DOI:
10.1039/D0FD00082E
(Paper)
Faraday Discuss., 2021,
226, 138-148
Diurnal and weekly patterns of primary pollutants in Beijing under COVID-19 restrictions
Received
28th June 2020
, Accepted 14th September 2020
First published on 18th September 2020
Abstract
Restrictions on movement in Beijing to limit the COVID-19 epidemic tended to reduce the emissions of primary pollutants. However, changes in pollutant concentrations are also affected by chemical transformation and meteorology. Decreases in concentrations were also not as obvious in Beijing when compared with Hubei Province, where lockdown was especially strict. Declines in concentrations between 2019 and 2020 are evident for both NO2 (37.0 to 26.2 μg m−3) and SO2 (5.86 to 4.15 μg m−3), but there was little evidence of change for PM2.5 (50.0 to 53.8 μg m−3) and CO (0.71 to 0.74 mg m−3). Despite this, Fourier analysis revealed that the weekly cycle of PM2.5 evident in 2019 was not apparent in 2020. In 2019, CO showed both diurnal and weekly signals, but these were absent under the restrictions of 2020. This suggests that while concentrations may remain relatively constant, the temporal distribution of pollutants can show subtle changes under restrictions imposed in attempts to limit the spread of the coronavirus.
Introduction
Urban air pollution is affected by a combination of meteorological factors, chemical processes and the strength of emissions. Emissions are a product of human activity, so substantial changes in daily life affect the concentration of air pollutants.1 The threat from the novel coronavirus, which emerged late in 2019, ultimately led to a pandemic, and subsequent quarantines, restrictions of movement or lockdowns. Although not without historical precedent,2 these were attempts to limit transmission of the disease at a global scale. The widespread social and economic disruption imposed by the restrictions affected the emission of air pollutants and often saw lowered concentrations that were reported in a rapidly growing list of studies from Europe,3,4 India,5 South East Asia,6,7 the US8 and South America.9,10 However, the influence of such controls on air quality was first observed for Hubei Province and more broadly within the Jingjinji megalopolis of China.8,11–16 While the restrictions on human activity and movement seem to have an obvious link to air pollution, it is necessary to recognise the subtlety of the change imposed by the mandated quarantine of entire populations. Many studies have sought to detect reduced air pollutant concentrations during lockdowns by comparing the lockdown period with those immediately before and after, or alternatively comparing 2020 with the same period of the previous year. Nevertheless, such attempts can be sensitive to differences in climate compared to the control period. Cole et al.12 attempted to remove the effects of weather from carefully tuned data and noted that the impact of the lockdown varied between pollutants. Others suggest that the changes were perhaps subtler than the vivid images that were derived from satellite products.17,18
Besides changes in the average concentration of air pollutants, it is possible that the cyclic patterns of urban air pollutant concentrations present another way to detect the effect of the coronavirus restrictions. Such cycles are driven by the daily patterns of meteorology, photochemistry and human behavior. Weekly cycles are a product of our working life, with the effects of holidays well known, especially the weekend effect, which arises because pollutant emissions differ between weekdays and weekends. This is a particularly characteristic pollutant pattern driven by human activity. The concentrations of pollutants such as CO, NOx, NO, SO2 and PM2.5,10 are typically found to be higher on weekdays, because of rush hour traffic, work patterns and industrial activities, but are also influenced by population and urbanization.19–21 Coal-fired power plants are a particularly important source of sulfur dioxide, which may vary during the day or week because of changed electricity demand. Ozone, as a secondary photochemical pollutant, shows more complex behavior: lower on weekdays than on weekends, most often because of titration by NO on weekdays.22 However, sometimes the concentration can be higher during the week.23,24 Sicard et al.25 have described the weekend effect in relation to ozone and used this as a way of assessing enhanced ozone concentrations in cities, due to the unprecedented reduction in NOx emissions and decreasing titration by NO.
This paper will explore the impact of changed social patterns on air pollution in Beijing during the early months of 2020. There was no mandatory lockdown in the megacity, but the government pressed people to remain indoors and working life changed, while outdoors social distancing was adopted. In February, with concern over COVID-19 at its height, non-essential workers were encouraged to remain home. Those working in core enterprises and government facilities (e.g. hospitals, electric power companies, the police, etc.) were to work normally and for some the period was exceptionally busy. The balance between those working at home and those working normally is difficult to establish. Authorities cancelled events and closed some 130 major tourist attractions, including the Forbidden City, the Palace Museum and the National Maritime Museum. Iconic and historic places often remained closed until the end of April.
The approach adopted in Beijing was more relaxed than the stricter policies of Wuhan and Hubei Province, where large changes in air pollutant concentrations were widely reported.12,13 Changes in air pollution in Beijing could readily be assumed to be less striking,17 so detection would need to be more careful in separating the effect of changes in emissions from the effect of changes in meteorology. In particular, it seems advantageous to examine changes in variability and pollutant cycles in the hope that these may be a more robust approach to determining the impact of lifestyle change and disruptions imposed as a response to the coronavirus. Specifically, the work presented here will use Fourier analysis to examine the diurnal and weekly patterns, the latter being potentially rather resistant to meteorological effects. The analysis here is restricted to the primary pollutants NO2, PM2.5, CO and SO2, so neglects secondary pollutants such as O3. Ozone may have increased during the periods of emission reduction as titration by NO decreased,25 but is avoided here because of the complexity of a pollutant strongly influenced by chemistry and photochemistry.
Method
Data and sources
The lockdown and restrictions adopted to reduce the spread of the coronavirus during the recent pandemic led China to enforce a lockdown in Hubei Province. Wuhan, a city of more than 11 million people was at the centre of the pandemic, so it became necessary to contain the disease by sealing off large parts of Hubei Province beginning on the morning of 23 Jan 2020. Thus for the first time, a megacity entered lockdown, and soon after, travel restrictions were imposed more widely. The streets of the great city on the Yangtze River became silent, almost a ghost town. As the wider significance of the epidemic emerged, The People’s Daily26 reported that the Standing Committee of China’s Central Committee met under the chairmanship of Xi Jinping at Chinese New Year, Saturday 25 January. It required that “leading officials must always stay on their jobs and stand at the frontline to safeguard social stability and ensure people have a peaceful and merry Chinese New Year festival”. Nevertheless, it was a difficult time with many celebrations cancelled. The statutory holiday period in mainland China starts on the Eve of Chinese New Year (set by the lunar calendar as 5 Feb 2019 and 25 Jan 2020) and covers seven days, although in 2020, this was extended by two further days because of the coronavirus epidemic. Much of the work presented here has focused on a period of restriction that corresponds to the lockdown period adopted in Wuhan and runs for 75 days from 24 Jan 2020 to 7 April 2020. In the case of the comparison period in 2019, which was not a leap year, the 75 days are extended to include April 8th. Air pollution measurements are available for 11 stations within Beijing’s 5th Ring Road and 11 outside, and these can be accessed on the website: http://aqicn.org/city/
Data analysis and statistics
The Fourier analysis used the fast Fourier transform (fft) available in Scilab 6.0.2 as part of its signal processing functions. There were gaps in the data and this would be best handled by the Lomb–Scargle algorithm. However, as the gaps in the data averaged across the Beijing sites were small, we chose to linearly interpolate these from neighbouring points using the interpolation function (interpln) available in Scilab. The statistical calculations also benefited from online tools, notably Vassarstats: http://vassarstats.net/
Results and discussion
Societal changes
Fig. 1 shows the changes in coal use, electricity generation and passenger and freight transport during the period of interest. The variation in monthly electricity generation for Beijing is shown in Fig. 1a. Initially it appears that there is little difference in the annual cycle between the two years. However, while the form is the same each year, the amounts are slightly different for each year, falling from a monthly value of 39.6 GW h in March 2019 to 37.1 GW h in March of 2020, an almost 10% decrease. Additionally, coal consumption across China significantly decreased, as widely commented on by the media, because the normal recovery after Chinese New Year was much delayed in 2020. Even when recovery occurred, it was to lower levels than normal for China. This is in line with more general observations globally, which have seen lowered energy demands during the COVID-19 restrictions. The changing electricity demand in Europe is well described by Narajewski and Ziel.27 In parallel there were reductions in carbon dioxide emissions.15 The images of the times of COVID-19 have often been pictures of roads in major cities free of traffic and almost abandoned to wildlife; in some cases lions have been seen sleeping on the roads in South Africa. In China there is evidence of reduced travel in terms of road passenger numbers in Beijing (Fig. 1b), which indicate dramatic declines from 2019 to 2020. Although February 2020 saw less road freight in Beijing than the year before, a rapid recovery was seen in March and April, when the quantities exceeded those for the same months of the previous year.
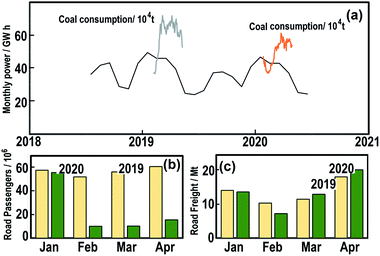 |
| Fig. 1 (a) Monthly electricity generation for Beijing and Chinese daily coal consumption. (b) Monthly numbers of road passengers in Beijing for 2019, shown as light bars, and 2020, shown as darker bars. (c) Monthly road freight in Beijing for 2019, shown as light bars, and 2020, shown as darker bars. | |
Temporal changes in primary pollutants
Fig. 2 shows averaged measurements of air pollutants from the monitoring stations (black diamonds) from January to the end of April in both 2019 and 2020, in and around Beijing smoothed with a 25 hour running mean. The smoothed standard deviations are marked as light coloured lines giving the upper and lower limits (negative lower bounds are not shown). The concentrations of NO2 averaged across urban and suburban sites in Beijing across similar periods are shown in Fig. 2a and b. Although the changes between the two years are not particularly striking, they hint at a decrease, which is in line with the reductions in column NO2 that began to be observed over China in satellite images from late January.13 Some reductions in column NO2 are typical around the Chinese New Year holiday, but in 2020 the reduction was especially noticeable.28 Bao and Zhang11 show that there were general improvements in SO2, PM2.5, PM10, NO2, and CO levels across 44 Chinese cities of the Jingjinji megalopolis. The averages of the daily NO2 concentrations measured in Beijing over the 75 days from 24 January were 37.0 ± 17.5 μg m−3 for 2019 and 26.2 ± 11.7 μg m−3 for 2020, a significant difference according to both Welch’s t-test (i.e. for unequal variances) and the Mann–Whitney test at p1 = 0.0001. It sometimes appears as if concentrations were less variable under COVID-19 restrictions,17 but the coefficients of variation (i.e. standard deviation divided by the mean) were similar: 0.47 and 0.45 for 2019 and 2020, respectively. The reductions in NO2 concentrations in Beijing are less impressive than those in Wuhan for example, where a lockdown was strictly enforced and the weekday NO2 level dropped from 45.4 μg m−3 in 2019 to 21.2 μg m−3 under lockdown.17 In Beijing the differences in NO2 concentrations may be more apparent at sites within the 5th Ring Road than those beyond.17
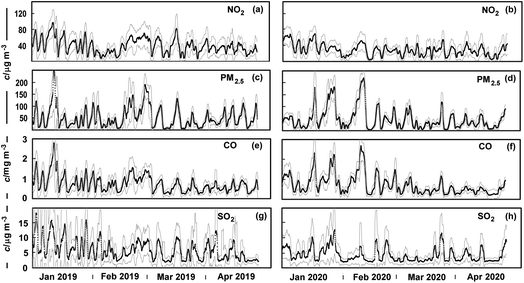 |
| Fig. 2 Average hourly measurements from the 22 monitoring stations across Beijing for January to April: (a) NO2 2019, (b) NO2 2020, (c) PM2.5 2019, (d) PM2.5 2020, (e) CO 2019, (f) CO 2020, (g) SO2 2019 and (h) SO2 2020. Note: these are 25 point running means and the grey lines represent the upper and lower bounds of the standard deviation. | |
The average PM2.5 concentrations across all 22 sites in Beijing for 2019 and 2020 do not appear to show a distinct improvement for 2020 (Fig. 2c and d). Indeed, the concentrations are a little higher in the more recent year: 50.0 ± 40 μg m−3 for 2019 and 53.8 ± 50 μg m−3 for 2020. The data has a large coefficient of variation, so it is hardly surprising that neither the t-test nor the Mann–Whitney test suggests a significant difference. The production of particulate material from celebratory fireworks used in the outlying parts of Beijing was especially notable in 2020, which may be the reason for the high concentrations over the Chinese New Year holiday in 2020.17 As with PM2.5 the concentrations of CO (Fig. 2e and f) do not appear to be substantially reduced across the 75 day period and the statistics for 2019 and 2020 bear this out: 0.71 ± 0.34 mg m−3 and 0.74 ± 0.51 mg m−3 with p1, t-test = 0.35; p1, MW = 0.23. Sulfur dioxide shows rather larger differences between the two years, and over the 75 day period the averages across the 22 Beijing sites were 5.86 ± 3.0 μg m−3 and 4.15 ± 2.4 μg m−3, a significant difference according to both the t-test and the Mann–Whitney test at p1 = 0.0001. This very much aligns with views that the changes under COVID-19 restrictions may be rather more nuanced than popularly thought and not equally found among different pollutants.12,17,18
This subtle difference between the COVID-19 period and previous years was explored by Cole et al.12 who reduced the effects of weather using a decision-tree-based random forest regression model.29 A number of groups have explored the changes due to the lockdown, comparing over a larger number of years,12,17,30 but this becomes complicated by the reduction of pollutant concentrations as Beijing increasingly reduced emissions to improve the city’s air quality. Here we have used Fourier analysis to assess the usefulness of cyclic variations in distinguishing the role of patterns of human behaviour in air pollutant concentrations. Although widely used in the geophysical sciences, Fourier analysis is less common in air pollution studies, although it has been used to investigate time series31 or to undertake spatial analyses.32 The approach here attempts to take advantage of shifts in the daily and weekly patterns of human activities, which can be rather different to those imposed by meteorology.
Cyclic patterns in pollutants
Fourier transforms of the 75 day hourly average concentrations across Beijing for similar periods each year are shown in Fig. 3. The analysis breaks the time series of concentrations down into the possible cycles and determines the power at different frequencies and to a lesser extent the phase shifts. Seventy-five days is 1800 hours, but the Nyquist frequency sets the highest frequency resolved to be half that of the sampling rate, so in this case 0.5 h−1. However, we are not so interested in these higher frequencies in this work as it focuses on the daily cycle (0.0417 h−1), a signal which would show 75 cycles in the record of 1800 hours, and the weekly cycle with a frequency of ∼0.006 h−1, showing 10.5 cycles within the 75 day record. These two important frequencies are marked on the x-axis of Fig. 3.
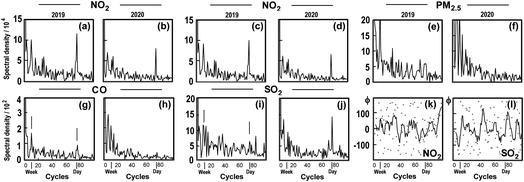 |
| Fig. 3 Fourier transforms of hourly concentration data across Beijing for 75 days starting on 24th January: (a) NO2 2019 within 5th Ring Road 2019, (b) NO2 2020 within 5th Ring Road and across all the sites 2019, (c) NO2, (d) NO2, (e) PM2.5 2019, (f) PM2.5 2020, (g) CO 2019, (h) CO 2020, (i) SO2 2019 and (j) SO2 2020, and the phase angle, in degrees for (k) NO2 within 5th Ring Road 2020 and (l) SO2 2020, where the smoothed trend line added is a 5-point running mean. Note: the x-axis shows the number of cycles in the 75 day record, so 75 marks the daily cycle, and the weekly cycle is expected at 10–11 as marked. The y-axis in figures (a)–(j) denotes spectral power which takes the units of concentration squared. The y-axis in figures (k) and (l) is the phase angle in degrees. | |
Fig. 3a and b show the low frequency spectrum (<0.06 h−1) in which the daily cycle is marked at 75, i.e. there are 75 cycles in the 1800 h record. The NO2 concentrations measured for 75 days at the 11 sites within Beijing’s 5th Ring Road in 2019 and 2020 show a strong diurnal signal (apparent at 75 cycles). It is evident in 2019, but it is also found during the COVID-19 period, although perhaps a little weaker, since the concentrations are lower. Nevertheless, under COVID-19, the diurnal pattern still stands out clearly. There is a weekly signal at 10 cycles in the record, but this is less prominent under COVID-19, no doubt reflecting the breakdown of the weekly cycle because of the limits imposed on outdoor activities and work routines. The analysis is repeated over all 22 sites in Beijing and reveals a similar picture (Fig. 3c and d); although the signal may be a little weaker, with slightly lower concentrations, the daily cycles remain clear.
The Fourier analyses of PM2.5 concentrations obtained from the hourly records averaged across all the 22 Beijing sites for 2019 and 2020 are shown in Fig. 3e and f. There is no evidence of a diurnal signal either for 2019 or under the COVID-19 restrictions of 2020. However, there is evidence of a weekly signal in 2019, but this vanishes in 2020. This is interesting because the simple averaged record taken over the 75 day periods suggested no significant difference in PM2.5 concentrations between the two years (i.e. 2019: 50 ± 40 μg m−3 and 2020: 54 ± 50 μg m−3). In Beijing, the intense working habits mean that typically, people in the metropolis are busy at work during workdays, so spend much time on shopping during the weekend, which means traffic volume can be high,33 and this may drive a characteristic weekly cyclic pattern.
Carbon monoxide shows both diurnal and weekly signals in 2019 (Fig. 3g), but nothing prominent under the COVID-19 restrictions of 2020 (Fig. 3h). However, the much discussed decrease in traffic flow likely led to an absence of diurnal and weekly cycles for traffic-generated CO under the COVID-19 restrictions. It is true that during 2020 there is a peak at 10 (i.e. about ten weekly cycles across the 75 day period), but the peak lies buried among other equally dominant peaks that represent low frequency cycles with 4 day, 5 day and 2 week periodicities. As with PM2.5, Fourier analysis of the CO records reveals a change that wasn’t evident when simply comparing mean concentrations (i.e. 2019: 0.71 ± 0.34 mg m−3 and 2020: 0.74 ± 0.51 mg m−3). The loss of the diurnal and weekly cycles in the record for 2020 fits well with the changed passenger travel shown in Fig. 1b.
Fig. 3i and j show the low frequency spectra for SO2 for the 22 Beijing sites for both 2019 (Fig. 3i), and 2020 (Fig. 3j). There is little evidence for either daily or weekly cycles in 2019, where any hints are lost in noise, but in 2020 a strong diurnal cycle emerges. This is likely a period when many industrial sources of SO2 were closed, but the background sources, such as power stations, had a regular diurnal output. Typically, electricity demand is lowest in the early hours of the morning, but rises to a peak during the day.34,35
It is possible to extract phase information in addition to frequency information from Fourier transforms. This is a little frustrating to interpret as the signal can be noisy, so here it has been smoothed with a 5-point running mean. In the case of NO2 in 2020 (Fig. 3k) the data suggests that the diurnal signal shows a phase angle, which is negative, suggesting that at midnight concentrations are low, but starting to rise. Sulfur dioxide has a phase angle that is also negative, although it varies greatly, which again suggests that concentrations are low but rising at midnight. This would be in agreement with typical urban electricity demand cycles and supports the contention made in the previous paragraph that the clear diurnal signal for SO2 which emerged in 2020 was the product of a predominant electricity generation source for SO2.
Fig. 4 explores the Fourier transform for SO2 further, by filtering out all but the signal that represents the daily cycle (i.e. with a wavelength between 23 and 25 hours). The averaged measured SO2 concentrations for the 75 day period are shown as hourly points in Fig. 4a and b for 2019 and 2020, respectively. The contribution from the daily cycle for each of the years is shown in Fig. 4c and d. This was derived by removing all other frequencies from the Fourier transform and inverting it to give the output waveform shown. It gives a hint of the strength of the daily source of SO2 across the 75 day record, with a more powerful daily signal in 2020. The inset shows the cycle over the first two days of the record, where it is evident that at midnight the SO2 concentrations are low but rising, in agreement with the phase angle depicted in Fig. 3l.
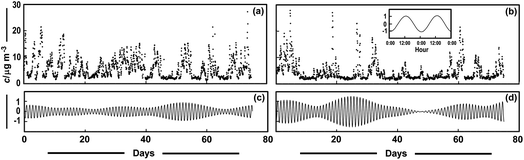 |
| Fig. 4 Daily average SO2 concentrations over 75 days starting on 24th January of 2019 (a) and 2020 (b), with concentration signals with a daily periodicity (i.e. 23–25 h) displayed for both 2019 (c) and 2020 (d). The inset of (b) shows the concentration signal with a daily periodicity for 24th and 25th January. | |
Fig. 5 shows the diurnal cycles for the air pollutants over the 75 day period averaged over the 22 Beijing sites and bears out some of the results from the Fourier analysis. The average NO2 concentrations for each hour of the day are shown in the histogram in Fig. 5a where the light bars represent the data from 2019 and the dark bars represent the year 2020. Although the year with so many restrictions because of COVID-19 has lower concentrations, the strong and similar diurnal pattern for NO2 is clear for both years. The Fourier analysis in Fig. 3e suggested that PM2.5 should have little diurnal variation, so the differences throughout the day apparent in Fig. 5b tend to reflect only slight changes. For CO, in 2019 the Fourier analysis in Fig. 3g suggests diurnal changes, which are apparent in the light bars in Fig. 5c, which shows larger concentrations in the morning, particularly associated with typical rush hour traffic. Under the COVID-19 restrictions, CO was more evenly spread throughout the day. Although on average concentrations were relatively high under the restrictions, sharp peaks in CO concentration due to morning rush hour traffic were absent. These peaks in congestion are not particularly distinctive in hourly traffic counts from earlier years, but are certainly in evidence,36 and unfortunately hourly data for the lockdown period is not yet publicly available. Sulfur dioxide shows a peak at midday in 2019, though only slight diurnal variation overall compared to 2020 when the diurnal cycle was stronger and the peak shifted to the middle of the afternoon. Once again we can see that even small changes in concentration can be accompanied by a rather noticeable change in the character of the diurnal pattern. There were slight changes in the weekly patterns as also noted in the discussion of Fig. 4, though these did not have the same explanatory power as the diurnal changes. However, in general, and a little surprisingly, weekends typically showed lower median concentrations than weekdays in 2020, though this was not always particularly significant (Mann–Whitney test for NO2p1 = 0.058; PM2.5p1 = 0.13; CO p1 = 0.0985 and SO2p1 = 0.0465). By contrast, across the same period in 2019, the weekend concentrations were higher, though not significantly so. Curiously, in 2020 SO2 shows high concentrations early in the week that are not easy to explain.
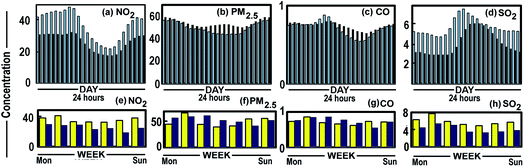 |
| Fig. 5 Diurnal profiles as hourly averages over 75 days starting on 24th January of 2019 (light bars) and 2020 (dark bars) for (a) NO2, (b) PM2.5, (c) CO and (d) SO2. Weekly profiles are shown for (e) NO2, (f) PM2.5, (g) CO and (h) SO2 for 2019 (light bars) and 2020 (dark bars). Note: the units are all μg m−3, except for CO where the units are mg m−3. | |
Conclusions
It is frequently argued that pollutant exposure has been reduced with lower emissions under the restrictions imposed to reduce infections by the novel coronavirus. It seems likely that there were some improvements, but these may not have been as deep or as widespread as popularly reported. Early reports did not always account for changes in weather conditions or long-term reductions in emissions that may occur year by year; reductions were readily attributed to COVID-19, at times with only limited statistical support. These issues may have led to the reductions being more strongly asserted than was warranted. A proper assessment of air quality under lockdown and other restrictions may be needed, so it is worth considering ways of undertaking this which might use dispersion models, consider back trajectories, or adopt neural networks or the signal processing suggested here, to obtain more reliable estimates of the origins of any change. The diurnal and weekly patterns of human behaviour leave traces in the pollutant concentration record, which provides a potential method for reducing the influence of meteorological variation. Careful analysis may become more important given predictions of a second wave of infections, which may well be more localised, as they have been in the Fengtai district of Beijing around Xinfadi Market in June, 2020. While the secondary pollutant ozone has been neglected here, it likely shows strong diurnal change, additionally influenced by photochemistry. It is something worthy of investigation in future, given the likely increases in ozone concentrations under lockdown. A better assessment of the pollution over time contributes to a growing understanding that is likely to be relevant in understanding increased health risks during exposure to the virus. The COVID-19 pandemic has had a wide range of environmental impacts, which seem relevant for future study.
Conflicts of interest
There are no conflicts to declare.
Acknowledgements
The authors would like to thank Johann Lüder of the Department of Materials and Optoelectronic Science at National Sun Yat-Sen University for reminding them of some of the fundamental issues in Fourier transformations.
Notes and references
- H. Mayer, Atmos. Environ., 1999, 33, 4029–4037 CrossRef CAS.
- P. Brimblecombe, City and Environment Interactions, 2020, 100028 Search PubMed.
-
A. Lewis, Air pollution falling across UK cities, latest data shows, National Centre for Atmospheric Science, 2020, https://www.ncas.ac.uk/en/18-news/3057-air-pollution-falling-across-uk-cities-latest-data-shows Search PubMed.
- A. Tobías, C. Carnerero, C. Reche, J. Massagué, M. Via, M. C. Minguillón, A. Alastuey and X. Querol, Sci. Total Environ., 2020, 726, 138540 CrossRef.
- S. Sharma, M. Zhang, J. Gao, H. Zhang and S. H. Kota, Sci. Total Environ., 2020, 728, 138878 CrossRef CAS.
- S. Abdullah, A. A. Mansor, N. N. L. M. Napi, W. N. W. Mansor, A. N. Ahmed, M. Ismail and Z. T. A. Ramly, Sci. Total Environ., 2020, 729, 139022 CrossRef CAS.
- M. S. M. Nadzir, M. C. G. Ooi, K. M. Alhasa, M. A. A. Bakar, A. A. A. Mohtar, M. F. F. M. Nor, M. T. Latif, H. H. Abd Hamid, S. H. Md Ali, N. M. Ariff, J. Anuar, F. Ahamad, A. Azhari, N. M. Hanif, M. A. Subhi, M. Othman and M. Z. Mohd Nor, Aerosol Air Qual. Res., 2020, 20(6), 1237–1248 CrossRef CAS.
- S. Muhammad, X. Long and M. Salman, Sci. Total Environ., 2020, 728, 138820 CrossRef CAS.
- G. Dantas, B. Siciliano, B. B. França, C. M. da Silva and G. Arbilla, Sci. Total Environ., 2020, 729, 139085 CrossRef CAS.
- L. Y. K. Nakada and R. C. Urban, Sci. Total Environ., 2020, 730, 139087 CrossRef CAS.
- R. Bao and A. Zhang, Sci. Total Environ., 2020, 139052 CrossRef CAS.
-
M. Cole, R. Elliott and B. Liu, The Impact of the Wuhan Covid-19 Lockdown on Air Pollution and Health: A Machine Learning and Augmented Synthetic Control Approach, Department of Economics, University of Birmingham, 2020 Search PubMed.
- F. Dutheil, J. S. Baker and V. Navel, Environ. Pollut., 2020, 263, 114466 CrossRef CAS.
- Q. Wang and M. Su, Sci. Total Environ., 2020, 728, 138915 CrossRef CAS.
- X. Yue, Y. Lei, H. Zhou, Z. Liu, H. Letu, Z. Cai, J. Lin, Z. Jiang and H. J. Liao, Transactions of Atmospheric Sciences, 2020, 43, 265–274 Search PubMed.
- K. Xu, K. Cui, L. H. Young, Y. F. Wang, Y. K. Hsieh, S. Wan and J. Zhang, Aerosol Air Qual. Res., 2020, 20, 1204–1221 CrossRef CAS.
- P. Brimblecombe and Y. Lai, Aerosol Air Qual. Res., 2020, 20(11), 2318–2331 CrossRef.
- Q. Schiermeier, Nature, 2020, 580, 313 CrossRef CAS.
- J. C. Cerro, V. Cerda and J. Pey, Atmos. Environ., 2015, 103, 138–146 CrossRef CAS.
- E. M. Martins, A. C. L. Nunes and S. M. Correa, J. Braz. Chem. Soc., 2015, 26, 1967–1975 CAS.
- G. T. Wolff, D. F. Kahlbaum and J. M. Heuss, J. Air Waste Manage. Assoc., 2013, 63, 292–299 CrossRef CAS.
- W. Y. Tang, C. S. Zhao, F. H. Geng, L. Peng, G. Q. Zhou, W. Gao, J. M. Xu and X. X. Tie, Sci. China, Ser. D: Earth Sci., 2008, 51, 1354–1360 CrossRef CAS.
- S. M. Huryn and W. A. Gough, Urban Climate, 2014, 8, 11–20 CrossRef.
- M. Xie, K. G. Zhu, T. J. Wang, P. L. Chen, Y. Han, S. Li, B. L. Zhuang and L. Shu, Sci. Total Environ., 2016, 551–552, 533–545 CrossRef CAS.
- P. Sicard, A. De Marco, E. Agathokleous, Z. Feng, X. Xu, E. Paoletti, J. J. D. Rodriguez and V. Calatayud, Sci. Total Environ., 2020, 139542 CrossRef CAS.
-
The People’s Daily, CPC leadership meets to discuss novel coronavirus prevention, control, 2020, http://en.people.cn/n3/2020/0125/c90000-9651799.htm Search PubMed.
-
M. Narajewski and F. Ziel, 2020, arXiv preprint arXiv:2004.14864.
- M. Bauwens, S. Compernolle, T. Stavrakou, J. F. Müller, J. van Gent, H. Eskes, P. F. Levelt, R. van der A, J. P. Veefkind, J. Vlietinck and H. Yu, Geophys. Res. Lett., 2020, e2020GL087978 CAS.
- T. V. Vu, Z. Shi, J. Cheng, Q. Zhang, K. He, S. Wang and R. M. Harrison, Atmos. Chem. Phys., 2019, 19, 11303–11314 CrossRef CAS.
- B. Silver, X. He, S. R. Arnold and D. V. Spracklen, Environ. Res. Lett., 2020, 15, 084021 CrossRef CAS.
- O. Tchepe and C. Borrego, J. Environ. Monit., 2010, 12, 544–550 RSC.
- Y. Xing, P. Brimblecombe and Z. Ning, Sci. Total Environ., 2019, 658, 1–7 CrossRef CAS.
- Z. Wu, M. Hu, P. Lin, S. Liu, B. Wehner and A. Wiedensohler, Atmos. Environ., 2008, 42, 7967–7980 CrossRef CAS.
- C. Barrington-Leigh, J. Baumgartner, F. Carter, E. Carter, B. E. Robinson, S. Tao and Y. Zhang, Nat. Energy, 2019, 4, 416–423 CrossRef.
- I. Staffell and S. Pfenninger, Energy, 2018, 145, 65–78 CrossRef.
- B. Jing, L. Wu, H. Mao, S. Gong, J. He, C. Zou, G. Song, X. Li and Z. Wu, Atmos. Chem. Phys., 2016, 16, 3161–3170 CrossRef CAS.
|
This journal is © The Royal Society of Chemistry 2021 |