DOI:
10.1039/D1EE01530C
(Review Article)
Energy Environ. Sci., 2021,
14, 4712-4739
Battery cost forecasting: a review of methods and results with an outlook to 2050†
Received
20th May 2021
, Accepted 29th June 2021
First published on 2nd August 2021
Abstract
Rechargeable batteries are a key enabler to achieve the long-term goal to transform into a climate-neutral society. Within this transformation, battery costs are considered a main hurdle for the market-breakthrough of battery-powered products. Encouraged by this, various studies have been published attempting to predict these, providing the reader with a large variance of forecasted cost that results from differences in methods and assumptions. This article creates transparency by identifying 53 studies that provide time- or technology-specific estimates for lithium-ion, solid-state, lithium–sulfur and lithium–air batteries among more than 2000 publications related to the topic. The relevant publications are clustered according to four applied forecasting methods: technological learning, literature-based projections, expert elicitations and bottom-up modeling. Method-specific assumptions are analyzed in-depth and discussed with regard to their results and empirical evidence. Further, 360 extracted data points are consolidated into a pack cost trajectory that reaches a level of about 70 $ (kW h)−1 in 2050, and 12 technology-specific forecast ranges that indicate cost potentials below 90 $ (kW h)−1 for advanced lithium-ion and 70 $ (kW h)−1 for lithium-metal based batteries. Recent studies show confidence in a more stable battery market growth and, across time-specific studies, authors expect continuously declining battery cost regardless of raw material price developments. However, large cost uncertainties are found to exist on technological and chronological levels that will remain a key challenge for researchers and industry in the future.
Broader context
In the global endeavor to combat climate change, more than 180 countries committed to the Paris Climate Agreement in order to transform into a climate-neutral society during the second half of this century. Adequate measures to achieve that goal include the extension of renewable energy usage and the decarbonization of transportation. A key enabler to implement these measures are rechargeable batteries that provide the possibility to decouple energy production and usage, and to replace fossil fuels, respectively. In addition to concerns regarding raw material and infrastructure availability, the levelized cost of stationary energy storage and total cost of ownership of electric vehicles are not yet fully competitive to conventional technologies, mainly due to high battery cost. However, battery costs have fallen fast during the last years and an accurate prediction of their future development is vital for profound research in academia and sustainable decisions in industry. This article outlines the most relevant literature on battery cost forecasting and provides transparency on methodological and technological details.
|
1. Introduction
The forecasting of battery cost is increasingly gaining interest in science and industry.1,2 Battery costs are considered a main hurdle for widespread electric vehicle (EV) adoption3,4 and for overcoming generation variability from renewable energy sources.5–7 Since both battery applications are supporting the combat against climate change, the increase of their market share is currently supported by the regulation of policy makers.8–11 Yet, during the last years, battery costs and especially those of lithium-ion batteries (LIBs), the state-of-the-art technology for EVs12,13 and potentially the most cost-effective technology for stationary energy storage,14,15 has rapidly declined driven by improvements in cell chemistry, process technology and the increase of production scale.1,3,16,17 Additionally, beside the optimization of LIBs, advanced lithium-based concepts such as solid-state batteries (SSBs), lithium–sulfur batteries (LSBs) and lithium–air batteries (LABs) are discussed intensely regarding further improvements in battery cost and parameters such as energy density.18–20 Considering the cost, these battery technologies promise further reductions,20–23 linked to decreased raw material cost (e.g., oxygen,24,25 sulfur26,27) or improved concepts of cell components (e.g., anode-free cells28,29). However, while LIBs are already produced on a large scale and industry players are investing billions of dollars in the construction of respective battery factories across the globe,30 SSBs, LSBs and LABs are currently produced at lab or pilot scale.19,31
Encouraged by the requirement for further reduced battery cost, various studies attempting to predict these have been published in the last decade. These studies aim to answer questions arising from a broad field of strategic subjects such as efficient subsidy designs,5,32 optimal R&D spending schemes,33,34 EV penetration forecasts,35,36 cost-effective technology choice14,37,38 and raw material market forecasts.39 However, for the year 2030, LIB pack cost forecasts range from below 100 to above 400 $ (kW h)−1,33,35 thus implying large cost uncertainties potentially resulting in inefficient policies,40 incorrect timing of mobility transitions,39 missed investment opportunities,32 and company bankruptcy filings.41
To allow for an in-depth understanding of the drivers behind this cost range, a review of these publications providing transparency with regard to applied forecasting methods and underlying assumptions is necessary. To date, such a review is not available within the scientific community. This study intends to close this gap and identifies 53 relevant publications with original battery cost or price forecasts from peer-reviewed literature by applying a framework to an initial number of more than 2000 studies related to battery cost. Subsequently, these publications are classified according to four superordinate forecasting methods (technological learning, literature-based projections, expert elicitations and bottom-up modeling). For each method, forecast results, relevant drivers and assumptions are identified, analyzed and discussed. From relevant studies, a total of 360 single data points is gathered. Based on this data set, a general LIB forecast trajectory throughout 2050 and technology-specific forecast ranges for LIBs by cathode technology, and LSBs and LABs are derived.
This review contributes to the research fields of battery technology and energy transition in multiple ways. It provides transparency by an in-depth analysis of the most relevant battery cost forecasts including application, applied method, underlying assumptions and forecasted values, Further, it provides a data base of extracted forecasts, discusses underlying assumptions and aggregates estimates into both, a forecast trajectory throughout 2050 and 12 technology-specific forecast ranges.
The remainder of this article is structured as follows: Section 2 describes the search strategy, outlines identified articles, describes the four forecasting methods and the method of analysis. Section 3 gives an overview of the relevant publications since 2010. Section 4 discusses and compares publications on comprehensive and method-specific levels and provides a future outlook. Section 5 summarizes the main findings and outlines contributions and limitations.
2. Search strategy, relevant studies and method of analysis
In order to identify relevant articles from peer-reviewed literature that provide original forecasts of battery cost, the Web of Science database has been searched and 2361 publications have been retrieved. The search strategy displayed in Table 1 has been used on article titles and abstracts published between January 2010 and February of 2021. Further, additional literature from the field has been identified based on a previously published review on battery cost models from 20202 and an application of the associated search strategy on the time interval between January 2020 and February 2021.
Table 1 Search strategy
Conceptualization |
Operationalization |
Keywords used |
“batter*” AND (“cost” OR “price”) AND (“forecast*” OR “predict*” OR “project*” OR “prospect*”) |
Field of search: document search |
Article title, abstract |
Focus: year |
2010–February 2021 |
Number of studies |
2361 |
By analyzing the abstracts of the search results, 53 articles that provide forecasts for lithium-based batteries have been identified and are listed in Table 2.
Table 2 Analyzed articles that provide original battery cost or price projections
|
Authors & year |
Publication title |
1 |
Baker et al. (2010) |
Battery technology for electric and hybrid vehicles: expert views about prospects for advancement |
2 |
Thiel et al. (2010) |
Cost and CO2 aspects of future vehicle options in Europe under new energy policy scenarios |
3 |
Gerssen-Gondelach and Faaij (2012) |
Performance of batteries for electric vehicles on short and longer term |
4 |
Weiss et al. (2012) |
On the electrification of road transport–learning rates and price forecasts for hybrid-electric and battery-electric vehicles |
5 |
Mayer et al. (2012) |
Feasibility study of 2020 target costs for PEM fuel cells and lithium-ion batteries: a two-factor experience curve approach |
6 |
Brodd and Helou (2012) |
Cost comparison of producing high-performance Li-ion batteries in the U.S. and in China |
7 |
Catenacci et al. (2013) |
Going electric: expert survey on the future of battery technologies for electric vehicles |
8 |
Gallagher et al. (2014) |
Quantifying the promise of lithium–air batteries for electric vehicles |
9 |
Patry et al. (2014) |
Cost modeling of lithium-ion battery cells for automotive applications |
10 |
Nelson et al. (2015) |
Cost savings for manufacturing lithium batteries in a flexible plant |
11 |
Matteson and Williams (2015, a) |
Learning dependent subsidies for lithium-ion electric vehicle batteries |
12 |
Eroglu et al. (2015) |
Critical link between materials chemistry and cell-level design for high energy density and low cost lithium–sulfur transportation battery |
13 |
Schünemann (2015) |
Modell zur Bewertung der Herstellkosten von Lithiumionenbatteriezellen |
14 |
Nykvist and Nilsson (2015) |
Rapidly falling costs of battery packs for electric vehicles |
15 |
Hagen et al. (2015) |
Lithium–sulfur cells: the gap between the state-of-the-art and the requirements for high energy battery cells |
16 |
Matteson and Williams (2015, b) |
Residual learning rates in lead–acid batteries: effects on emerging technologies |
17 |
Petri et al. (2015) |
Material cost model for innovative Li-ion battery cells in electric vehicle applications |
18 |
Sakti et al. (2015, a) |
A techno-economic analysis and optimization of Li-ion batteries for light-duty passenger vehicle electrification |
19 |
Berg et al. (2015) |
Rechargeable batteries: grasping for the limits of chemistry |
20 |
Wood et al. (2015) |
Prospects for reducing the processing cost of lithium ion batteries |
21 |
Ciez and Whitacre (2016, a) |
The cost of lithium is unlikely to upend the price of Li-ion storage systems |
22 |
Cole et al. (2016) |
Utility-scale lithium-ion storage cost projections for use in capacity expansion models |
23 |
Sakti et al. (2017, b) |
Consistency and robustness of forecasting for emerging technologies: the case of Li-ion batteries for electric vehicles |
24 |
Schmidt et al. (2017, a) |
The future cost of electrical energy storage based on experience rates |
25 |
Kittner et al. (2017) |
Energy storage deployment and innovation for the clean energy transition |
26 |
Berckmans et al. (2017) |
Cost projection of state-of-the-art lithium-ion batteries for electric vehicles up to 2030 |
27 |
Ciez and Whitacre (2017, b) |
Comparison between cylindrical and prismatic lithium-ion cell costs using a process based cost model |
28 |
Cano et al. (2018) |
Batteries and fuel cells for emerging electric vehicle markets |
29 |
Few et al. (2018) |
Prospective improvements in cost and cycle life of off-grid lithium-ion battery packs: an analysis informed by expert elicitations |
30 |
Vaalma et al. (2018) |
A cost and resource analysis of sodium-ion batteries |
31 |
Schmuch et al. (2018) |
Performance and cost of materials for lithium-based rechargeable automotive batteries |
32 |
Edelenbosch et al. (2018) |
Transport electrification: the effect of recent battery cost reduction on future emission scenarios |
33 |
Safoutin et al. (2018) |
Predicting the future manufacturing cost of batteries for plug-in vehicles for the U.S. Environmental Protection Agency (EPA) 2017–2025 light-duty greenhouse gas standards |
34 |
Nykvist et al. (2019) |
Assessing the progress toward lower priced long-range battery electric vehicles |
35 |
Schmidt et al. (2019, b) |
Projecting the future levelized cost of electricity storage technologies |
36 |
Philippot et al. (2019) |
Eco-efficiency of a lithium-ion battery for electric vehicles: influence of manufacturing country and commodity prices on GHG emissions and costs |
37 |
Wentker et al. (2019) |
A bottom-up approach to lithium-ion battery cost modeling with a focus on cathode active materials |
38 |
Hsieh et al. (2019) |
Learning only buys you so much: Practical limits on battery price reduction |
39 |
Schnell et al. (2019, a) |
Prospects of production technologies and manufacturing costs of oxide-based all-solid-state lithium batteries |
40 |
Comello and Reichelstein (2019) |
The emergence of cost-effective battery storage |
41 |
Zhou et al. (2019) |
Learning curve with input price for tracking technical change in the energy transition process |
42 |
Schneider et al. (2019) |
A modeling framework to assess specific energy, costs and environmental impacts of Li-ion and Na-ion batteries |
43 |
Schnell et al. (2020, b) |
Solid versus liquid—a bottom-up calculation model to analyze the manufacturing cost of future high-energy batteries |
44 |
Ciez and Steingart (2020) |
Asymptotic cost analysis of intercalation lithium-ion systems for multi-hour duration energy storage |
45 |
Duffner et al. (2020, a) |
Battery plant location considering the balance between knowledge and cost: a comparative study of the EU-28 countries |
46 |
Yan and Obrovac (2020) |
Quantifying the cost effectiveness of non-aqueous potassium-ion batteries |
47 |
Mongird et al. (2020) |
An evaluation of energy storage cost and performance characteristics |
48 |
Nemeth et al. (2020) |
Lithium titanate oxide battery cells for high-power automotive applications–electro-thermal properties, aging behavior and cost considerations |
49 |
Beuse et al. (2020) |
Projecting the competition between energy-storage technologies in the electricity sector |
50 |
Penisa et al. (2020) |
Projecting the price of lithium-ion NMC battery packs using a multifactor learning curve model |
51 |
He et al. (2020) |
Greenhouse gas consequences of the China dual credit policy |
52 |
Duffner et al. (2021, b) |
Large-scale automotive battery cell manufacturing: analyzing strategic and operational effects on manufacturing costs |
53 |
Mauler et al. (2021) |
Economies of scale in battery cell manufacturing: the impact of material and process innovations |
After the identification of relevant publications, these have been analyzed according to the following framework:
• The year of publication,
• the applied forecasting method, the technique used to derive battery cost forecasts,
• the battery application, the examined final product for which battery costs are estimated,
• the battery technology, the technical concept the investigated battery is based on,
• the forecast item, the object of the forecast being battery cost or price,
• the forecast level, the evaluated stage in the battery value chain from material to pack,
• the forecast horizon, the latest point in time for which values are reported,
• the forecasting period, the time interval between publication and forecast horizon,
• the method-specific set of assumptions, the underlying parameters or beliefs that drive forecasting values,
• the forecasted values, the time- or technology-specific values of the forecast item studies are reporting.
In addition, to further structure the analysis, publications have been classified by four superordinate forecasting methods that have been applied within these publications in order to derive cost or price estimates: technological learning, literature-based projection, expert elicitation and bottom-up modeling. These methods are briefly described in the following.
Technological learning, in literature also referred to as learning curve or experience curve analysis, assumes a fundamental relationship between technology cost and one or more learning parameters.42 This method has been introduced in the past century and since then, has been applied in strategic industries such as airplane43,44 or ship manufacturing.45 With regard to energy technologies beside batteries, major areas of research have been electricity generation46–48 and fuel production49–51 from renewable sources. In order to derive cost projections, first, the historical correlation between cost and the learning parameter is examined and learning rates are calculated. Second, this learning rate is combined with future expectations for the learning parameter and future cost estimates can be obtained.
In literature-based projections, forecasts are derived by the aggregation of previously published predictions. These meta-forecasts are based on the idea that forecast accuracy can be increased by combining individual projections.52,53 This method has been used to derive economic trends in general and to obtain cost and price forecasts in particular.54 In addition to batteries, it has been applied in cost forecasting of power-to-gas technologies55 and hydrogen fuel production.56 Consulted sources include academic publications as well as industry and analyst reports that are combined by an aggregation technique such as the determination of time-specific means or forecast ranges.
In expert elicitations, future-oriented cost estimates are derived by a structured interview process between authors and experts. This approach has been used widely specifically for cost forecasting of energy technologies.57,58 Other than batteries, the method has been applied to predict fuel cell,59 electrolyser,60 as well as wind61 and solar energy cost.62,63 Questions during the interview process can be asked on different technology levels such as product-, component- or process level and can reflect distinct external scenarios such as regulatory support or R&D funding levels.62,64
Bottom-up modeling describes an approach to translate technical parameters underlying the product and production process into technology cost.65 This method has been applied in various manufacturing industries.65–67 Regarding energy technologies other than batteries, it has been used to project costs for fuel cells and electrolysers,68,69 renewable energy technologies70–72 and integrated energy systems.73,74 In order to derive cost projections, the product is first separated into its individual components, required resources and processes are assigned, and cumulative cost are calculated. Regarding cost forecasting, estimates can be obtained by simulating parameter sets that reflect technological advances.75,76 These parameter sets can either be defined by the authors or by external sources such as academic literature, industry reports or experts.65
In order to compare assumptions and results of the studies in Section 4, the focus is set on battery cost on pack-level in $ (kW h−1), since the majority of studies agrees on this reporting format. Whenever values for multiple applications are reported, the forecast dedicated to electric vehicle batteries is preferred. Among the relevant studies, several studies predict the development of battery prices instead of cost. In general, prices do not equal costs since they reflect the willingness to pay of customers in contrast to costs that represent the value of all input factors required for the production of the battery combined.77,78 In the battery industry, prices are further influenced by strategic pricing, long-term contracts and rebates to utilize excess production capacity.79,80 Industry experts report that battery prices may be set below current cost levels in order to gain market share, stimulate overall battery demand and compensate for potential losses with expected profits in the future.75,77,81 In addition, several diversified corporations exist among market leaders that are assumed to subsidize their growing battery business with profits from other industries.81 In general, the increase of a company's market share is intended to strengthen its future cost position by allowing for economies of scale in manufacturing and purchasing, and by providing favorable access to raw materials and capital, and as a result, increasing future profits and raising the entry barrier for new competitors.82 However, details on company-specific prices, costs and profit margins are not publicly available and differences are difficult to assess.40,80 In battery literature both terms are frequently used interchangeable, a phenomenon reported earlier,39,77,80 which may be explained by different perspectives on the same value, since the price paid to a battery manufacturer represents the cost to the manufacturer of the final product. Therefore, in line with other studies,1,80 prices are treated the same way as cost in this study, but are signified differently (in all figures, solid polygons signify prices and hollow polygons costs). If values are reported on a different level than packs, surcharge rates are applied to allow for a comparative analysis. These rates are based on recent peer-reviewed publications and are +30.89% to adjust from cell to pack level,32 +33.51% from material to cell level,83 +16.14% from electrode stack to material level,83 and have been validated with recent estimates of industry experts.84–87 If forecasts are reported in a different currency, respective values in $ are calculated with regard to exchange rates in the year of publication provided in the data base of the Federal Reserve.88 In all studies reporting total battery costs, the respective value is divided by mentioned battery sizes. All values on the forecast level originally reported can be retrieved in the ESI.† Further, for publications reporting multiple time-specific values, as in the case of the elicitation of various experts, the arithmetic mean is calculated. Yet if central values or scenarios are reported, the central value for each year is taken into account. For publications reporting technology-specific forecasts, cost uncertainties originating from parameter variations such as battery design or price assumptions, are made transparent. Study-specific sources such as referenced tables or figures are provided in the ESI.† In order to set time-specific estimates into empirical context, we include industry price observations86,89 from 2010 to 2020. The respective source has been chosen since values are based on a market average, are frequently cited in official reports81,90 and are publicly available.
3. Review battery cost forecasting
A comprehensive overview of the analyzed publications sorted by their year of publication and classified by battery application, forecasting method, forecast horizon, battery technology‡ alongside noteworthy additional aspects relevant for battery cost is presented in Fig. 1. In the following, the publications associated to each of the four introduced forecasting methods are described in brief. Studies that use multiple or a combination of methods are classified according to their focused approach.
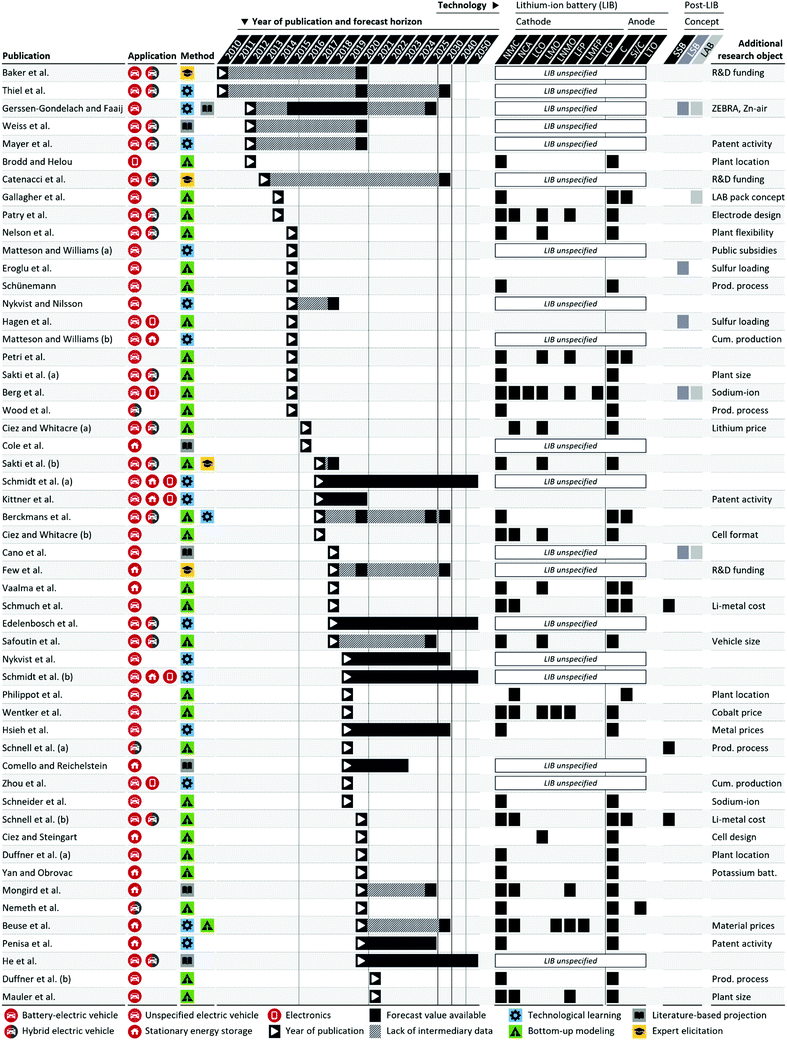 |
| Fig. 1 Overview of the analyzed publications on battery cost forecasting including year of publication, battery application, forecasting method, forecast horizon, battery technology and noteworthy additional aspects relevant for battery cost. | |
3.1. Technological learning
Thiel et al. (2010) compare the total cost of ownership of EVs and conventional vehicles for the years 2010, 2020 and 2030 by applying a method based on technological learning.36 The battery is identified as the major cost driver in both, plug-in hybrid and battery EVs and induces high cost penalties on these vehicles. The authors suggest that policy measures such as public R&D funding or temporary subsidies are required for a market breakthrough of these technologies in their initial phase. Consequently, increased sales volumes lead to an expected drop in LIB cost to 258 € (kW h)−1 until 2020 and to between 188 and 200 € (kW h)−1 in 2030, leading to competitive CO2 abatement cost. Gerssen-Gondelach and Faaij (2012) examine the prospects of five selected battery technologies including LIB, LSB and LAB and their impact on the total driving cost of purely EVs.38 Battery cost is determined to be one of the most relevant criteria among eight investigated battery properties. In their technological learning approach, forecasted pack-level LIB cost range from 990 $ (kW h)−1 in 2012 to 210 $ (kW h)−1 in 2020 based on different initial values and learning rate scenarios. For LSB and LAB, a literature review is conducted and forecasted values range from 250 to 500 $ (kW h)−1 for LSB and 300 to 700 $ (kW h)−1 for LAB, respectively. The authors conclude that even though other battery technologies promise advantages in cost and performance, only LIBs may fulfill all requirements in the medium term. Mayer et al. (2012) challenge the feasibility of industry cost targets for 2020 of high-energy and high-power LIBs and fuel cell stacks for vehicle traction.91 Cost reductions are based on technological learning assumptions of both, the growth of production volumes and patent activity. Based on initial high-energy LIB cost of 871 € (kW h)−1, the authors calculate a drop to 309 € (kW h)−1 until 2020 based on the most optimistic assumptions, still exceeding the target of 300 € (kW h)−1. Matteson and Williams (2015, a) quantify the required amount of public subsidies for EVs in the U.S. in order to reach LIB prices of 300 $ (kW h)−1.40 Based on different learning rate scenarios and the frequency of policy adjustments, the authors find cumulative required funds to be between 2 and 34 billion $ and underline their high sensitivity to learning rate variations. Nykvist and Nilsson (2015) review more than 80 estimates of LIB battery pack cost for EVs.† The authors find that LIB pack cost decreased by about 14% annually between 2007 and 2014, leading to a decline from above 1000 to 410 $ (kW h)−1. A steeper decline that has previously been reported, which is explained by the high cost in the early phase of EV sales growth, characterized by low production volumes, high pack variance and immature pack production processes that allowed for rapid learning. Further cost reductions from battery R&D improvements and economies of scale are expected by the authors and a cost level of 230 $ (kW h)−1 is projected based on technological learning for 2017–2018. Matteson and Williams (2015, b) evaluate LIB price competitiveness with lead–acid technology as a function of cumulative battery production.41 Technology-specific price trajectories are calculated by separating material and residual cost and applying a technological learning method. For large-format LIBs, 6500 GW h of cumulative production are forecasted to be necessary to reach price parity. By taking into account future cost improvements for both technologies, the authors conclude that LIB prices will not undercut those of lead–acid batteries for more than twenty years. Schmidt et al. (2017, a) forecast price developments for eleven electrical energy storage technologies including LIB for EVs, electronics and stationary energy storage until 2050.14 Technology-specific price reductions are projected based on experience rates. The authors find that, independent of technology, battery pack prices range from 150 to 200 $ (kW h)−1 once a total installed capacity of 1 TW h is reached. Based on market growth assumptions, this capacity threshold is estimated to be first surpassed by LIB battery packs for EVs in 2027, likely making LIB the most cost-competitive storage technology with expected battery pack prices between 36 to 96 $ (kW h)−1 in 2050. Kittner et al. (2017) combine learning-by-doing (increased production output) and learning-by-researching (increased patent activity) in their approach to predict LIB battery prices for EVs, electronics and stationary energy storage until 2020.32 For EV battery packs, prices are estimated to drop from the 2017 level of 178 to 124 $ (kW h)−1 in 2020. Further, the authors find that the allocation of public funds to R&D activities might play a larger role than deployment incentives since they allow for cost reductions in a shorter time frame. Edelenbosch et al. (2018) test the sensitivity of sales projections for hybrid and purely EVs in different battery cost and climate policy scenarios until 2050.92 While their article uses exogenous battery cost forecasts, the authors provide endogenous trajectories in the supplementary information, that are based on technological learning and include total battery pack cost that range from 3400 to 8650 $ (90 to 230 $ (kW h)−1 if divided by expected battery sizes) in 2050. In their article, the authors highlight the importance of the lower boundary of battery cost, since global EV sales shares exceeding 15% will require battery cost to fall below 100 $ (kW h)−1. Nykvist et al. (2019) evaluate the progress of EV attributes and assess their economic competitiveness compared to conventional cars throughout 2030.80 In order to model the impact of reduced LIB cost, the authors apply the learning methodology of Nykvist and Nilsson (2015) and extend the underlying data set by 25 recent battery cost estimates. Compared to the original data, accelerated cost reductions and a narrower variance of estimates are observed. By taking the updated data set into account, the authors expect EVs to achieve competitiveness at pack-level costs of 150 $ (kW h)−1 in 50% of U.S. car market segments by 2020. Further cost reductions to a level ranging from 43 and 119 $ (kW h)−1 are estimated until 2030. Schmidt et al. (2019, b) evaluate the impact of technology-specific price reductions on levelized cost of storage for twelve power system applications throughout 2050.37 The authors project reductions for LIB dedicated to EVs, electronics, residential and utility-scale stationary energy storage. For EVs, battery pack prices between 23 and 67 $ (kW h)−1 are projected for the year 2050. The authors state that, for most stationary applications, LIB is likely to become the most cost-efficient technology by 2030 due to reductions reinforced by knowledge spillovers from other markets such as EVs. Hsieh et al. (2019) project pack-level price estimates for NMC-based LIB until 2030 and set a particular focus on material cost.39 By applying a two-stage learning model that separates cost improvements of active material cost and residual LIB pack cost and considers mineral and material price floors, battery prices between 93 and 141 $ (kW h)−1 are estimated for 2030. The authors infer that, due to LIB cost reductions limited by material prices, EVs might still not be able to fully compete with conventional vehicles by then. As a consequence, they advise policy makers to focus on stimulating R&D for alternative battery chemistries and on stabilizing raw material prices. Zhou et al. (2019) compare the price performance of LIBs and lead–acid batteries based on cumulative battery production.93 For lead–acid batteries, the authors apply a decomposition method that separates technological learning into variations in material prices, material quantities and residual cost, while for LIB a single factor learning approach is used. LIB prices are estimated to fall to 100 $ (kW h)−1 once cumulative production reaches 2500 GW h. Beuse et al. (2020) investigate the economic competitiveness of six technologies for stationary energy storage throughout 2030.15 For LIBs, price trajectories are derived by applying a one-factor technological learning method that integrates material cost floors. In a first step, average LIB prices are projected for 2030 based on material price scenarios and average LIB material compositions, resulting in pack-level prices of 110 and 157 $ (kW h)−1 in 2030 for average and high raw material prices, respectively. In a second step, the authors use a bottom-up method in order to identify specific LIB technologies that can serve as a technological hedge against high material prices. The choice of LFP or LMFP cathodes (107 $ (kW h)−1) is shown to be most promising in mitigating high raw material prices in 2030 compared to LNMO, NCA, NMC622, NMC811, LMR-NMC and HE-NMC-based batteries.§ The authors conclude that LIBs are likely to outcompete other stationary energy storage in all considered applications by 2030 and warn of risks associated with a technological lock-in. Penisa et al. (2020) project prices of NMC-specific LIBs with a focus on stationary energy storage until 2025 by taking multiple approaches of technological learning.94 A two-factor model based on cumulative LIB demand and patent activity shows the most statistically sound results and yields LIB pack-level prices of 92 $ (kW h)−1 in 2025. From their four-factor model reflecting increased lithium and cobalt prices, the authors derive a nonetheless decreasing LIB price trend and conclude that the effect of learning and innovation outweighs raw material price effects.
3.2. Literature-based projection
Weiss et al. (2012) compare expected future prices of hybrid-electric and battery-EVs to conventional cars based on the development of technology-specific price differentials.95 While a methodology of technological learning is applied for the entire cost of electrification (battery, electric motor, inverter, controller, powertrain integration), average battery-specific cost of 320 € (kW h)−1 are discussed for 2020 and 100 € (kW h)−1 for 2030 based on analyst reports. Based on their results, a price breakeven between battery-electric and conventional vehicles is not to be expected before 2026. By analyzing literature and various industry sources, Cole et al. (2016) derive cost projections for utility-scale stationary LIB energy storage to forecast the split of U.S. energy generation capacity and the deployment of battery storage capacity until 2050.96 In a scenario-based approach, three trajectories for LIB battery pack cost are derived that range from 64 to 255 $ (kW h)−1 for the year 2050. They demonstrate that lower battery cost lead to an increase in the share of renewable energy generation and the deployment of battery energy storage, both resulting in a decrease of natural-gas-powered energy generation capacity. Cano et al. (2018) evaluate the suitability of seven energy storage and conversion technologies in different sectors of the transportation market.97 Among twelve criteria, cost is considered a primary concern for future vehicle owners and ranges for three lithium-based battery technologies are derived based on analyst, industry and literature sources. Identified pack cost range from 70 to 250 $ (kW h)−1 for LIBs, from 36 to 130 $ (kW h)−1 for LSBs and from 70 to 200 $ (kW h)−1 for LABs. The authors state that LIBs may not possess sufficient technological potential to meet performance requirements of all transportation sectors. Hence, LIBs may be replaced in vehicles by lower cost LSBs if their challenges such as poor cycle life are overcome. LABs are facing similar challenges in cycle life, but due to an inferior specific power may not be able to serve as a stand-alone battery for vehicle traction. Comello and Reichelstein (2019) forecast levelized cost of energy storage for LIB stationary energy storage systems under policy scenarios in Germany and California.5 The authors base their forecast on various stationary system price estimates from industry and academia. A continuously decreasing price level is observed and prices of 86 to 164 $ (kW h)−1 are expected by 2023. Based on this trend, decreased levelized costs of energy storage are expected that allow for profitable investments in LIB stationary storage systems in both examined locations. Mongird et al. (2020) compare the annualized cost of ten stationary energy storage technologies throughout 2025. Among other model input parameters, current capital cost estimates are derived from various analyst, industry and institutional sources. By applying a method based on arithmetic means and an annual capital cost improvement of 5%, the authors predict LIBs to have the lowest capital cost of 189 $ (kW h)−1 and the lowest annualized cost of all considered battery technologies in 2025.98 He et al. (2020) evaluate the consequences of different policy instruments on EV sales and cumulative greenhouse gas emissions in China.99 Their market penetration model relies on LIB cost predictions from multiple literature, analyst and institutional sources that range from 67 to 110 (kW h)−1 in 2050. While reduced battery costs result in higher market shares of EVs, increasing the efficiency of internal combustion engines is shown to result in significantly higher cumulative greenhouse gas emissions potentials under current policy.
3.3. Expert elicitation
Baker et al. (2010) asked experts to estimate the performance and cost for LIB technology after a 10 year period of different levels of U.S. government R&D spending.34 For annual R&D expenditures of $70 million, LIB cost of 232 $ (kW h)−1 are expected to be achievable. They conclude that present-day public R&D investments in battery technologies may avoid significant future CO2 tax burdens, encouraging to further focus on their development. Catenacci et al. (2013) asked experts from science and industry about the optimal allocation of public battery R&D investment in the EU and its expected impact on battery cost in 2030 for hybrid and purely EVs.33 Among seven battery technologies, experts chose to allocate the highest share of R&D funding to LIB due to its advanced maturity level. However, the effect of these investments varies widely across expert opinions and expected 2030 LIB battery cost range from 200 to 750 $ (kW h)−1. Few et al. (2018) conduct an expert elicitation to obtain estimations of cost and cycle life of LIB battery packs for stationary energy storage for the years 2020 and 2030.75 Regarding cost, the authors ask for their expectations based on different R&D funding scenarios and for major drivers of expected cost reductions. For 2020, experts’ pack cost estimates range from 50 to 657 $ (kW h)−1, major drivers being economies of scale, incremental improvements in cell chemistry and engineering potentials in battery management. For 2030, the estimates are between 20 and 511 $ (kW h)−1 and respective reductions are mainly driven by more fundamental improvements in cell chemistry. Even though aforementioned factors are expected to play a more significant role in cost reductions than R&D funding by the experts, the authors advise policy makers to reflect an identified lack of funding dedicated to bring technologies from lab to large-scale in their future support schemes.
3.4. Bottom-up modeling
Brodd and Helou (2012) compare bottom-up manufacturing cost LIBs using NMC|C-chemistry dedicated to electronics between plants located in the U.S. and China.100 Depending on plant location, production volume and degree of automation, calculated cost range from 1.58 to 2.18 $ per cell. Energy-specific cost or cell energy content are not reported. Across scenarios, plants located in China are shown to exhibit cost advantages mainly induced by lower labor cost. However, cost differences between countries are shown to narrow down to 0.07 $ per cell for highly automated plants with high production volumes. Gallagher et al. (2014) conduct a mass, volume and cost analysis for LABs dedicated to EVs.22 The authors base their cost calculation on the publicly available battery performance and cost model (BatPaC101) and derive best-case (e.g., neglecting potential required gold catalysts) LAB cost of 70 to 200 $ (kW h)−1 depending on pack concept and share of useable energy. Results are compared to those of LIBs using LMR-NMC|Si, LMR-NMC|C, and NMC111|C chemistries that range from 95 to 267 $ (kW h)−1. Since advanced LIBs such as LMR-NMC|Si may approach both energy density and cost of batteries using lithium metal anodes, the authors conclude that the former present lower risk pathways for automotive manufacturers by avoiding lithium-metal-specific challenges related to lithium deposition and solid electrolyte interphase formation. Patry et al. (2014) compare bottom-up cost of automotive LIBs using different cathode materials and electrode thicknesses to provide guidelines for future cost reductions.102 Calculated costs for NMC111|C, NCA|C, LMO|C and LFP|C-based cells with varying electrode thickness range from 233 and 402 $ (kW h)−1. The authors show that cathode materials characterized by lower capacities such as LFP and LMO lead to higher cell cost, despite lower cathode material prices. Further, they identify an increase of the electrode thickness as a key lever for cost reduction that needs to be carefully balanced with power and durability requirements. Nelson et al. (2015) investigate manufacturing cost for LIB packs dedicated to purely and hybrid EVs and set a particular focus on cost potentials in flexible plants.103 Four types of batteries using NMC|C and LMO|C chemistries are investigated and resulting pack cost range from 161 to 226 $ (kW h)−1. The authors show that, below specific production volume thresholds, the manufacturing cost of each battery type can benefit from a combined production in a flexible plant by an increased exploitation of economies of scale. They further outline that battery packs need to comply with joint restrictions in battery design for flexible manufacturing and that associated cost benefits decline with increasing production volumes. Eroglu et al. (2015) conduct a cost and performance analysis for LSBs dedicated to purely EVs.104 The authors investigate electrode and cell design considerations and their impact on system-level properties. Key parameters for LSB pack price are shown to be sulfur loading in the cathode, excess lithium metal at the anode, electrolyte volume fraction and sulfur to carbon ratio. Based on the variation of these parameters and additional material cost uncertainties, reported prices range from 80 to 270 $ (kW h)−1. The authors conclude that, in order to meet automotive cost and performance targets, LSBs should exhibit sulfur loadings above 8 mA h cm−2 while maintaining durability targets of 1000 cycles or 15 years. Schünemann (2015) investigates the effect of variations in design and process parameters on manufacturing cost of NMC|C cells dedicated to EVs.105 It is shown that an increased mass loading of the electrodes results in a more favorable ratio between active and inactive materials that decreases material cost and increases energy density. Despite opposing cost effects in the manufacturing process such as increased investments for longer dryers, cell costs are shown to decrease from 189 to 156 € (kW h)−1. Hagen et al. (2015) analyze the gap between the state-of-the-art and the requirements for high-energy LSBs.23 The authors calculate cost based on a cell format used in electronics and EVs and identify crucial design parameters to be sulfur loading in the cathode and sulfur utilization. Based on defined parameter intervals, resulting material cost range from 70 to 250 $ (kW h)−1. In order to exhibit cost and energy density similar to LIBs, the authors state that a sulfur loading of 6 mA h cm−2 is required and targets for sulfur utilization, sulfur fraction and electrolyte sulfur ratio are stated. Petri et al. (2015) forecast LIB cost for EVs based on different cathode and anode technologies.106 Material cost are calculated for LR-NMC|Si, LR-NMC|C, LMO|C, LFP|C and NMC|C-based chemistries that range from 93 to 111 € (kW h)−1. The authors underline that innovative cell materials with high specific energies could help reduce LIB material cost in the future. Sakti et al. (2015, a) analyze and optimize manufacturing cost of LIBs dedicated to purely and hybrid EVs.107 Based on material costs derived from a battery design optimization model, related processing cost in a cell and pack production plant are calculated bottom-up for NMC111|C packs. Three scenarios for parameters in the production process, material prices and battery design constraints are set up and resulting EV pack cost range from 200 to 370 $ (kW h)−1. Berg et al. (2015) compare cost and performance of different LIB, LSB, LAB and sodium-ion battery cells based on an energy-cost model.108 Regarding LIB cost, the authors compare LR-NMC|C, LNMO|C, NCA|C, NMC111|C, LCO|C, LFP|C and LCP|C cell chemistries and derive estimates that range from 191 to 295 $ (kW h)−1. LSB and LAB cell cost are calculated to be 154 $ (kW h)−1 and 105 $ (kW h)−1, respectively. The authors expect that LIBs using nickel- and lithium-rich chemistries will dominate electronic and automotive applications in the foreseeable future due to either comparable cost or fundamental challenges related to their alternatives. Wood et al. (2015) investigate potential reductions in the processing cost of LIBs.109 For the production of NMC|C packs, the cost impact of aqueous electrode processing, increasing electrode thickness and an optimized wetting and formation procedure are evaluated. By implementing these measures collectively, pack-level costs are shown to decrease from 503 to 352 $ (kW h)−1 by decreased electrode processing cost, energy consumption and formation time. Ciez and Whitacre (2016, a) investigate the sensitivity of LIB cost to variations in lithium raw material prices.110 The authors vary the lithium price from 0 to 25 $ kg−1 and quantify the impact on cell cost of four battery types classified by application requirements, being high-energy and high-power, and used cathode material, being NCA|C and LMO|C. Reported cell cost range from 162 to 435 $ (kW h)−1, mainly due to different requirements and cathode materials, variations from lithium price volatility remain below 10%. They conclude that the thread of lithium price increases will have limited impact on the battery market and future cost reductions. Sakti et al. (2017, b) test the consistency of expert forecasts of LIB pack-level cost estimates for hybrid and purely EVs with both, the sum of experts’ component-level estimates and a cost estimate derived by a process-based cost model fed with the experts’ expectations regarding underlying cell design, material and process.77 Expert- and method-specific pack-level estimates for purely EV batteries vary from 255 to 766 $ (kW h)−1 for the year 2018. In addition to the high range of estimates among experts, most experts’ immediate pack-level estimates are found to be inconsistent with their component-level forecasts and the bottom-up cost modeling results. Berckmans et al. (2017) develop market and LIB cost and price projections for hybrid and purely EVs. The authors calculate bottom-up cost estimates for NMC622|C and NMC622|SiC-based battery packs of 432 and 293 $ (kW h)−1, respectively.35 Based on current LIB market prices and the calculated cost differences between both battery types, the authors use a technological learning method for material and processing cost to project 2030 prices of 76 and 50 $ (kW h)−1, respectively. They conclude that reduced battery prices will be reflected in EV purchase prices and will drive their mass adoption. Ciez and Whitacre (2017, b) evaluate the impact of cell format on LIB cost by using a process-based cost model. The authors investigate cylindrical and prismatic formats, different cell dimensions, electrode thicknesses and plant production volumes.111 For LMO|C, NCA|C and NMC|C-based chemistries, reported cell cost range from 163 to 439 $ (kW h)−1. The authors conclude that even though chemistry itself plays a substantial role in cell cost,
the prismatic format offers cost potential for all chemistries. Vaalma et al. (2018) compare cost and resource availability of LIBs and sodium-ion batteries. For the first, pack-level cost based on BatPac calculations of NMC622|SiC, NMC622|C and LMO|C ranging from 234 to 259 $ (kW h)−1 are reported for stationary application.21 In all three evaluated scenarios, LIBs are shown to undercut sodium-ion battery cost levels at current raw material prices. Nevertheless, the authors underline potential raw material shortages due to insufficient lithium production expansion that may increase the attractiveness of sodium-ion batteries. Schmuch et al. (2018) evaluate performance and cost of LIBs and SSBs for EVs. For LIBs, material costs are calculated for LMR-NMC|SiC, NMC811|SiC, LMR-NMC|C, NCA|C and NMC622|C-based chemistries that range from 51 to 79 $ (kW h)−1 at electrode stack level.3 For SSBs, a concept of LMR-NMC cathode, sulfidic LPS solid electrolyte and lithium metal anode is evaluated and material costs of 59 to 127 $ (kW h)−1 at electrode stack level are reported. The wide range of SSB results from significant uncertainties related to the cost of lithium metal foil and the amount of lithium depleted during battery operation. Based on their analysis, the authors expect further cost improvements of LIBs by material advances, economies of scale and automation, helping them maintain a dominant position in the automotive market throughout 2030. In a project for the U.S. Environmental Protection Agency, Safoutin et al. (2018) project LIB pack cost, battery size, battery power and motor power capabilities for the year 2025.112 After calculating required properties of NMC/LMO|C and NMC-622|C batteries for several plug-in hybrid and purely EV types, the authors feed BatPac with relevant data to derive battery cost estimates. For the year 2025, bottom-up pack-level NMC622|C costs from 115 to 223 $ (kW h)−1 are derived depending on EV size. Philippot et al. (2019) analyze the influence of plant location on greenhouse gas emissions and cost of LIB manufacturing.113 In both aspects, the production of NCA|C packs with 2% anode silicon content between plants located Korea, China, Poland, Germany, Sweden, France and the U.S. are compared. Resulting pack-level cost for large-scale manufacturing range from 155 € (kW h)−1 in Poland to 180 € (kW h)−1 in Korea. Since higher variabilities are found for greenhouse gas emissions, the authors conclude that a country's electricity mix is a key parameter for the impact of battery manufacturing on climate change. Wentker et al. (2019) compare the cost of LIBs based on different cathode materials. In their bottom-up model, respective cell energy content and material cost for an automotive cell format are calculated.114 Resulting cost for LNMO|C, LMO|C, NCA|C, NMC111|C, NMC442|, NMC532|C, NMC622|C, NMC811|C, LR-NMC|C and LFP|C range from 65 to 88 $ (kW h)−1 on material level. The authors conclude that advanced chemistries such as nickel-rich materials promise both, potentials in energy density and cost. Schnell et al. (2019) outline prospects of production technologies and cost of oxide-based SSBs.20 Based on a cell format for hybrid EVs, the authors calculate bottom-up material cost for concepts using LNMO, NMC811 or HE-NMC cathodes, an oxidic LLZ solid electrolyte and a lithium metal anode. Production costs for cell manufacturing are based on a potential future process derived from already industrialized fuel cell and ceramic capacitor fabrication. Estimated large-scale cell cost range from 120 to 415 $ (kW h)−1, depending on cathode material and price for the solid electrolyte. The authors conclude that cost of oxide-based SSBs could become competitive to LIBs if LLZ prices fall below 60 $ kg−1 or lighter solid electrolytes are developed. Schneider et al. (2019) compare specific energy, cost and greenhouse gas emissions of LIBs and sodium-ion batteries. Current automotive LIB cell cost based on NMC111|C are estimated to be 186 $ (kW h)−1, significantly below the evaluated sodium-ion alternatives. According to the authors, this finding is mainly due to the lower specific charges and voltage of the active materials of sodium-ion batteries, leading to higher material requirements and longer processing times per kW h of capacity. They further show current LIB superiority regarding greenhouse gas emissions and attribute this fact to the same mechanism. Consequently, the authors state that sodium-ion batteries can only become competitive if a performance similar to LIBs is achieved. Schnell et al. (2020) compare the manufacturing cost of LIBs with sulfide and oxide-based SSBs based on an NMC811 cathode and a cell format dedicated to hybrid EVs.115 For LIBs using graphite and Si composite anodes, cell cost of 119 and 107 $ (kW h)−1 are calculated, respectively. For SSBs with lithium metal anode, cell costs range from 86 to 132 $ (kW h)−1 using a sulfide solid electrolyte (LPS), and from 123 to 267 $ (kW h)−1 using an oxide solid electrolyte (LLZ). The large variances in respective cost can be attributed to the high uncertainty in solid electrolyte prices in their study. In addition, the authors evaluate the cost of a sulfide-based SSB with graphite anode and derive cell cost of 159 $ (kW h)−1. The authors conclude that, in contrast to all other investigated SSBs, sulfide-based SSBs with lithium metal anode have the potential to become competitive to LIBs if LPS prices drop below 60 $ kg−1. Ciez and Steingart (2020) investigate performance and cost of different LIB cell designs and formats in grid storage applications.116 For LMO|C-based chemistries, the authors investigate bobbin cells, a format known from alkaline batteries and compare it to pouch cells with different electrode thicknesses. Cell-level costs of 105 to 180 $ (kW h)−1 are reported for pouch formats with an electrode thickness of 100 μm. Due to the higher electrode loading of bobbin cells, these are shown to be cost competitive with pouch cells up to an electrode thickness of 300 μm, but are unlikely to be suitable for EV applications due to limited rate capability. Duffner et al. (2020) analyze the suitability of the EU27 countries and the UK for LIB cell manufacturing.30 Country-specific indicators are gathered to compare both, potentials in the knowledge required for LIB production and location-based cost for energy, labor and buildings. Based on an NMC622|C chemistry for EVs, cell costs range from 92 to 98 $ (kW h)−1 from Bulgaria to Denmark, respectively. While leading countries can be found in both single categories, no country is found to be superior in both categories. The authors conclude that the location decision is company-specific and that experienced market leaders may favor lower cost countries and new entrants potentially prefer locations with sufficient LIB expertise. Yan and Obrovac (2020) quantify the cost-effectiveness of LIBs and potassium batteries for stationary energy storage.117 For LIBs based on NMC622|C chemistry, costs of 147 $ (kW h)−1 are reported on pack-level. Potassium batteries are shown to have overall cost drawbacks due to their inferior energy density, despite cost advantages in current collectors. The authors conclude that competitiveness to LIBs can only be achieved by significantly improving the currently low cycle life. Nemeth et al. (2020) evaluate performance and price of LIBs using graphite and LTO anodes in automotive applications.118 Price estimations for NMC111|C and NMC111|LTO are based on BatPac and are reported to range from 85 to 220 $ (kW h)−1 for mass-manufactured large high-energy cells. High-energy LIBs using LTO anodes are shown to result in higher prices than those using graphite anodes due to their lower energy density. The authors conclude that LTO-based LIBs are more suitable for high-power automotive applications due to their excellent rate capability. Duffner et al. (2021) evaluate cost of LIBs for EVs based on improvement potentials related to the categories production process, material and cell design, and location.76 Initial NMC622|C-based cell cost are calculated to be 106 $ (kW h)−1 and are forecasted to reach a level of 64 $ (kW h)−1 by changing the cell chemistry to NMC811|C and simulating eleven additional improvements from the three categories. By analyzing the effect between categories, process improvements are shown to result in the highest cost decrease. The authors conclude that future cost reduction efforts should focus on process improvements in mixing, coating, stacking, formation and aging. Mauler et al. (2021) analyze the effect of material and process innovations on the cost-efficient plant scale in LIB production for EVs.17 LIB cells using LFP|C, NCA|C, NMC811|C, and LR-NMC|C analyzed based on current and future scenarios of the production process and cell cost between 75 and 145 $ (kW h)−1 are calculated. The authors identify electrode manufacturing to be the bottleneck process for plant sizing and conclude
that the analyzed innovations lead to a more than fivefold increase in plant scales required for cost-efficient production.
4. Analysis of publications and identification of future needs
4.1. Analysis across forecasting methods
An overview of forecasted pack-level ranges in the 53 analyzed studies sorted by year of publication including an extract of the parameter set of upper and lower bounds, applied forecasting method, forecast item and the original forecast level before conversion to pack-level is presented in Fig. 2. Regarding the analyzed technologies, 50 studies investigate LIB (signified by solid bars), three studies SSB, five studies LSB and four studies LAB technology (dashed bars). In addition, four studies apply two different forecasting methods. For two of these, respective values could be separated, yielding the total of 64 distinct columns. The two remaining multi-method studies have been classified according to their methodological focus. One study did not provide energy-specific cost or respective battery energy content, but has been included as an empty column.100 Most of the resulting ranges are derived by bottom-up modeling (33 forecasts), followed by technological learning (16), literature-based projection (10) and expert elicitation (4). Battery cost is the most reported forecast item (40 forecasts) and the majority of originally derived ranges is on pack-level (34). Across the examined studies, reported values range from 20 to 1543 $ (kW h)−1. This extensive spread can be explained by a look at the parameter extractions across methods (positioned above the colored bars in each column of Fig. 2). Exceptionally high ranges can be observed for early time-specific studies with long forecasting periods using technological learning or literature-based projection, which can be dedicated to a higher initial level of battery cost reported at the beginning of the decade that are not included in the ranges of later studies. Further, the majority of studies that involve experts exhibit high ranges, which can be explained by a high variability and disagreement among experts reported by all respective authors. Even though most studies applying a bottom-up method do report values for a specific year, cost uncertainties are observable for different technological and market assumptions. A closer look at the parameter extracts reveals a variety of interacting dimensions that are investigated across methods and affect battery cost across methods. Apart from time, these include battery technology, battery design and format, production process and technology, plant location, plant size, material prices, vehicle size, cumulative production, R&D funding, and public subsidies. From the analysis between methods and parameter sets, we derive that studies using technological learning, literature-based projection and expert elicitation are, in most cases, applied to derive battery cost in the time dimension (i.e., for a specific year), whereas studies using bottom-up modeling show a focus on battery technology (i.e., technological concept such as cathode and anode technology). For this reason, in the following method-specific analysis, we focus on time for the first three methods and on battery technology for the latter. In addition, we include remarks regarding the effect of the further outlined criteria.
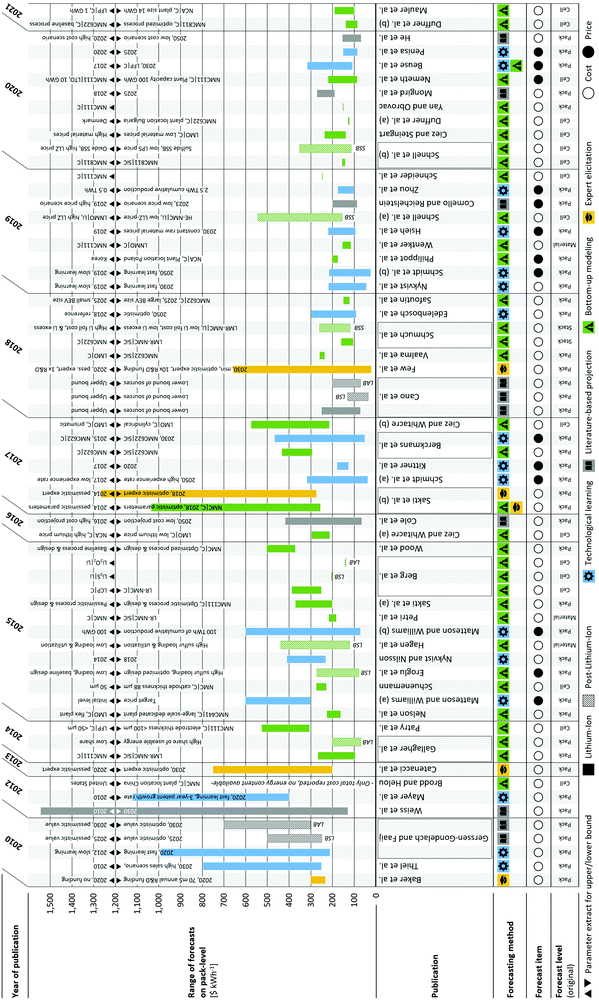 |
| Fig. 2 Overview of forecasted pack-level ranges in the analyzed studies including year of publication, parameter set for upper and lower bounds, forecasting method, forecast item, and originally reported forecast level. | |
4.2. Technological learning
The results of examined publications that derive time-specific battery cost forecasts based on technological learning are summarized in Fig. 3. We further include industry-average price observations86,89 on pack-level for comparison that are displayed as a histogram. When looking at the empirical price development from 2010 to 2020, high absolute reductions can be perceived especially in the first half of the decade from 1160 $ (kW h)−1 in 2010 to 384 $ (kW h)−1 in 2015. This reduction has been explained by high cost in the early phase of EV sales growth, characterized by low production volumes, high pack variance and immature pack production processes that allowed for fast learning.1 In the second half of the decade, more moderate absolute reductions are observable that reach a level of 137 $ (kW h)−1 in 2020. Similar to the development of industry data, all authors expect a decline in LIB cost. Study-specific average estimates range from 1120 $ (kW h)−1 for 2010 to 63 $ (kW h)−1 for 2030. In comparison to the industry average, the majority of forecasts until 2020 are above empirical price observations and high variances in forecast levels and developments can be identified across studies and time of publication. These variances can be attributed to differences in methodological variants and specific assumptions, both of which will be discussed in further detail.
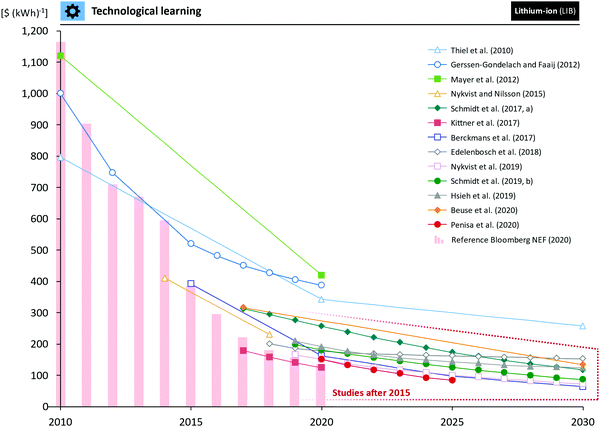 |
| Fig. 3 Forecasted values of studies applying technological learning methods to derive time-specific estimates. | |
The central methods used in the respective publications can be further specified in three different approaches. First, the 1-factor approach that relates forecast values to the future development of one learning factor. This is the most common approach for battery cost forecasts and used as the central method in nine studies.1,14,15,35–38,80,92 Second, the multi-factor approach, which is characterized by cost or price reductions that are derived based on the future development of multiple learning factors. Regarding forecasts in this study, a 2-factor approach is applied by three studies.32,91,94 Third, only applied by one study,39 a 2-stage approach, meaning that technological learning is conducted in two subsequent stages for different cost components with specific learning rates. While in some of these studies, more than one approach is used in order to validate assumptions, one central method is stated to explain cost developments most accurately. This study-specific approach is highlighted in bold in Table 3 where detailed assumptions of the respective publications are displayed.
Table 3 Publications applying technological learning and details regarding specific methods and assumptions
Publication |
Approach |
Forecast item |
Learning factor |
Learning rate |
Integration of material prices |
Description |
CAGR |
Value |
Time period for calculation |
Industry for calculation |
In bold: central method applied in the respective study. |
Thiel et al. (2010)
|
1-Factor
|
Cost
|
Cum. battery sales
|
39%
|
10%
|
≤2002
|
Battery-specific
|
No
|
Gerssen-Gondelach and Faaij (2012)
|
1-Factor
|
Cost
|
Cum. battery production
|
64%
|
9–17%
|
1993–2004
|
Battery-specific
|
Feasibility test
|
Mayer et al. (2012)
|
2-Factor
|
Price
|
Cum. battery sales, cum. patents
|
27%
|
8%
|
1991–2005
|
Battery-specific
|
No
|
30%
|
27%
|
|
1-Factor |
Price |
Cum. battery sales |
27% |
14% |
1991–2005 |
Battery-specific |
No |
Nykvist and Nilsson (2015)
|
1-Factor
|
Cost
|
Cum. battery sales
|
100%
|
9%
|
2006–2014
|
Battery-specific
|
No
|
Schmidt et al. (2017, a)
|
1-Factor
|
Price
|
Cum. installed capacity
|
35%
|
15.9%
|
2010–2016
|
Battery-specific
|
Feasibility test
|
Kittner et al. (2017)
|
2-Factor
|
Price
|
Ann. battery production, cum. PCT patents
|
13%
|
16.9%
|
1991–2015
|
Battery-specific
|
Feasibility test (See 4-factor)
|
10%
|
2% per 100 PCT patents
|
|
1-factor |
Price |
Ann. battery production |
13% |
17.3% |
1991–2015 |
Battery-specific |
(See 4-factor) |
|
1-Factor |
Price |
Cum. battery production |
16% |
15.5% |
1991–2015 |
Battery-specific |
(See 4-factor) |
|
1-Factor |
Price |
Cum. patents |
10% |
31.4% |
1991–2015 |
Battery-specific |
(See 4-factor) |
|
4-Factor |
Price |
Ann. battery production, cum. patents, lithium/cobalt price |
13% |
14.8% |
1991–2015 |
Battery-specific |
Lithium & cobalt price included |
10% |
n/a |
n/a, n/a |
n/a, n/a |
Berckmans et al. (2017)
|
1-Factor
|
Price
|
Ann. battery sales
|
30%
|
23.5%
|
≤1984
|
Chemical processing
|
Only in bottom-up
|
Edelenbosch et al. (2018)
|
1-Factor
|
Cost
|
Cum. battery sales
|
n/a
|
n/a
|
n/a
|
n/a
|
n/a
|
Nykvist et al. (2019)
|
1-Factor
|
Cost
|
Cum. battery sales
|
36%
|
16.8%
|
1991–2016
|
Battery-specific
|
No
|
Schmidt et al. (2019, b)
|
1-Factor
|
Price
|
Cum. installed capacity
|
28%
|
19.1%
|
2010–2017
|
Battery-specific
|
No
|
Hsieh et al. (2019)
|
2-Stage (1-factor)
|
Price
|
Cum. installed NMC battery capacity
|
43%
|
16.5% (pack)
|
2010–2016
|
Battery-specific
|
Floor for mineral & material cost
|
3.5% (NMC)
|
Beuse et al. (2020)
|
1-Factor
|
Price
|
Ann. installed capacity
|
20%
|
20.8%
|
2010–2017
|
Battery-specific
|
Material cost floor
|
Penisa et al. (2020)
|
2-Factor
|
Price
|
Cum. NMC battery sales, cum. PCT patents
|
11%
|
21.2%
|
2007–2019
|
Battery-specific
|
Feasibility test (See 4-factor)
|
10%
|
3% per 100 PCT patents
|
|
1-Factor |
Price |
Cum. NMC battery demand |
11% |
25.3% |
2007–2019 |
Battery-specific |
(See 4-factor) |
|
1-Factor |
Price |
Cum. PCT patents |
10% |
35.9% |
2007–2019 |
Battery-specific |
(See 4-factor) |
|
4-Factor |
Price |
Cum. NMC battery demand, cum. PCT patents, lithium/cobalt price |
Variables in 4-factor model stated to be statistically insignificant |
Lithium & cobalt price included |
Across all studies, at least one type of battery production, capacity or sales volume is defined as a learning factor, which has been applied in various studies on energy technologies46 and has been shown to be a particularly reliable metric in technological forecasting.119 The authors relate their time-specific forecasts to “experience”, namely cumulative battery production,38 cumulative battery sales1,36,80,91,92 or cumulative installed battery capacity,14,37,39 and “economies of scale” such as annual battery production,15,32,35 all of which will be referred to as “the battery market”, for the sake of simplicity. Regardless of the specific learning factor, each predicted value is significantly impacted by the determination of three parameters. First, the initial value of the time series that affects the level of subsequent estimates. Second, the learning rate, also referred to as experience rate if prices are concerned, that signifies the reduction rate in the forecast item for each doubling of the learning factor. Third, the expected growth rate of the learning factor that represents the speed of progress throughout the forecasting period and allows for a translation into a chronological scale. Regarding all three assumptions, remarkable differences are conceivable. The initial values can be extracted from the time-specific development in Fig. 3. Unsurprisingly, a decreasing trend is observable for initial values with an advancing year of publication that has been described earlier.1 For both other parameters, that largely determine the slope of the forecast series, an unambiguous development is not apparent and will be discussed in detail. Learning and experience rates alongside the underlying expectations for future battery market growth, calculated as compound annual growth rate (CAGR) of the learning factor in the forecasting period, are displayed in Fig. 4a and are provided in the ESI.† Market growth assumptions, mostly based on the respective metrics for EVs from various analyst, industry and agency reports combined with assumptions regarding vehicle battery size, range from 11 to 100% per annum. In spite of this vast range, differences in timing and length of the forecasting period as well as individual beliefs regarding future policy support can justify these variances and need to be taken into account in a comparison.46 The variability in this assumption is particularly large for earlier studies, whereas assumptions of market growth converge between 11 and 43% p.a. for studies after 2015, further narrowing to between 28 and 43% p.a. for those who forecast until 2030, indicating confidence in a more stable level of future battery market growth in academic literature. Regarding learning and experience rates, a differentiated analysis between 1-factor and 2-factor forecasts is essential since, based on the same data set, 2-factor approaches generally find lower factor-specific rates due to partial allocation of cost reductions to other factors.46 For 1-factor models, a convergence can be perceived for learning and experience rates that range from 9% to 24% across publications and stabilize at a higher level between 16% and 24% in studies after 2015. For 2-factor models that also integrate the growth in patent activity, although only supported by three studies, similar observations can be made for the learning rate related to the battery market.
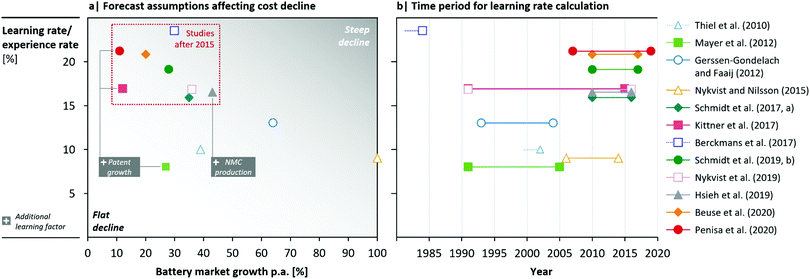 |
| Fig. 4 Assumptions for learning rates and battery market growth (a) and time period for the learning rate calculation (b). | |
A major determinant of the learning rate is the time period chosen for its calculation.120 Since learning rates are derived from the historical correlation between the learning factor (in most cases, the battery market) and the forecast item (battery cost or price), results may vary across years. The analysis of the relationship between learning rates and underlying time periods is a common research object for technologies in the renewable energy sector such as solar modules,121 wind turbines and farms,46,122 or hydro power plants.120 For batteries, study-specific time periods for the calculation of learning rates are displayed in Fig. 4b. When examining this relationship, an unambiguous development cannot be identified. However, when taking into account that Berckmans et al. derive their learning rate from a study that investigates chemical processing in general (see Table 3), an observation can be made for the remaining studies that obtain their learning rate based on a battery-specific market analysis. Among these studies, authors that integrate the years 2015 to 2019 into their calculation derive higher learning rates. The effect of both, stabilizing market assumptions and converging battery-specific learning rates, finds its expression in less volatile forecasts from studies after 2015, depicted in Fig. 3 as lines at the lower end between 2017 and 2030. In a comparative analysis between the methodological variants among this limited dataset, we find that even though the 2-factor approach yields the lowest time-specific results, it relies on lower initial values than the other examined variants. Regarding the 1-factor approach, above mentioned similarities lead to comparable slopes across studies, excluding Edelenbosch et al. that could not be analyzed in detail due to limited transparency of assumptions. In contrast, the forecasts of Hsieh et al., using a 2-stage approach, tend to level off beginning from 2025. This can be explained by two specific characteristics of their approach. First, the authors separate learning between active material synthesis and pack production. They derive, based on historical synthesis cost of NMC-111, slower learning for the active material (learning rate 3.5%) compared to pack production (16.5%). Second, they include increasing mineral cost floors to account for expected developments in cobalt, nickel and lithium prices. This potentially opposing trend to reduced battery cost reflects the growing awareness of risks concerning raw material prices and supply.123–125
4.3. Literature-based projections
The pack-level results of publications that derive time-specific forecasts based on literature sources are summarized in Fig. 5 and range from 1093 $ (kW h)−1 for 2010 to 104 $ (kW h)−1 for 2030. All authors expect decreasing LIB cost throughout their forecasting period, however, similar to the studies using technological learning, differences in methods exist that are further outlined in the following.
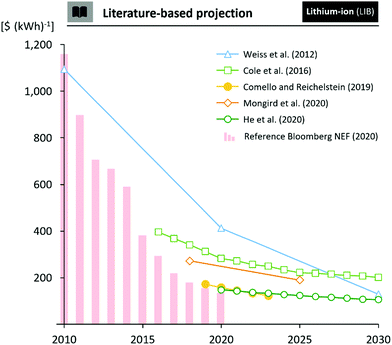 |
| Fig. 5 Forecasted values of studies applying literature-based projections. | |
The five studies providing literature-based projections have been analyzed regarding their forecast horizon, the applied aggregation technique and the type, number and year of publication of consulted literature sources. An overview of this analysis is presented in Table 4. All examined studies apply different aggregation techniques to the values extracted from literature. Weiss et al. combine the values from 8 sources into time-specific forecast ranges from a minimum to a maximum value until 2030. Cole et al. use the median value from 8 literature sources for the initial level, and calculate median annual reductions from the studies to derive subsequent levels of their cost trajectory throughout 2050. Comello and Reichelstein base their forecast until 2023 on the arithmetic mean of time-specific forecasts found in 8 literature sources. Mongird et al. exclude the three lowest battery cost values extracted from 20 sources and use the arithmetic mean of the remaining values as the starting point for their forecast. The authors assume a 5% annual decrease in battery cost to derive a future estimate for 2025. He et al. calculate the arithmetic mean of time-specific literature values for the years 2020, 2025, 2030, 2050 based on 9 sources, and apply linear interpolation to derive cost forecasts for intermediate years. Regarding the type of examined literature, all studies consult academic and analyst publications, while Mongird et al. additionally includes press articles and He et al. government targets, respectively. Three of the studies rely on direct sources no older than 4 years prior to publication, while Mongird et al. includes sources dating 6 years back. For Cole et al., details regarding the time of publication are not provided for all sources.
Table 4 Analysis of publications providing time-specific literature-based projections
Publication |
Weiss et al. |
Cole et al. |
Comello and Reichelstein |
Mongird et al. |
He et al. |
Year of publication |
2012 |
2016 |
2019 |
2020 |
2020 |
Forecast horizon |
2030 |
2050 |
2023 |
2025 |
2050 |
Aggregation technique |
Aggregation of literature values to time-specific forecast ranges |
Calculation of median from literature values as starting point, cost decline based on time-specific median reductions |
Calculation of arithmetic mean of time-specific literature values |
Removal of optimistic literature values, arithmetic mean of remaining values as starting point, constant annual cost decline |
Calculation of arithmetic mean of time-specific literature values for specific years, linear interpolation for intermediate years |
Source types |
Academic literature, analyst reports |
Academic literature, analyst reports |
Academic literature, analyst reports |
Academic literature, analyst reports, press articles |
Academic literature, analyst reports, government targets |
Number of direct sources |
8 |
8 |
8 |
20 |
9 |
Publication of earliest direct source |
2008 |
≤2015 |
2017 |
2014 |
2016 |
When comparing the results of the different studies with the industry observations until 2020 displayed in Fig. 5, Weiss et al. are in line with the empirical benchmark for 2010 but are furthest for 2020 compared to other studies. Similar to the observation in technological learning studies, this reflects a previous underestimation of the speed of battery cost reductions1,80 that is underlined by a decline in the initial values from the literature-based studies with advancing year of publication. For the studies after 2015, Cole et al. and Mongird et al. are most pessimistic compared to industry observations. While the median-based approach of Cole et al. is less sensitive to extreme values and hence reduces the influence of particular optimistic sources, Mongird et al. exclude the most optimistic estimates from their average-based approach. Both studies further rely on sources from 2015 or earlier. In contrast, the studies of Comello and Reichelstein and He et al. rely on an unadjusted average that equally reflects optimistic predictions, rely on more recent sources, and derive the lowest estimates in a time-specific comparison. However, it should be noted that the authors of the latter two studies also integrate estimates from the agency that provided the industry observations for our study, which contributes to their close proximity to the empirical benchmark in Fig. 5.
4.4. Expert elicitations
The average results of examined publications that derive time-specific LIB cost forecasts based on expert elicitations are summarized in Fig. 6 and reach from 644 $ (kW h)−1 for 2013 to 177 $ (kW h)−1 for 2030. In line with the aforementioned methods, experts expect decreasing battery cost and results vary significantly between studies.
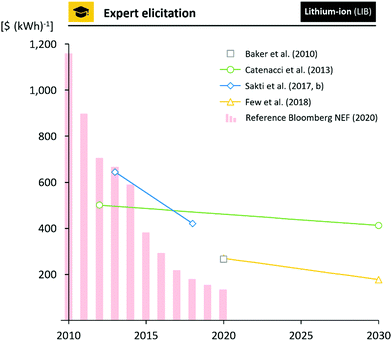 |
| Fig. 6 Forecasted values of studies based on expert elicitations. | |
Experts across studies express difficulties in predicting future battery cost due to the variance of material prices, unclear future production volumes, dynamic evolution of battery characteristics,33 doubts concerning battery safety34 and an overwhelming number of other factors influencing costs.75 All authors report limited consensus among experts and a high level of variability in their estimates,33,34,75,77 reflected in the large forecast intervals for 2030 in Catenacci et al. from 200 to 750 $ (kW h)−1 and in Few et al. from 20 to 511 $ (kW h)−1 that is mentioned in the respective studies. One reason for the variances between the four studies may result from differences in size and composition of the expert group. In the studies of Baker et al., Catenacci et al. and Few et al., 7, 14 and 11 experts are interviewed, respectively, the majority having an academic background. In contrast, Sakti et al. consult 12 experts exclusively from industry and consulting. Baker et al. and Catenacci et al. find an almost equal share of optimists and pessimists among experts but do not assign respective identities due to confidentiality reasons, whereas Few et al. reports experts from academia to be more optimistic than those from industry. We make a similar observation by comparing the results from the two most unequally distributed groups in this analysis. 5 of the 7 experts interviewed by Baker et al. in 2010 are from academia and the average estimate of battery cost among experts is 265 $ (kW h)−1 for 2020, an optimistic estimate at the time. In their publication of 2017 that only relies on industry specialists, Sakti et al. obtain approximately 50% higher average estimates for 2018, ranging among the pessimistic forecasts for the same year. In addition to disagreements between experts, three authors find a number of responses to be intrinsically inconsistent by specific consistency checks33,75,77 and Sakti et al. and Catenacci et al. report inconclusive answers to make up a significant share of 55% and 28%, respectively. To further analyze the studies based on expert elicitation, the different cost reduction drivers mentioned by experts have been gathered from each of the studies and allocated to the three categories material & product, process, and other cost reduction levers. While the both most recent studies ask specific questions on experts’ assumed cost reduction levers and provide more detailed information, the earlier studies, potentially due to a different focus, mention experts’ rationales only occasionally and detailed responses are not available. The expectations of the consulted experts regarding cost reduction drivers and their time-specific effectiveness are displayed in Table 5.
Table 5 Experts' expectations regarding cost reduction levers and their time-specific effectiveness
|
2018 |
2020 |
2030 |
Material & product |
♦ Change to NMC|C, LFP|C, NCA|C cell chemistry |
▲ Incremental chemistry changes |
▲ Cathode/anode changes |
♦ Improvements of cathode and anode specific capacities |
▲ Cobalt-free cathode materials |
▲ Binder improvements |
♦ Higher cell capacities |
■ Replacement of cobalt |
▲ Separator improvements |
♦ Evolutionary material or design improvements |
■ New materials |
▲ Solid-state electrolytes |
♦ Improved electrolytes |
▲ Better graphites |
▲ Electrolyte/additive improvements |
♦ Silicon anodes |
▲ Electrolyte/additive improvements |
▲ Water-based chemistry |
♦ Increased electrode thickness |
▲ More silicon in anodes |
● Technological improvements |
♦ Liquid pack cooling |
▲ Cell format changes |
♦ Cost reduction battery management system |
▲ Packaging improvements |
▲ BMS improvements |
▲ Engineering improvements |
▲ Integrated design/standardization |
Process |
♦ Electrode manufacturing improvements |
▲ New manufacturing techniques |
● Improvement of production yield |
♦ Improvement of production yield |
▲ Increased automation |
Other |
|
▲ Economies of scale |
● Learning-by-doing |
■ Increased R&D funding |
● Increased R&D funding |
Source |
♦ Sakti et al. (2017) |
■ Baker et al. (2010) |
● Catenacci et al. (2013) |
▲ Few et al. (2018) |
▲ Few et al. (2018) |
A closer observation of the categorized cost reduction levers reveals interesting findings. Most strikingly, the consulted experts mention most frequently levers that are linked to the material & product category. For 2018 and 2020, experts expect rather incremental improvements in cathode and anode materials, cell design and pack technology that lead to an increased energy density and/or lower cost. For 2030, more fundamental improvements such as solid electrolytes or water-based chemistries are mentioned. Regarding production process and other improvements, experts expect improvements by new manufacturing techniques, increased production yield, economies of scale and an increase in R&D funding. However, in comparison, process-related answers remain on a rather general level. We find Few et al. to describe a lack of process knowledge across the consulted experts regarding energy consumption and Sakti et al. to report more than half of the experts feeling uncomfortable in answering process-related questions. These findings indicate an underrepresentation of process specialists in the analyzed studies that may lead to an underestimation of future battery cost potentials in expert elicitation. Despite the mentioned limitations, we consider expert elicitation an important tool in predicting battery cost due to its unique ability to provide insights into experts’ beliefs and doubts regarding fundamentally new technologies characterized by a lack of historical data and experience.
4.5. Bottom-up modeling
For the analysis of studies that apply bottom-up modeling, we focus on battery technology as outlined at the beginning of this section. In order to compare the results of the different studies, we classify lithium-based batteries according to common practice in battery research19,31,115 in LIB characterized by intercalation electrodes and a liquid electrolyte, SSB characterized by a solid electrolyte, and LSB and LAB using sulfur and atmospheric air or oxygen on the cathode side, respectively. In addition, SSBs, LSBs and LABs typically use lithium metal on the anode side. To compare the factors that drive forecasted values, we focus on determinants of energy density and material price assumptions, but if required to explain differences, outline additional assumptions. A battery's energy density is considered a major driver of both, its material and processing cost.3,19,126 This is due to the fact that by increasing energy density, an improved ratio between active and inactive materials can be achieved, resulting in lower energy-specific cost for inactive materials. Regarding manufacturing, more output in terms of energy (i.e., kilowatt hours) can be produced without additional production equipment, production footprint and respective investments, having a decreasing effect on processing cost. Apart from energy density that indicates the amount of material required per kilowatt hour, material prices drive material costs that account for 60 to 80% of the battery pack.101,107 For LIB technologies, we focus on the one hand on active material specific capacity and discharge potential, since these largely determine cell and pack energy density,127,128 and active material price on the other (please note that price and cost are not always explicitly differentiated here, with price we refer to the value that is used as the base for cathode cost calculation). The vast majority of LIB estimates varies by cathode technology and base their results on a common graphite anode. Hence, the focus is set on cathode technologies and respective properties and price assumptions are compared. For the average discharge potentials of cathode materials, we rely on literature values,3,108,128 since the examined studies seldomly state these explicitly. In the following, we briefly describe the examined cathode technology, the average of identified forecasts among studies that provide a related estimate and the average of available assumptions. For the calculation of the average forecast, we choose the baseline if only one estimate is provided and the optimistic estimate if a range is projected. This calculated average hence includes future-oriented aspects such as expected improvements in cell design, production process and economies of scale. Due to the large number of 75 displayed estimates, we focus on a comparison between the assumptions underlying the extreme values in each category. Further details of all studies are included in the ESI.† We order the following descriptions by average cathode specific capacities, but group NMC materials together. In each material category, studies are sorted according to their time of publication. An overview of study-specific results together with specified anode technology if different from graphite and assumed cathode active material properties and price is presented in Fig. 7.
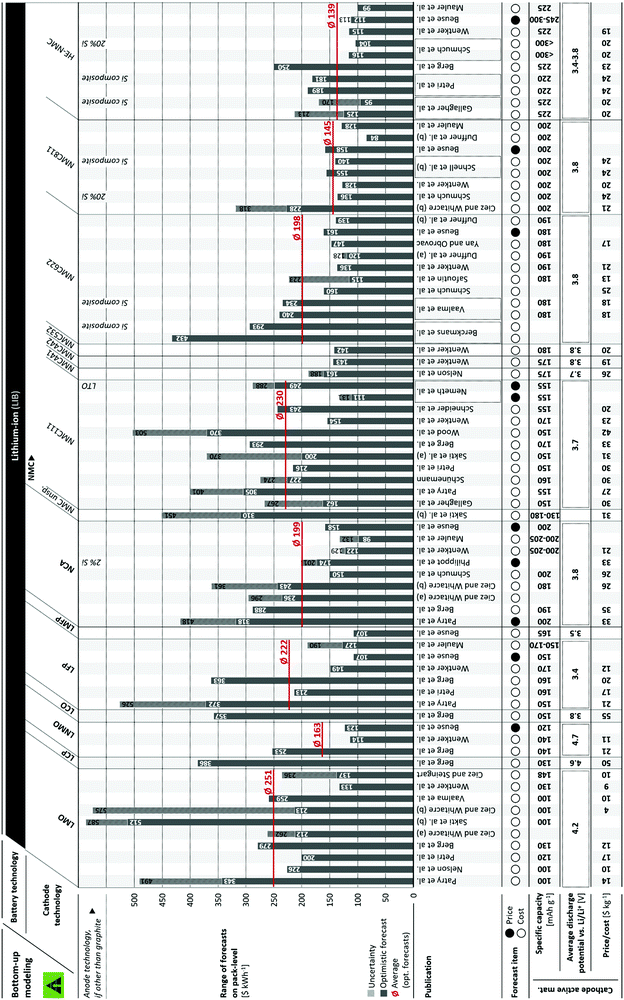 |
| Fig. 7 Overview of study-specific results, grouped by cathode technology, specified anode technology if different from graphite, assumed cathode active material properties and price. | |
The cubic spinel oxide LMO (LiMn2O4) is a cathode active material that provides excellent rate capability but limited energy density and cycle life.128,129 Based on ten estimates, we calculate an average of 251 $ (kW h)−1 for LMO-based battery packs. It exhibits an average discharge potential of ∼4.2 V and related studies assume a specific capacity of 114 mA h g−1 and a material price of 10.7 $ kg−1 on average. The minimum value of 133 $ (kW h)−1 obtained from the study of Wentker et al. is based on a specific capacity of 130 mA h g−1 and a material price of 9 $ kg−1. The study of Sakti et al. (a) that includes the maximum of displayed values of 587 $ (kW h)−1 for LMO-based battery packs, mentions a specific capacity of 100 mA h g−1, being the minimum value among the examined studies, while no details are available regarding cathode material prices. The cobalt-containing olivine LCP (LiCoPO4) cathode active material offers a high working potential for high-energy cells but faces challenges regarding self-discharge and cycle life.130,131 It exhibits an average discharge potential of ∼4.6 V and an estimate of 386 $ (kW h)−1 is obtained from the study of Berg et al. based on a specific capacity of 130 mA h g−1 and a material price of 50 $ kg−1. The spinel oxide LNMO (LiNi0.5Mn1.5O4) material is considered a potential environmentally benign and low cost cathode active material3,132 and exhibits the highest average discharge potential of ∼4.7 V among the examined materials, which exceeds the stability window of common electrolytes.108 Based on three estimates, we calculate an average of 163 $ (kW h)−1 and average assumptions for specific capacity of 133 mA h g−1 and material price of 16.0 $ kg−1. While both studies assume a similar specific capacity of 140 mA h g−1, the minimum value obtained from Wentker et al. of 114 $ (kW h)−1 is based on a material price of 11 $ kg−1, the maximum value obtained from the study of Berg et al. is based on a price of 21 $ kg−1. The layered-oxide LCO (LiCoO2) cathode active material offers high cycle life and low self-discharge but its application is mainly limited by the high, cobalt-induced material cost.108,133 It exhibits an average discharge potential of ∼3.8 V and an estimate of 357 $ (kW h)−1 is obtained from the study of Berg et al. based on a specific capacity of 150 mA h g−1 and a material price of 55 $ kg−1. The olivine LFP (LiFePO4) cathode active material has advantages regarding safety, cycle life and sustainability,17,134 but exhibits a limited average discharge potential of ∼3.4 V. Based on six estimates, we calculate an average of 222 $ (kW h)−1 and average assumptions for specific capacity of 158 mA h g−1 and material price of 17.5 $ kg−1. The minimum estimate of 107 $ (kW h)−1 taken from Beuse et al. assumes a specific capacity of 150 mA h g−1 and does not provide LFP material prices. The maximum estimate of 526 $ (kW h)−1 obtained from Patry et al. assumes a similar capacity, and the highest available material price of 21 $ kg−1. The lower estimate of Beuse et al. can be dedicated to their applied methodology that adds to bottom-up calculated material cost a technological learning approach for residual pack components. The olivine LMFP (LiMn0.8Fe0.2PO4) cathode active material offers high rate capability, favorable safety and low material cost135,136 and, compared to LFP, a higher average discharge potential of ∼3.5 V. Beuse et al. calculate a price of 107 $ (kW h)−1 based on a specific capacity of 165 mA h g−1. The nickel-rich layered-oxide NCA (LiNi0.8Co0.15O2) cathode active material offers high energy density but, compared to layered-oxide materials with lower nickel content, exhibits lower cycle life and a lower thermal stability.3 Based on nine estimates, we calculate an average of 199 $ (kW h)−1 for NCA-based battery packs. It exhibits an average discharge potential of ∼3.8 V and related studies assume a specific capacity of 196 mA h g−1 and a material price of 29.0 $ kg−1 on average. The minimum value of 98 $ (kW h)−1 obtained from the study of Mauler et al. is based on a specific capacity of 205 mA h g−1 and the authors assume a cathode thickness of 100 μm, an improved production process in an optimally scaled manufacturing plant. The maximum value of 418 $ (kW h)−1 obtained from the study of Patry et al. assumes a specific capacity of 200 mA h g−1 and a material price of 33 $ kg−1. Further, the respective estimate is based on a maximum electrode thickness of 50 μm, the lowest among compared estimates. The layered-oxide NMC family includes several cathode active materials based on the stoichiometry LiNixMnyCozO2 (x + y + z = 1) that are signified by a 3-digit affix that represents the share of respective metals in the formula. While respective materials exhibit average discharge potentials of ∼3.7 to ∼3.8 V, other properties vary depending on their composition. Materials based on a higher nickel content exhibit higher specific capacities, and hence offer increased energy density, but face challenges regarding thermal stability and cycle life.128,137 Further, this family includes high-energy HE-NMC materials that are characterized by an increased lithium and/or manganese content and exceed specific capacities of aforementioned NMC materials, but exhibit slightly lower discharge potentials.3,133 Regarding NMC111-based batteries, we calculate an average value of 230 $ (kW h)−1 based on eleven estimates. Related studies assume a specific capacity of 156 mA h g−1 and a material price of 29.6 $ kg−1 on average. The minimum value of 111 $ (kW h)−1 obtained from the study of Nemeth et al. is based on a specific capacity of 155 mA h g−1 and an annual plant production capacity of 100 GW h, material prices are not provided. The maximum value of 418 $ (kW h)−1 taken from Wood et al. assumes a specific capacity of 150 mA h g−1, the highest material price of 42 $ kg−1 and a plant capacity <0.1 GW h. Regarding NMC622-based batteries, we calculate an average value of 198 $ (kW h)−1 based on eleven estimates. Related studies assume a specific capacity of 184 mA h g−1 and a material price of 18.6 $ kg−1 on average. The minimum value of 115 $ (kW h)−1 taken from Safoutin et al. is based on a specific capacity of 180 mA h g−1 and the lowest material price of 13 $ kg−1. For the maximum value of 432 $ (kW h)−1 taken from Berckmans et al., the authors state it is based on a low production volume, and assumptions of cathode specific capacity and material price are unavailable. Regarding NMC811-based batteries, we calculate an average value of 145 $ (kW h)−1 based on eight estimates. Related studies all assume a specific capacity of 200 mA h g−1 and a material price of 22.7 $ kg−1 on average. While for the minimum value of 84 $ (kW h)−1 obtained from Duffner et al. material prices are unavailable, the authors assume an electrode thickness of 100 μm, a 35 GW h plant, an optimized process, a 99% cell yield rate, a labor rate of 10 $ h−1 and building cost of 1292 $ m−2. In comparison, for the maximum value of 318 $ (kW h)−1 obtained from Ciez et al. (b), the authors assume an electrode thickness of 70 μm, a 2 GW h plant, a baseline process, a 95% yield rate, a labor rate of 18 $ h−1, and building cost of 3000 $ m−2. Regarding HE-NMC-based batteries, we calculate an average value of 139 $ (kW h)−1 based on ten estimates. Related studies assume a specific capacity of 226 mA h g−1 and a material price of 21.4 $ kg−1 on average. The minimum value of 95 $ (kW h)−1 taken from Gallagher et al. is based on a specific capacity of 225 mA h g−1, a material price of 20 $ kg−1 and a maximum electrode thickness of 200 μm. The maximum value of 250 $ (kW h)−1 obtained from the study of Berg et al. assumes a specific capacity of 225 mA h g−1, a material price of
23 $ kg−1 and a maximum electrode thickness of 100 μm.
Overall bottom-up forecasted values for LIB range from 84 to 587 $ (kW h)−1 and both, between material categories and between studies in each category, high variances can be observed. The calculated average of forecasts remains above 200 $ (kW h)−1 for LIBs using either low specific capacity or low voltage cathode active materials and is around this threshold for NCA and NMC622-based batteries. Significantly lower average values are forecasted for LIBs based on high-voltage LNMO or high-capacity NMC811 and HE-NMC. Regarding active material price assumptions, calculated averages for materials not containing cobalt such as LMO, LNMO and LFP are lowest, while the high-cobalt materials LCP and LCO exhibit the highest prices. Average prices of NMC and NCA materials range between both aforementioned groups. In addition to the elemental composition, active material prices are influenced by market dynamics, chosen process route, precursor prices, and atmospheric requirements for their production.138 This can partly explain differences in average prices for NMC and NCA materials. Also having a higher cobalt share, NMC111 exhibits the highest average price among these materials. NCA, NMC622, NMC811 and HE-NMC on the one hand benefit from lower cobalt and/or higher manganese contents, having a decreasing effect on material prices. On the other hand, higher nickel contents require increased processing efforts139–142 and producers currently charge higher profit margins on nickel-rich materials.138 Even though variances between estimates cannot be explained by different price assumptions alone, we find model inputs to significantly vary for LMO between 4 and 17 $ kg−1, for LNMO between 11 and 21 $ kg−1, for LFP between 12 and 21 $ kg−1, for NCA between 21 and 35 $ kg−1, for NMC111 between 20 and 42 $ kg−1, for NMC622 between 13 and 25 $ kg−1, and for graphite between 10 and 22 $ kg−1. In addition to material and cell-related potentials, recent publication indicate further cost reductions on pack-level, achievable by an improved packing efficiency through cell-to-pack technology,143,144 that have to date not been investigated in detail.
For SSB, LSB and LAB, we focus on the comparison of assumptions regarding specific energy and prices of crucial materials. As opposed to an organic liquid in LIBs, SSBs are characterized by a solid electrolyte and can be further classified by the material type into polymer, oxide, and sulfide solid electrolytes.31 Polymer SSBs are characterized by high safety and favorable mechanical properties for the contact between electrodes and solid electrolyte, but suffer from low ion conductivity at room temperature and poor rate performance.18,145 Oxide SSBs offer advantages in electrochemical and temperature stability, but are limited in their ion conductivity and exhibit rigid mechanical properties with an increased risk of mechanical failures.31,146 Sulfide SSBs promise higher conductivities, the potential for fast charging, and good contact between electrode and electrolyte, but face challenges due to a narrow electrochemical window and moisture sensitivity.145–147 We identified three studies that analyze oxide and/or sulfide-based SSBs, find high uncertainties in solid electrolyte and anode active material prices, and hence include these in addition to cathode material price assumptions into our analysis. Similar to SSBs, LSBs and LABs have so far not been commercialized at a large scale.19 Both exhibit high theoretical specific energy and use the earth-abundant, low-cost elements sulfur and oxygen on the cathode side, respectively.148,149 We identified three studies that provide forecasts for LSB and two studies for LAB and analyze specific energy and active material prices. An overview of study-specific results for SSB, LSB and LAB, alongside technological and material price assumptions is presented in Fig. 8.
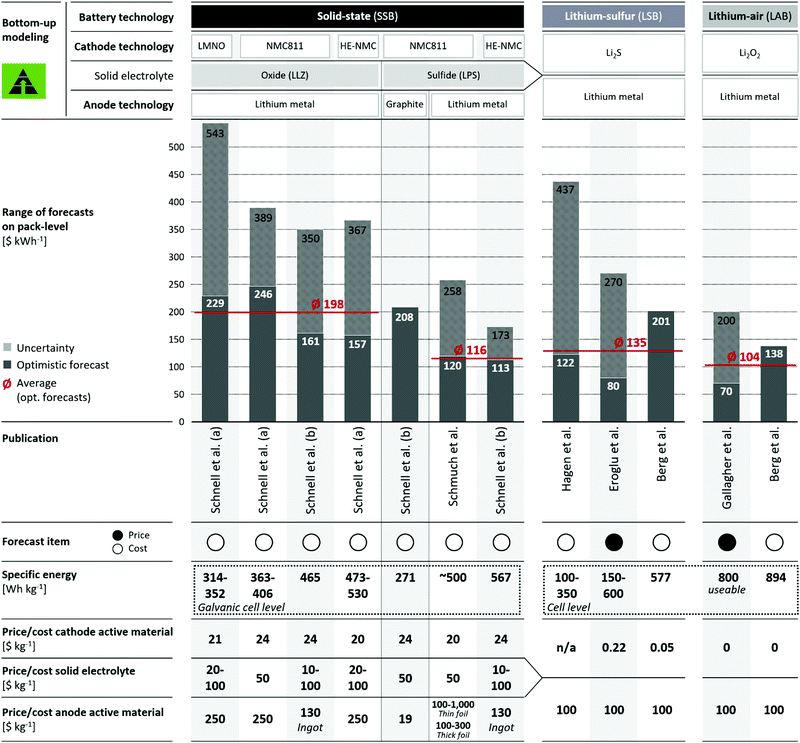 |
| Fig. 8 Overview of study-specific results for SSB, LSB and LAB, alongside technological and material price assumptions. | |
The cost estimates of SSBs using lithium metal anodes have been sorted by the specific energy on galvanic cell level (i.e., excluding housing). The range of specific energies in the study of Schnell et al. (a) results from the assumed cathode thickness variation from 70 to 150 μm. A trend towards higher specific energies can be observed for SSBs using lithium metal anodes, the sulfide LPS electrolyte and higher capacity cathode active materials. This trend is reflected in decreasing cost estimates from left to right. One notable exception is the estimate of Schnell et al. (b) for a sulfide SSB with graphite anode which can be dedicated to the lower specific energy compared to other sulfide SSBs. The authors find that this SSB does not provide a specific energy advantage or cost benefit over a similar LIB. For the SSBs with lithium metal anodes, significant cost variations are reported that, beside the range of cathode thicknesses from Schnell et al. (a) result from high uncertainties in the price of solid electrolytes ranging from 10 to 100 $ kg−1 for both, LLZ (lithium lanthanum zirconate, not further specified) and LPS (lithium thiophosphate, not further specified), lithium metal foil ranging from 100 to 1000 $ kg−1, and the required lithium excess ranging from 50 to 300%. For oxide SSB, based on four estimates, we calculate an average of 198 $ (kW h)−1. The minimum and maximum value of 157 and 543 $ (kW h)−1 obtained from the same study of Schnell et al. (a) is based on a HE-NMC cathode active material priced at 20 $ kg−1 with a thickness of 150 μm and an LLZ price of 10 $ kg−1 on the one hand, and LNMO at 21 $ kg−1 with a thickness of 70 μm and an LLZ price of 100 $ kg−1 on the other. For sulfide SSB with lithium metal anode, based on two estimates, we calculate an average of 116 $ (kW h)−1. The minimum value of 113 $ (kW h)−1 obtained from the study of Schnell et al. (b) is based on a HE-NMC cathode active material priced at 24 $ kg−1 with a thickness of 100 μm, an LPS price of 10 $ kg−1, a price of 130 $ kg−1 for lithium ingots (i.e., base material for lithium foil), and lithium excess of 50%. The maximum value of 258 $ (kW h)−1 obtained from the study of Schmuch et al. is based on a NMC811 cathode active material priced at 20 $ kg−1 with a thickness of 65 μm, an LPS price of 50 $ kg−1, a price of 300 $ kg−1 for thick lithium foil, and lithium excess of 300%. The forecasts for LSBs have been sorted by the minimum cell specific energy assumed in the respective studies. For the forecasts of Hagen et al. and Eroglu et al., variances in specific energy can be dedicated to the variation of design parameters such as sulfur loading and cathode thickness, that in turn lead to significant forecast uncertainties. Since the available assumptions of material prices are similar, aforementioned assumptions can explain the decreasing trend from the pessimistic cost of 437 $ (kW h)−1 obtained from Hagen et al. based on a sulfur loading of 1 mg cm−2 and a cathode thickness of 70 μm, over the pessimistic value of 270 $ (kW h)−1 from Eroglu et al. based on a sulfur loading of ∼3 mg cm−2 and a cathode thickness of 70 μm, to the cost estimate of 201 $ (kW h)−1 obtained from Berg et al. based on a sulfur loading of 8.5 mg cm−2 and a cathode thickness of 100 μm. Regarding their optimistic estimates, Hagen et al. and Eroglu et al. both increase cathode thickness to 150 μm, but differ in the maximum sulfur loading of 6 and ∼12 mg cm−2, respectively. Consequently, Eroglu et al. calculate the minimum forecast of 80 $ (kW h)−1. Based on three results, we calculate an average of 135 $ (kW h)−1. The forecasts for LABs have been sorted by the cell specific energy stated in the respective studies. Gallagher et al. only provide cell useable specific energy of 800 W h kg−1 based on a useable state-of-charge window of 85%. However, the uncertainty of their forecasts from 70 to 200 $ (kW h)−1 results from the variation in this assumption, the exact range of respective state-of-charge windows is not specified, rendering a comparison difficult. Both studies further agree on the same price assumptions of cathode and anode active materials. Based on two estimates, we calculate an average of 104 $ (kW h)−1.
Similar to LIBs, we find high variances for SSB, LSB and LAB forecasts. For SSBs, high uncertainties are related to solid electrolyte prices, lithium metal foil price, and required lithium excess. Solid electrolyte prices range from 10 to 100 $ kg−1, potentially since their large-scale synthesis process and related precursors are to date not determined.150–152 The required lithium excess varies from 50 to 300% between studies, influencing both energy density and cost. Further, lithium metal foil prices range from 100 to 1000 $ kg−1, assuming an integration of the lithium metal foil during the cell production process. Recently presented anode free concepts for SSBs have so far not been investigated regarding their cost impact. Within these concepts, the anode forms in situ on an initially lithium-free current collector during cycling.28,29,153,154 This concept offers further cost potentials, since sufficient cycle life can be achieved with no lithium excess,155,156 and cost-intensive foil production is rendered obsolete.19,157 Uncertainties for LSBs are mainly related to the amount of useable sulfur in the cell, expressed by sulfur loadings between 1 and ∼12 mg cm−2, and for LABs, derived variations can be dedicated to uncertainties in the share of useable energy. While LSBs and LABs benefit from low cathode material prices and show the lowest potential cost levels of 80 and 70 $ (kW h)−1. Interestingly, these forecasts are based on the lowest lithium metal anode price assumptions in our study.
4.6. An outlook to 2050 and the impact of material prices
The predicted time-specific LIB estimates from 22 studies that use a technological learning, literature-based or expert elicitation approach, yielding 237 data points, are gathered on the left-hand side of Fig. 9. Based on these, a rational regression (R2 value of 0.80) is applied in order to derive an aggregation of individual expectations throughout 2050 (indicated by a solid black line). We emphasize that this should not be considered as a literature-based forecast to 2050, but merely as a comprehensive picture of forecasted values from the past decade. Further, empirical price observations86,89 are included (signified as a histogram from 2010 to 2020). Rapidly decreasing forecasts from above 1000 $ (kW h)−1 to about 400 $ (kW h)−1 are observable from 2010 to 2015, when expected reductions tend to slow down and cost estimates reach a level of ∼230 $ (kW h)−1 in 2020. Further declines are expected for the next decades when aggregated forecasts reach levels of 132 $ (kW h)−1 in 2030, 92 $ (kW h)−1 in 2040 and 71 $ (kW h)−1 in 2050, respectively. Based on these expectations, the DoE target of 125 $ (kW h)−1 may be reached in 2032 at which EVs are expected to reach cost parity with conventional vehicles.158 In comparison to empirical evidence available for the time frame for 2010 to 2020, most forecasted values remain above market observations. While for the time frame between 2010 and 2014, estimated values are in line or below the empirical observations, in the period between 2015 and 2020, 90% of forecasted values are more pessimistic than observed prices. This is expressed by a continuously widening gap between the solid black line and the light red bars and indicates that forecasts in the examined literature have been on the pessimistic end in the past. Further, the persistent span of estimates above 130 $ (kW h)−1 throughout 2050 underlines the uncertainty associated with the prediction of LIB cost that will remain a key challenge in the future for researchers and companies in the field.
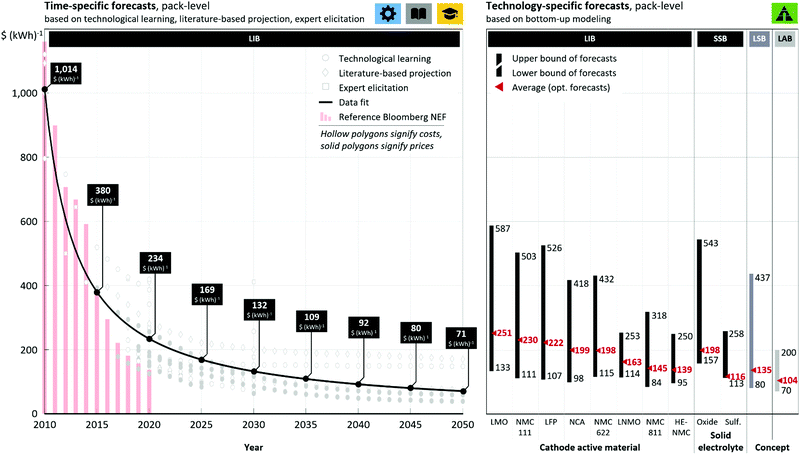 |
| Fig. 9 Overview of time-specific and technology-specific forecasts. | |
On the right-hand side of Fig. 9, technology-specific forecasts for LIB, SSB, LSB and LAB have been gathered. These are based on 26 studies for LIB (yielding 103 data points), three studies for SSB (twelve data points), three studies for LSB (five data points), and two studies for LAB (three data points). For LIB, forecasted values range from 84 to 587 $ (kW h)−1, with significant uncertainties across cathode technologies. The calculated average of forecasts drops from 251 $ (kW h)−1 for LIBs based on low specific capacity LMO to 139 $ (kW h)−1 for high capacity HE-NMC. Pack-level cost potentials below 100 $ (kW h)−1 are only shown for LIBs using NCA, NMC811 and HE-NMC in the examined studies. For sulfide SSB, LSB and LAB forecasts, the lowest averages of 116, 135 and 104 $ (kW h)−1 are calculated and for both of the latter, the lowest cost potentials of 80 and 70 $ (kW h)−1 are indicated. When comparing time- and technology-specific LIB forecasts, a decreasing trend can be perceived along the time dimension and for more advanced materials and concepts. However, the feasibility of around half of time-specific forecasts after 2030 is currently not supported by bottom-up LIB calculations. Nevertheless, for LIBs, further cost reductions are to be expected on pack-level by cell-to-pack technology that have so far been not investigated in detail. For lithium metal batteries, in particular anode-free cell concepts promise future cost potentials by eliminating cost-intensive lithium foil processing in cell production and the necessity for lithium excess, that are currently not reflected in the displayed forecasts.
Market analysts express a growing concern regarding the impact of rising raw material prices on battery cost.159,160 On the one hand material demand is expected to grow significantly,161,162 on the other, currently committed investments for future mining and refining capacities are considered insufficient163,164 and recycling volumes will not have a notably easing impact before 2030.161,165 The studies in our analysis that examine material price fluctuations send less alarming signals with regard to battery cost. Lithium precursor price increases are shown to have a minor impact on NMC, NCA and LMO-based LIB cost.110,111 Further, time-specific forecasts that reflect increasing price developments for lithium, cobalt and/or nickel nevertheless derive declining battery cost for NMC or NCA-based chemistries.15,39,94 In addition, several LIB technologies are shown to be less vulnerable for increasing cobalt and nickel prices and as such present a lower risk pathway to reduced battery cost.15 In contrast, we find significant forecast risks associated with the lithium metal anode used in SSB, LSB and LAB, where price assumptions vary significantly between studies. With regard to SSBs, the price of solid electrolytes further drives uncertainty, still impeding an exact forecast of SSB competitiveness.
5. Summary and conclusion
In the present article, 53 studies on battery cost forecasting published in the scientific community have been reviewed that apply four general methods to derive predictions. Our analysis underlines that there is no such thing as the battery cost. The conducted literature review reveals a multitude of interacting dimensions that researchers investigate in order to find the right answers to their specific questions. We find studies applying technological learning, literature-based projection and expert elicitation to focus on time-specific forecasting, and bottom-up modeling to focus on technology-specific forecasting. Every single study that provides time-based projections expects LIB cost to fall, even if increasing raw and battery material prices are taken into account. Recent technological learning studies expect higher battery-specific learning potentials and show confidence in a more stable battery market growth. Literature-based projections are shown to differ in both, consulted data sources and applied aggregation technique, but can provide forecasts with limited effort. Expert elicitations allow for insights in opinions and doubts regarding fundamentally new battery technologies, however, our analysis indicates a lack of consulted experts with sufficient knowledge of process improvements in the examined studies. While the majority of past estimates is found to exceed the chosen empirical benchmark, the consolidation of 237 time-specific data points representing academic expectations, yields a continuously declining trajectory that reaches pack-level value of 71 $ (kW h)−1 in 2050. Studies based on bottom-up modeling evaluate the technological concepts of LIB, SSB, LSB and LAB. Most studies examine LIB technology and base their estimates on a variety of cathode technologies. Among those, advanced high-voltage or high-capacity materials indicate the lowest potential cost levels that reach down to 84 $ (kW h)−1. Among concepts that integrate a lithium-metal anode, sulfide SSB, LSB and LAB promise cost advantages and a minimum value of 70 $ (kW h)−1 is forecasted in respective studies. Across studies and methods, we find high uncertainty in the level of forecasted values on chronological and technological level that will remain a key challenge for researchers and companies in the field. This uncertainty reflects different assumptions or beliefs regarding market expectations, material prices, and technological specifications underlying each of the examined studies.
Our study provides transparency on the drivers behind this uncertainty and sheds light on relevant publications on battery cost. It contributes to the field of battery technology in particular, and to the field of energy transition in general by first, presenting a systematic overview of 53 battery cost forecasting studies regarding time of publication, forecast horizon, applied forecasting method, battery application and examined technology, providing readers with a navigator to identify a study suitable for their needs. Second, by creating transparency on method-specific forecast drivers and assumptions, thereby allowing readers to forge an informed opinion on forecasts and enable a choice that matches their beliefs. Third, by providing a data base consisting of the presented 360 forecast data points and more than 1000 scenario-specific estimates extracted from the examined studies. Fourth, by aggregating these data points into a trajectory throughout 2050 and into 12 technology-specific forecast ranges.
Our analysis is limited by several aspects that readers should be aware of. First, even though we applied a multi-step approach to retrieve relevant publications, the number of identified studies for several methods and technologies is limited and does not allow for general conclusions regarding their forecasting quality. Second, while in economic literature a clear distinction between the definition of cost and prices exists, in battery literature both terms are frequently used interchangeable. In our analysis, both terms have been treated equally but have been signified differently in all figures. Third, an explanation between all forecasted values that is sufficiently brief and conclusive is not always achievable since some models are stated to exceed a number of 250 parameters and full transparency is seldomly provided. For further details we refer to Section 2, the ESI,† and the original source. Fourth, the calculated average of technology-specific forecasts includes baseline estimates and, if ranges are provided, optimistic estimates. Hence, it represents an optimistic view on technology, and has been marked accordingly in all figures.
This review demonstrates a strong belief in both, declining future battery cost and its transformative impact on the energy and mobility sector. Our analysis provides the required transparency for an understanding of the economics behind battery technology, which is vital for a smooth transition towards a climate-neutral future.
Funding sources
This research did not receive any specific grant from funding agencies in the public, commercial, or not-for-profit sectors.
Conflicts of interest
The authors of this article state that there are no conflicts of interest related to this manuscript.
References
- B. Nykvist and M. Nilsson, Rapidly falling costs of battery packs for electric vehicles, Nat. Clim. Change, 2015, 5, 329–332 CrossRef
.
- F. Duffner, M. Wentker, M. Greenwood and J. Leker, Battery cost modeling: A review and directions for future research, Renewable Sustainable Energy Rev., 2020, 127, 109872 CrossRef
.
- R. Schmuch, R. Wagner, G. Hörpel, T. Placke and M. Winter, Performance and cost of materials for lithium-based rechargeable automotive batteries, Nat. Energy, 2018, 3, 267–278 CrossRef CAS
.
- Cutting cobalt, Nat. Energy, 2020, 5, 825 CrossRef
.
- S. Comello and S. Reichelstein, The emergence of cost effective battery storage, Nat. Commun., 2019, 10, 2038 CrossRef PubMed
.
- R. M. Darling, K. G. Gallagher, J. A. Kowalski, S. Ha and F. R. Brushett, Pathways to low-cost electrochemical energy storage: a comparison of aqueous and nonaqueous flow batteries, Energy Environ. Sci., 2014, 7, 3459–3477 RSC
.
-
R. Madlener and J. M. Specht, Business Opportunities and the Regulatory Framework, 2018, pp. 296–326 10.1039/9781788015530-00296
.
- H. H. Heimes, A. Kampker, A. Haunreiter, H. Davids and D. Klohs, Product-requirement-model to approach the identification of uncertainties in battery systems development, Int. J. Interact. Des. Manuf., 2020, 14, 911–922 CrossRef
.
- M. Marinaro,
et al., Bringing forward the development of battery cells for automotive applications: Perspective of R&D activities in China, Japan, the EU and the USA, J. Power Sources, 2020, 459, 228073 CrossRef CAS
.
- A. Mezősi, L. Szabó and S. Szabó, Cost-efficiency benchmarking of European renewable electricity support schemes, Renewable Sustainable Energy Rev., 2018, 98, 217–226 CrossRef
.
- I. Staffell,
et al., The role of hydrogen and fuel cells in the global energy system, Energy Environ. Sci., 2019, 12, 463–491 RSC
.
- A. Opitz, P. Badami, L. Shen, K. Vignarooban and A. M. Kannan, Can Li-Ion batteries be the panacea for automotive applications?, Renewable Sustainable Energy Rev., 2017, 68, 685–692 CrossRef CAS
.
- L. J. Aaldering, J. Leker and C. H. Song, Analysis of technological knowledge stock and prediction of its future development potential: The case of lithium-ion batteries, J. Cleaner Prod., 2019, 223, 301–311 CrossRef
.
- O. Schmidt, A. Hawkes, A. Gambhir and I. Staffell, The future cost of electrical energy storage based on experience rates, Nat. Energy, 2017, 2, 17110 CrossRef
.
- M. Beuse, B. Steffen and T. S. Schmidt, Projecting the Competition between Energy-Storage Technologies in the Electricity Sector, Joule, 2020, 4, 2162–2184 CrossRef CAS
.
- A. S. Mundada, K. K. Shah and J. M. Pearce, Levelized cost of electricity for solar photovoltaic, battery and cogen hybrid systems, Renewable Sustainable Energy Rev., 2016, 57, 692–703 CrossRef
.
- L. Mauler, F. Duffner and J. Leker, Economies of scale in battery cell manufacturing: The impact of material and process innovations, Appl. Energy, 2021, 286, 116499 CrossRef
.
- S. Randau,
et al., Benchmarking the performance of all-solid-state lithium batteries, Nat. Energy, 2020, 5, 259–270 CrossRef CAS
.
- F. Duffner,
et al., Post-lithium-ion battery cell production and its compatibility with lithium-ion cell production infrastructure, Nat. Energy, 2021, 6, 123–134 CrossRef CAS
.
- J. Schnell,
et al., Prospects of production technologies and manufacturing costs of oxide-based all-solid-state lithium batteries, Energy Environ. Sci., 2019, 12, 1818–1833 RSC
.
- C. Vaalma, D. Buchholz, M. Weil and S. Passerini, A cost and resource analysis of sodium-ion batteries, Nat. Rev. Mater., 2018, 3, 18013 CrossRef
.
- K. G. Gallagher,
et al., Quantifying the promise of lithium–air batteries for electric vehicles, Energy Environ. Sci., 2014, 7, 1555 RSC
.
- M. Hagen,
et al., Lithium-Sulfur Cells: The Gap between the State-of-the-Art and the Requirements for High Energy Battery Cells, Adv. Energy Mater., 2015, 5, 1401986 CrossRef
.
- H.-D. Lim,
et al., Reaction chemistry in rechargeable Li–O 2 batteries, Chem. Soc. Rev., 2017, 46, 2873–2888 RSC
.
- F. Wu and Y. Yu, Toward True Lithium-Air Batteries, Joule, 2018, 2, 815–817 CrossRef
.
- P. Bonnick and J. Muldoon, The Dr Jekyll and Mr Hyde of lithium sulfur batteries, Energy Environ. Sci., 2020, 13, 4808–4833 RSC
.
- Z.-J. Zheng, H. Ye and Z.-P. Guo, Recent progress on pristine metal/covalent-organic frameworks and their composites for lithium–sulfur batteries, Energy Environ. Sci., 2021, 14, 1835–1853 RSC
.
- J. Schnell,
et al., All-solid-state lithium-ion and lithium metal batteries – paving the way to large-scale production, J. Power Sources, 2018, 382, 160–175 CrossRef CAS
.
- S. Nanda, A. Gupta and A. Manthiram, Anode-Free Full Cells: A Pathway to High-Energy Density Lithium-Metal Batteries, Adv. Energy Mater., 2021, 11, 2000804 CrossRef CAS
.
- F. Duffner, O. Krätzig and J. Leker, Battery plant location considering the balance between knowledge and cost: A comparative study of the EU-28 countries, J. Cleaner Prod., 2020, 264, 121428 CrossRef
.
- J. Janek and W. G. Zeier, A solid future for battery development, Nat. Energy, 2016, 1, 16141 CrossRef
.
- N. Kittner, F. Lill and D. M. Kammen, Energy storage deployment and innovation for the clean energy transition, Nat. Energy, 2017, 2, 17125 CrossRef
.
- M. Catenacci, E. Verdolini, V. Bosetti and G. Fiorese, Going electric: Expert survey on the future of battery technologies for electric vehicles, Energy Policy, 2013, 61, 403–413 CrossRef
.
- E. Baker, H. Chon and J. Keisler, Battery technology for electric and hybrid vehicles: Expert views about prospects for advancement, Technol. Forecast. Soc. Change, 2010, 77, 1139–1146 CrossRef
.
- G. Berckmans,
et al., Cost Projection of State of the Art Lithium-Ion Batteries for Electric Vehicles Up to 2030, Energies, 2017, 10, 1314 CrossRef
.
- C. Thiel, A. Perujo and A. Mercier, Cost and CO2 aspects of future vehicle options in Europe under new energy policy scenarios, Energy Policy, 2010, 38, 7142–7151 CrossRef
.
- O. Schmidt, S. Melchior, A. Hawkes and I. Staffell, Projecting the Future Levelized Cost of Electricity Storage Technologies, Joule, 2019, 3, 81–100 CrossRef
.
- S. J. Gerssen-Gondelach and A. P. C. Faaij, Performance of batteries for electric vehicles on short and longer term, J. Power Sources, 2012, 212, 111–129 CrossRef CAS
.
- I.-Y. L. Hsieh, M. S. Pan, Y.-M. Chiang and W. H. Green, Learning only buys you so much: Practical limits on battery price reduction, Appl. Energy, 2019, 239, 218–224 CrossRef
.
- S. Matteson and E. Williams, Learning dependent subsidies for lithium-ion electric vehicle batteries, Technol. Forecast. Soc. Change, 2015, 92, 322–331 CrossRef
.
- S. Matteson and E. Williams, Residual learning rates in lead–acid batteries: Effects on emerging technologies, Energy Policy, 2015, 85, 71–79 CrossRef CAS
.
- L. Argote and D. Epple, Learning Curves in Manufacturing, Science, 1990, 247, 920–924 CrossRef CAS PubMed
.
- T. P. Wright, Factors Affecting the Cost of Airplanes, J. Aeronaut. Sci., 1936, 3, 122–128 CrossRef
.
- A. Alchian, Reliability of Progress Curves in Airframe Production, Econometrica, 1963, 31, 679 CrossRef
.
- L. Rapping, Learning and World War II Production Functions, Rev. Econ. Stat., 1965, 47, 81 CrossRef
.
- E. S. Rubin, I. M. L. Azevedo, P. Jaramillo and S. Yeh, A review of learning rates for electricity supply technologies, Energy Policy, 2015, 86, 198–218 CrossRef
.
- S. Yeh and E. S. Rubin, A review
of uncertainties in technology experience curves, Energy Econ., 2012, 34, 762–771 CrossRef
.
- S. Samadi, The experience curve theory and its application in the field of electricity generation technologies – A literature review, Renewable Sustainable Energy Rev., 2018, 82, 2346–2364 CrossRef
.
- R. J. Detz, J. N. H. Reek and B. C. C. van der Zwaan, The future of solar fuels: when could they become competitive?, Energy Environ. Sci., 2018, 11, 1653–1669 RSC
.
- B. Lee,
et al., Projected economic outlook and scenario analysis for H 2 production by alkaline water electrolysis on the basis of the unit electricity price, the learning rate, and the automation level, Sustainable Energy Fuels, 2019, 3, 1799–1807 RSC
.
- G. Glenk and S. Reichelstein, Economics of converting renewable power to hydrogen, Nat. Energy, 2019, 4, 216–222 CrossRef CAS
.
- A. F. Atiya, Why does forecast combination work so well?, Int. J. Forecast., 2020, 36, 197–200 CrossRef
.
-
A. Timmermann, Forecast Combinations, 2006, ch. 4, pp. 135–196 DOI:10.1016/S1574-0706(05)01004-9
.
- R. T. Clemen, Combining forecasts: A review and annotated bibliography, Int. J. Forecast., 1989, 5, 559–583 CrossRef
.
- M. Thema, F. Bauer and M. Sterner, Power-to-Gas: Electrolysis and methanation status review, Renewable Sustainable Energy Rev., 2019, 112, 775–787 CrossRef CAS
.
- D. Apostolou and G. Xydis, A literature review on hydrogen refuelling stations and infrastructure. Current status and future prospects, Renewable Sustainable Energy Rev., 2019, 113, 109292 CrossRef
.
- J. E. Bistline, Energy technology expert elicitations: An application to natural gas turbine efficiencies, Technol. Forecast. Soc. Change, 2014, 86, 177–187 CrossRef
.
- V. Bosetti,
et al., Sensitivity to energy technology costs: A multi-model comparison analysis, Energy Policy, 2015, 80, 244–263 CrossRef
.
- M. M. Whiston,
et al., Expert assessments of the cost and expected future performance of proton exchange membrane fuel cells for vehicles, Proc. Natl. Acad. Sci. U. S. A., 2019, 116, 4899–4904 CrossRef CAS PubMed
.
- O. Schmidt,
et al., Future cost and performance of water electrolysis: An expert elicitation study, Int. J. Hydrogen Energy, 2017, 42, 30470–30492 CrossRef CAS
.
- R. Wiser,
et al., Expert elicitation survey predicts 37% to 49% declines in wind energy costs by 2050, Nat. Energy, 2021, 6, 555–565 CrossRef
.
- V. Bosetti, M. Catenacci, G. Fiorese and E. Verdolini, The future prospect of PV and CSP solar technologies: An expert elicitation survey, Energy Policy, 2012, 49, 308–317 CrossRef
.
- L. T. Lam, L. Branstetter and I. L. Azevedo, A sunny future: expert elicitation of China's solar photovoltaic technologies, Environ. Res. Lett., 2018, 13, 034038 CrossRef
.
- M. G. Use Morgan, and abuse) of expert elicitation in support of decision making for public policy, Proc. Natl. Acad. Sci. U. S. A., 2014, 111, 7176–7184 CrossRef CAS PubMed
.
- C. Hueber, K. Horejsi and R. Schledjewski, Review of cost estimation: methods and models for aerospace composite manufacturing, Adv. Manuf.: Polym. Compos. Sci., 2016, 2, 1–13 Search PubMed
.
- M. Schulze, S. Seuring and C. Ewering, Applying activity-based costing in a supply chain environment, Int. J. Prod. Econ., 2012, 135, 716–725 CrossRef
.
- R. Curran, S. Raghunathan and M. Price, Review of aerospace engineering cost modelling: The genetic causal approach, Prog. Aerosp. Sci., 2004, 40, 487–534 CrossRef
.
- R. Anghilante, D. Colomar, A. Brisse and M. Marrony, Bottom-up cost evaluation of SOEC systems in the range of 10–100 MW, Int. J. Hydrogen Energy, 2018, 43, 20309–20322 CrossRef CAS
.
- S. Harboe,
et al., Manufacturing cost model for planar 5 kWel SOFC stacks at Forschungszentrum Jülich, Int. J. Hydrogen Energy, 2020, 45, 8015–8030 CrossRef CAS
.
-
K. A. W. Horowitz, M. Woodhouse, H. Lee and G. P. Smestad, A bottom-up cost analysis of a high concentration PV module, 2015, p. 100001 DOI:10.1063/1.4931548
.
- A. Elia, M. Taylor, B. Ó Gallachóir and F. Rogan, Wind turbine cost reduction: A detailed bottom-up analysis of innovation drivers, Energy Policy, 2020, 147, 111912 CrossRef
.
- N. L. Chang,
et al., A bottom-up cost analysis of silicon–perovskite tandem photovoltaics, Prog. Photovoltaics Res. Appl., 2021, 29, 401–413 CrossRef CAS
.
- L. M. H. Hall and A. R. Buckley, A review of energy systems models in the UK: Prevalent usage and categorisation, Appl. Energy, 2016, 169, 607–628 CrossRef
.
- P. Lopion, P. Markewitz, M. Robinius and D. Stolten, A review of current challenges and trends in energy systems modeling, Renewable Sustainable Energy Rev., 2018, 96, 156–166 CrossRef
.
- S. Few,
et al., Prospective improvements in cost and cycle life of off-grid lithium-ion battery packs: An analysis informed by expert elicitations, Energy Policy, 2018, 114, 578–590 CrossRef
.
- F. Duffner, L. Mauler, M. Wentker, J. Leker and M. Winter, Large-scale automotive battery cell manufacturing: Analyzing strategic and operational effects on manufacturing costs, Int. J. Prod. Econ., 2021, 232, 107982 CrossRef
.
- A. Sakti,
et al., Consistency and robustness of forecasting for emerging technologies: The case of Li-ion batteries for electric vehicles, Energy Policy, 2017, 106, 415–426 CrossRef
.
-
M. J. Yoo and R. Glardon, Manufacturing Operations Management, World Scientific Publishing, 2018 Search PubMed
.
- BloombergNEF, Lithium-ion Battery Costs and Market, 1 http://enerjiye.com/wp-content/uploads/2018/12/battery-market.pdf, 2017.
- B. Nykvist, F. Sprei and M. Nilsson, Assessing the progress toward lower priced long range battery electric vehicles, Energy Policy, 2019, 124, 144–155 CrossRef
.
- U.S. Department of Energy, Cost and Price Metrics for Automotive Lithium-Ion Batteries, https://www.energy.gov/sites/prod/files/2017/02/f34/67089 EERE LIB cost vs price metrics r9.pdf, 2017.
-
M. E. Porter, Competitive Strategy: Techniques for Analyzing Industries and Competitors, The Free Press, 1998 Search PubMed
.
- A. Kwade,
et al., Current status and challenges for automotive battery production technologies, Nat. Energy, 2018, 3, 290–300 CrossRef
.
- Avicienne Energy, Impact of the EV Market Growth on Lithium-ion Batteries and Raw Materials Supply 2019-2030, 2020.
- Roland Berger, Battery Recycling is a Key Market of the Future: Is it also an Opportunity for Europe? https://www.rolandberger.com/en/Insights/Publications/Battery-recycling-is-a-key-market-of-the-future-Is-it-also-an-opportunity-for.html, 2019.
- BloombergNEF, Battery Pack Prices Cited Below $100/kW h for the First Time in 2020, While Market Average Sits at $137/kW h, https://about.bnef.com/blog/battery-pack-prices-cited-below-100-kwh-for-the-first-time-in-2020-while-market-average-sits-at-137-kwh/, 2021.
- Benchmark Mineral Intelligence, Low Cost EVs = Low Cost Batteries = Controlling Your Supply Chain, https://twitter.com/sdmoores/status/1280221160605712386/photo/1, 2020.
- Federal Reserve. Foreign Exchange Rates, Data Download Program, https://www.federalreserve.gov/datadownload/Download.aspx?rel=H10&series=60f32914ab61dfab590e0e470153e3ae&filetype=spreadsheetml&label=include&layout=seriescolumn&from=01/01/2010&to=12/31/2020, 2021.
- BloombergNEF, A Behind the Scenes Take on Lithium-ion Battery Prices, https://about.bnef.com/blog/behind-scenes-take-lithium-ion-battery-prices/, 2019.
- European Commission, Li-ion batteries for mobility and stationary storage applications, https://publications.jrc.ec.europa.eu/repository/bitstream/JRC113360/kjna29440enn.pdf, 2018.
- T. Mayer, D. Kreyenberg, J. Wind and F. Braun, Feasibility study of 2020 target costs for PEM fuel cells and lithium-ion batteries: A two-factor experience curve approach, Int. J. Hydrogen Energy, 2012, 37, 14463–14474 CrossRef CAS
.
- O. Y. Edelenbosch, A. F. Hof, B. Nykvist, B. Girod and D. P. van Vuuren, Transport electrification: the effect of recent battery cost reduction on future emission scenarios, Clim. Change, 2018, 151, 95–108 CrossRef
.
- D. Zhou, H. Ding, P. Zhou and Q. Wang, Learning curve with input price for tracking technical change in the energy transition process, J. Cleaner Prod., 2019, 235, 997–1005 CrossRef
.
- X. N. Penisa,
et al., Projecting the Price of Lithium-Ion NMC Battery Packs Using a Multifactor Learning Curve Model, Energies, 2020, 13, 5276 CrossRef CAS
.
- M. Weiss,
et al., On the electrification of road transport – Learning rates and price forecasts for hybrid-electric and battery-electric vehicles, Energy Policy, 2012, 48, 374–393 CrossRef
.
-
W. J. Cole, C. Marcy, V. K. Krishnan and R. Margolis, Utility-scale lithium-ion storage cost projections for use in capacity expansion models, in 2016 North American Power Symposium (NAPS) 1–6 (IEEE, 2016) DOI:10.1109/NAPS.2016.7747866.
- Z. P. Cano,
et al., Batteries and fuel cells for emerging electric vehicle markets, Nat. Energy, 2018, 3, 279–289 CrossRef
.
- K. Mongird,
et al., An Evaluation of Energy Storage Cost and Performance Characteristics, Energies, 2020, 13, 3307 CrossRef CAS
.
- X. He,
et al., Greenhouse gas consequences of the China dual credit policy, Nat. Commun., 2020, 11, 5212 CrossRef CAS PubMed
.
- R. J. Brodd and C. Helou, Cost comparison of producing high-performance Li-ion batteries in the U.S. and in China, J. Power Sources, 2013, 231, 293–300 CrossRef CAS
.
-
P. A. Nelson, S. Ahmed, K. G. Gallagher and D. W. Dees, Modeling the Performance and Cost of Lithium-Ion Batteries for Electric-Drive Vehicles, 2019 Search PubMed
.
- G. Patry, A. Romagny, S. Martinet and D. Froelich, Cost modeling of lithium-ion battery cells for automotive applications, Energy Sci. Eng., 2015, 3, 71–82 CrossRef
.
- P. A. Nelson, S. Ahmed, K. G. Gallagher and D. W. Dees, Cost savings for manufacturing lithium batteries in a flexible plant, J. Power Sources, 2015, 283, 506–516 CrossRef CAS
.
- D. Eroglu, K. R. Zavadil and K. G. Gallagher, Critical Link between Materials Chemistry and Cell-Level Design for High Energy Density and Low Cost Lithium-Sulfur Transportation Battery, J. Electrochem. Soc., 2015, 162, A982–A990 CrossRef CAS
.
-
J. H. Schünemann, Modell zur Bewertung der Herstellkosten von Lithiumionenbatteriezellen, Technische Universität Braunschweig, 2015 Search PubMed
.
- R. Petri, T. Giebel, B. Zhang, J.-H. Schünemann and C. Herrmann, Material cost model for innovative li-ion battery cells in electric vehicle applications, Int. J. Precis. Eng. Manuf. Technol., 2015, 2, 263–268 CrossRef
.
- A. Sakti, J. J. Michalek, E. R. H. Fuchs and J. F. Whitacre, A techno-economic analysis and optimization of Li-ion batteries for light-duty passenger vehicle electrification, J. Power Sources, 2015, 273, 966–980 CrossRef CAS
.
- E. J. Berg, C. Villevieille, D. Streich, S. Trabesinger and P. Novák, Rechargeable Batteries: Grasping for the Limits of Chemistry, J. Electrochem. Soc., 2015, 162, A2468–A2475 CrossRef CAS
.
- D. L. Wood, J. Li and C. Daniel, Prospects for reducing the processing cost of lithium ion batteries, J. Power Sources, 2015, 275, 234–242 CrossRef CAS
.
- R. E. Ciez and J. F. Whitacre, The cost of lithium is unlikely to upend the price of Li-ion storage systems, J. Power Sources, 2016, 320, 310–313 CrossRef CAS
.
- R. E. Ciez and J. F. Whitacre, Comparison between cylindrical and prismatic lithium-ion cell costs using a process based cost model, J. Power Sources, 2017, 340, 273–281 CrossRef CAS
.
- M. Safoutin, J. McDonald and B. Ellies, Predicting the Future Manufacturing Cost of Batteries for Plug-In Vehicles for the U.S. Environmental Protection Agency (EPA) 2017–2025 Light-Duty Greenhouse Gas Standards, World Electr. Veh. J., 2018, 9, 42 CrossRef
.
- M. Philippot, G. Alvarez, E. Ayerbe, J. Van Mierlo and M. Messagie, Eco-Efficiency of a Lithium-Ion Battery for Electric Vehicles: Influence of Manufacturing Country and Commodity Prices on GHG Emissions and Costs, Batteries, 2019, 5, 23 CrossRef CAS
.
- M. Wentker, M. Greenwood and J. Leker, A Bottom-Up Approach to Lithium-Ion Battery Cost Modeling with a Focus on Cathode Active Materials, Energies, 2019, 12, 504 CrossRef CAS
.
- J. Schnell, H. Knörzer, A. J. Imbsweiler and G. Reinhart, Solid versus Liquid—A Bottom-Up Calculation Model to Analyze the Manufacturing Cost of Future High-Energy Batteries, Energy Technol., 2020, 8, 1901237 CrossRef CAS
.
- R. E. Ciez and D. Steingart, Asymptotic Cost Analysis of Intercalation Lithium-Ion Systems for Multi-hour Duration Energy Storage, Joule, 2020, 4, 597–614 CrossRef CAS
.
- Z. Yan and M. N. Obrovac, Quantifying the cost effectiveness of non-aqueous potassium-ion batteries, J. Power Sources, 2020, 464, 228228 CrossRef CAS
.
- T. Nemeth, P. Schröer, M. Kuipers and D. U. Sauer, Lithium titanate oxide battery cells for high-power automotive applications – Electro-thermal properties, aging behavior and cost considerations, J. Energy Storage, 2020, 31, 101656 CrossRef
.
- B. Nagy, J. D. Farmer, Q. M. Bui and J. E. Trancik, Statistical Basis for Predicting Technological Progress, PLoS One, 2013, 8, e52669 CrossRef CAS PubMed
.
- A. McDonald and L. Schrattenholzer, Learning rates for energy technologies, Energy Policy, 2001, 29, 255–261 CrossRef
.
-
M. Junginger, W. van Sark and A. Faaij, Technological Learning in the Energy Sector, Edward Elgar Publishing, 2010 DOI:10.4337/9781849806848
.
- M. Junginger, A. Faaij and W. Turkenburg, Global experience curves for wind farms, Energy Policy, 2005, 33, 133–150 CrossRef
.
- R. E. Ciez and J. F. Whitacre, Examining different recycling processes for lithium-ion batteries, Nat. Sustainable, 2019, 2, 148–156 CrossRef
.
- G. Zubi, R. Dufo-López, M. Carvalho and G. Pasaoglu, The lithium-ion battery: State of the art and future perspectives, Renewable Sustainable Energy Rev., 2018, 89, 292–308 CrossRef
.
- T. M. Gür, Review of electrical energy storage technologies, materials and systems: challenges and prospects for large-scale grid storage, Energy Environ. Sci., 2018, 11, 2696–2767 RSC
.
-
W. Bernhart, Challenges and Opportunities in Lithium-ion Battery Supply, Future Lithium-ion Batteries, Royal Society of Chemistry, 2019, ch. 13, pp. 316–334 10.1039/9781788016124-00316
.
- T. Placke, R. Kloepsch, S. Dühnen and M. Winter, Lithium ion, lithium metal, and alternative rechargeable battery technologies: the odyssey for high energy density, J. Solid State Electrochem., 2017, 21, 1939–1964 CrossRef CAS
.
- D. Andre,
et al., Future generations of cathode materials: an automotive industry perspective, J. Mater. Chem. A, 2015, 3, 6709–6732 RSC
.
- M.-J. Lee, S. Lee, P. Oh, Y. Kim and J. Cho, High Performance LiMn 2 O 4 Cathode Materials Grown with Epitaxial Layered Nanostructure for Li-Ion Batteries, Nano Lett., 2014, 14, 993–999 CrossRef CAS PubMed
.
- S. Brutti,
et al., Interplay between local structure and transport properties in iron-doped LiCoPO 4 olivines, J. Mater. Chem. A, 2017, 5, 14020–14030 RSC
.
- J. Ludwig and T. Nilges, Recent progress and developments in lithium cobalt phosphate chemistry- Syntheses, polymorphism and properties, J. Power Sources, 2018, 382, 101–115 CrossRef CAS
.
- G. Liang, V. K. Peterson, K. W. See, Z. Guo and W. K. Pang, Developing high-voltage spinel LiNi 0.5 Mn 1.5 O 4 cathodes for high-energy-density lithium-ion batteries: current achievements and future prospects, J. Mater. Chem. A, 2020, 8, 15373–15398 RSC
.
- W. Zuo,
et al., Li-rich cathodes for rechargeable Li-based batteries: reaction mechanisms and advanced characterization techniques, Energy Environ. Sci., 2020, 13, 4450–4497 RSC
.
- S. S. Sharma and A. Manthiram, Towards more environmentally and socially responsible batteries, Energy Environ. Sci., 2020, 13, 4087–4097 RSC
.
- Y. Wang, C.-Y. Wu, H. Yang and J.-G. Duh, Rational design of a synthetic strategy, carburizing approach and pore-forming pattern to unlock the cycle reversibility and rate capability of micro-agglomerated LiMn 0.8 Fe 0.2 PO 4 cathode materials, J. Mater. Chem. A, 2018, 6, 10395–10403 RSC
.
- H.-H. Ryu, H. H. Sun, S.-T. Myung, C. S. Yoon and Y.-K. Sun, Reducing cobalt from lithium-ion batteries for the electric vehicle era, Energy Environ. Sci., 2021, 14, 844–852 RSC
.
- J. Kim,
et al., A highly stabilized nickel-rich cathode material by nanoscale epitaxy control for high-energy lithium-ion batteries, Energy Environ. Sci., 2018, 11, 1449–1459 RSC
.
- W. Li, E. M. Erickson and A. Manthiram, High-nickel layered oxide cathodes for lithium-based automotive batteries, Nat. Energy, 2020, 5, 26–34 CrossRef CAS
.
- X. Wu,
et al., Effect of stirring environment humidity on electrochemical performance of nickel-rich cathode materials as lithium ion batteries, Ionics, 2020, 26, 5427–5434 CrossRef CAS
.
- X. Li,
et al., Optimal synthetic conditions for a novel and high performance Ni-rich cathode material of LiNi 0.68 Co 0.10 Mn 0.22 O 2, Sustainable Energy Fuels, 2018, 2, 1772–1780 RSC
.
- A. L. Lipson,
et al., Stabilizing NMC 811 Li-Ion Battery Cathode through a Rapid Coprecipitation Process, ACS Appl. Energy Mater., 2021, 4, 1972–1977 CrossRef CAS
.
- U. Kim,
et al., Microstructure-Controlled Ni-Rich Cathode Material by Microscale Compositional Partition for Next-Generation Electric Vehicles, Adv. Energy Mater., 2019, 9, 1803902 CrossRef
.
- X.-G. Yang, T. Liu and C.-Y. Wang, Thermally modulated lithium iron phosphate batteries for mass-market electric vehicles, Nat. Energy, 2021, 6, 176–185 CrossRef CAS
.
- H. Wang,
et al., An experimental study on the thermal characteristics of the Cell-To-Pack system, Energy, 2021, 227, 120338 CrossRef
.
- G. Zhu,
et al., Fast Charging Lithium Batteries: Recent Progress and Future Prospects, Small, 2019, 15, 1805389 CrossRef PubMed
.
- P.-J. Lian,
et al., Inorganic sulfide solid electrolytes for all-solid-state lithium secondary batteries, J. Mater. Chem. A, 2019, 7, 20540–20557 RSC
.
- C. Wang,
et al., All-solid-state lithium batteries enabled by sulfide electrolytes: from fundamental research to practical engineering design, Energy Environ. Sci., 2021, 14, 2577–2619 RSC
.
- Z. Ma,
et al., A review of cathode materials and structures for rechargeable lithium–air batteries, Energy Environ. Sci., 2015, 8, 2144–2198 RSC
.
- Q. Pang, X. Liang, C. Y. Kwok and L. F. Nazar, Advances in lithium–sulfur batteries based on multifunctional cathodes and electrolytes, Nat. Energy, 2016, 1, 16132 CrossRef CAS
.
- K. Yuan,
et al., Methods and Cost Estimation for the Synthesis of Nanosized Lithium Sulfide, Small Struct., 2021, 2, 2000059 CrossRef
.
- A. Jonderian and E. McCalla, The role of metal substitutions in the development of Li batteries, part II: solid electrolytes, Mater. Adv., 2021, 2, 2846–2875 RSC
.
- A. Banik,
et al., On the underestimated influence of synthetic conditions in solid ionic conductors, Chem. Sci., 2021, 12, 6238–6263 RSC
.
- J. Qian,
et al., Anode-Free Rechargeable Lithium Metal Batteries, Adv. Funct. Mater., 2016, 26, 7094–7102 CrossRef CAS
.
- X. Yang, K. R. Adair, X. Gao and X. Sun, Recent advances and perspectives on thin electrolytes for high-energy-density solid-state lithium batteries, Energy Environ. Sci., 2021, 14, 643–671 RSC
.
- Y.-G. Lee,
et al., High-energy long-cycling all-solid-state lithium metal batteries enabled by silver–carbon composite anodes, Nat. Energy, 2020, 5, 299–308 CrossRef CAS
.
- K. J. Kim, J. J. Hinricher and J. L. M. Rupp, High energy and long cycles, Nat. Energy, 2020, 5, 278–279 CrossRef CAS
.
- M. J. Wang, E. Carmona, A. Gupta, P. Albertus and J. Sakamoto, Enabling “lithium-free” manufacturing of pure lithium metal solid-state batteries through in situ plating, Nat. Commun., 2020, 11, 5201 CrossRef CAS PubMed
.
- U.S. Department of Energy, Overview of the DOE VTO Advanced Battery R&D Program, https://www.energy.gov/sites/prod/files/2016/06/f32/es000_howell_2016_o_web.pdf, 2016.
- Benchmark Mineral Intelligence, Lithium-ion battery cell prices fall to $110/kW h, but raw material risk looms large, https://www.benchmarkminerals.com/membership/lithium-ion-battery-cell-prices-fall-to-110-kwh-but-raw-material-risk-looms-large-2/, 2020.
-
G. Sachs, Electric automakers must brace for rising battery materials costs, Goldman says, CNBC, https://www.cnbc.com/2021/03/24/goldman-electric-cars-face-rising-battery-lithium-nickel-cobalt-costs.html, 2021.
- C. Xu,
et al., Future material demand for automotive lithium-based batteries, Commun. Mater., 2020, 1, 99 CrossRef
.
- H. Hao,
et al., Impact of transport electrification on critical metal sustainability with a focus on the heavy-duty segment, Nat. Commun., 2019, 10, 5398 CrossRef PubMed
.
-
J. Fraser, J. Anderson, J. Lazuen, Y. Lu, O. Heathman, N. Brewster, J. Bedder and O. Masson, Study on future demand and supply security of nickel for electric vehicle batteries, Publications Office of the European Union, Luxembourg, 2021, ISBN 978-92-76-29139-8, DOI:10.2760/212807, JRC123439.
- Benchmark Mineral Intelligence, Lithium-ion battery supply chain technology development and investment opportunities, https://www.benchmarkminerals.com/wp-content/uploads/20200608-Vivas-Kumar-Carnegie-Mellon-Battery-Seminar-V1.pdf, 2020.
- X. Guo, J. Zhang and Q. Tian, Modeling the potential impact of future lithium recycling on lithium demand in China: A dynamic SFA approach, Renewable Sustainable Energy Rev., 2021, 137, 110461 CrossRef CAS
.
Footnotes |
† Electronic supplementary information (ESI) available. See DOI: 10.1039/d1ee01530c |
‡ Battery technologies have been categorized into lithium-ion (LIB), solid-state (SSB), lithium–sulfur (LSB) and lithium–air batteries (LAB). LIBs are further classified by the cathode technologies lithium nickel manganese cobalt oxide (NMC), lithium nickel cobalt aluminum oxide (NCA), lithium cobalt oxide (LCO), lithium manganese oxide (LMO), lithium nickel manganese oxide (LNMO), lithium iron phosphate (LFP), lithium iron manganese phosphate (LMFP), lithium cobalt phosphate (LCP), and by the anode technologies graphite (C), silicon composite (Si/C) and lithium titanate oxide (LTO). |
§ Cathode technology LMR-NMC: lithium and manganese-rich lithium nickel manganese cobalt oxide; HE-NMC: high-energy lithium nickel manganese cobalt oxide. Further details regarding cathode technologies are included in Section 4. |
|
This journal is © The Royal Society of Chemistry 2021 |