DOI:
10.1039/D0AN02206C
(Paper)
Analyst, 2021,
146, 2358-2367
Monitoring the neurotransmitter release of human midbrain organoids using a redox cycling microsensor as a novel tool for personalized Parkinson's disease modelling and drug screening†
Received
10th November 2020
, Accepted 10th February 2021
First published on 17th February 2021
Abstract
In this study, we have aimed at developing a novel electrochemical sensing approach capable of detecting dopamine, the main biomarker in Parkinson's disease, within the highly complex cell culture matrix of human midbrain organoids in a non-invasive and label-free manner. With its ability to generate organotypic structures in vitro, induced pluripotent stem cell technology has provided the basis for the development of advanced patient-derived disease models. These include models of the human midbrain, the affected region in the neurodegenerative disorder Parkinson's disease. Up to now, however, the analysis of so-called human midbrain organoids has relied on time-consuming and invasive strategies, incapable of monitoring organoid development. Using a redox-cycling approach in combination with a 3-mercaptopropionic acid self-assembled monolayer modification enabled the increase of sensor selectivity and sensitivity towards dopamine, while simultaneously reducing matrix-mediated interferences. In this work, we demonstrate the ability to detect and monitor even small differences in dopamine release between healthy and Parkinson`s disease-specific midbrain organoids over prolonged cultivation periods, which was additionally verified using liquid chromatography–multiple reaction monitoring mass spectrometry. Furthermore, the detection of a phenotypic rescue in midbrain organoids carrying a pathogenic mutation in leucine-rich repeat kinase 2, upon treatment with the leucine-rich repeat kinase 2 inhibitor II underlines the practical implementability of our sensing approach for drug screening applications as well as personalized disease modelling.
With yet unresolved etiology, Parkinson's disease is the second most common neurodegenerative disease worldwide.1 The heterogeneous disease is characterized by the accumulation of the protein α-synuclein and the loss of dopaminergic neurons within the substantia nigra of the human midbrain. As a consequence of dopamine (DA) depletion within the striatum, Parkinson's disease ultimately leads to a variety of debilitating motor and non-motor symptoms.2 Significant progress has been made to unravel the underlying causes of the disease, most notably through the identification of key risk factors of genetic (e.g. SNCS, GBA, and LRRK2 genes) and environmental origin (e.g. pesticide exposure).3,4 Among the genetic risk factors one of the most common pathogenic modification is the G2019S mutation of the leucine-rich repeat kinase 2 (LRRK2). This mutation has been associated with many hereditary cases as well as sporadic cases of Parkinson's disease, rendering LRRK2 a potential target for novel drug candidates.5 Despite these advancements little is known about the complex and time-dependent interplay of these predisposing risk factors ultimately leading up to the onset of the disease. This gap in knowledge is further reflected by the clinical need for disease-modifying or neuroprotective strategies, which until today remains unmet. The high failure rates of putative drug candidates in clinical trials can be explained at least in part by the inability of current disease models to adequately replicate the hallmarks of the pathology.6,7 While existing animal models have provided valuable information on partial aspects of the disease, they remain mere approximations, incapable of recapitulating the degenerative nature of Parkinson's disease.8 With the emergence of induced pluripotent stem cell (iPSC) technology, however, opportunities to generate more physiologically relevant patient-derived in vitro models, including that of the human midbrain, have opened up.9 Human midbrain organoids (hMOs) represent a promising tool for modelling not only developmental but also degenerative processes of the human midbrain in vitro.10,11 The analysis of hMOs is limited to time-consuming and invasive strategies including immunohistochemistry and PCR. In order to monitor crucial aspects in organoid development such as differentiation, disease onset, and progression, however, the development and establishment of novel non-invasive sensing strategies are imperative.10–13 Due to its involvement in the neurodegenerative disease the catecholamine DA has been considered an ideal biomarker.2,14 Therefore, DA has been investigated with several analytical approaches including chromatography,15,16 spectrometry,17–19 and electrochemistry.20,21 The latter constitutes the most straightforward, rapid, and multiplexable among DA detection strategies.22–28 Electrochemical quantification of DA encompasses several techniques including chronoamperometry, differential pulse voltammetry, as well as fast-scan cyclic voltammetry.20 All these techniques were used to detect the biogenic amine neurotransmitter DA in vivo (e.g. rat brain),27,29 in the human blood, as well as in vitro using two-dimensional cell cultures.25 Although electrochemical analysis techniques are powerful tools for the detection of electroactive molecules such as DA, they have remained severely limited in their applicability for biologically relevant implementations, due to low neurotransmitter concentrations, rapid polymerization of oxidation products, protein fouling as well as the presence of physiological interferences such as ascorbic acid (AA). Due to its high concentrations within the matrix of interest (e.g. cell culture medium, CSF), the negatively charged interferent AA (pH = 7.4) has been of particular interest in the development of DA sensors. To that end several strategies have been developed including the modification of sensor surfaces with negatively charged functional groups (Nafion,30 self-assembled monolayers26,27), providing sterical hindrance by introducing molecularly imprinted polymers28 as well as providing increased specificity through the immobilization of enzymes.29 Among these, electrode modification using self-assembled monolayers of 3-mercaptopropionic acid (MPA) has emerged as an auspicious strategy for DA detection. This approach combines the advantages of self-assembled monolayers namely the ability to functionalize electrode surfaces in a simple, convenient and flexible manner with the ability of MPA to reduce AA interferences and albumin adhesion via the electrostatic force exerted by the negatively charged groups located at the MPA's tail.27 While these approaches have shown to improve sensor selectivity, parallelization, throughput, and automation, required to increase reproducibility, however, remain challenging. Besides surface modifications, different electrode geometries and setups have been considered to increase sensor performance. One of which is redox cycling, a technique wherein diffusive mass transfer is improved by placing two working microelectrodes in close proximity.31,32 In other words, by keeping one microelectrode at an oxidizing potential (generator) and poising the other at a reducing potential (collector), a reversible redox species such as DA, will undergo continuous oxidation to the DA quinone at a generator electrode, followed by diffusion as well as reduction to DA at a nearby collector electrode. Due to the geometrical arrangement of the interdigitated electrodes the diffusion layers of the collector and generator overlap, resulting in an optimized diffusive mass transfer (DA and DA quinone) that will continuously replenish DA at the generator electrode and DA quinone at the collector electrode, ultimately resulting in signal amplification (see Fig. 1).33 Interestingly, so far, published redox cycling-based DA sensors are predominantly operated in simplistic matrices (e.g. TRIS buffer) that do not account for protein fouling and interferences typically encountered in biological applications.
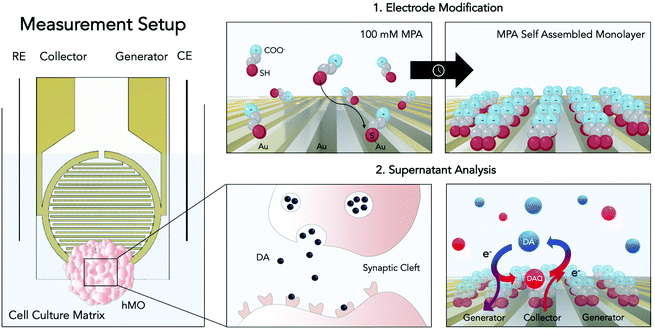 |
| Fig. 1 Schematic of the improved electrochemical dopamine (DA) detection method. On the left panel: the electrochemical set-up consisting of two interdigitated electrodes (generator and collector) with a counter (CE) and a reference electrode (RE). On the right panels: the modification process and its application. By employing thiol-modification a self-assembled monolayer of MPA is created on the gold sensor surface in order to reduce negative interferences (top left and top right panels). Dopaminergic neurons within the hMO secrete DA within the synaptic cleft (bottom left panel). Using redox-cycling on interdigitated and MPA modified gold thin-film electrodes DA can enter a cycle of oxidation (generator electrode) to dopamine quinone (DAQ) and reduction to DA (collector electrode, bottom right panel), enabling its electrochemical detection. The image was generated using Biorender.com. | |
In this study, we have combined the advantages of a redox cycling approach with a sensor surface modification strategy using self-assembled monolayers of 3-mercaptopropionic acid (MPA) to detect DA in complex cell culture matrices. It is important to note that DA, under physiological measurement conditions, is a reactive and unstable molecule. In other words, studying cellular dynamics in a time-resolved manner requires the implementation of rapid in situ detection methods. To demonstrate a broader applicability of our sensing strategy, advanced iPSC-derived three-dimensional hMOs were used to determine distinct differences in DA release between isogenic pairs over prolonged cultivation periods of up to 5 weeks. The results from our electrochemical sensor subsequently were verified by liquid chromatography–multiple reaction monitoring mass spectrometry (LC-MRM-MS). The practical applicability of our sensor was demonstrated by the assessment of personalized treatment options, wherein hMOs were treated with the LRRK2 inhibitor II. We demonstrate that the LRRK2 inhibitor II can elicit phenotypic rescue within hMOs carrying a pathogenic mutation in LRRK2-G2019S.
Methods
Chemicals
3-Mercaptopropionic acid (MPA), DA hydrochloride (DA·HCl), L-DOPA, L-DOPA-(phenyl-d3), DOPAC, γ-aminobutyric acid (GABA), (−)-epinephrine, (−)-norepinephrine, L-ascorbic acid (AA), KNO3, Na2S2O5, EDTA and H2O2 were bought from Sigma-Aldrich (Austria). Potassium hexacyanoferrate(II) trihydrate was bought from Fluka, potassium hexacyanoferrate(III) was bought from Alfa Aesar. KOH was bought from Lachema, absolute ethanol was bought from CL Chemlab. Formic acid LC-MS grade and acetonitrile LC-MS grade were bought from Merck. DI water (18.2 MΩ cm−1) was obtained with a Simplicity UV water purification system (Merck). Phosphate Buffer Saline (PBS) 10X was bought from VWR.
Electrochemical methods
Prior to thiol modification, the interdigitated gold microsensors (Micrux, ED-IDE3-Au, 5 μm gaps and width, 180 pairs of fingers, 3.5 mm ∅ sensing area) were cleaned following a published cleaning protocol by Heiskanen et al.34 Preceding the cleaning process the electrodes were pre-treated by sonication in isopropanol and water for 10 minutes each. Electrodes were then submerged in a mixture of H2O2 (25% v/v) and KOH (50 mM) for 10 minutes, followed by a potential sweep from −200 mV to −1200 mV (vs. Ag/AgCl) in 50 mM KOH. Before electrode modification, the sensors were rinsed in DI water, followed by absolute ethanol. The cleaned sensors were modified with MPA following the published protocol by Spégel et al.26 To that end the microelectrodes were modified by a 2 h incubation process in a 100 mM MPA solution in ethanol and subsequently rinsed with ethanol and water to remove any non-binding thiols. Modified electrodes were stored in perforated centrifuge tubes at room temperature.
MPA electrode modification was confirmed with electrochemical impedance spectroscopy, using a sinusoidal perturbation of 10 mV amplitude, with a 100 mHz–100 kHz scan in a solution of 5 mM potassium hexacyanoferrate(II) and 5 mM potassium hexacyanoferrate(III) in 0.1 M KNO3.
The electrochemical setup was composed of the MPA modified interdigitated sensor containing two comb-like gold working electrodes, with both gaps and band widths of 5 μm. A Pt wire was used as a counter electrode and a chlorinated Ag wire as a pseudo-reference. The 4-electrode electrochemical cell was placed in a homemade Faraday cage and controlled by a potentiostat (VMP3, Bio-Logic) connected in bipotentiostat mode using low current modules (Low current module, Bio-Logic). 20 μL of each sample were applied on the interdigitated circular sensing area. The potential program consisted in applying an oxidizing potential of +0.25 V at one electrode (generator), and a reducing potential of −0.1 V to the other one (collector) for 60 seconds (for potential optimization data see ESI Fig. S2 and 3†). For both electrodes averaged intensities were recorded with an acquisition rate of 0.1 s and the readout was the charge flown in the last 30 seconds. The signals were background subtracted (either PBS for characterization studies or cultivated cell culture medium for hMO analyses). Stock solutions were prepared freshly each day in nitrogen purged solvents (DI water for stocks, PBS or medium for final dilution).
To ensure comparability and reproducibility of the sensitivity within the experimental application of our sensor, we have performed a DA calibration curve in cultivated medium prior to each hMO supernatant analysis (accepted coefficient of variance <
10%). For each measurement the supernatants of three organoids were pooled. Measurements were performed in technical triplicates.
LC-MRM-MS analysis
The supernatant was mixed 1
:
1 with a preservation solution (8 mM Na2S2O5, 2 mM EDTA) and frozen at −80 °C for storage. The frozen samples were thawed on the day of analysis and triplicates of 50 μl were centrifuged at 12
000 rpm for 20 minutes using 3 kDa MWCO centrifuge filters (VWR). The filtrate and the standard dilutions used for calibration were spiked with L-DOPA-(phenyl-d3) as internal standard to reach a constant concentration in the injected samples. Samples were kept in an autosampler at 15 °C and 1 to 5 μl were injected in the UHPLC-MS/MS system (Shimadzu, LCMS-8060), equipped with a C18 column (Waters, Acquity C18 SB, 150 mm length, 1.8 μm particles, 2.1 mm ID, 100 Å pore size), a precolumn cartridge (Waters, Acquity UPLC HSS C18 VanGuard Precolumn, 5 mm length) at 40 °C, using acetonitrile and 0.1% aqueous formic acid as eluents (details see ESI Table S6†). L-DOPA, GABA, epinephrine, norepinephrine, DA and DOPAC were detected and quantified by Multiple Reaction Monitoring (MRM, details for MRM transitions and LODs and LOQs see ESI Tables S7 and 8†). Analyte identification was guaranteed by the presence of at least two analyte-specific MRM transitions. The loss of sample during filter centrifugation was carefully validated and monitored periodically. Based on this validation a correction factor was introduced to take into account sample loss within the final quantitation. The correction factor was calculated by comparing the DA signal of a freshly cultivated cell culture medium with a fresh standard solution in water.
hNESC maintenance, hMO generation and cultivation
Human neuroepithelial stem cell (hNESC, provided by the University of Luxembourg) lines (see Table 1) were derived from human iPSCs as previously described.35
hMO line |
CRISPR/Cas9 |
LRRK2 gene |
Description of the used hMO lines with information on whether they were gene edited (CRISPR/Cas9) and what sequence is found in the LRRK2 gene (healthy (WT) or with the PD related mutation G2019S). For further information, see ESI Table S10.† |
Healthy |
No |
WT |
Healthy-Mut |
Yes |
G2019S |
PD2 |
No |
G2019S |
PD2-GC |
Yes |
WT |
PD1 |
No |
G2019S |
For the generation of hMOs 3000 cells were seeded into each well of an ultra-low attachment round bottom 96-well plate (Greiner). Cells were incubated at 37 °C, 5% CO2. Seeded cells were kept under maintenance conditions (1
:
1 mixture of DMEM-F12 (Sigma Aldrich) and Neurobasal medium (Gibco) supplemented with 1
:
200 N2 supplement (Invitrogen), 1
:
100 B27 supplement lacking vitamin A (Invitrogen), 1% L-glutamine, 1% penicillin/streptomycin (Invitrogen), 3 μM CHIR-99021 (Axon Medchem), 0.5 μM SAG (Merck), 10 μM SB-431542 (Ascent Scientific), 250 nM LDN (Sigma), 5 μM ROCK-inhibitor (Sigma Aldrich) and 200 μM AA (Sigma)) for 7 days. Subsequently, pre-patterning was started by the withdrawal of LDN, ROCK-inhibitor and SB-431542. After 3 days the concentration of CHIR was reduced to 0.7 μM. On day 9 of differentiation, the medium was changed to neuronal maturation medium including 10 μM DAPT, 500 μM dbcAMP, 10 ng mL−1 hBDNF and hGDNF (Peprotech), 1 ng mL−1 TGF-β3 (Peprotech) as well as 2.5 ng mL−1 Activin A (Thermo Fisher Scientific). The organoids were kept under static culture conditions with media changes every other to third day for up to 70 days. Subsequently, hMOs were fixed with 4% PFA at room temperature overnight before being washed with PBS three times. The drug screening experiment was performed by adding the LRRK2 inhibitor II (Sigma-Aldrich) to the cell culture medium (0.5 μM) from differentiation day 7, while the control hMOs were cultured in cell culture medium containing the vehicle (DMSO).
Immunofluorescence
Cultured and fixed hMOs were embedded in a 3% low-melting-point agarose (Biozym) in PBS. Subsequently, 50 μm thick sections were cut using a vibratome (Leica VT1000s) and center-sections were used for assessing TH/FOXA2/TUJ1 expression. Prior to the immunostaining, sections were permeabilized using 0.5% Triton X-100 in PBS. Depending on the antibody, permeabilization times varied between 30 min and 2 h. Unspecific antigen blocking was achieved by incubating cut sections for 2 h in 2.5% donkey serum (Sigma-Aldrich, D9663), 2.5% BSA, 0.1% Triton X-100 and 0.1% sodium azide, followed by primary antibody incubation at 4 °C for 48 h on a shaker. Antibodies were diluted in blocking buffer as follows: rabbit anti-TH (1
:
1000, Abcam), chicken anti-TUJ1 (1
:
600, Millipore). This was followed by the incubation with secondary antibodies diluted in PBS containing 0.01% Triton X-100 and Hoechst-33342 nuclear dye (1
:
1000, Sigma-Aldrich). All secondary antibodies (Invitrogen) were conjugated to Alexa Fluor fluorochromes. Sections were mounted in Fluoromount-G mounting medium (Southern Biotech) and analyzed employing a confocal laser scanning microscope (Zeiss LSM 710).
Results and discussion
Electrochemical characterization of the sensor
To verify successful electrode modification employing the organosulfur compound MPA, electrochemical impedance spectroscopy was employed. Impedance analysis of the MPA-modified interdigitated electrodes resulted in a significant increase of the semicircle radius in the Nyquist plot, with an increase of the charge transfer resistance from 0.9 ± 0.1 kΩ (mean ± SD, n = 3) for the control electrodes up to 3.5 ± 0.3 kΩ for the MPA modified electrodes (see Fig. 2a). This increase in resistance to charge transfer can be attributed to the successful immobilization of the sensor surface with self-assembled monolayers of MPA. In a subsequent experiment the effect of MPA modification on sensor sensitivity and signal stability was assessed. While results depicted in Fig. 2b show that MPA modification has resulted in a lowered DA signal, as previously reported by Tsai et al.,27 a significant reduction in signal drift was observed (ESI Fig. S1 and Table S1†). This improved signal stability points at the elimination of sensor surface fouling events. To evaluate the effects of common interferents, sensor responses to AA, norepinephrine, epinephrine, DOPAC, L-DOPA, and GABA were evaluated.29 To match the concentrations of the respective cell culture medium, AA was set at a concentration of 200 μM. The catecholamines norepinephrine, epinephrine, DA, DOPAC and L-DOPA were analyzed at a concentration of 5 μM, based on the levels of the most abundant catecholamine in the medium: L-DOPA, which was assessed during preliminary LC-MRM-MS studies (data not shown). Similarly, GABA concentration was set at a concentration of 40 μM based on preliminary LC-MRM-MS studies (data not shown). Due to its high concentration within the analyte and its similar redox potential to the catecholamine DA, a particular interest of our interference study was concerned with AA mediated signal contributions. While both DA and AA signals decreased at the generator (see Fig. 2c) after MPA modification, the AA signal was suppressed 5-fold stronger, thus drastically reducing its interference potential. In turn, at the collector electrodes the AA signal contribution was completely eliminated (see Fig. 2d) supporting previous findings which demonstrate rapid hydrolysis of the oxidation product dehydroascorbic acid.31
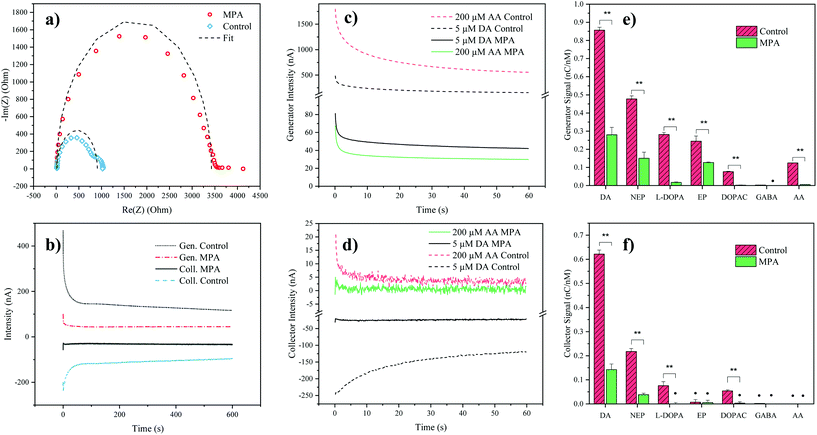 |
| Fig. 2 (a) Nyquist plot of MPA modified and pristine electrodes. Dashed curves represent the fitting done using the Randles circuit; (b) Analysis of 5 μM DA in PBS over time (10 minutes) for MPA modified and control electrodes, including generator (Gen.) and collector (Coll.) signals; (c and d) Generator and collector intensity signals (background subtracted, PBS) of AA and DA, of modified (MPA) and control electrodes, respectively.; (e and f) Generator and collector signals for different biomolecules. (Last 30 seconds charge, background subtracted (PBS) and divided by the concentration of the analyzed solution) DA (DA), norepinephrine (NEP), L-DOPA, epinephrine (EP), DOPAC, GABA and L-ascorbic acid (AA). Error bars represent the propagated standard deviation (n = 3), signals marked with the symbol (•) are not significantly different from the PBS background (α = 0.05, two tailed t-test). | |
While AA enhanced the DA signal at the generator electrode, the presence of AA decreased the DA signal at the collector electrode. This observation can be explained by a previously introduced hypothesis claiming that AA reduces DA-quinone back to DA.31 This means that the DA-quinone reduction, which results in an increase in DA concentration gradient at the generator, enhances signal at the generator, while the depletion of DA-quinone diminishes the signal at the collector (see ESI Fig. S4†). Overall, these results demonstrate improved DA detection at the generator in the presence of MPA-modified electrodes. To further assess the influence and/or interference of other neurotransmitters, background subtracted signals of both generator (see Fig. 2e) and collector electrodes (see Fig. 2f) with and without MPA modification were compared. Similar to the effect seen for AA, MPA modification resulted in significantly (p < 0.01) reduced sensitivities towards the interferents: L-DOPA, DOPAC, norepinephrine and epinephrine.
Notably, a particularly strong signal reduction was observed in the case of negatively charged interferents such as L-DOPA, DOPAC, and AA. This can be explained by the preferential repulsion of anions by MPA, which at physiological pH is negatively charged itself (see Fig. 2e and f).27 While the two catecholamines epinephrine and norepinephrine displayed a similar signal reduction to DA, which can be explained by their similarity in molecular structure and redox potentials, DA nonetheless resulted in the signal with the highest intensity. This observation can be explained by the different intracyclization rates of DA-quinone (kDA = 0.13 ± 0.05 s−1), norepinephrine-quinone (kNEP = 0.98 ± 0.52 s−1), and epinephrine-quinone (kEP = 87 ± 10 s−1).38 In order words, DA displays the highest redox cycling signal while norepinephrine and epinephrine display reduced or silent signals (epinephrine at the collector).
While the MPA modification did not result in the elimination of the signals from both epinephrine and norepinephrine, it has to be noted that these potentially interfering neurotransmitters were undetectable (S/N < 3) in the hMO supernatants, when assessed using LC-MRM-MS. Therefore, the influence of epinephrine and norepinephrine on the overall signal can be considered negligible. While L-DOPA showed a higher selectivity (see Table 2) at the generator electrode, LC-MRM-MS studies of the hMO supernatant detected its presence in the micromolar range. This necessitates considering a potential signal contribution caused by L-DOPA. However, it is important to note that L-DOPA, being the precursor of DA, might reinforce a phenotypic difference when the generator electrode is employed in the comparative assessment of healthy and diseased hMOs. More importantly, selectivity over AA at the generator improved by 702% (see Table 2), which is crucial given its high concentration in the cell culture medium.
Table 2 Biomolecule selectivity to DA
|
Selectivity to DA of MPA sensor (SD), % change compared to unmodified sensor |
Generator |
Collector |
Selectivity was calculated as the ratio of the DA signal (nC/nM) and the signal of each compound listed. Not detected: the compound's signal after electrode modification was not significantly different from background (α = 0.05, n = 3, two-tailed t-test). The percentages indicate the gain or loss in selectivity after the electrode modification. A detailed version of this table is presented in the ESI Table S4.† |
Norepinephrine |
1.9 (0.05), +4% |
3.7 (0.02), +30% |
L-DOPA |
15.8 (0.04), +419% |
Not detected |
Epinephrine |
2.2 (0.04), −37% |
Not detected |
AA |
55 (0.04), +702% |
Not detected |
DOPAC |
115.2 (0.04), +934% |
Not detected |
GABA |
Not detected |
Not detected |
Next, the reproducibility of the sensor and its time stability was assessed. Intra- and inter-batch variability were calculated by analyzing DA sensitivities across three batches prepared on different days, showing acceptable coefficients of variance (CV < 10%, see ESI Table S2†). Time stability was monitored over a period of 12 days showing no significant change (α = 0.05, see ESI Fig. S5†) in DA sensitivity over time. Finally, the effect of long-term storage was evaluated by comparing DA responses of electrodes (n = 3) directly after manufacture and after 20 months of storage, revealing an average loss of 33% sensitivity, while maintaining comparable DA selectivity against AA (mean ± SD, 58 ± 9, see ESI Table S3†). Overall, these results show that the combination of redox cycling and MPA self-assembled monolayers represent a promising strategy for the noninvasive monitoring of hMOs in Parkinson's disease models.
Next, to evaluate sensor performance calibration curves of the catecholamine DA were obtained for MPA-modified electrodes in both PBS as well as cultivated cell culture medium (see ESI Fig. S6†). In both matrices the generator provided higher sensitivity, while the collector electrode allowed for higher selectivity (only DA and norepinephrine could be detected). Calibration in cultured medium resulted in decreased sensitivities (slopes) at both the generator (−80%) and the collector (−89%), as well as reduced linear ranges (see ESI Table S5†), highlighting the significant impact of complex matrices in DA electroanalysis. As previously mentioned, by contributing to the generator's signal, L-DOPA will strengthen a phenotypic difference between healthy and diseased hMOs. Taking this, a higher noise and persistent negative interferences of AA at the collector into consideration, only the generator signal was used for the subsequent application of the sensor in hMO supernatant analysis.
Neurotransmitter profiling using LC-MRM-MS
In order to verify our electrochemical sensor results, an LC-MRM-MS method specific for hMO supernatants was established. In particular, profiles of the neurotransmitters epinephrine, norepinephrine, DA, GABA as well as the DA precursor L-DOPA and the DA metabolite DOPAC were investigated in this study. The method was optimized for analyte detection in cell culture medium with minimum sample preparation to detect and quantify the analytes of interest (chromatographic details see ESI Fig. S7, Tables S6 and S7†). LOQs ranged between 8 nM (epinephrine) and 45 nM (L-DOPA) (details see ESI Table S8†). Intra-day repeatability for hMO supernatant analysis showed acceptable results revealing an averaged coefficient of variation <
10%, see ESI Table S9.† LC-MRM-MS results verified not only the presence of the neurotransmitters GABA, L-DOPA, DA and DOPAC in the supernatant of the analyzed hMOs, but also confirmed the ability of our electrochemical method to detect the presence of the catecholamine DA within the matrix of the tested hMOs (see Table 3).
Table 3 Biomolecule concentrations in the supernatants of hMOs derived from four individual iPSC lines at day 60 of differentiation as measured by LC-MRM-MS
hMO line |
Concentration (nM) (SD) |
L-DOPA |
DA |
DOPAC |
GABA |
Norepinephrine and epinephrine signals were below the respective LODs (35 nM and 320 nM respectively). Standard deviations (SD) were calculated from a technical triplicate (n = 3) of a pooled hMO supernatant (n = 3). For a description of the hMOs lines see Table 1. |
Healthy |
1912.9 (81.5) |
252.3 (6.8) |
1066.5 (58.4) |
55 120 (2826) |
Healthy-Mut |
1661.5 (49.3) |
78.4 (0.3) |
271.1 (1.7) |
45 641 (580) |
PD2 |
1199.5 (93.8) |
40.2 (1.6) |
201 (9.4) |
10 696 (515) |
PD2-GC |
1422.2 (107.5) |
45.3 (3.7) |
259.8 (25.9) |
13 840 (2068) |
Electrochemical analysis of healthy and diseased hMOs
As practical application of our novel sensing strategy in complex biological matrices, supernatants from hMOs derived from five individual iPSC lines (see Table 1) were investigated. In total, iPSC lines from one healthy (Healthy) and two diseased individuals (PD1, PD2) carrying the pathogenic LRRK2-G2019S mutation were used in this study. To provide adequate control lines, the iPSC line from the healthy patient was genetically modified to carry the G2019S mutation in LRRK2 (Healthy-Mut), while the iPSC cell line from patient PD2 was corrected for said mutation (PD2-Mut). To confirm successful hMO maturation immunohistochemical analysis was performed. Characteristic for differentiated hMOs, immunohistochemistry revealed both TUJ1-positive neurons as well as tyrosine hydroxylase (TH) positive neurons in all of the analyzed hMOs (see ESI Fig. S8†).
Moreover, significant differences (p < 0.05, one tail t-tests) in electrochemical sensor signals were obtained for the supernatants of mature hMOs at day 60 of differentiation (see Fig. 3a). Interestingly, differences in the DA levels were observed not only between healthy and diseased organoids but also within the individual isogenic pairs. In other words, the introduction of a single mutation in the LRRK2 gene of healthy organoids resulted in a significant reduction of DA (Fig. 3a Healthy-Mut, −26% signal, p < 0.05), indicating that the pathogenic G2019S mutation alone already causes a reduction in DA biosynthesis, a hallmark of Parkinson's disease. Similarly, the correction for the pathogenic mutation in the diseased organoid (PD2-GC) resulted in a significant (p < 0.05) increase in DA production. Notably, while the gene correction in the diseased organoid increased the sensor signal by 65%, it is still significantly lower compared to the healthy organoid (−34%, p < 0.01), highlighting the multifactorial nature of Parkinson's disease.
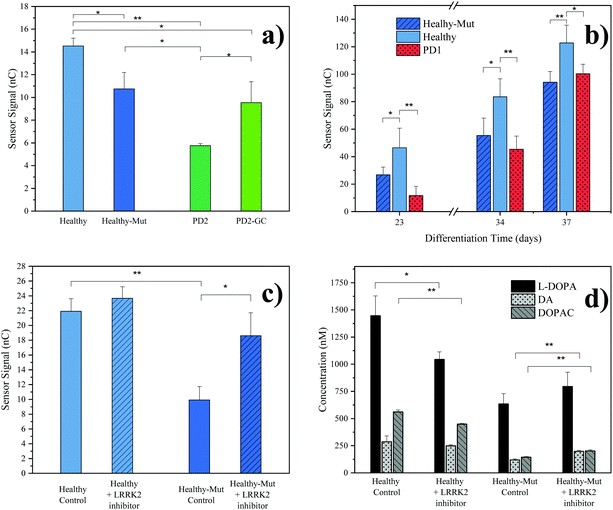 |
| Fig. 3 hMOs supernatant analyses. (a) Comparison of electrochemical results at differentiation day 60 with different hMO lines. Error bars: standard error of the mean (SEM), n = 3, background subtracted signals (cultivated cell culture medium); (b) time resolved monitoring of organoid supernatant. Error bars: SEM, n = 3, background subtracted signals; (c) evaluation of the effect of LRRK2 inhibitor II drug (0.5 μM) on healthy and LRRK2 mutated hMOs (control, DMSO), differentiation day 46. Error bars: SEM, n = 2, background subtracted signals; (d) LC-MRM-MS results of the LRRK2 inhibitor II experiment, differentiation day 46. Error Bars: standard deviation, n = 3. p values for one tailed t-test: * p < 0.05, ** p < 0.01. Presented results were obtained from a pooled hMO supernatant (n = 3). For a description of hMO lines see Table 1. | |
Since our redox-cycling sensor also detects the presence of norepinephrine and epinephrine as well as the DA precursor L-DOPA, albeit to a lesser extent, LC-MRM-MS was employed to verify the obtained electrochemical results in more detail. In a series of experiments, the supernatants of mature hMOs (day 60 of differentiation) were analyzed and listed in Table 3. Similar to the electrochemical sensor, the LC-MRM-MS method showed distinct differences between the individual organoid lines regarding the neurotransmitter DA (e.g. Healthy-Mut, −69%). In addition, LC-MRM-MS analysis revealed a decrease in both L-DOPA (e.g. Healthy-Mut, −13%) and DOPAC (e.g. Healthy-Mut, −75%) levels, while the two catecholamines norepinephrine and epinephrine were undetectable. As such both L-DOPA and DOPAC variations could potentially contribute to the recorded signal at the electrochemical sensor. However, sensor characterization has shown that MPA modification provides good DA selectivity in the presence of the two biomolecules.
In a subsequent experiment, the supernatant of growing organoids was tested over a period of 37 days using our electrochemical sensor (see Fig. 3b). The first neurotransmitter release signal (S/N > 3) was detected as early as day 23 of differentiation, at which point already distinct differences in sensor signals between healthy hMOs (Healthy) and healthy hMOs carrying the mutation in LRRK2 (Healthy-Mut) could be identified. Interestingly, these early individual phenotypic differences were detected over the entire maturation period of 37 days, underlining the ability of the developed sensor for time-resolved monitoring of hMOs.
In a final set of experiments, the potential of our redox-cycling sensing approach for personalized drug screening applications was assessed, by treating healthy (Healthy) and CRISPR/Cas9 mutated (Healthy-Mut) hMOs with the LRRK2 inhibitor II. While no distinctive influence was detected for healthy hMOs, a significant signal increase (+87%) was obtained following the treatment of hMOs carrying the mutation in the LRRK2 (Healthy-Mut) gene (see Fig. 3c), thus indicating a phenotypic rescue in the presence of the LRRK2 inhibitor II. This observation was further confirmed using LC-MRM-MS measurements (Fig. 3d), where significant increases (p < 0.01) in DA (+67%) and DOPAC (+40%) levels were detected when treated with the kinase inhibitor LRRK2 inhibitor II.
In summary, the herein presented redox-cycling approach not only reliably detected differences in the release of the catecholamine DA in between individual hMOs in their complex matrix in a time-resolved manner, but furthermore demonstrated phenotypic rescue upon treatment with the kinase inhibitor LRRK2 inhibitor II. As such it presents a powerful tool for both in vitro disease modelling as well as personalized drug screening applications.
Conclusions
Overall, the presented sensing approach combines the advantages of selective electrode modification with a redox cycling-based signal amplification strategy to detect DA release of hMOs in a complex biological matrix. While the application of a self-assembled 3-mercaptopropionic acid monolayer efficiently repelled negatively charged molecules such as AA, L-DOPA and DOPAC, redox cycling of DA provided stable, reliable and robust current signals. Using this novel combination, for the first time, time-resolved, non-invasive and label-free detection of DA release was conducted in supernatants of healthy and diseased hMOs. It is important to note that no artificial exocytosis trigger was needed for signal detection, thus making our redox cycling sensing approach an ideal and non-invasive screening tool in neurobiology.
While the negatively charged biomolecules such as AA, L-DOPA and DOPAC could successfully be repelled by the negative charge of the MPA modified sensor surface, interference from the two catecholamines epinephrine and norepinephrine was reduced by addressing their difference in intracyclization rate through employing a redox cycling strategy. Although the interference could not be eliminated, it has to be noted that both biomolecules were not detectable in the supernatant of the hMOs and as such can be considered negligible.
Using our sensing method even small differences between isogenic pairs of hMOs were detected, highlighting the influence of individual genetic backgrounds in neurodegenerative diseases. These differences across individual midbrain organoid lines were monitored over a prolonged period of time (e.g. weeks and months), making this approach a potential candidate for the non-invasive monitoring of Parkinson's onset and disease progression in patient-derived samples. Practical application of our technology for personalized drug testing was demonstrated using both healthy and LRRK2-G2019S mutated hMOs. Following treatment with the LRRK2 inhibitor II, phenotypic rescue, expressed by an increase of DA release, was detected only in hMOs carrying the pathogenic mutation in the LRRK2-G2019S gene. In future, we plan to expand our drug screening study to include a larger number of patient-derived hMOs to evaluate different drug-candidates, combinations thereof and dose–response relationships for personalized therapy options in Parkinson's disease.
Associated content
Ethical statement
All experiments were performed in accordance with the national and international ethics guidelines, and approved by the ethics committee at the University of Luxembourg and the national ethics committee (CNER, Comité National d'Ethique de Recherche). Informed consents were obtained from human participants of this study.
Author contributions
CZ, SS, PE, SB, JCS, MR, and JR designed the experiments. CZ, SS, and PE drafted the manuscript. CZ and SS conducted all the experiments and performed data analysis. SB, EB, LS, and JCS provided the hMOs and performed immunohistochemical analyses. PC and MMD established the HPLC-MRM-MS method, performed analyses and analyzed the data.
The manuscript was written through contributions of all authors./All authors have given approval to the final version of the manuscript.
Conflicts of interest
There are no conflicts of interest to declare.
Acknowledgements
CZ wishes to thank Prof. Camilla Ferrante (University of Padova, Italy) for her support. We thank Edis Saini for his support in the acquisition of part of the electrochemical data during his internship at TU Wien.
The JCS's lab is supported by the Fonds National de la Recherche (FNR) Luxembourg in the M-era. Net project NanoPD (INTER/MERA/17/11760144). Further work from the JCS lab was supported by a Proof-of-Concept grant from the Fonds National de la Recherche (FNR) Luxembourg (FNR/PoC16/11559169). Further, this is an EU Joint Program – Neurodegenerative Disease Research (JPND) project (INTER/JPND/15/11092422) receiving funding from the FNR.
References
- D. B. Ramsden, R. B. Parsons, S. L. Ho and R. H. Waring, The aetiology of idiopathic Parkinson's disease, Mol. Pathol., 2001, 54(6), 369–380 CAS.
- J. A. Obeso, M. Stamelou, C. G. Goetz, W. Poewe, A. E. Lang, D. Weintraub, D. Burn, G. M. Halliday, E. Bezard, S. Przedborski, S. Lehericy, D. J. Brooks, J. C. Rothwell, M. Hallett, M. R. DeLong, C. Marras, C. M. Tanner, G. W. Ross, J. W. Langston, C. Klein, V. Bonifati, J. Jankovic, A. M. Lozano, G. Deuschl, H. Bergman, E. Tolosa, M. Rodriguez-Violante, S. Fahn, R. B. Postuma, D. Berg, K. Marek, D. G. Standaert, D. J. Surmeier, C. W. Olanow, J. H. Kordower, P. Calabresi, A. H. V. Schapira and A. J. Stoessl, Past, present, and future of Parkinson's disease: A special essay on the 200th Anniversary of the Shaking Palsy: The Shaking Palsy: Past, Present and Future, Mov. Disord., 2017, 32(9), 1264–1310 CrossRef CAS.
- W. Satake, Y. Nakabayashi, I. Mizuta, Y. Hirota, C. Ito, M. Kubo, T. Kawaguchi, T. Tsunoda, M. Watanabe, A. Takeda, H. Tomiyama, K. Nakashima, K. Hasegawa, F. Obata, T. Yoshikawa, H. Kawakami, S. Sakoda, M. Yamamoto, N. Hattori, M. Murata, Y. Nakamura and T. Toda, Genome-wide association study identifies common variants at four loci as genetic risk factors for Parkinson's disease, Nat. Genet., 2009, 41(12), 1303–1307 CrossRef CAS.
- A. Verstraeten, J. Theuns and C. Van Broeckhoven, Progress in unraveling the genetic etiology of Parkinson disease in a genomic era, Trends Genet., 2015, 31(3), 140–149 CrossRef CAS.
- B. D. Lee, V. L. Dawson and T. M. Dawson, Leucine rich repeat kinase 2 (LRRK2) as a potential therapeutic target for Parkinson's disease, Trends Pharmacol. Sci., 2012, 33(7), 365–373 CrossRef CAS.
- W. Poewe, K. Seppi, C. M. Tanner, G. M. Halliday, P. Brundin, J. Volkmann, A.-E. Schrag and A. E. Lang, Parkinson disease, Nat. Rev. Dis. Primers, 2017, 3, 17013 CrossRef.
- W. G. Meissner, M. Frasier, T. Gasser, C. G. Goetz, A. Lozano, P. Piccini, J. A. Obeso, O. Rascol, A. Schapira, V. Voon, D. M. Weiner, F. Tison and E. Bezard, Priorities in Parkinson's disease research, Nat. Rev. Drug Discovery, 2011, 10(5), 377–393 CrossRef CAS.
- M. Jucker, The benefits and limitations of animal models for translational research in neurodegenerative diseases, Nat. Med., 2010, 16(11), 1210–1214 CrossRef CAS.
- K. Takahashi and S. Yamanaka, Induction of Pluripotent Stem Cells from Mouse Embryonic and Adult Fibroblast Cultures by Defined Factors, Cell, 2006, 126(4), 663–676 CrossRef CAS.
- H. Kim, H. J. Park, H. Choi, Y. Chang, H. Park, J. Shin, J. Kim, C. J. Lengner, Y. K. Lee and J. Kim, Modeling G2019S-LRRK2 Sporadic Parkinson's Disease in 3D Midbrain Organoids, Stem Cell Rep., 2019, 12(3), 518–531 CrossRef CAS.
- L. M. Smits, L. Reinhardt, P. Reinhardt, M. Glatza, A. S. Monzel, N. Stanslowsky, M. D. Rosato-Siri, A. Zanon, P. M. Antony, J. Bellmann, S. M. Nicklas, K. Hemmer, X. Qing, E. Berger, N. Kalmbach, M. Ehrlich, S. Bolognin, A. A. Hicks, F. Wegner, J. L. Sterneckert and J. C. Schwamborn, Modeling Parkinson's disease in midbrain-like organoids, npj Parkinson's Dis., 2019, 5(1), 5 CrossRef.
- J. Jo, Y. Xiao, A. X. Sun, E. Cukuroglu, H.-D. Tran, J. Göke, Z. Y. Tan, T. Y. Saw, C.-P. Tan, H. Lokman, Y. Lee, D. Kim, H. S. Ko, S.-O. Kim, J. H. Park, N.-J. Cho, T. M. Hyde, J. E. Kleinman, J. H. Shin, D. R. Weinberger, E. K. Tan, H. S. Je and H.-H. Ng, Midbrain-like Organoids from Human Pluripotent Stem Cells Contain Functional Dopaminergic and Neuromelanin-Producing Neurons, Cell Stem Cell, 2016, 19(2), 248–257 CrossRef CAS.
- A. S. Monzel, L. M. Smits, K. Hemmer, S. Hachi, E. L. Moreno, T. van Wuellen, J. Jarazo, J. Walter, I. Brüggemann, I. Boussaad, E. Berger, R. M. T. Fleming, S. Bolognin and J. C. Schwamborn, Derivation of Human Midbrain-Specific Organoids from Neuroepithelial Stem Cells, Stem Cell Rep., 2017, 8(5), 1144–1154 CrossRef CAS.
- F. N. Emamzadeh and A. Surguchov, Parkinson's Disease: Biomarkers, Treatment, and Risk Factors, Front. Neurosci., 2018, 12, 612 CrossRef.
- G. Cannazza, M. M. Carrozzo, A. S. Cazzato, I. M. Bretis, L. Troisi, C. Parenti, D. Braghiroli, S. Guiducci and M. Zoli, Simultaneous measurement of adenosine, dopamine, acetylcholine and 5-hydroxytryptamine in cerebral mice microdialysis samples by LC–ESI-MS/MS, J. Pharm. Biomed. Anal., 2012, 71, 183–186 CrossRef CAS.
- G. M. Anderson and J. G. Young, Determination of neurochemically important compounds in physiological samples using HPLC, Schizophr. Bull., 1982, 8(2), 333–348 CrossRef CAS.
- S. S. An, J. Kim and S. R. Ankireddy, Selective detection of dopamine in the presence of ascorbic acid via fluorescence quenching of InP/ZnS quantum dots, Int. J. Nanomed., 2015, 113 CrossRef.
- A. a. Manaf, M. Ghadiry, R. Soltanian, H. Ahmad and C. K. Lai, Picomole Dopamine Detection Using Optical Chips, Plasmonics, 2016, 1–6 Search PubMed.
- M. I. Zibaii, H. Latifi, A. Asadollahi, A. H. Bayat, L. Dargahi and A. Haghparast, Label Free Fiber Optic Apta-Biosensor for In vitro Detection of Dopamine, J. Lightwave Technol., 2016, 34(19), 4516–4524 CAS.
- K. Jackowska and P. Krysinski, New trends in the electrochemical sensing of dopamine, Anal. Bioanal. Chem., 2013, 405(11), 3753–3771 CrossRef CAS.
- E. S. Bucher and R. M. Wightman, Electrochemical Analysis of Neurotransmitters, Annu. Rev. Anal. Chem., 2015, 8(1), 239–261 CrossRef CAS.
- H. Ghadimi, M. R. Mahmoudian and W. J. Basirun, A sensitive dopamine biosensor based on ultra-thin polypyrrole nanosheets decorated with Pt nanoparticles, RSC Adv., 2015, 5(49), 39366–39374 RSC.
- J. Njagi and S. Andreescu, Response to Enzyme-Linked Biosensors: Michaelis−Menten Kinetics Need Not Apply, J. Chem. Educ., 2010, 87(9), 907–907 CrossRef CAS.
- S. J. Park, S. H. Lee, H. Yang, C. S. Park, C.-S. Lee, O. S. Kwon, T. H. Park and J. Jang, Human Dopamine Receptor-Conjugated Multidimensional Conducting Polymer Nanofiber Membrane for Dopamine Detection, ACS Appl. Mater. Interfaces, 2016, 8(42), 28897–28903 CrossRef CAS.
- L. Sasso, A. Heiskanen, F. Diazzi, M. Dimaki, J. Castillo-León, M. Vergani, E. Landini, R. Raiteri, G. Ferrari, M. Carminati, M. Sampietro, W. E. Svendsen and J. Emnéus, Doped overoxidized polypyrrole microelectrodes as sensors for the detection of dopamine released from cell populations, Analyst, 2013, 138(13), 3651 RSC.
- C. Spégel, A. Heiskanen, J. Acklid, A. Wolff, R. Taboryski, J. Emnéus and T. Ruzgas, On-Chip Determination of Dopamine Exocytosis Using Mercaptopropionic Acid Modified Microelectrodes, Electroanalysis, 2007, 19(2–3), 263–271 CrossRef.
- T.-C. Tsai, C.-X. Guo, H.-Z. Han, Y.-T. Li, Y.-Z. Huang, C.-M. Li and J.-J. Jason Chen, Microelectrodes with gold nanoparticles and self-assembled monolayers for in vivo recording of striatal dopamine, Analyst, 2012, 137(12), 2813–2820 RSC.
- C. Xue, Q. Han, Y. Wang, J. Wu, T. Wen, R. Wang, J. Hong, X. Zhou and H. Jiang, Amperometric detection of dopamine in human serumby electrochemical sensor based on gold nanoparticles doped molecularly imprinted polymers, Biosens. Bioelectron., 2013, 49, 199–203 CrossRef CAS.
- J. Njagi, M. M. Chernov, J. C. Leiter and S. Andreescu, Amperometric Detection of Dopamine in Vivo with an Enzyme Based Carbon Fiber Microbiosensor, Anal. Chem., 2010, 82(3), 989–996 CrossRef CAS.
- P. Capella, B. Ghasemzadeh, K. Mitchell and R. N. Adams, Nafion-coated carbon fiber electrodes for neurochemical studies in brain tissue, Electroanalysis, 1990, 2(3), 175–182 CrossRef CAS.
- A. Aggarwal, M. Hu and I. Fritsch, Detection of dopamine in the presence of excess ascorbic acid at physiological concentrations through redox cycling at an unmodified microelectrode array, Anal. Bioanal. Chem., 2013, 405(11), 3859–3869 CrossRef CAS.
- M. Hu and I. Fritsch, Application of Electrochemical Redox Cycling: Toward Differentiation of Dopamine and Norepinephrine, Anal. Chem., 2016, 88(11), 5574–5578 CrossRef CAS.
- P. Tomčík, Microelectrode Arrays with Overlapped Diffusion Layers as Electroanalytical Detectors: Theory and Basic Applications, Sensors, 2013, 13(10), 13659–13684 CrossRef.
- A. R. Heiskanen, C. F. Spégel, N. Kostesha, T. Ruzgas and J. Emnéus, Monitoring of Saccharomyces cerevisiae Cell Proliferation on Thiol-Modified Planar Gold Microelectrodes Using Impedance Spectroscopy, Langmuir, 2008, 24(16), 9066–9073 CrossRef CAS.
- L. M. Smits, L. Reinhardt, P. Reinhardt, M. Glatza, A. S. Monzel, N. Stanslowsky, M. D. Rosato-Siri, A. Zanon, P. M. Antony, J. Bellmann, S. M. Nicklas, K. Hemmer, X. Qing, E. Berger, N. Kalmbach, M. Ehrlich, S. Bolognin, A. A. Hicks, F. Wegner, J. L. Sterneckert and J. C. Schwamborn, Modeling Parkinson’s disease in midbrain-like organoids, npj Parkinson’s Dis., 2019, 5, 5 CrossRef.
- X. Qing, J. Walter, J. Jarazo, J. Arias-Fuenzalida, A.-L. Hillje and J. C. Schwamborn, CRISPR/Cas9 and piggyBac-mediated footprint-free LRRK2-G2019S knock-in reveals neuronal complexity phenotypes and α-Synuclein modulation in dopaminergic neurons, Stem Cell Res., 2017, 24, 44–50 CrossRef CAS.
- P. Reinhardt, M. Glatza, K. Hemmer, Y. Tsytsyura, C. S. Thiel, S. Höing, S. Moritz, J. A. Parga, L. Wagner, J. M. Bruder, G. Wu, B. Schmid, A. Röpke, J. Klingauf, J. C. Schwamborn, T. Gasser, H. R. Schöler and J. Sterneckert, Derivation and Expansion Using Only Small Molecules of Human Neural Progenitors for Neurodegenerative Disease Modeling, PLOS ONE, 2013, 8(3), e59252 CrossRef CAS.
- M. Hu and I. Fritsch, Redox Cycling Behavior of Individual and Binary Mixtures of Catecholamines at Gold Microband Electrode Arrays, Anal. Chem., 2015, 87(4), 2029–2032 CrossRef CAS.
Footnotes |
† Electronic supplementary information (ESI) available: Details on experimental methods and results for: electrochemistry, LC-MRM-MS, cell culture and immunohistochemistry (PDF). See DOI: 10.1039/d0an02206c |
‡ These authors contributed equally. |
§ Current address: Medical University Vienna, Austria |
¶ Current address: Pregenerate GmbH, Vienna, Austria |
|
This journal is © The Royal Society of Chemistry 2021 |