DOI:
10.1039/C6MB00237D
(Paper)
Mol. BioSyst., 2016,
12, 2394-2407
Systems level analysis of the Chlamydomonas reinhardtii metabolic network reveals variability in evolutionary co-conservation†
Received
31st March 2016
, Accepted 14th June 2016
First published on 14th June 2016
Abstract
Metabolic networks, which are mathematical representations of organismal metabolism, are reconstructed to provide computational platforms to guide metabolic engineering experiments and explore fundamental questions on metabolism. Systems level analyses, such as interrogation of phylogenetic relationships within the network, can provide further guidance on the modification of metabolic circuitries. Chlamydomonas reinhardtii, a biofuel relevant green alga that has retained key genes with plant, animal, and protist affinities, serves as an ideal model organism to investigate the interplay between gene function and phylogenetic affinities at multiple organizational levels. Here, using detailed topological and functional analyses, coupled with transcriptomics studies on a metabolic network that we have reconstructed for C. reinhardtii, we show that network connectivity has a significant concordance with the co-conservation of genes; however, a distinction between topological and functional relationships is observable within the network. Dynamic and static modes of co-conservation were defined and observed in a subset of gene-pairs across the network topologically. In contrast, genes with predicted synthetic interactions, or genes involved in coupled reactions, show significant enrichment for both shorter and longer phylogenetic distances. Based on our results, we propose that the metabolic network of C. reinhardtii is assembled with an architecture to minimize phylogenetic profile distances topologically, while it includes an expansion of such distances for functionally interacting genes. This arrangement may increase the robustness of C. reinhardtii's network in dealing with varied environmental challenges that the species may face. The defined evolutionary constraints within the network, which identify important pairings of genes in metabolism, may offer guidance on synthetic biology approaches to optimize the production of desirable metabolites.
Introduction
Cells carry out and regulate their metabolism through an extended network of biochemical reactions. Ecological niches, environmental conditions, and the genetic make-up of organisms impact the organization of metabolic networks.1–5 While morphological complexity of life has increased during evolution, shared metabolic pathways as well as conserved catalysts are readily observable within the different lineages.6–8 Random events such as gene duplication and recombination may contribute to the emergence of new enzymes within pathways6,9 and the expansion of metabolic pathways into functional modules.9,10 However, the conservation, topological positions, and functional roles of newly-emerged enzymes across metabolic networks are not determined by chance alone.6,11–13 For instance, in prokaryotes, a set of non-random, essential, and ancient proteins seem to carry out core metabolic activities.6,14 The expansion of metabolic pathways takes place by addition of homologous enzymes that topologically act in the vicinity of the ancestral ones.9,11 Furthermore, the essential cores of metabolic networks contain most of the structural diversities of the associated genes with respect to fold representation.6 The expanded networks are found to display scale-free network characteristics in all three domains of life,12 where highly connected enzymes evolve more slowly.15–17 Other studies have shown that, in yeast, the phylogenetic distributions of conditionally essential genes are likely to be more restricted.18 These observations point to the multiplicity of selective pressures and constraints on the evolution of metabolic enzymes.
How might the sequence spaces of enzymes be explored by evolution? The idea of adaptive landscapes in evolution was first introduced in the 1930s to conceptualize the evolvability potential of organisms.19 This concept was further developed into coevolution and the dependency of fitness landscapes was mathematically modeled, e.g., using the NK model, describing the changes in the ruggedness of the landscape with respect to dependency on the function of other genes.20–25 In broad terms, the linkage between phylogenetic affiliations and their functional groupings26,27 can be viewed as a consequence of linked fitness landscapes which may be detectable from co-conservation of genes.
In recent years, reconstruction of genome-scale metabolic networks has elucidated the fundamental aspects of metabolic network formation and evolution.1,5,6 Extensive work has been done on studying the architecture of metabolic networks, linking topology, evolution and function of metabolic enzymes. Von Mering et al.28 have shown that a large portion of metabolic enzymes cluster together in a modular fashion within metabolic networks. Such findings have been further corroborated by Zhao et al.29 where they identified a core–periphery modular organization of the network within which the peripheral modules show a more cohesive coevolution as compared to the core pathways. Kanehisa et al.30 have further ascertained the latter finding where they suggested that the core metabolic pathways might have evolved in an individualized fashion, whereas the peripheral or extensions were driven by modular sets of enzymes and reactions.
The evolutionary dynamics of metabolic genes are not characterized in C. reinhardtii and still not fully resolved in any eukaryote, particularly with respect to the relationship with distant lineages. We addressed this gap here by extending the information content of a genome-scale metabolic network that we recently reconstructed.31 We defined evolutionary affinities of the network with 13 major eukaryotic lineages representing most if not all major eukaryotic lineages, some of which reside very distant to C. reinhardtii. We looked at the evolutionary dynamics of gene pairs by distinguishing highly conserved pairs with those that are conserved in a subset of lineages. This information was then integrated with topological and metabolic analyses in conjunction with gene expression data to determine if there is concordance between evolutionary affinities, expression, and functional constraints within the C. reinhardtii network. Furthermore, we carried out interolog analysis to assess the rewiring of the metabolic networks in yeast and Arabidopsis.
Materials and methods
Evolutionary affinity assignments
Evolutionary conservation was assigned by comparing the translated sequence of C. reinhardtii metabolic ORFs with the annotated whole proteomes of the available fully sequenced genomes from 13 lineages representing major eukaryotic lineages (Tables S1, S2 and Method S1, ESI†).
Network transformation
To transform the investigated metabolic network into a protein-centric one, gene-reaction-metabolite association information of the network was used to generate the corresponding protein-centric network. Two enzymes were connected with an edge either if they are co-enzymes or if they have substrate–product relationship (Fig. S1, ESI†). For instance, an edge was extended from enzyme E1 to enzyme E2 if a product of a reaction catalyzed by E1 is a substrate of a reaction catalyzed by E2. The following metabolites were excluded in this construction as currency metabolites: H+, H2O, ATP, Pi, ADP, CoA, NAD, NADH, NADP, NADPH, PPi, O2, AMP, CO2 and NH4.
Co-conservation analyses
To evaluate the evolutionary dynamics between gene products, we first constructed a profile for each gene in the network. The profile for each gene is a vector with a row and 13 values (one for each of the 13 eukaryotic lineages). “1” in the column indicates conservation, while “0” indicates non-conservation. Thus, the profile is a representation of gene conservation/non-conservation status in each of the 13 eukaryotic lineages (Table S3, ESI†). We calculated mutual information (MI), Euclidean distance (ED), and profile similarity definition (PD) for each gene profile pairs (Method S2, ESI†). We then randomized profiles and evaluated MI and PD for 1000 random trials and identified statistically significant MI and PD values.
Functional (GO enrichment) analyses of dynamic and static sub-networks
Over-representation of GO terms in gene sets was determined by using the Biological Networks Gene Ontology tool (BiNGO) (http://www.psb.ugent.be/cbd/papers/BiNGO/).32 BiNGO retrieves the relevant GO annotation and then tests for significance using the hypergeometric test. This tool was used to identify functional enrichment of genes identified through various performed analyses. The enrichment score represents the degree of enrichment (P-values) calculated from hypergeometric distribution, which determines the significance (P < 0.05) of overrepresented term enrichment within a list of genes present in iRC1080, the C. reinhardtii metabolic network used in this study. Correction for multiple testing was not performed.
Synthetic interaction analysis
The maximum in silico growth rate for all possible double-gene deletion combinations in the network (more than 500
000 pairs) was predicted using COBRA Toolbox v.2 under two different conditions of dark and autotrophic light growth. The COBRA toolbox (COBRA = Constraint-Based Reconstruction and Analysis) is a comprehensive collection of tools developed for in silico model-based analysis and reconstruction of metabolic networks.33,34 To simulate growth under dark with acetate (or “DA”), light flux was set to zero and an acetate uptake of up to 10 mmol gDW−1 h−1 was permitted to provide a source of energy and carbon. The wild-type maximum growth rate was 0.7 mmol gDW−1 h−1. Simulation of growth under light with no acetate (or “LNA”) was achieved by setting the acetate intake flux to zero and light flux to 646 mmol gDW−1 h−1. These parameters resulted in a biomass productivity of 0.3 mmol gDW−1 h−1 for wild-type autotrophic metabolism. The value obtained for each double-gene deletion was divided by the wild-type growth rate under both conditions to get the growth rate ratio for each in silico deletion mutant. We only considered the deletion pairs in which the decrease in the metabolic output was greater in the double deletion compared to the sum of the respective single deletions. We classified double deletions that result in zero growth as synthetic lethal and those that reduce the metabolic output as “synthetic sick”. We note that the number of synthetic sick interactions under DA conditions was more than those under LNA in some categories including (100–80)%, (40–20)% and (20–0)%. We did not look at positive synthetic interactions. We note that although there may be limitations in the predictive capabilities of this type of modelling,35 the generated predictions have been validated experimentally in many different cases.36
Statistical analyses of double-gene deletions
To check the normality of the profile distance distribution within the synthetically interacting gene sets, we performed Kolmogorov–Smirnov (KS) tests to measure the maximum absolute difference between our data and standard normal distribution. The standard normal distribution was obtained from profile distances between all the 1086 genes in the network (Method S3, ESI†). Hypergeometric distribution is a measure of the probability that describes the number of successes in a sequence of n draws from a finite population without replacement. In our analysis, we performed hypergeometric tests on the profile distance data of the synthetic interactions genes. The following values were chosen: >1, <2, ≥2, >+3. The tests provide a statistical measure to examine if the synthetic interaction distances are enriched for larger than random network values or not (Method S4, ESI†).
Coupled reaction set analysis
The 2190 reactions of iRC1080 were classified randomly into 20 sets of 100 reactions. iRC1080 is a re-constructed genome scale metabolic network model that accounts for 1080 genes, 2190 reactions and 1086 unique metabolites. It includes 83 subsystems distributed across 10 cellular compartments.31 The solution space was constrained for growth under LNA or DA. The correlated sets of reactions (or co-sets) were obtained using an extension script to the COBRA Toolbox. Genes associated with the co-sets were identified using the findgenesfromReaction function in the COBRA Toolbox. The profile distance of all possible gene pairs between correlated reactions in each co-set was calculated and presented; a hypergeometric test was carried out to evaluate enrichment for short or long evolutionary profile distances was carried out as described in the Synthetic interaction section. The co-sets with only one pair of genes were not considered in this analysis.
C. reinhardtii strain growth and RNA isolation
C. reinhardtii (strain CC-503) was grown as described before.37,38 Briefly, the cells were grown at room temperature (22–25 °C) either in the dark with acetate as a carbon source in Tris-acetate-phosphate (TAP) medium or under continuous white light (with a photosynthetic photon flux of 60 μmol m−2 s−1) without acetate in Tris-phosphate (TP) medium containing 100 mg l−1 carbenicillin. C. reinhardtii cells were harvested at the mid-log phase by centrifugation at 2000 rpm (650 g) for 10 min. Total RNA was isolated from pelleted cells using the TRIzol reagent (Invitrogen). The isolated RNA was treated with 0.08 U μl−1 RNase-free DNase I enzyme (Ambion) to remove any residual cellular DNA. The integrity and quality of the RNA were assessed uisng an Agilent 2100 Bioanalyzer (Agilent) and an RNA Pico 6000 kit according to the manufacturer's instruction. RNA samples with RNA integrity number (RIN) values greater than 7.5 were used in subsequent transcriptome sequencing as described below.
Transcriptome sequencing and gene expression analyses
Transcriptome libraries were constructed using the Roche cDNA Rapid Library protocol; reagents were obtained from 454 Life Sciences Corp., Roche (New York, NY). Briefly, polyadenylated fractions of isolated RNAs were enriched through two rounds of oligo dT selection and the obtained RNAs were fragmented through metal-catalyzed cleavage. The first and second strand cDNA syntheses were carried out according to the Roche recommended protocol. Briefly, polyadenylated fractions of isolated RNAs were enriched through two rounds of oligo dT selection and the obtained RNAs were fragmented through metal-catalyzed cleavage. The first and second strand cDNA syntheses were carried out according to the Roche recommended protocol. The obtained cDNAs were used as input materials for a Roche GS Rapid Library Preparation kit to generate libraries suitable for 454 FLX sequencing. The resulting libraries were purified and clonally amplified in emulsion PCR reactions in the presence of library binding beads according to the manufacturer's instruction (454 Life Sciences Corp., Roche). After amplification and disruption of emulsions, the beads carrying the amplified DNA library were recovered and enriched. The sequencing was performed on a Roche 454 Genome Sequencer Instrument using GS FLX Titanium Sequencing chemistry (XLR70) for 200 flow cycles. Base calling and other primary data processing were done using the GS FLX v2.3 software. Two full runs were carried out for each growth condition, providing technical replicates for each condition. Each run produced between 1.11 and 1.35 million reads with average read lengths ranging from 306 (±112) to 392 (±126) bases. The obtained reads were mapped to a complete set of annotated ORF encoding proteins with metabolic functions using the gsMapper (v2.3) software tool.39 This set of reference sequences consisted of approximately ∼2000 sequences derived from Augustus 5 annotation of JGI v4.0 assembly of the genome. A minimum overlap length of 40 nt and minimum overlap identity of 90% were used to align the reads. The total number of aligned reads to this reference set was used in RPKM calculations according to Mortazavi et al.40 Differential gene expression was assessed using NOIseq (http://bioinfo.cipf.es/noiseq/doku.php?id=start) using default parameters of the software.41
The raw reads for each library were deposited in the NCBI BioSample database and they are accessible through Sequence Read Archive (SRA) accession number SRP065253.
Results
Transformed metabolic network and evolutionary affinities of genes
Metabolic network models describe functional and topological connectivity between metabolites, reactions, and their associated genes. We previously reported a genome-scale reconstruction of the C. reinhardtii metabolic network.31 The network provides a global map of C. reinhardtii metabolic circuitry, including full connectivity between metabolites, genes, and associated reactions. The reconstructed network, iRC1080, accounts for the function of over a thousand genes, as many unique metabolites, and twice as many reactions. The network spans 83 metabolic subsystems in 10 cellular compartments. iRC1080 is an experimentally validated model of C. reinhardtii's metabolism capable of predicting genome-wide metabolic fluxes. This network, as in all reconstructed functional metabolic networks, is a metabolite-centric network where nodes represent metabolites, and links (or edges) between the nodes are associated with reactions. Each reaction is typically associated with one or more gene products; multiple reactions may also be associated with a single gene or metabolite. A transformation of this network to a gene-centric network, where nodes correspond to gene products and edges represent metabolites (or links between enzyme complexes) was needed for our analyses.42 Following the removal of currency metabolites, we used the gene-reaction-metabolite associations described in the network to carry out this transformation (Fig. S1, ESI†). The resulting network (Fig. S2, ESI†) consists of 11
094 edges (connections) between 1086 metabolic gene products, with an average connectivity of ∼21 and a clustering coefficient of 0.57. The network has 14 connected components; 1040 of the 1081 nodes reside in its largest component. We note that the average degree and clustering coefficient of the network are higher than a typical protein–protein interaction network, alluding to a high interconnectivity of metabolic genes and pathways in the network.
We extended the information content of iRC1080 by defining the evolutionary affinities (i.e., sequence similarity) of genes in the C. reinhardtii network (Table S1, ESI†) with protein-coding genes of major eukaryotic lineages. We interrogated over 250 annotated genomes spanning 13 eukaryotic lineages (Table S2, ESI†) with BLAST and clustered the obtained high scoring hits to assign the affinities (Fig. 1 and Table S3, ESI†). The highest number of affinities is assigned to Viridiplantae (green plants) with Stramenopiles (or heterokonts, which include diatoms, golden, and brown algae) and Metazoa (animals) occupying the next two largest groups. Members of Diplomonadida, which do not possess true mitochondria, have the lowest number of affinities assigned. Interestingly, Choanoflagellida, a group of flagellates closely related to animals have a significantly lower number of assigned affinities compared to animals.43 We note that approximately 200 genes in the network remain unassigned to any eukaryotic lineage (other than C. reinhardtii or potentially to other green alga), as their affinities fall below our set threshold of P < 0.001. These genes are likely to have homology to cyanobacteria and other prokaryotes, while a subset may be Chlamydomonas-specific.
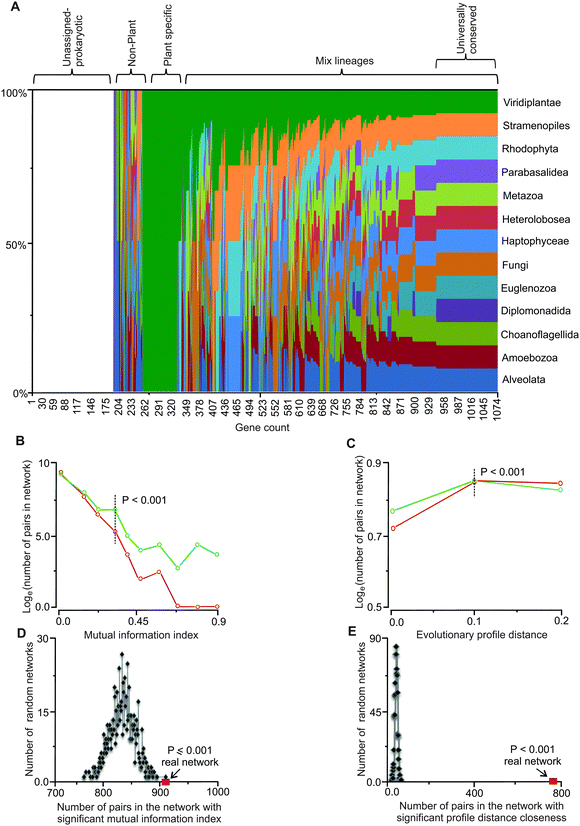 |
| Fig. 1 Evolutionary affinities and co-conservation of genes in the network. (A) Phylogenetic affinities of genes in the network are shown as the fraction of total for each of the 13 eukaryotic lineages explored. The list of the genes and the lineages are provided in Tables S1 and S2 (ESI†), respectively. (B) Comparison of distribution of mutually informative pairs between the real and randomized networks. Mutual information of neighboring genes (nodes) in the network is a measure of dynamic co-conservation (see Methods). The y-axis is in the natural log scale; plots in green and red represent the real and random networks, respectively. At the mutual information index value of 0.3, the difference between gene pairs in the random networks and the real network becomes statistically significant (P = 0.001); this value (i.e., 0.3) was used to identify mutually informative pairs. Four hundred and fifty five genes form 908 gene pairs in the network with index values of 0.3 or higher. (C) Comparison of distribution of number of pairs and evolutionary profile distances between the real and randomized networks (see Methods). Evolutionary profile distances are a measure of co-conservation; only the gene pairs that are conserved in at least 50% of the lineages were included. The plots in green and red represent evolutionary profile distance values of gene pairs in the real and random networks, respectively. The y-axis is in the natural log scale. Profile distances of 0.1 or less (dotted vertical line) display statistically significant differences between the real and random networks, these gene pairs are referred to as statically co-conserved pairs. Two hundred and twenty three genes form 775 such pairs in the real network. All values have been normalized to the maximum value and represented in the graph. (D and E) Mutual information and evolutionary profile distance in randomized networks. Based on randomization of the network threshold, the values for the mutual information index and evolutionary profile distance were set. For every pair of genes in the network, if the mutual information index values were higher than or the profile distance values were less than the set thresholds, those pairs were considered dynamically or statistically co-conserved gene pairs, respectively. (D) The real network (red square) has the highest number of high MI pairs compared to all randomized network (P ≤ 0.001). (E) The number of gene pairs with low PD values in the real network (red square) is significantly higher than the randomized network (P < 0.001). | |
Evolutionary concordances of gene pairs
The obtained conservation information was used to define an evolutionary profile vector for each protein sequence in the network. Each vector carries a 0 or 1 for each of the 13 lineages; therefore, the vector describes the evolutionary affinities of the gene (see Methods) in a format amenable to quantitative analyses. Phylogenetic correspondence has been used to identify and assign functions to genes and their pathways.26,27,44 Here, we defined and integrated phylogenetic information with independently derived functional gene assignments and network topology to empirically link network connectivity with evolutionary affinities. Entropy analyses with respect to evolutionary conservation of genes in the network was carried out by defining a mutual information index, MI,45 for all directly connected gene pairs in the network. We generated 1000 random networks by randomizing affinity profile vectors while maintaining the network properties intact and then carried out MI analysis on the random profile networks. Based on the randomization results, a mutual information index value of ∼0.3 or higher occurs at the probability of P < 0.001 (Fig. 1B). We used this MI value as our threshold for identifying co-conserved pairs. We observed 908 pairs of genes in the network with MI values equal or higher than this threshold (Table S4, ESI†). Four hundred and fifty five genes (nodes) constitute this group. These results show that co-conservation of a significant number of genes (42%) in the network (i.e., 455 out of 1081) is linked to their placements in the network. We consider these as dynamically co-conserved genes because they share a similar vector profile, but may not be conserved across all 13 interrogated lineages.
Universally conserved gene pairs have low MI values and cannot be detected as statistically significant pairs (P < 0.001) while they are clearly evolutionarily constrained. We examined the co-occurrence of highly conserved gene pairs in the network by calculating evolutionary profile distances for each neighboring pair in the network and compared them to randomized network distances. At the normalized distance threshold value of 0.1, occurrences of pairs with 0.1 or lower profile distances become statistically significant relative to random networks (P < 0.001) (Fig. 1C). With this threshold, 775 pairs comprised of 223 gene products (21% of genes in the network) can be detected (Table S5, ESI†). Because these gene pairs have similar profiles that are conserved across most or all of the 13 lineages, we refer to these as statically co-conserved pairs.
We further corroborated the mutual information and evolutionary profile distance analyses by randomizing the network structure (while maintaining the affinity vectors intact) and investigated how many dynamic or static pairs occur in the random networks. The randomized networks in both cases show a statistically significant lower number (P ≤ 0.001) of dynamic and static co-conserved pairs relative to the real network (Fig. 1D and E) indicating that the occurrences of these pairs (at the threshold value used) are not random.
Two sub-networks were reconstructed to examine connectivities within the dynamic and static groups (Fig. 2A and B); the gene pairs not assigned as being dynamic or static are not included in these sub-networks. GO term enrichment of the static (low evolutionary profile distance pairs) and dynamic (high MI) pairs showed a number of overlapping terms; however, most terms were enriched uniquely in the dynamic and static networks; “calcium ion binding” was the only term that was shared between the two sub-networks (Fig. 3A and B and Fig. S3, ESI†). GO terms that were exclusively enriched within the static pairs included nucleotide kinase activity and oxidoreductase activity, acting on sulphur group of donors. On the other hand, galactosidase activity and intramolecular oxidoreductase activity (transposing C
C bonds) were enriched within the dynamic pairs (Fig. S3, ESI†). These results demonstrate that there is considerable segregation between the two sub-networks both topologically and functionally.
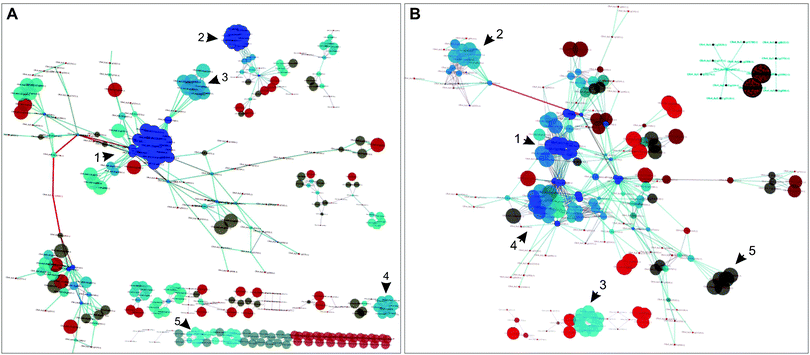 |
| Fig. 2 Dynamically and statically co-conserved pairs in the network. (A) A subnetwork based on the identified dynamic pairs was reconstructed to highlight the connectivity between dynamically co-conserved pairs; non-dynamic nodes were not included in this network. The indicated numbers designate regions of the network described in the text and Fig. S6 (ESI†). The color of the nodes represents their degree (blue highest, dark-red lowest); the size of the nodes corresponds to the clustering coefficient of the nodes. (B) A subnetwork based on the statically co-conserved gene pairs was reconstructed to highlight the connectivity of these genes; non-static genes are not included in this network. | |
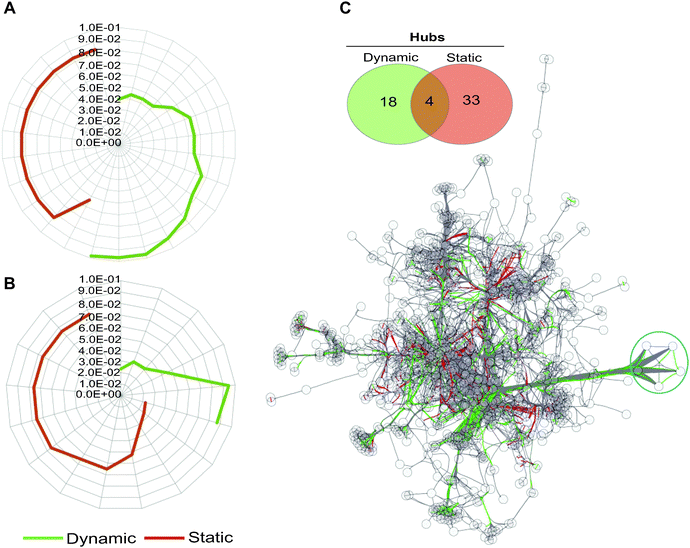 |
| Fig. 3 Gene ontology (GO) term analysis of dynamically and statically co-conserved pairs and their hubs in the network. Uniqueness of GO terms for the dynamic and static sub-networks and their associated enrichment P-values are shown for biological process (A) and molecular function (B) ontologies. For each set, over representation probabilities were determined using the C. reinhardtii metabolic network as a reference (see also Fig. S3, ESI†). No overlap is observed between the dynamic and static GO terms at any significance level in biological process ontologies, while an overlap of a single term is detected for molecular function in (B). (C) The hubs in the network (defined as nodes forming the top 20% of highly connected genes) that show evidence of dynamic and static co-conservation are shown in the network (linked respectively with green and red edges) and reported in the Venn diagram. The blue circle marks five of the most connected hubs in the network. | |
The dynamic network is fragmented and displays more varied conservation. It consists of 89 connected components, many of which consist of isolated bi- or tri-gene groups; its largest connected component consists of 171 genes. The static sub-network is smaller (223 genes) but less fragmented compared to the dynamic sub-network – it encompasses 14 connected components in contrast to 89 components of the dynamic sub-network and its nodes have a higher average degree (6.95 vs. 3.99). The static sub-network is nearly universally conserved.
Hubs, or highly connected nodes in biological networks, often carry important or essential functions.46 To investigate if the hubs in the transformed network show segregation with respect to their co-conservation, we identified highly connected nodes (Table S6, ESI†) and then classified them as dynamic or static on the basis of their interaction with their partnering nodes. We found that hubs with dynamically evolving partners have little overlap with statically evolving hubs (Fig. 3B), which suggests a functional distinction between the two types of hubs. Indeed, the distinction between the two hub types can be observed in the metabolic processes they are involved in; many of the dynamic hubs are involved in photosynthesis or lipid metabolism, whereas the low evolutionary profile distance hubs are involved in central metabolism but not photosynthesis (Tables S7 and S8, ESI†).
Taking the five most connected hubs as examples, four of the five are exclusively dynamic and one is a dual static and dynamic hub (Fig. 3C and Fig. S4, ESI†). The four dynamic hubs encode ferredoxins and are involved in photosynthesis or other metabolic processes such as lipid metabolism. These four hubs have distinct affinities including the following lineages: fungi, Alveolata, Rhodophyta, Stramenopiles, and Viridiplantae. Several distinct ferredoxins are known to be differentially expressed under a variety of specialized conditions.47 For example, in C. reinhardtii, FDX3 has been shown to be involved in nitrogen assimilation, FD4 in glycolysis and response to reactive oxygen species, and FDX5 in hydrogenase maturation under anoxic conditions. Both FDX1 and FDX2 serve as the primary electron donor for NADPH and H2 production, however the electron transfer speed of FDX2 is less than half as fast as that of FDX2,47 so C. reinhardtii is capable of modulating the speed of NADPH and H2 production by differentially expressing these FDXs. Environmental condition variability would have a strong impact on the differential expression and most likely evolutionary maintenance of C. reinhardtii's ferredoxins. The fifth hub in this group encodes an acyl-carrier protein (ACP2), which is involved in lipid metabolism. The encoding gene is conserved across all lineages except for Diplomonadida. There are only three other dual hubs in the network; these encode CYC1 (cytochrome c), a CYC1 paralog, and EamA transporter. Overall, our results support the hypothesis that the dynamic hubs have emerged to fulfill the metabolic fitness of the species under specialized or specific conditions with shared constraints. On the other hand, static hubs are not determinants of specialized metabolic functions, rather they perform universally shared functions.
Dynamic and static metabolic interologs in yeast and Arabidopsis
Conservation of interactions among orthologs was described by Walhout et al.48 in the context of protein–protein interactions and was later shown to be observable at statistically significant rates.49 We thus investigated the extent to which the identified dynamic and static pairs occur in yeast and Arabidopsis following the transformation of their metabolic networks to gene-centric ones based on their GO terms (Method S5, ESI†). For these analyses, we required the ortholog pairs (i.e., interologs) to be directly linked with each other in their respective networks as their counterparts were in the C. reinhardtii network. We compared the interologs of C. reinhardtii/A. thaliana and C. reinhardtii/S. cerevisiae for the identified static and dynamic pairs. From 908 dynamic pairs, we identified 343 and 66 ortholog pairs in A. thaliana and S. cerevisiae, respectively. From 775 static pairs, 427 and 87 ortholog pairs in A. thaliana and S. cerevisiae were identified, respectively. The identified orthologs were then mapped to A. thaliana and S. cerevisiae networks to examine if they form interologs. For the dynamic pairs, 142 interologs (41.4%) in A. thaliana and 18 interologs (27%) in S. cerevisiae could be identified within their respective networks. We found 203 (47.5%) and 45 pairs (51.7%) in A. thaliana and S. cerevisiae occurring as static interologs, respectively. The level of metabolic interologs that our analyses detect is comparable to protein–protein interaction interologs.49
To examine if the dynamic and static interologs are distinguishable with respect to function, we carried out GO enrichment analyses for the identified interologs. The interolog analysis (Fig. 4 and Fig. S5, ESI†) showed that for the static pairs, many enriched GO terms overlap between C. reinhardtii/A. thaliana and C. reinhardtii/S. cerevisiae; and for dynamic pairs, none of the significantly enriched GO terms overlap. For example, a GO term uniquely enriched in the dynamic interologs of C. reinhardtii/A. thaliana but not in C. reinhardtii/S. cerevisiae is the cGMP biosynthetic process. In Chlamydomonas, nitric oxide (NO)-dependent guanylate cyclases (GCs) mediate nitrogen-assimilatory signalling by forming cGMP from GTP in the presence of extracellular ammonium.50 The presence of these interologs in C. reinhardtii/A. thaliana but not in C. reinhardtii/S. cerevisiae indicates dynamic evolution of these components of the nitrogen assimilation signalling pathway in plants but not in yeast50 which is consistent with dynamic pairs being involved in specialized functions. Altogether, these results indicate that while some rewiring of metabolic functions have occurred during evolution, a significant level of conservation has persisted, which in turn attests to a persistence of selective pressure in the course of evolution. As expected, less rewiring is observed in static pairs, particularly in yeast, which is consistent with the centrality of static pairs in the network.
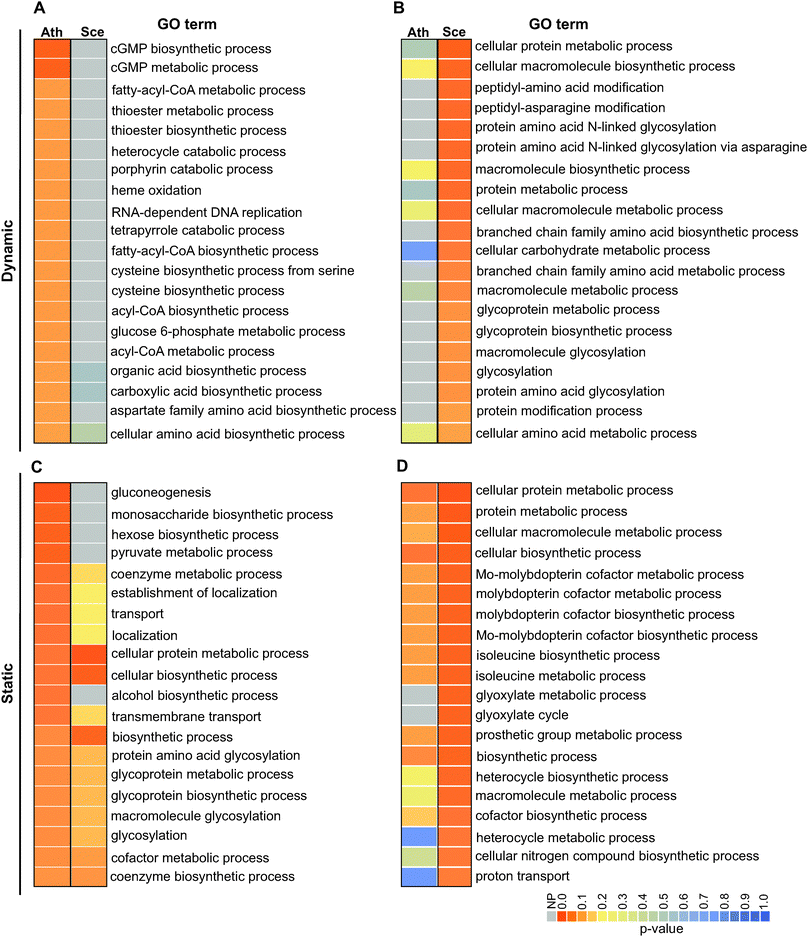 |
| Fig. 4 Gene ontology (GO) analysis of dynamic and static pairs of C. reinhardtii interologs with S. cerevisiae and A. thaliana. GO terms (biological process) of dynamically co-conserved interologs of C. reinhardtii/A. thaliana, and those of C. reinhardtii/S. cerevisiae are shown in (A) and (B); similarly, GO terms of statically co-conserved interologs of C. reinhardtii/A. thaliana, and those of C. reinhardtii/S. cerevisiae are shown in (C) and (D). For each set, enrichment analysis of terms was carried out using the C. reinhardtii metabolic network as reference (see Methods). Table heatmaps were used to visualize the top 20 GO terms based on obtained enrichment P-values. Heatmaps of A and C were sorted based on lowest C. reinhardtii/A. thaliana GO term P-values and heatmaps B and D were sorted based on C. reinhardtii/S. cerevisiae GO term P-values. Ath: A. thaliana, Sce: S. cerevisiae, NP: not present. | |
Differential functional enrichment in dynamic and static sub-networks
Highly connected regions of the network, or network modules, often mark biological complexes with genes involved in related functions. We used a network module detection algorithm, MCODE,51 to define highly connected regions of the two sub-networks. MCODE detected 41 modules for dynamically co-conserved gene pairs, ranking each defined module. The top 5 sub-networks identified based on the MCODE scores are shown in Fig. S6 (ESI†). The highest score was 19.263 with 20 nodes and 183 edges and the lowest score was 3 with 3 nodes and 3 edges (a significant result has a score of greater than 1). We explored the enrichment under GO terms (biological process) for these top 5 modules using BiNGO.32 GO categories were analyzed for enrichment in the top 5 sub-networks with a P-value less than 0.05. The detected processes included the carboxylic acid metabolic process, oxidative phosphorylation, cobalamin metabolic process, tetrahydrobiopterin metabolic process and fatty acid oxidation. The hypergeometric test was used to determine GO annotation overrepresented amongst each cluster (Table S9, ESI†).
For statically co-conserved gene pairs, MCODE lists 21 sub-networks with the highest score of 12.211 (20 nodes and 116 edges) and the lowest score of 2.667 (4 nodes and 4 edges). The top GO terms (biological process) found were lipid glycosylation, glycoside metabolic process and cofactor metabolic process.
There was only one significantly enriched GO term (lipid modification) with overlap between the top five modules of dynamically and statically co-conserved pairs. This indicates that (1) the dynamic and static networks are modular, (2) the largest modules have distinct and non-overlapping functions, and (3) the largest dynamic modules are enriched in specialized functions, while the static modules are involved in more general metabolic functions.
Gene expression and evolutionary affinity dynamics
We hypothesized that there will be detectable relationships between evolutionary and temporal dynamics such that genes and modules up regulated by light would display distinct evolutionary dynamics not observed in dark-induced genes. To explore this hypothesis, we grew C. reinhardtii under light with no organic carbon source and in complete darkness with acetate as a source of energy and then carried out transcriptome analysis of their respective messenger RNAs. These conditions roughly correspond to autotrophic metabolism (as in higher plants) and aerobic heterotrophic metabolism, respectively. Following normalization, we were able to detect metabolic transcripts that were differentially up regulated under light and dark (Fig. 5A, B, Table S10 and Fig. S7, ESI†). By mapping temporal expression information to the dynamic and static gene pairs, we found a significantly higher number of dynamic pairs in the light induced group than the dark induced genes (Fig. 5C). In contrast, we found that the static pairs were enriched under the dark conditions. Comparing significant changes in expression with predicted fluxes (Fig. S8 and Method S6, ESI†) showed that almost half of the active reactions (329 out of 750 reactions) are concurrent with the flux needed. Among 329 reactions, 87 were up regulated and 242 were down regulated based on their transcription. We identified the metabolic subsystems and their locations for flux-expression correlated up- and down-regulated genes. Among 242 down-regulated genes, the largest group of 90 genes was in the glycerolipid metabolism subsystem and 115 genes were from the Cytosol compartment. For up regulated genes, the highest group of 9 was in the fatty acid biosynthesis subsystem and 28 genes were located in the cytosol as shown in Fig. S9 and Table S11, ESI†.
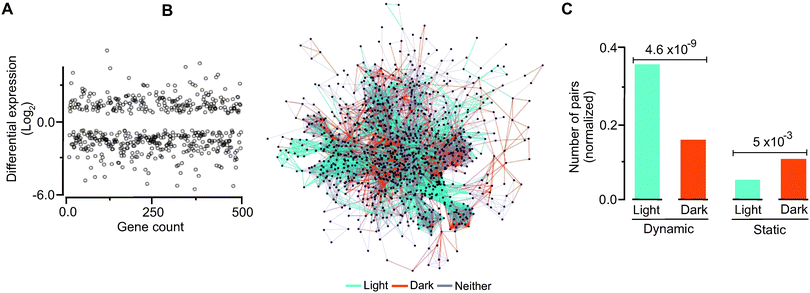 |
| Fig. 5 Temporal dynamics and co-conservation. (A) Identification of light and dark up regulated genes using NOISeq. RNAs isolated in cells grown in the dark with acetate as an energy source or in light with no acetate were subjected to transcriptome sequencing. There are 299 light condition and 211 dark condition genes that are significantly up regulated. About half of the genes in the network could be identified as being up regulated under one of the two conditions (genes not displaying significant differential regulation are not shown in the figure). (B) Light and dark gene pairs mapped to the network. The network representation visually shows that up regulated genes in light tend to form independent units or modules in the network, while dark up regulated genes tend to be positioned centrally in the network, connecting modules. (C) Temporal dynamics and co-conservation. Light and dark up regulated genes were plotted for gene pairs in each of the co-conservation groups (i.e., dynamic and static). The light up regulated gene pairs tend to be dynamic, while dark up regulated gene pairs are more static; the statistical significances for differences between the occurrences of dynamic and static pairs with respect to light–dark regulation are indicated. | |
Synthetic interactions and evolutionary profile distances
To investigate if there are non-topological relationships between gene function and evolutionary profile distance, evolutionary profile distances between synthetically interacting genes in the network can be investigated. Unlike yeast in which double gene deletion studies can be done experimentally, such experiments in C. reinhardtii are not presently feasible. Therefore, we carried out in silico double-gene deletions using our reconstructed model (iRC1080) under simulated dark and light conditions (over 500
000 double deletions under each condition, Fig. 5A, Tables S12 and S13, ESI†) and predicted the resulting biomass yields accordingly. We further binned the interactions according to the resulting level of biomass reduction (Fig. S10, ESI†) and calculated their pairwise evolutionary profile distances. The pairwise profile distances (Euclidean distances) between synthetically interacting genes showed a range of values and in many cases values of above 1, indicating that the genes that are involved in the interactions have distinct evolutionary affinities.
We carried out the Kolmogorov–Smirnov (KS) test for measuring the maximum absolute difference between our data and the standard normal distribution with the null hypothesis being that the distances between the interacting pairs follow that of random interactions in the network. The standard normal distribution was obtained from evolutionary profile distances between all 1086 genes in the network. As illustrated in Table S14 (ESI†), the KS test revealed that the synthetic interaction distribution is not a standard normal distribution. A significant difference was observed between the random pairs in the network and the synthetic interaction profile distances under both light and dark simulated biomass production. These results show that the evolutionary affinities of the genes involved in the synthetic interactions under both conditions of growth in light with no acetate (LNA) and in the dark with acetate (DA) differ from the overall pairwise distance distributions of the network.
To test if the interacting pairs are enriched for short or long evolutionary profile distances as compared to random pairs in the network, we performed hypergeometric tests for enrichment of distances greater than or equal to 1, 2 and 3 for both light with no acetate or LNA and dark with acetate or DA conditions. We observed that under LNA conditions, synthetic interactions with values greater than 2 are significantly enriched; in contrast, under DA conditions, interactions with profile distances of less than 1 and 2 show a significant enrichment (Fig. 6B and Table S15, ESI†). GO term enrichment analysis was carried out on each of the different bins under both growth conditions and major results are found in Table S16 (ESI†). The lists of double-gene knockouts under two different conditions; DA and LNA, were used to create the gene interaction networks using Cytoscape and compare the selected KEGG pathways for each condition (Fig. S11A and B, ESI†). The KEGG pathway enrichment between two conditions (light and dark) for synthetic lethal conditions shows the enrichment of a number of pathways common between the two conditions (Fig. S12, ESI†). As an example, synthetic interactions in the KEGG pathway are shown in Fig. S13–S17 (ESI†).
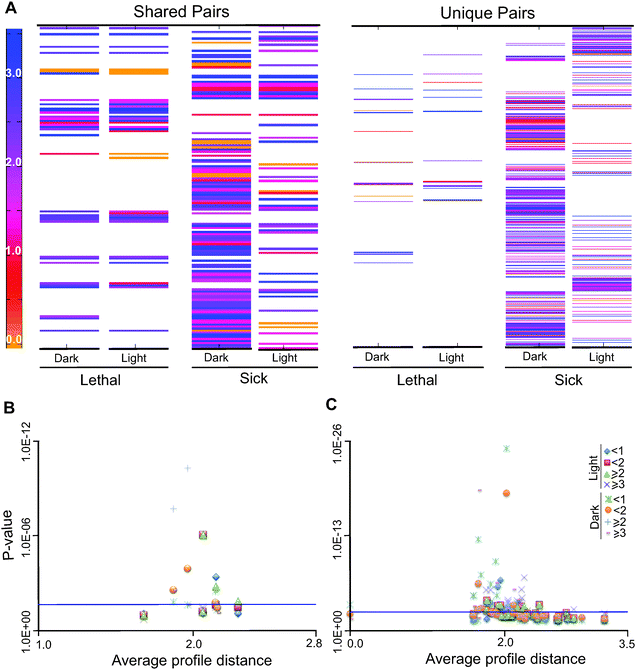 |
| Fig. 6 Evolutionary profile affinity distances and functional analysis of the C. reinhardtii network. (A–C) Double gene deletion analyses were carried out under light with no acetate or dark with acetate to define synthetically interacting pairs, the identified pairs were then binned according to the severity of their effects on predicted biomass production. The bins represent strict inequalities for the upper bounds. (A) Heat maps that represent the shared and unique synthetic interaction gene pairs under LNA and DA. Shared pairs are the pairs that are shared between lethal and sick under light with no acetate or dark with acetate. Unique pairs are pairs that are unique for lethal or sick under LNA or DA conditions. The color gradient from yellow to blue corresponds to the evolutionary profile distances between the pairs from 0 to 4. (B) Hypergeometric tests were done for enrichment of indicated distances for binned synthetically interacting pairs under LNA or DA conditions. (C) The results of hypergeometric tests are shown for enrichment of different evolutionary profile distances found in each co-set under LNA and DA conditions. The blue line above the x-axis marks the 0.05 probability threshold for statistical significance. | |
Coupled reactions and evolutionary profile distances
Coupled or correlated sets (co-sets) of reactions are the reactions that function together in the metabolic process.52,53 The biological significance of these linked reactions has been observed. For instance in the case of genetic disorders, mutations in correlated reactions can often lead to the same disease phenotype.54 The 2190 reactions of iRC1080 were classified randomly into 20 sets with 100 reactions each, the solution space for each 100 was explored and constrained for growth under LNA or DA. The co-sets were obtained using COBRA Toolbox functions (Tables S17 and S18, ESI†).
We identified the genes associated with the reactions in the co-sets and calculated the evolutionary profile distances of all possible gene pairs between reactions (we note that some reactions are associated with multiple genes and some reactions have no associated genes) (Fig. 6C). As in synthetic pairs (described in the previous section), we observed many of the distances to be greater than 1, indicating different phylogenetic profiles among the genes. A hypergeometric test was carried out in relation to the random evolutionary profile distances of the whole network (all possible gene combinations in the network). The co-sets with only one pair of genes were not considered in this analysis. The test revealed the statistical significance of distance values of less than 2 and values of 3 or greater (Tables S19 and S20, ESI†). The enrichment probabilities become more significant with distances of less than one, or 3 or greater. These analyses indicate that the enrichments of co-sets are bipartite relative to random network distances, with over-representation of both short and long distances within the sets.
Discussion
Genes in metabolic networks tend to be well-conserved,55 however their co-conservation dynamics are not well understood. Through our analyses on a model organism that can be considered complex with respect to its phylogenetic affinities, we find metabolic genes to display distinct dynamics with respect to their conservation, network topologies, and functional relationships. A significant percentage of the genes in the network were identified as being involved in either a dynamic or static co-conservation. The herein described detection of dynamic and static pairs in the network, their non-random segregation at the network level, and their expression dynamics, provide evidence for constraints embedded in the evolution of metabolic networks. This in turn provides evidence for conservation of selective constraints between eukaryotic species in different lineages for some node-pairs in the network. However, while the occurrences of co-conserved pairs are statistically significant, the majority of gene-pairs in the network do not display statistically significant co-conservation, indicating a lack of uniformity in detectable co-conservation relationships in the network. This may also reflect major instances of discontinuity in functional interactions between genes across phylogeny.56–58
The presence of non co-conserved pairs in the network, which constitute the majority of pairs, implies that functional constraints for these genes are not shared between C. reinhardtii and the explored lineages in the context of the studied metabolic network, at least not in the context of neighboring gene-pairs in the network in a consistent manner. This fluidity in co-conservation, which we also observe in functional analyses of the network, in turn suggests that rewiring of metabolic pathways may be a significant contributing force behind evolutionary adaptations as recent data have suggested being the case in genetic interaction and transcription networks.59,60
Our analyses identify most network hubs as either dynamic or static with very few having characteristics of both. This is a consequence of the topological segregation of dynamic and static pairs. As we have demonstrated, this segregation is also manifested with respect to both regulation, as judged on the basis of enrichment under light and dark growth conditions (Fig. 5C), and with respect to function as indicated on the basis of differential enrichments of GO terms. With respect to the latter, we note that this differential enrichment is observable at the level of the entire subnetwork (Fig. 3A and B and Fig. S3, ESI†) as well as at the module level (Fig. S6 and Table S9, ESI†). Taken together, the observed topological, temporal, and functional segregation of the static and dynamic pairs and hubs suggests that these segregated organizations may provide adaptive values in varying evolutionary niches. In biological terms, the different ferredoxins that form major hubs in the network are expected not to be interchangeable as they may have different redox potentials.61 As we have illustrated, these proteins have different evolutionary affinities and mostly demonstrate dynamic co-conservation, which likely reflect different biochemical requirements in species belonging to different lineages. Taking into account the crucial functions of ferredoxins and their involvements in large sets of reactions and pathways,62 selective pressures in maintaining the optimal redox potential can be expected for a specific set of ferredoxins in each lineage. This and other similar hypotheses fall in line with what Fang et al., 2013 set forth in terms of gene co-expression and evolution.63 They concluded that selective pressure acts on the relationship between genes rather than on individual genes, which may further explain the maintaining of a set of ferredoxins within the C. reinhardtii metabolic network.
Our analyses show a range of evolutionary profile distances for genes in coupled reaction sets as well as those with predicted synthetic interactions, which as in our topological analyses, point to fluctuations in co-conservation within the network despite a shared or related function. Synthetic pairs identified under “dark” metabolism are enriched for pairs with (Euclidean) distances of 1 or less in their phylogenetic profiles, indicating that these gene pairs have very similar phylogenetic profiles. Gene pairs showing synthetic interactions under light growth are enriched in distant values of 2 or greater and less than 1, the former indicates distant evolutionary profile distances despite a related function in the network. Genes in the co-sets show a similar bimodal enrichment with some extremes observable, that is, some co-sets are enriched with less than 1 and some are enriched for values of equal or greater than 3.
Notably, co-sets under both dark and light conditions, and with a range of profile distances are shown to be involved in purine catabolism, N-glycan biosynthesis, and fatty acid biosynthesis. Importantly, the N-glycan biosynthesis pathway involves an intersection of light and dark relevant co-sets with long and short profile distances, respectively (Note S1, ESI†). Furthermore, synthetic lethal interactions link N-glycan metabolism and fructose and mannose metabolism (profile distance of 3.6), and the pentose-phosphate pathway with the biosynthesis of steroids (profile distance of 0) under light conditions. As for dark condition interactions, amino acid synthesis and nitrogen metabolism are observed to interact with a profile distance of 3.4 (Note S2, ESI†).
It is to be noted that correlated reactions as well as synthetic interactions can be distant in the network topologically (a few examples are shown in Fig. S13–S17, ESI†). Therefore, enrichment for large evolutionary profile distances may coincide with distant placements in the network. As such, the C. reinhardtii network can be hypothesized to have assembled by evolutionary adaptive processes in such a way that evolutionary rigidity (exemplified as statically co-conserved pairs and short distances in synthetic and co-set pairs) and plasticity (exemplified by dynamically co-conserved pairs and long distances in synthetic and co-set pairs) are segregated. The need for such plasticity may be evident at the physiological level with the recent observation that a wide range of metabolites can be utilized by C. reinhardtii as nitrogen sources, including di- and tripeptides as well as a number of D-amino acids.64 Moreover, when buffering of pathways is required, the network architecture makes use of genes with dissimilar phylogenetic profiles. These findings provide an alternative and a wider perspective on metabolic network architecture and evolution.
Author contributions
K. S.-A., L. G., S. B., H. Y., and J. P. conceived the study; L. G. and X. Y. carried out the experiments; P. N., A. J. and A. C. carried out network transformations; A. C., B. D., B. K., A. J., D. R. N., and H. C. carried out data analyses; S. B., A. C., B. D., and R. L. C. carried out phylogenetic, expression, network and FBA analyses; K. S.-A., A. C., B. K., B. D., S. B., L. G., D. R. N., K. J., and J. K. wrote the manuscript.
Acknowledgements
This research was supported by New York University Abu Dhabi Research funds AD060 (to K. S.-A.); New York University Abu Dhabi Institute grant G1205-1205i (to K. S.-A.); the Office of Science (Biological and Environmental Research), US Department of Energy, Grant DE-FG02-07ER64496 (to K. S.-A. and J. P.); Institute Sponsored Research funds from the Dana-Farber Cancer Institute Strategic Initiative; US National Institute of General Medical Sciences Grants GM097358 and GM104424 (to H. Y.), and GM088244 (to J. P.); and Gordon and Betty Moore Foundation GBMF 2550.04 Life Sciences Research Foundation postdoctoral fellowship (to R. L. C.). SB acknowledges the UK Medical Research Council fund (U105185859) for support. We thank Khaled Hazzouri for his comments on the manuscript and the anonymous reviewers for their insightful comments and suggestions.
References
- J. F. Matias Rodrigues and A. Wagner, PLoS Comput. Biol., 2009, 5, e1000613 CrossRef PubMed.
- M. Parter, N. Kashtan and U. Alon, BMC Evol. Biol., 2007, 7, 169 CrossRef PubMed.
- K. Takemoto, J. C. Nacher and T. Akutsu, BMC Bioinf., 2007, 8, 303 CrossRef PubMed.
- A. Mazurie, D. Bonchev, B. Schwikowski and G. A. Buck, BMC Syst. Biol., 2010, 4, 59 CrossRef PubMed.
- C. Pal, B. Papp, M. J. Lercher, P. Csermely, S. G. Oliver and L. D. Hurst, Nature, 2006, 440, 667–670 CrossRef CAS PubMed.
- Y. Zhang, I. Thiele, D. Weekes, Z. Li, L. Jaroszewski, K. Ginalski, A. M. Deacon, J. Wooley, S. A. Lesley, I. A. Wilson, B. Palsson, A. Osterman and A. Godzik, Science, 2009, 325, 1544–1549 CrossRef CAS PubMed.
- S. B. Carroll, Nature, 2001, 409, 1102–1109 CrossRef CAS PubMed.
- B. Khraiwesh, E. Qudeimat, M. Thimma, A. Chaiboonchoe, K. Jijakli, A. Alzahmi, M. Arnoux and K. Salehi-Ashtiani, Sci. Rep., 2015, 5, 17434 CrossRef CAS PubMed.
- J. J. Diaz-Mejia, E. Perez-Rueda and L. Segovia, Genome Biol., 2007, 8, R26 CrossRef PubMed.
-
K. Salehi-Ashtiani, J. Koussa, B. S. Dohai, A. Chaiboonchoe, H. Cai, K. A. Dougherty, D. R. Nelson, K. Jijakli and B. Khraiwesh, Biomass and Biofuels from Microalgae, Springer, 2015, pp. 173–189 Search PubMed.
- R. Alves, R. A. Chaleil and M. J. Sternberg, J. Mol. Biol., 2002, 320, 751–770 CrossRef CAS PubMed.
- H. Jeong, B. Tombor, R. Albert, Z. N. Oltvai and A. L. Barabasi, Nature, 2000, 407, 651–654 CrossRef CAS PubMed.
- E. Almaas, B. Kovacs, T. Vicsek, Z. N. Oltvai and A. L. Barabasi, Nature, 2004, 427, 839–843 CrossRef CAS PubMed.
- C. Wan, B. Borgeson, S. Phanse, F. Tu, K. Drew, G. Clark, X. Xiong, O. Kagan, J. Kwan and A. Berzginov, Data in Brief, 2016, 6, 715–721 CrossRef PubMed.
- D. Vitkup, P. Kharchenko and A. Wagner, Genome Biol., 2006, 7, R39 CrossRef PubMed.
- C. Lu, Z. Zhang, L. Leach, M. J. Kearsey and Z. W. Luo, Genome Biol., 2007, 8, 407 CrossRef PubMed.
- R. Mahadevan and B. O. Palsson, Biophys. J., 2005, 88, L07–L09 CrossRef CAS PubMed.
- B. Papp, C. Pal and L. D. Hurst, Nature, 2004, 429, 661–664 CrossRef CAS PubMed.
- S. Wright, Genetics, 1931, 16, 97–159 CAS.
- S. A. Kauffman and E. D. Weinberger, J. Theor. Biol., 1989, 141, 211–245 CrossRef CAS PubMed.
- S. A. Kauffman and S. Johnsen, J. Theor. Biol., 1991, 149, 467–505 CrossRef CAS PubMed.
- S. A. Kauffman and E. D. Weinberger, J. Theor. Biol., 1989, 141, 211–245 CrossRef CAS PubMed.
- F. J. Poelwijk, D. J. Kiviet, D. M. Weinreich and S. J. Tans, Nature, 2007, 445, 383–386 CrossRef CAS PubMed.
- S. Bershtein, M. Segal, R. Bekerman, N. Tokuriki and D. S. Tawfik, Nature, 2006, 444, 929–932 CrossRef CAS PubMed.
- X. He, W. Qian, Z. Wang, Y. Li and J. Zhang, Nat. Genet., 2010, 42, 272–276 CrossRef CAS PubMed.
- D. Eisenberg, E. M. Marcotte, I. Xenarios and T. O. Yeates, Nature, 2000, 405, 823–826 CrossRef CAS PubMed.
- S. V. Date and E. M. Marcotte, Nat. Biotechnol., 2003, 21, 1055–1062 CrossRef CAS PubMed.
- C. Von Mering, E. M. Zdobnov, S. Tsoka, F. D. Ciccarelli, J. B. Pereira-Leal, C. A. Ouzounis and P. Bork, Proc. Natl. Acad. Sci. U. S. A., 2003, 100, 15428–15433 CrossRef CAS PubMed.
- J. Zhao, G.-H. Ding, L. Tao, H. Yu, Z.-H. Yu, J.-H. Luo, Z.-W. Cao and Y.-X. Li, BMC Bioinf., 2007, 8, 311 CrossRef PubMed.
- M. Kanehisa, FEBS Lett., 2013, 587, 2731–2737 CrossRef CAS PubMed.
- R. L. Chang, L. Ghamsari, A. Manichaikul, E. F. Hom, S. Balaji, W. Fu, Y. Shen, T. Hao, B. O. Palsson, K. Salehi-Ashtiani and J. A. Papin, Mol. Syst. Biol., 2011, 7, 518 CrossRef PubMed.
- S. Maere, K. Heymans and M. Kuiper, Bioinformatics, 2005, 21, 3448–3449 CrossRef CAS PubMed.
- N. E. Lewis, H. Nagarajan and B. O. Palsson, Nat. Rev. Microbiol., 2012, 10, 291–305 CrossRef CAS PubMed.
- J. Koussa, A. Chaiboonchoe and K. Salehi-Ashtiani, BioMed Res. Int., 2014, 2014, 649453 CrossRef PubMed.
- J. D. Orth, I. Thiele and B. Ø. Palsson, Nat. Biotechnol., 2010, 28, 245–248 CrossRef CAS PubMed.
- S. A. Becker, A. M. Feist, M. L. Mo, G. Hannum, B. Ø. Palsson and M. J. Herrgard, Nat. Protoc., 2007, 2, 727–738 CrossRef CAS PubMed.
- J. M. Flowers, K. M. Hazzouri, G. M. Pham, U. Rosas, T. Bahmani, B. Khraiwesh, D. R. Nelson, K. Jijakli, R. Abdrabu and E. H. Harris, Plant Cell, 2015, 27, 2353–2369 CrossRef CAS PubMed.
-
R. Abdrabu, S. K. Sharma, B. Khraiwesh, K. Jijakli, D. R. Nelson, A. Alzahmi, J. Koussa, M. Sultana, S. Khapli and R. Jagannathan, Essentials of Single-Cell Analysis, Springer, 2016, pp. 363–382 Search PubMed.
- L. Ghamsari, S. Balaji, Y. Shen, X. Yang, D. Balcha, C. Fan, T. Hao, H. Yu, J. A. Papin and K. Salehi-Ashtiani, BMC Genomics, 2011, 12(suppl. 1), S4 CrossRef CAS PubMed.
- A. Mortazavi, B. A. Williams, K. McCue, L. Schaeffer and B. Wold, Nat. Methods, 2008, 5, 621–628 CrossRef CAS PubMed.
- S. Tarazona, F. Garcia-Alcalde, J. Dopazo, A. Ferrer and A. Conesa, Genome Res., 2011, 21, 2213–2223 CrossRef CAS PubMed.
- H. Yu, Y. Xia, V. Trifonov and M. Gerstein, Genome Biol., 2006, 7, R55 CrossRef PubMed.
- M. Carr, B. S. Leadbeater, R. Hassan, M. Nelson and S. L. Baldauf, Proc. Natl. Acad. Sci. U. S. A., 2008, 105, 16641–16646 CrossRef CAS PubMed.
- M. Pellegrini, E. M. Marcotte, M. J. Thompson, D. Eisenberg and T. O. Yeates, Proc. Natl. Acad. Sci. U. S. A., 1999, 96, 4285–4288 CrossRef CAS.
- S. Balaji, M. M. Babu and L. Aravind, J. Mol. Biol., 2007, 372, 1108–1122 CrossRef CAS PubMed.
- A. L. Barabasi, N. Gulbahce and J. Loscalzo, Nat. Rev. Genet., 2011, 12, 56–68 CrossRef CAS PubMed.
- E. A. Peden, M. Boehm, D. W. Mulder, R. Davis, W. M. Old, P. W. King, M. L. Ghirardi and A. Dubini, J. Biol. Chem., 2013, 288, 35192–35209 CrossRef CAS PubMed.
- A. J. M. Walhout, R. Sordella, X. Lu, J. L. Hartley, G. F. Temple, M. A. Brasch, N. Thierry-Mieg and M. Vidal, Science, 2000, 287, 116–122 CrossRef CAS PubMed.
- H. Yu, N. M. Luscombe, H. X. Lu, X. Zhu, Y. Xia, J.-D. J. Han, N. Bertin, S. Chung, M. Vidal and M. Gerstein, Genome Res., 2004, 14, 1107–1118 CrossRef CAS PubMed.
- A. de Montaigu, E. Sanz-Luque, A. Galván and E. Fernández, Plant Cell, 2010, 22, 1532–1548 CrossRef CAS PubMed.
- G. D. Bader and C. W. Hogue, BMC Bioinf., 2003, 4, 2 CrossRef PubMed.
- A. P. Burgard, E. V. Nikolaev, C. H. Schilling and C. D. Maranas, Genome Res., 2004, 14, 301–312 CrossRef CAS PubMed.
- J. A. Papin, J. L. Reed and B. O. Palsson, Trends Biochem. Sci., 2004, 29, 641–647 CrossRef CAS PubMed.
- N. Jamshidi and B. Ø. Palsson, Mol. Syst. Biol., 2006, 2, 38 CrossRef PubMed.
- J. Castresana, Biochim. Biophys. Acta, Bioenerg., 2001, 1506, 147–162 CrossRef CAS.
- C. J. Ryan, A. Roguev, K. Patrick, J. Xu, H. Jahari, Z. Tong, P. Beltrao, M. Shales, H. Qu, S. R. Collins, J. I. Kliegman, L. Jiang, D. Kuo, E. Tosti, H.-S. Kim, W. Edelmann, M.-C. Keogh, D. Greene, C. Tang, P. Cunningham, K. M. Shokat, G. Cagney, J. P. Svensson, C. Guthrie, P. J. Espenshade, T. Ideker and N. J. Krogan, Mol. Cell, 2012, 46, 691–704 CrossRef CAS PubMed.
- A. Roguev, S. Bandyopadhyay, M. Zofall, K. Zhang, T. Fischer, S. R. Collins, H. Qu, M. Shales, H.-O. Park, J. Hayles, K.-L. Hoe, D.-U. Kim, T. Ideker, S. I. Grewal, J. S. Weissman and N. J. Krogan, Science, 2008, 322, 405–410 CrossRef CAS PubMed.
- A. Frost, M. G. Elgort, O. Brandman, C. Ives, S. R. Collins, L. Miller-Vedam, J. Weibezahn, M. Y. Hein, I. Poser, M. Mann, A. A. Hyman and J. S. Weissman, Cell, 2012, 149, 1339–1352 CrossRef CAS PubMed.
- S. R. Collins, A. Roguev and N. J. Krogan, Methods Enzymol., 2010, 470, 205–231 CAS.
- M. Madan Babu, S. A. Teichmann and L. Aravind, J. Mol. Biol., 2006, 358, 614–633 CrossRef CAS PubMed.
- R. K. Cammack, K. K. Rao, C. P. Bargeron, K. G. Hutson, P. W. Andrew and L. J. Rogers, Biochem. J., 1977, 168, 205–209 CrossRef CAS PubMed.
- G. Hanke and P. Mulo, Plant, Cell Environ., 2013, 36, 1071–1084 CrossRef CAS PubMed.
- G. Fang, K. D. Passalacqua, J. Hocking, P. M. Llopis, M. Gerstein, N. H. Bergman and C. Jacobs-Wagner, BMC Genomics, 2013, 14, 450 CrossRef PubMed.
- A. Chaiboonchoe, B. S. Dohai, H. Cai, D. R. Nelson, K. Jijakli and K. Salehi-Ashtiani, Front. Bioeng. Biotechnol., 2014, 2, 3389 CrossRef PubMed.
Footnotes |
† Electronic supplementary information (ESI) available. See DOI: 10.1039/c6mb00237d |
‡ These authors contributed equally to this work. |
§ Present address: Genocea Biosciences, 100 Acorn Park Drive, Cambridge, MA, USA. |
¶ Present address: BGI-Shenzhen, Shenzhen 518083, China. |
|| Present address: Departments of Obstetrics & Gynecology, Nanfang Hospital, Southern Medical University, Guanzhou, 510515, China. |
|
This journal is © The Royal Society of Chemistry 2016 |