DOI:
10.1039/D0NR07983A
(Communication)
Nanoscale, 2021,
13, 6016-6023
Visualizing tumour self-homing with magnetic particle imaging†
Received
8th November 2020
, Accepted 17th February 2021
First published on 8th March 2021
Abstract
Due to their innate tumour homing capabilities, in recent years, circulating tumour cells (CTCs) have been engineered to express therapeutic genes for targeted treatment of primary and metastatic lesions. Additionally, previous studies have incorporated optical or PET imaging reporter genes to enable noninvasive monitoring of therapeutic CTCs in preclinical tumour models. An alternative method for tracking cells is to pre-label them with imaging probes prior to transplantation into the body. This is typically more sensitive to low numbers of cells since large amounts of probe can be concentrated in each cell. The objective of this work was to evaluate magnetic particle imaging (MPI) for the detection of iron-labeled experimental CTCs. CTCs were labeled with micro-sized iron oxide (MPIO) particles, administered via intra-cardiac injection in tumour bearing mice and were detected in the tumour region of the mammary fat pad. Iron content and tumour volumes were calculated. Ex vivo MPI of the tumours and immunohistochemistry were used to validate the imaging data. Here, we demonstrate for the first time the ability of MPI to sensitively detect systemically administered iron-labeled CTCs and to visualize tumour self-homing in a murine model of human breast cancer.
Introduction
Tumour self-homing describes a phenomenon where circulating tumour cells (CTCs) that have shed from a primary tumour into the circulation can return to grow at their original tumour site. This concept was first described by Norton and Massagué in 2006 and is thought to be driven by both a leaky vasculature that permits CTC recruitment, as well as a permissive tumour microenvironment that promotes CTC survival and growth.1,2 Due to their tumour targeting capabilities, in recent years, self-homing CTCs have been repurposed as delivery vehicles for anti-cancer therapeutics. This has included the delivery of oncolytic viruses, pro-drug activatable suicide genes, and transgenes that alter the tumour microenvironment.3–9 This strategy has shown exciting progress towards treating primary tumours, single organ metastases and most recently, multi-organ metastases however, further refinement is needed in order to optimize self-homing CTCs for potential clinical translation. Tools that enable the fate of systemically administered CTCs to be noninvasively monitored over time would provide valuable information about the kinetics and efficiency of CTC infiltration into tumours, their proliferation and persistence over time, any unwanted off-tumour accumulation, as well as information to better understand therapeutic response in individual subjects.
Cellular imaging can be used to noninvasively study a specific cell population or cellular process in vivo. Previous studies have used imaging reporter genes, namely bioluminescence imaging (BLI)4,9 and positron emission tomography (PET) reporters,7,8 to track CTCs in preclinical models. For instance, in 2007, Power and colleagues used dual-enzyme BLI, a commonly used optical modality, to noninvasively monitor both the carrier cells (i.e., cancer cells) and their viral payload in vivo. By using this system, they demonstrate independent monitoring of cell vehicle distribution and virus associated luciferase enzymes. Our group has recently used dual BLI to visualize spontaneous whole-body breast cancer metastases expressing Renilla luciferase, and systemically administered theranostic CTCs expressing Firefly luciferase. Additionally, as a step towards tracking CTCs in patients, Reinshagen et al., recently demonstrated that the PET reporter gene, herpes simplex virus-thymidine kinase (HSV-TK) can be used to monitor the fate of therapeutic CTCs derived from glioblastoma.
An alternative method for tracking cells is to pre-label them with imaging probes prior to transplantation into the body.10–16 These techniques are complementary to reporter gene techniques and often used for short term tracking of the initial arrest of cells at a target site, due to probe dilution during cell division. An advantage of probe-based tracking is that it does not require genetic engineering of cells, which may be more feasible for translational purposes. Moreover, probe-based cell tracking is often much more sensitive to low numbers of cells compared to reporter gene technologies due to the fact that large amounts of probe can be concentrated into each cell. Our group has previously shown MRI of cells loaded with superparamagnetic iron oxide (SPIO) particles with single cell sensitivity in various mouse models.13,14,16 We and others have used this extensively to track cell types such as cancer cells, stem cells and immune cells.10–16 However, some limitations of SPIO-based MRI are that SPIOs create a loss of signal and quantitation of signal loss and the number of iron-labelled cells in a particular region is challenging.11 Magnetic particle imaging (MPI) is an emerging imaging technique that sensitively and specifically detects SPIOs.17–25 In MPI, SPIOs result in positive signal and the signal strength is linearly proportional to the number of SPIOs, which allows for truly quantitative imaging. A few groups have started to explore the potential of MPI as a novel cell tracking technology with various SPIOs (e.g., ferumoxytol or ferucarbotran).18–24 However, very few groups have explored the use of MPI to track the biodistribution of systemically administered SPIO-labeled cells. In this work, we monitor the fate of SPIO-labeled experimental CTCs in tumour bearing mice and demonstrate for the first time, the visualization of tumour self-homing in a mouse model of breast cancer with high sensitivity MPI.
Methods
Cell labeling
MDA-MB-231 and MDA-MB-231BR-eGFP cells were each maintained in Dulbecco's modified eagle media (DMEM) containing 10% fetal bovine serum (FBS) at 37 °C and 5% CO2. For cell labeling, 2 × 106 adherent cells were incubated with 25 μg Fe per mL micron-sized iron-oxide (MPIO) particles (0.9 μm in diameter, 63% magnetite, labeled with Flash Red; Bangs Laboratory, Fishers, IN, USA) for 24 hours. Cells were washed three times with Hanks balanced salt solution (HBSS) and then trypsinized with 0.25% Trypsin-EDTA. The cells were then collected and thoroughly washed three more times with HBSS to remove unincorporated MPIO before cell injection and in vitro evaluation. Cell labeling had no effect on cell viability, and labeling efficiency was assessed by Perl's Prussian blue (PPB) staining.
Iron particle characterization
MPI relaxometry was performed for MPIO and Vivotrax. Vivotrax is currently the most commonly used SPIO for MPI of cells and therefore it was used here to evaluate MPIO. Samples of both Vivotrax and MPIO were made by diluting the iron particle solution in PBS so that the samples contained the same amount of iron (30 mg). Each sample was scanned separately on the MPI system in RELAX mode. Each scan takes approximately one minute to acquire per sample. MPI relaxometry measures the net magnetization of a specific sample in the presence of a varying applied magnetic field as it goes from negative to positive and back. The output is the derivative of the Langevin function, also called the point spread function (PSF). The signal intensity, or height, of the PSF, reflects the sensitivity of the SPIO. The relative sensitivity is reported for MPIO, compared to Vivotrax. The full-width half maximum (FWHM) relates to the spatial resolution of the SPIO. To estimate the resolution in mm, the FWHM (given in T) was divided by the gradient strength (T m−1).
Animal model
The animals were cared for in accordance with the standards of the Canadian Council on Animal Care (Guide to the Care and Use of Experimental Animals, vol. 1, 2nd edn, 1993) and all studies were performed under an approved protocol (2015-0558) of the University of Western Ontario's Council on Animal Care. A primary mammary fat pad (MFP) tumour was generated by injecting 1 × 106 MDA-MB-231 cells into the lower right MFP of 4 female NOD scid gamma (NSG) mice (6–7 weeks old; Charles River Laboratories, Wilmington, MA, USA). Cells were suspended in 0.05 mL of HBSS per injection. After 41 days of primary tumour growth, mice received an intracardiac (IC) injection of 5 × 105 MPIO labeled MDA-MB-231BR-eGFP cells into the left ventricle. An IC injection was chosen to mimic the natural metastatic spread of cancer cells in the circulation and to avoid the excessive trapping of cells in the lungs that is seen when a tail-vein injection is used. Cells were suspended in 0.1 mL of HBSS and image guided injections were performed using a Vevo 2100 ultrasound system (VisualSonics Inc., Toronto, ON, CAN). Tumour volume was manually measured with calipers in two perpendicular dimensions, and the volume was estimated using the following formula = 0.52 (width)2 (length), to approximate the volume of an ellipsoid (mm3).26,27
MPI acquisition
Full body magnetic particle images of tumour-bearing mice were acquired 72 hours following intracardiac injection of MPIO-labeled CTCs (day 44). Images were collected on a Momentum™ scanner (Magnetic Insight Inc., Alameda, CA, USA) using the 3D high sensitivity scan mode. In this mode, tomographic images were acquired using a 3 T m−1 gradient, 35 projections and a FOV 12 × 6 × 6 cm, for a total scan time ∼1 hour per mouse. Mice were anesthetized with 2% isoflurane in 100% oxygen during these scans. 3D high sensitivity images of ex vivo tumours were acquired using the same parameters.
MPI calibration and signal quantification
A calibration line was generated to determine the relationship between the MPI signal and iron content. Samples were made with 1 μL aliquots of MPIO beads and imaged using the same parameters as in vivo images. The following samples of iron content were tested: 0.7 μg, 1.05 μg, 1.4 μg, 2.1 μg, and 2.8 μg of iron. All five samples were scanned together, separated by 2 cm on the MPI bed.
Images were analyzed utilizing Horos imaging software (Annapolis, MD, USA). The MPI signal intensities were set to full dynamic range to best represent the full range of signal in a specific region of interest (ROI; i.e. calibration samples or tumours), prior to manually outlining the signal. The signal intensities from the in vivo images had to be adjusted to visualize tumour signal, decreasing window level and width to compensate for the high liver signal (max = 0.133, min = 0.01). Areas of interest from in vivo 3D images were manually outlined, slice by slice, creating a 3D volume. These ROIs (determined from tumour signal) were copied and pasted onto the contralateral MFP of the same mouse to assure an equivalent volume of control tissue was used for quantification of signal. Total MPI signal was calculated by mean signal × volume (mm3). To derive the calibration line the total MPI signal was plotted against known iron content of the samples and a linear regression was performed. The iron content in tumours and contralateral MFPs was calculated by Iron content (μg) = Total signal/Slope of calibration line.
MRI acquisition
MRI scans were performed on a 3 T MR750 clinical scanner (General Electric) equipped with a custom-built, insertable gradient coil and mouse brain solenoidal radiofrequency coil.13,16 Tumour samples were tightly placed in MR compatible tubes to avoid motion artifacts. Images were acquired using a balanced Steady State Free Precession (bSSFP) imaging sequence [Fast Imaging Employing Steady State Acquisition (FIESTA) on the GE system] which has been previously optimized for iron detection.28 The scan parameters were: repetition time (TR) = 7 ms, echo time (TE) = 3.5 ms, bandwidth (BW) = 31.25 kHz, flip angle (FA) = 35 degrees, averages (NEX) = 2, phase cycles = 4, matrix = 200 × 200. Total scan time was approximately 25 minutes per sample.
Histology and immunohistochemistry
Following imaging, all mice were sacrificed by isoflurane overdose and perfused with 4% paraformaldehyde. Tumours were excised and placed in paraformaldehyde for an additional 24 hours. Fixed tissue was processed, paraffin embedded and cut into 10 μm sections. Select sections were stained for iron with Perl's Prussian blue (PPB) or stained for GFP by immunohistochemistry.
Statistical analysis
Statistics were calculated using GraphPad Prism 7 Software. Pearson's rank correlation was used to determine the relationship between total MPI signal and iron content. In vivo data was expressed as mean ± SEM and analyzed by a Student's t test. Differences were considered statistically significant at *p < 0.05.
Results
In vitro studies
Fig. 1A shows the MPI relaxometry data for MPIO and Vivotrax which provides information on iron particle sensitivity and resolution. The amplitude of the PSF for MPIO was higher than Vivotrax; the relative sensitivity was 1.5 for MPIO compared to 1.0 for Vivotrax. The FWHM was larger for MPIO; the calculated resolution for MPIO was 4.5 mm versus 1.7 mm for Vivotrax, using a 6.1 T m−1 gradient. MDA-MB-232BR-GFP cells were efficiently labeled with MPIO. The Perl's Prussian blue stain shows intracellular iron in blue within the breast cancer cells that appear pink (Fig. 1B). Images acquired of five MPIO samples with known iron content were acquired to determine the relationship between MPI signal and iron content (Fig. 1C). A linear relationship between iron content and MPI signal was observed (R2 = 0.99, p < 0.0001) (Fig. 1D). This calibration line was used to quantify iron content of MPIO labeled MDA-MB-231BR cells in in vivo and ex vivo images.
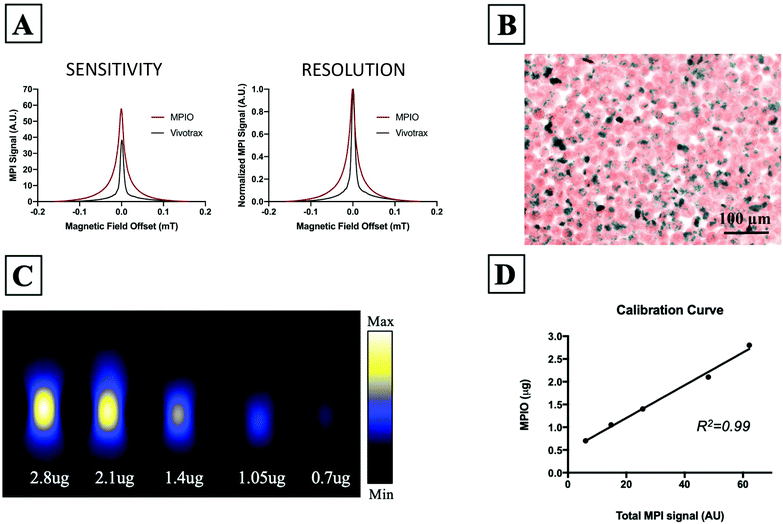 |
| Fig. 1 (A) MPI relaxometry data provides information on SPIO sensitivity and resolution. The signal amplitude of the PSFs for MPIO and Vivotrax show that the relative sensitivity of MPIO is 1.5 compared to 1.0 for Vivotrax. The resolution of MPIO was 4.5 mm and the resolution of Vivotrax was 1.7 mm. (B) Experimental CTCs were efficiently labeled with MPIO as seen with PPB staining (iron = blue). (C) Images of samples with known iron content were used to generate a calibration curve for quantification purposes. (D) A linear relationship between iron content and MPI signal was observed (R2 = 0.99, p < 0.0001). The slope of this line was used to calculate iron content from MPI signal, along with the total MPI signal measured from the images. | |
In vivo studies
Fig. 2A shows magnetic particle images of tumour bearing mice 72 hours after receiving 5 × 105 MPIO labeled MDA-MB-231BR cells. These images were scaled to display the full dynamic range of signal across all mice. MPI signal can be clearly visualized in the lower right MFP tumour. Signal was also detected in lungs of some of the mice, likely due to iron labeled cells that were trapped following intracardiac injection, as well as, signal in the abdomen, presumably due to iron in the mouse feed and/or in the liver. The distribution of MPI signal throughout the body can be visualized by scrolling through the complete 3D dataset (Video file 1). We found that iron content in the lower right MFP (tumour bearing) (M = 0.8 ± 0.2 μg) was significantly higher than in the contralateral MFP (M = 0.3 ± 0.1 μg). The average tumour volume measured by calipers was 230 ± 40 mm3. We calculated the average MPIO cell loading to be 30 pg per cell. This corresponds to 30
000–70
000 cells at the tumor bearing MFP.
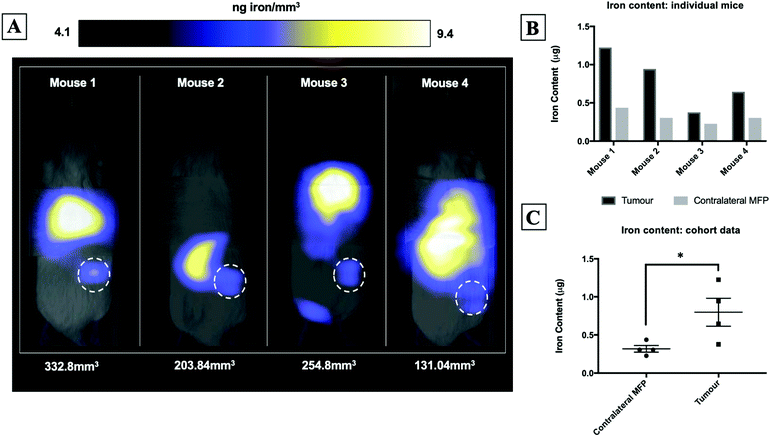 |
| Fig. 2 MPI signal, representing iron-labeled experimental CTCs, was detected in the lower right MFP tumour of all mice (A). MFP tumour burden (mm3) measured by calipers is shown directly below each mouse. Iron content in the lower right MFP (tumour bearing) (M = 0.8 ± 0.2 μg) was significantly higher than in the contralateral MFP (M = 0.3 ± 0.1 μg) (B/C). | |
Ex vivo studies
MPIO labeled MDA-MB-231BR cells were also visualized within MFP tumours using ex vivo imaging (Fig. 3). In magnetic particle images, the iron distribution of 3 different tumour samples of varying sizes were visualized (Fig. 3A/B/C). The average iron content per tumour was 1.5 ± 0.2 μg (Fig. 3B). Iron labeled cells were also visualized throughout tumours as regions of signal void using iron-sensitive ex vivo MRI (Fig. 3D).
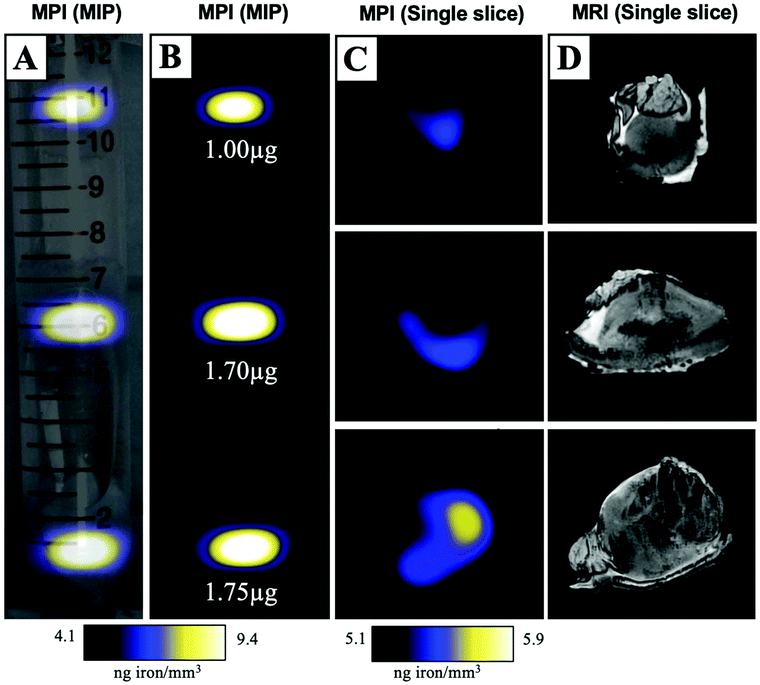 |
| Fig. 3
Ex vivo tumour samples were placed in a 15 mL falcon tube and imaged with MPI and MRI. A maximum intensity projection (MIP) of MPI signal was overlaid onto a brightfield image for anatomical reference (A/B). Individual slices from the 3D MPI dataset were qualitatively assessed for correspondence with areas of signal void in MRI (C/D). | |
Microscopy and immunohistochemistry
Mice were sacrificed 44 days after MFP cell injection. Iron labeled cancer cells were visualized within the MFP tumour using PPB staining (Fig. 4A). Immunostaining of adjacent sections demonstrated that the location of GFP positive cells corresponded with the location of iron (Fig. 4B). This suggests the MPI and MRI signal we are seeing in iron-based imaging techniques is from iron-labeled, GFP expressing CTCs throughout the tumour.
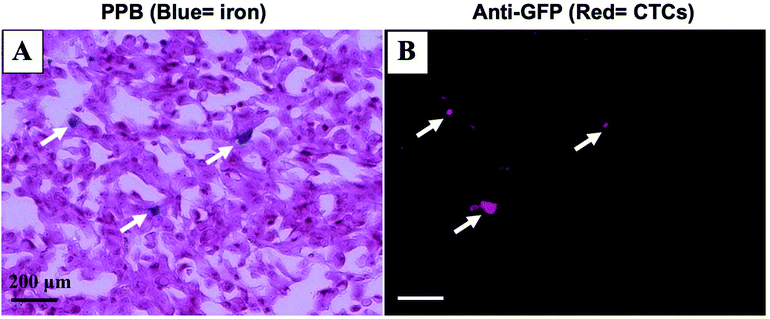 |
| Fig. 4 Iron labeled CTCs (blue) were visualized within the MFP tumour using PPB staining (A). Immunostaining of adjacent tumour sections confirmed the presence of GFP positive cells (red) including some that correspond with the location of iron (B). (scale bar 200 μm). | |
Discussion
A number of different cell-based vectors have previously been developed for the targeted delivery of anti-cancer therapeutics to primary and metastatic lesions.29–35 While some cell types have shown promise due to their innate homing capabilities (i.e. Immune cells and stem cells), new strategies to overcome intratumoural immunological barriers and on-target off-tumour effects are urgently needed. CTCs may represent a novel cell-based platform for therapy whereby, they are highly efficient at homing to established tumour sites, can be readily engineered and expanded ex vivo, and could be generated from an individual patient's tumour to avoid an unwanted immune response. Our group and others have shown exciting progress towards the development of self-homing CTCs for the treatment of primary and metastatic lesions however, further study is warranted to optimize self-homing CTCs for clinical translation.3–9 Here, we demonstrate for the first time, the ability of MPI to sensitively track SPIO-loaded experimental CTCs and to visualize tumour self-homing in a murine model of human breast cancer.
In this work, we detected iron-labeled MDA-MB-231BR-eGFP cells that had migrated to an MDA-MB-231 primary tumour, which we hypothesized may be due to a well-established tumour microenvironment. Previous work by Kim et al., has shown that an MDA-MB-231 tumour can produce chemoattractants, IL-6 and IL-8, that are capable of actively recruiting CTCs from the bloodstream.36 Additionally, our group and others have previously shown using TNBC models that metastatic variants are more likely to return to seed an established primary tumour compared to the respective parental cell line.9,36 Vilalta and colleagues have also shown in the murine 4T1 breast cancer model that irradiation of tumours induces the expression of granulocyte-macrophage colony stimulating factor (GM-CSF) that can act to enhance the recruitment of CTCs.37 Their evidence suggests radiation induced self-homing may act as a potential mechanism for cancer recurrence in the clinic. Future work will look to investigate how the production of these cytokines relates to the amount of CTC self-homing, and whether we can visualize these differences over time with MPI.
Previous studies by Kim et al., have suggested a linear relationship may exist between primary tumour burden and the number of CTCs that home to a tumour site such that, a larger tumour will effectively recruit more CTCs than a smaller tumour.36 In our model, the primary MDA-MB-231 MFP tumour had 41 days to grow prior to the administration of experimental CTCs. Despite observing a range in primary tumour volumes across mice, a clear relationship between MPI signal, representing the amount of CTC homing, and the size of the MFP tumour was not observed. This may be due to variability in the number of CTCs that reach the tumour site following IC injection, probe dilution, or imaging prior to all CTCs reaching the tumor site. Furthermore, the animal having the smallest measured tumour burden (approximately 133 mm3) had MPI signal far above our estimated detection threshold, suggesting MPI may have the capability to detect much fewer CTCs than shown in this study. Future work should look to decrease tumour burden as well as alter the number of CTCs that are administered to better determine the sensitivity of MPI for detecting and longitudinally monitoring self-homing CTCs in vivo. Additionally, understanding the ratio of tumour cells to self-homing CTCs needed for a therapeutic effect will be crucial when moving towards the use of self-homing CTCs for the treatment of primary and metastatic lesions.
Our group and others have previously applied cellular MRI to track various iron-loaded cell types in vivo including immune cells, stem cells, cancer cells and pancreatic islets. Previous work has shown that labeling cancer cells with iron does not cause significant changes in cell viability, proliferation, apoptosis or metastatic efficiency making it an ideal probe for clinically relevant imaging studies.16,38 However, iron-based cell tracking techniques provide a limited imaging window as the probe gets diluted through cancer cell division.15 In this work, we performed MPI 72 hours following systemic injection of iron-labeled experimental CTCs. While we saw substantial MPI signal in all of the tumours, signal could be further enhanced by optimizing the timeline to visualize self-homing prior to probe dilution. Furthermore, we observed MPI signal in the abdomen of all mice. This may be a result of having iron in the mouse feed or having iron-labeled CTCs elsewhere in the body, either in the form of metastases or trapped cells following injection. This was not detrimental to our study since the tumour bearing MFP is far enough away from the abdomen to avoid signal overlap however, an iron-free diet as well as alternative injection routes should be considered for future studies. Optical imaging could also be employed in future studies to differentiate between signal generated from engineered CTCs and other sources of iron within the body.
Conclusions
In this work, we employed high sensitivity MPI to noninvasively visualize SPIO-loaded experimental CTCs in tumour bearing mice. Further, we demonstrate a clinically relevant model of tumour self-homing whereby, SPIO-labeled CTCs migrated, and were detected at the established MFP tumour site 72 hours following systemic administration. Future work will look to further build MPI as a valuable tool for visualizing whole-body tumour self-homing, which will be extremely valuable in understanding the fate of therapeutic CTCs and the potential mechanisms driving tumour self-homing throughout the body.
Conflicts of interest
There are no conflicts of interest to declare.
Acknowledgements
The authors would like to acknowledge funding from the Canadian Foundation for Innovation. Some figures were created with BioRender.com.
References
- L. Norton and J. Massagué, Nat. Med., 2006, 12, 875–878 CrossRef CAS PubMed.
- P. Carmeliet and R. K. Jain, Nature, 2000, 407, 249–257 CrossRef CAS PubMed.
- Z. Raykov, G. Balboni, M. Aprahamian and J. Rommelaere, Int. J. Cancer, 2004, 109, 742–749 CrossRef CAS PubMed.
- A. T. Power, J. Wang, T. J. Falls, J. M. Paterson, K. A. Parato, B. D. Lichty, D. F. Stojdl, P. A. Forsyth, H. Atkins and J. C. Bell, Mol. Ther., 2007, 15, 123–130 CrossRef CAS PubMed.
- S. M. Freeman, C. N. Abboud, K. A. Whartenby, C. H. Packman, D. S. Koeplin, F. L. Moolten and G. N. Abraham, Cancer Res., 1993, 53, 5274–5283 CAS.
- J. Garcia-Castro, J. Martinez-Palacio, R. Lillo, F. Garca-Sanchez, R. Alemany, L. Madero, J. A. Bueren and M. Ramirez, Cancer Gene Ther., 2005, 12, 341–349 CrossRef CAS PubMed.
- E. Dondossola, A. S. Dobroff, S. Marchi, M. Card.-Vila, H. Hosoya, S. K. Libutti, A. Corti, R. L. Sidman, W. Arap and R. Pasqualini, Proc. Natl. Acad. Sci. U. S. A., 2006, 113, 2223–2228 CrossRef PubMed.
- C. Reinshagen, D. Bhere, S. H. Choi, S. Hutten, I. Nesterenko, H. Wakimoto, E. Le Roux, A. Rizvi, W. Du, C. Minicucci and K. Shah, Sci. Transl. Med., 2018, 10(449), eaao3240 CrossRef PubMed.
- K. M. Parkins, V. P. Dubois, J. J. Kelly, Y. Chen, P. J. Foster and J. A. Ronald, Theranostics, 2020, 10(17), 7925–7937 CrossRef CAS PubMed.
- J. M. Gaudet, A. M. Hamilton, Y. Chen, M. S. Fox and P. J. Foster, Magn. Reson. Med., 2017, 78, 713–720 CrossRef CAS PubMed.
- A. V. Makela, D. H. Murrell, K. M. Parkins, J. Kara, J. M. Gaudet and P. J. Foster, Top. Magn. Reson. Imaging, 2016, 25, 177–186 CrossRef PubMed.
- A. Khurana, H. Nejadnik, R. Gawande, G. Lin, S. Lee, S. Messing, R. Castaneda, N. Derugin, L. Pisani, T. F. Lue and H. E. Daldrup-Link, Radiology, 2012, 264, 803–811 CrossRef PubMed.
- C. Heyn, C. V. Bowen, B. K. Rutt and P. J. Foster, Magn. Reson. Med., 2005, 53, 312–320 CrossRef PubMed.
- K. M. Parkins, A. M. Hamilton, A. V. Makela, Y. Chen, P. J. Foster and J. A. Ronald, Sci. Rep., 2016, 6, 1–9 CrossRef PubMed.
- V. Economopoulos, Y. Chen, C. McFadden and P. J. Foster, Transl. Oncol., 2013, 6, 347–354 CrossRef PubMed.
- C. Heyn, J. A. Ronald, S. S. Ramadan, J. A. Snir, A. M. Barry, L. T. MacKenzie, D. J. Mikulis, D. Palmeri, J. L. Bronder, P. S. Steeg and T. Yoneda, Magn. Reson. Med., 2006, 56, 1001–1010 CrossRef PubMed.
- J. W. M. Bulte, Adv. Drug Delivery Rev., 2019, 138, 293–301 CrossRef CAS PubMed.
- E. Y. Yu, M. Bishop, B. Zheng, R. M. Ferguson, A. P. Khandhar, S. J. Kemp, K. M. Krishnan, P. W. Goodwill and S. M. Conolly, Nano Lett., 2017, 17, 1648–1654 CrossRef CAS PubMed.
- B. Zheng, T. Vazin, P. W. Goodwill, A. Conway, A. Verma, E. U. Saritas, D. Schaffer and S. M. Conolly, Sci. Rep., 2015, 5, 14055 CrossRef PubMed.
- B. Zheng, P. Marc, E. Yu, B. Gunel, K. Lu, T. Vazin, D. V. Schaffer, P. W. Goodwill and S. M. Conolloy, Theranostics, 2016, 6, 291 CrossRef CAS PubMed.
- H. Nejadnik, P. Pandit, O. Lenkov, A. P. Lahiji, K. Yerneni and H. E. Daldrup-Link, Mol. Imaging Biol., 2019, 21, 465–472 CrossRef CAS PubMed.
- J. W. M. Bulte, P. Walczak, M. Janowski, K. M. Krishnan, H. Arami, A. Halkola, B. Gleich and J. Rahmer, Tomography, 2015, 1, 91–97 CrossRef CAS PubMed.
- O. C. Sehl, A. V. Makela, A. M. Hamilton and P. J. Foster, Tomography, 2019, 5, 367 CrossRef PubMed.
-
J. M. Gaudet, A. V. Makela and P. J. Foster, AACR: Non-invasive detection and quantification of tumor-associated macrophage density with magnetic particle imaging, 2019 Search PubMed.
- X. Y. Zhou, K. E. Jeffris, Y. Y. Elaine, B. Zheng, P. W. Goodwill, P. Nahid and S. M. Conolly, Phys. Med. Biol., 2017, 62, 9 CrossRef PubMed.
- D. M. Euhus, C. Hudd, M. C. LaRegina and F. E. Johnson, J. Surg. Oncol., 1986, 31, 229–234 CrossRef CAS PubMed.
- M. M. Tomayko and C. P. Reynolds, Cancer Chemother. Pharmacol., 1989, 24, 148–154 CrossRef CAS PubMed.
- E. J. Ribot, F. M. Martinez-Santiesteban, C. Simedrea, P. S. Steeg, A. F. Chambers, B. K. Rutt and P. J. Foster, Magn. Reson. Imaging, 2011, 34, 231–238 CrossRef PubMed.
- M. Mimeault, R. Hauke and S. K. Batra, Clin. Pharmacol. Ther., 2007, 82, 673–691 CrossRef PubMed.
- J. Ankrum and J. M. Karp, Trends Mol. Med., 2010, 16, 203–209 CrossRef PubMed.
- K. S. Aboody, A. Brown, N. G. Rainov, K. A. Bower, S. Liu, W. Yang, J. E. Small, U. Herrlinger, V. Ourednik, P. M. Black and X. O. Breakefield, Proc Natl Acad Sci U. S. A., 2001, 97, 777 Search PubMed.
- K. I. Park, B. T. Himes, P. E. Stieg, A. Tessler, I. Fischer and E. Y. Snyder, Exp. Neurol., 2006, 199, 179–190 CrossRef CAS PubMed.
- M. Alieva, J. R. Bago, E. Aguilar, C. Soler-Botija, O. F. Vila, J. Molet, S. S. Gambhir, N. Rubio and J. Blanco, PLoS One, 2012, 7, 4 CrossRef PubMed.
- J. R. Bagó, K. T. Sheets and S. D. Hingtgen, Neural stem cell therapy for cancer, Methods, 2016, 99, 37–43 CrossRef PubMed.
- K. Song, N. Benhaga, R. L. Anderson and R. Khosravi-Far, Cancer Res., 2006, 66, 12 Search PubMed.
- M. Y. Kim, T. Oskarsson, S. Acharyya, D. X. Nguyen, X. H.
F. Zhang, L. Norton and J. Massagué, Cell, 2009, 139, 1315–1326 CrossRef PubMed.
- M. Vilalta, M. Rafat, A. J. Giaccia and E. E. Graves, Cell Rep., 2014, 8, 402–409 CrossRef CAS PubMed.
- R. Rohani, S. N. de Chickera, C. Willert, Y. Chen, G. A. Dekaban and P. J. Foster, Mol. Imaging Biol., 2011, 13, 679–694 CrossRef PubMed.
Footnote |
† Electronic supplementary information (ESI) available. See DOI: 10.1039/d0nr07983a |
|
This journal is © The Royal Society of Chemistry 2021 |