318–1336 CAS.
- H.-Q. Nguyen, D. Lee, Y. Kim, M. Paek, M. Kim, K.-S. Jang, J. Oh, Y.-S. Lee, J. E. Yeon, D. M. Lubman and J. Kim, Anal. Chem., 2019, 91, 13297–13305 CrossRef CAS PubMed.
- E. S. Choi, H. A. Faruque, J. H. Kim, K. J. Kim, J. E. Choi, B. A. Kim, B. Kim, Y. J. Kim, M. H. Woo, J. Y. Park, K. Hur, M. Y. Lee, D. S. Kim, S. Y. Lee and E. Kim, Diagnostics, 2021, 11, 620 CrossRef CAS PubMed.
-
C. Thery, A. Clayton, S. Amigorena and G. Raposo, Curr. Protoc. Cell Biol, 2006, ch. 3, Unit 3.22 Search PubMed.
- Y. Sun, Z. Han, H. Liu, S. He, L. Yang and J. Liu, Nanoscale, 2015, 7, 6619–6626 RSC.
- X. Yan, P. Li, B. Zhou, X. Tang, X. Li, S. Weng, L. Yang and J. Liu, Anal. Chem., 2017, 89, 4875–4881 CrossRef CAS PubMed.
- S. Gibb and K. Strimmer, Bioinformatics, 2012, 28, 2270–2271 CrossRef CAS PubMed.
- J. Chong, O. Soufan, C. Li, I. Caraus, S. Li, G. Bourque, D. S. Wishart and J. Xia, Nucleic Acids Res., 2018, 46, W486–W494 CrossRef CAS PubMed.
- Y. Yang, Y. Lin, Z. Chen, T. Gong, P. Yang, H. Girault, B. Liu and L. Qiao, Anal. Chem., 2017, 89, 12556–12561 CrossRef CAS PubMed.
- R. J. Lobb, M. Becker, S. W. Wen, C. S. Wong, A. P. Wiegmans, A. Leimgruber and A. Moller, J. Extracell. Vesicles, 2015, 4, 27031 CrossRef PubMed.
- D. Wu, J. Yan, X. Shen, Y. Sun, M. Thulin, Y. Cai, L. Wik, Q. Shen, J. Oelrich, X. Qian, K. L. Dubois, K. G. Ronquist, M. Nilsson, U. Landegren and M. Kamali-Moghaddam, Nat. Commun., 2019, 10, 3854 CrossRef PubMed.
- J. Webber and A. Clayton, J. Extracell. Vesicles, 2013, 2, 19861 CrossRef.
- J. Chen, Y. Xu, Y. Lu and W. Xing, Anal. Chem., 2018, 90, 14207–14215 CrossRef CAS PubMed.
- S. Jerez, H. Araya, R. Thaler, M. C. Charlesworth, R. Lopez-Solis, A. M. Kalergis, P. F. Cespedes, A. Dudakovic, G. S. Stein, A. J. van Wijnen and M. Galindo, J. Cell. Biochem., 2017, 118, 351–360 CrossRef CAS PubMed.
- A. Gualerzi, S. A. A. Kooijmans, S. Niada, S. Picciolini, A. T. Brini, G. Camussi and M. Bedoni, J. Extracell. Vesicles, 2019, 8, 1568780 CrossRef CAS PubMed.
- A. Rygula, K. Majzner, K. M. Marzec, A. Kaczor, M. Pilarczyk and M. Baranska, J. Raman Spectrosc., 2013, 44, 1061–1076 CrossRef CAS.
- N. Singhto, A. Vinaiphat and V. Thongboonkerd, Sci. Rep., 2019, 9, 13834 CrossRef PubMed.
- O. Peterka, R. Jirasko, M. Chocholouskova, L. Kuchar, D. Wolrab, R. Hajek, D. Vrana, O. Strouhal, B. Melichar and M. Holcapek, Biochim. Biophys. Acta, Mol. Cell Biol. Lipids, 2020, 1865, 158634 CrossRef CAS PubMed.
- R. Zhang, Q. Qin, B. Liu and L. Qiao, Anal. Chem., 2018, 90, 3863–3870 CrossRef CAS PubMed.
- C.-C. Lin, C.-Y. Lin, C.-J. Kao and C.-H. Hung, Sens. Actuators, B, 2017, 241, 513–521 CrossRef CAS.
Footnote |
† Electronic supplementary information (ESI) available. See DOI: 10.1039/d1an01163d |
|
This journal is © The Royal Society of Chemistry 2021 |
Click here to see how this site uses Cookies. View our privacy policy here.