DOI:
10.1039/C7NR08360B
(Review Article)
Nanoscale, 2018,
10, 881-906
Technical challenges of working with extracellular vesicles
Received
9th November 2017
, Accepted 8th December 2017
First published on 13th December 2017
Abstract
Extracellular Vesicles (EVs) are gaining interest as central players in liquid biopsies, with potential applications in diagnosis, prognosis and therapeutic guidance in most pathological conditions. These nanosized particles transmit signals determined by their protein, lipid, nucleic acid and sugar content, and the unique molecular pattern of EVs dictates the type of signal to be transmitted to recipient cells. However, their small sizes and the limited quantities that can usually be obtained from patient-derived samples pose a number of challenges to their isolation, study and characterization. These challenges and some possible options to overcome them are discussed in this review.
1 Introduction
Extracellular Vesicles (EVs) are involved in the transmission of biological signals between populations of prokaryotic and eukaryotic cells. As central mediators of intercellular communication, EVs are involved in many cellular processes, such as compensating for stress conditions, triggering physiological responses that contribute to the maintenance of cellular integrity, organismal homeostasis1–3 and regulating a range of biological activities. Their potential applications for diagnosis and guiding therapeutics, as well as determining prognosis of pathological conditions has allowed the field of EV-studies to grow steadily in recent years.
The term EVs, coined by the International Society for Extracellular Vesicles (ISEV, http://www.isev.org), categorizes vesicles based on their biogenesis or release pathway, and include exosomes (50–100 nm in diameter), originated from the endocytic pathway and with buoyant densities of 1.11–1.19 g mL−1,4 shedding microparticles/microvesicles (100–1000 nm) released directly from the plasma membrane,5 apoptotic blebs (50 nm–2 μm; produced as a consequence of indiscriminate apoptotic disintegration),6 large oncosomes (1–10 μm)7 and other miscellaneous EV subsets.8 As the sizes of the different EVs-subsets overlap, as well as their cargo, several groups have now started characterizing the composition of EV-subtypes. Recent papers claim successful subclassification of EVs based on general surface proteomic profiling,9,10 or on transcriptional profiles of individual EV populations.11,12 EV subtypes have been isolated by a number of means, including recovery at different centrifugation forces, different filters, at slightly different positions in density gradients, via immuno-isolation by different surface molecules, chromatography or by flow cytometric sorting.
At present, functions of EVs have not been fully elucidated. However, they appear to be able to modulate host–pathogen interactions13 and to contribute to several pathological conditions such as infectious and inflammatory diseases, neurological disorders and cancer. EVs are particularly important in clinical settings, largely because they contain a wealth of biomarkers that can be used to monitor clinical status, chemotherapy-response,14 disease progression,15 and many relevant and diverse clinical conditions. The composition of EVs is not random and each EV-cargo delivers specific molecular messages. Indeed these nanosized membrane vesicles transmit signals by proteins, lipids, nucleic acids, and sugars, and the unique molecular pattern of this package dictates the type of extracellular signal to be transmitted to recipient cells.1 Despite the promise of revealing informative markers of medical interest, working with these small particles poses many technical challenges. In addition to the concerns over data analysis, there are further uncertainties over protocol standardisation and how to define the pre-analytical and analytical variables that impact upon outcome measures.16 The aim of this review is to outline the most relevant challenges related to working with EVs, specifically discussing the advantages and disadvantages of different approaches and suggesting alternative routes to better overcome frequent issues. A detailed scheme of EV purification and characterization methods is presented in Fig. 1.
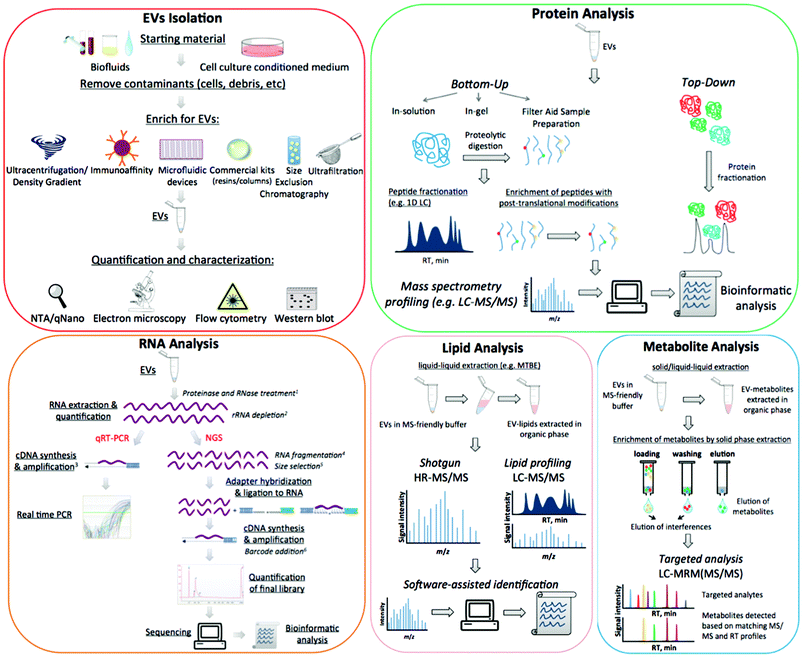 |
| Fig. 1 Scheme of EVs isolation, quantification and characterization, including the analysis of EVs molecular content. The superscript numbers on the figures indicate the need of each step as follows: 1 – to ensure intravesicular origin of RNAs; 2 – to remove abundant ribosomal RNAs that may be present as fragments in EVs, and therefore not visible by Bioanalyzer analysis; 3 – cDNA synthesis may be performed with transcript-specific or general primers; cDNA pre-amplification before real time PCR is optional; 4 – to break down long RNA molecules; 5 – to separate small RNAs (<200 nt) from long RNAs (>200 nt); 6 – to tag molecules from different samples and enable multiplex analysis. | |
2 Pre-analytical factors
2.1 EVs derived from biofluids
EVs have been isolated from diverse biofluids including blood,17 urine,18 saliva,19 breast milk,20 cerebrospinal21 and ascitic fluids,22 gastric juice,23 bile,24 sputum,25 bronchoalveolar lavage,26 semen27 and tears.28 The viscosity of these fluids, as well as their fat and protein content are highly variable, which may affect EVs purity and yield and, therefore require the protocols to be adjusted according to the biofluid of interest. Standardized pre-analytical steps are crucial to minimizing artefacts in EV-analysis, particularly when EVs are derived from complex body fluids such as blood. The amount, purity and content of biofluid-derived EVs may be affected by numerous factors including age, gender, ethnicity, body mass index, disease, use of medications, general lifestyle, and dietary habits.29 Ideally, these factors should be taken into account and normalized across all study subjects (patients and any relevant controls).
Other important considerations include methods of blood collection, as shear force may induce hemolysis, which has been shown to alter the expression of some plasma miRNA molecules30 and may also affect EV-derived content. Whereas EV counts appear to remain stable overtime in samples stored with no agitation, gentle agitation (used to simulate blood transportation) leads to an artificial and strong release of platelet-derived EVs.31 Additionally, anticoagulants have been shown to be capable of preventing the formation of EV-blood cell aggregates (aggregation was reduced in EDTA-preserved blood).32 Blood preserved with EDTA also appears to reduce the above-mentioned agitation effects on platelet-derived EVs if compared to citrate or heparin. Storage time is another important factor: Fendl et al. 2016 found comparable amounts of EVs in freshly drawn blood (collected in heparin, EDTA or citrate) but increased amounts of EVs were observed after 3 h storage, in an anticoagulant-dependent fashion, with increments varying from 2× (EDTA) to 10× (citrate), primarily due to platelet-derived EVs.31 Also critical is the careful separation of platelets from plasma with adequate centrifugations; the International Society on Thrombosis and Haemostasis recommends blood collection in citrated tubes and two successive centrifugations at 2500g for 15 minutes at room temperature.33 However, EDTA has been recently suggested as a valuable option in clinical settings.32
Another factor that needs to be considered when selecting EVs isolation protocols across different biofluids is the volume of starting material, as some biofluids may need to be concentrated prior to EVs isolation such as urine, an important source for patients diagnosed with renal pathologies, prostate and bladder cancers,18 and other conditions including the parasitic infection schistosomiasis.34 A recent study compared several filters and concluded that the best for recovering EVs from plasma, urine and EV-spiked PBS was a regenerated cellulose membrane with pores capable of retaining particles above 10 kDa.35 Liang et al., have also demonstrated the feasibility of concentrating the EVs using a double-filtration microfluidic device capable of isolating, concentrating and quantifying urinary EVs, using 8 mL of the pre-filtered (0.22 μm filter) and 20
000g centrifuged urine-supernatant collected from bladder cancer patients and controls.36 Using this approach the authors demonstrated that the principle of size-exclusion using two polycarbonate membranes (with pore sizes of 200 and 30 nm) permitted the concentration of EVs within this size-range and the later study of CD63 expression using ELISA. Urine-derived CD9-positive exosomes have also been isolated using magnetic beads37 and Gilani et al. have also shown that digital flow cytometry can be a good approach for quantifying the expression of markers of interest in urine-derived EVs from renal-injury patients.38
Besides biofluid-volume, specific methodological parameters need to be optimised during EVs isolation according to their inherent biochemical properties. For example, the pellet of EVs isolated from breast milk by 100
000g ultracentrifugation was found to be too solid to resuspend, because of the high abundance of whey and casein protein in milk.39 One proposed solution was to purify the 10
000g supernatant through an overnight sucrose density gradient, allowing separation of EVs from protein complexes.39
2.2 Cell culture conditioned medium
The use of cell culture conditioned medium enables a more controlled environment for EV isolation. Nonetheless, there are important aspects that may directly influence EV yields that should be considered. The choice between culture medium containing EV-depleted fetal bovine serum (FBS) versus culture medium with no FBS (serum starvation of cells) needs to be considered. This choice implies that an abrupt change to serum-free medium will likely cause a major stress to cells and lead to altered EV secretion.40 On the other hand, rigorous EV depletion of FBS needs to be thoroughly performed, using long ultracentrifugation protocols (16 h at ≥100
000g) in order to maximize the removal of FBS-derived EVs.41–43 Recent evidence, however, suggests that even this extended ultracentrifugation cannot remove contamination from bovine small RNAs, some of which can be mis-annotated as human RNAs.44 Apoptosis-induction control experiments can also be performed to exclude the possibility that EVs, and consequently the putative isolated EV-RNAs, are due to cell death,45 especially as many experiments report changes in RNAs upon EV induction as opposed to the steady-cell state. Other factors that need to be carefully considered include cell culture matrices and plastics, exact culture medium composition/volume, cell passage, cell confluency and viability, mycoplasma-status and other microbial contamination. If conditioned medium will be stored before EVs isolation, it is crucial to clear it of cells and cellular debris by centrifugation before freezing, and to minimize subsequent thaws.
2.3 Storage of EVs
Lőrincz et al. performed a detailed analysis of the effect of storage in different conditions (+20 °C, +4 °C, −20 °C, −80 °C for 1, 7 or 28 days) on the physical and functional properties of EVs derived from human neutrophilic granulocytes.46 Flow cytometry, dynamic light scattering and electron microscopy were used for measuring EVs physical properties (number, size and morphology), and an antibacterial assay was used to test the functional property of EVs. They found that storage (even at −20 or −80 °C) significantly altered the functional properties of EVs, although their number and morphology stayed constant. In another study, a 7-fold increase in the number of phosphatidylserine-exposing EVs was found in EVs isolated from plasma samples that had undergone a single freeze–thaw cycle, suggesting changes in the vesicle membrane phospholipids, whereas in urine-derived EVs the same effect was not observed.47 Kalra et al. spiked EVs isolated from a colorectal cancer cell line into plasma samples and measured TSG101 protein expression after 10, 30 and 90 days in storage at 4 °C, −20 °C or −80 °C, as well as PKH67 dye labeling and uptake by cells (−20 °C for 30 days).48 They found that all storage conditions yielded EVs with detectable TSG101 and capable of being transferred into cells.
Although several studies have investigated whether storage impacts specific characteristics of EVs, there is a lack of large-scale analysis of EVs cargo changes that may be caused by storage. To the best of our knowledge, no study has evaluated transcriptomic, proteomic and lipidomics alterations that may be induced upon EVs storage. Until more in-depth “omics” studies are performed, in addition to functional assays, the understanding of the true stability of EVs remains incomplete. In this sense, we recommend EVs to be evaluated as soon as possible after their isolation, especially for functional studies, avoiding repeated freezing and thawing cycles.
3 EV isolation protocols
There are several EV isolation and characterization techniques available, and each has its own set of pre-analytical factors that may influence yields. The ISEV has issued several position papers highlighting the importance of standardization of sample collection and EV isolation and characterization methods, which summarize all the detailed information that should be recorded and provided in publications.40,49,50
Launched recently, the EV-TRACK database (http://evtrack.org) is an excellent initiative in the EV-research field to encourage standardization of vesicle isolation and characterization methods.51 This initiative was created by an international consortium of 92 researchers from 12 countries, who evaluated and scored the experimental parameters of 1226 recently published EV-related articles. The EV-METRIC is a summary score of the article's adherence to and detailed reporting nine experimental parameters. The fact that the average EV-METRIC across all biofluid studies is only 20% indicates how the heterogeneous EV-field is currently deficient in methodological reporting. The online database is searchable, allowing easy identification and comparison of EV-related articles. Another interesting feature is that the authors can annotate their study's database entries, adding experimental details that may have been overlooked during publication. Hopefully, EV-TRACK will contribute to better transparency and reporting in EVs publications, allowing improved interpretation and reproducibility of experiments.
3.1 Ultracentrifugation (UC)
The selection of an EV-isolation method largely depends on the source of sample. For less complex samples obtained from cell cultures, it is beneficial to use differential UC with no preliminary steps, an approach that has long been considered the most efficient EV isolation technique. New isolation methods appear often, usually involving column enrichment or precipitation. However, the most popular primary isolation methods still in use are based on UC.52 On the other hand, isolation of EVs derived from complex biological fluids may benefit from the use of multiple methods to remove specific components (e.g. platelets, lipoproteins or protein complexes) before UC,53 or even alternative isolation methods, involving column enrichment, sequential filtering or precipitation. However, as a means of first EV-isolation step, UC is a cost-efficient and widely accepted way to isolate EVs.52
The isolation of EVs through differential UC relies on several centrifugation steps with increased centrifugal force to produce sequential pellets of cells and cell-debris (300–1000g), microvesicles (10
000–20
000g) and exosomes (100
000g). Some have described the use of serial ultrafiltration through 0.22 and 0.45 μm filters before pelleting54 and variation of the centrifugation forces have been implemented in different protocols.
Advantages of UC include low cost – as ultracentrifuges are readily available in many labs – and the capacity to spin a wide range of volumes from a few millilitres up to >100 mL. However, there are a number of problems associated with isolating EVs by UC. One of the major caveats is the co-purification of non EV-associated proteins, particularly protein aggregates and lipoproteins,55 which may be interpreted as integral or enriched EV components in downstream analyses. Ultrafiltration can be coupled with UC to reduce EV-protein aggregates.56 Attention should be given to centrifugation forces applied to EVs. Nordin et al., have shown some degree of EV disruption after UC for 70 min at 120
000g.56 Therefore, when the recovery of intact EVs using UC is an aim, we recommend the use of a maximum of 100
000g.
3.2 Density gradients (DG)
An approach that is commonly used to overcome the problem of co-purification is to follow UC with further purification of EVs in a sucrose DG which makes use of EV density for better separation (26). EVs of a particular size and origin have a particular floatation density, usually in the range of 1.08–1.22 g mL−1.57 Several protocols have been described,58 the majority of which involve resuspending an EV-enriched pellet following UC, overlaying a sucrose gradient in various buffers which may also contain deuterium oxide, performing UC again and collecting the appropriate fractions which are enriched in EVs. Differential UC shows the presence of larger vesicles by laser particle size measurement, and protein concentration studies show that DG UC yields higher protein concentration and more target exosome proteins.59
Unfortunately, differential UC can be a lengthy process with the most time taken for the UC itself. For clinical grade data or highly enriched samples it is recommended to use multiple centrifugation speeds that will considerably lengthen the process, reducing its applicability for clinical settings.60 Whereas sucrose gradient UC is relatively time consuming, it undoubtedly results in the isolation of purer EV samples than UC alone.59 However, due to fractionation a relatively high starting concentration of EVs is required.61 Besides being able to isolate purer EVs, it should be noted that contaminants that have the same density of EVs, such as some viruses,62 plasma-derived high- and low-density lipoproteins63,64 and uromodulin and albumin65 from urine, are likely to contaminate the EVs isolated by DGs. It has been shown that the similar floatation densities of HIV virus particles and EVs make them indistinguishable by conventional gradient separation.66 Presumably, the co-purification of EVs and virus particles could be considered a universal problem due to common viral contaminations of cell cultures which go undetected. Cantin et al. found that it was possible to separate HIV particles and EVs using commercially available OptiPrep DG solution.66 This employs a gradient of iodixanol rather than sucrose, and has been used for isolating fractions of pure EVs for proteomic analysis from conditioned cell culture medium67 and plasma.48
3.3 Immunoaffinity
An approach to improve the purity of the EVs population is to use immunoisolation, whereby EVs preparations are incubated with antibody-coated latex17 or magnetic beads,68 allowing their separation based on the expression of markers of interest. This technique has the potential to specifically pull-down EVs with a particular surface marker while excluding contaminating particles or other EVs populations. A study comparing immunoisolation with DG separation and UC for the isolation of human colon cancer-derived EVs showed that immunoisolation produced the highest EVs yield as measured by quantitative protein mass spectrometry.61
As immunoisolation is not compatible with large-volume samples, samples with low EV content must be concentrated prior to incubation with antibody-coated beads. Therefore, immunoisolation is commonly used as an additional purification step following conventional EVs isolation from large sample volumes using UC and possibly DG,17 making this a lengthy procedure. When small volumes of starting material contain sufficient EVs to isolate and analyse (as with serum), immunoisolation can be used to isolate EVs directly.69 Importantly, when isolating EVs from serum, it is essential to pre-coat the beads with a molecule such as sulfobetaine to reduce the non-specific adsorption of abundant serum proteins. This single-step EV isolation technique may prove to be a valuable diagnostic tool for identifying disease markers in EVs.69,70
While one of the key advantages of immunoisolation is its specificity based on the chosen antibody, this approach may also be limiting since it is likely to isolate merely a sub-population of EVs. There is still poor understanding of which EVs markers are present on different EVs subpopulations, and what the subcellular and cellular origins of these EVs are. For example, there are no accepted, exclusive markers to distinguish between exosomes and microvesicles despite their distinct mechanisms of biogenesis.71 In a diagnostic setting, the best case scenario would be to have a cell-type specific surface markers which are differentially regulated during disease and released in EVs as recently reported.68
While immunoisolation has been successfully used for analysis of EVs by mass spectrometry61 and RNA sequencing,72 the use of immunoisolated EVs in functional studies is more difficult due to the challenge of dissociating high yields of active EVs from the beads. Chaotropic agents such as NaCl have been successfully used to elute EVs from beads where EVs have been shown to retain at least some functionality.73 However, in spite of successful elution of EVs using mild conditions, small changes in EVs size and surface structure can occur.74,75 Thus, care must be taken when interpreting results from functional analyses using immunoisolated EVs.
3.4 Microfluidics
Microfluidics technologies can be used to isolate EVs populations of interest. Here, molecules enriched in the EVs membrane, such as specific lipids and proteins, can be used to isolate them.69 Many groups have already described the use of customised on-chip devices to isolate EVs, partly because this approach enables the use of low input sample. Another important point is the feasibility of EVs evaluation directly from bodily fluids (without the need for prior extraction steps), which can be of particular interest in the diagnostic setting.76 Combining microfluidics with previously described immunoaffinity has resulted in an immunoaffinity microfluidic device based on CD63, an abundant tetraspanin present in the EVs membrane.77 More recently, the Exochip, another on-chip device allows not only EVs isolation based on CD63 immunoaffinity, but also their quantification by fluorescent dye staining.78
EV features, such as electric properties, shape, size and density, can also be exploited in the development of customised chips.79 As a note of caution we must state that it remains to be demonstrated if antibody-isolation approaches somehow modify the cargo or the functionality of EVs and if their biological activity is dependent on the simultaneous signalling given by a more diverse EVs set, that can not be isolated by specific antibodies.
3.5 Size exclusion chromatography (SEC)
EVs with different sizes can be separated using Size Exclusion Chromatography (SEC), a technique that has been more and more used currently, allowing the recovery of pure vesicles with increased functionality, purity and integrity. Advantages of SEC include the reduction of EVs-aggregation during the isolation procedure,56 the efficient separation of EV from soluble proteins and capability of isolating pure, intact and biologically active EVs.80 SEC removes 99% of the soluble plasma proteins and >95% of HDL from the purest fraction of EVs, does not induce aggregation of EVs, and retains the integrity and biological activity of EVs.56,80–82 Contaminants such as von Willebrand factor and LDL are unexpected based on particle size, but these can still be found possibly complexed to EVs.63,83 SEC allows the EVs environment to be changed, such as from plasma to buffer, with no or minimal detrimental effects on EVs themselves. Also, it is fast, 10 to 20 minutes per sample, and relatively inexpensive,84 which makes it more applicable in a clinical setting. A disadvantage of SEC is the dilution of EVs sample, which often requires second step i.e. re-concentration of EVs by ultrafiltration.56,80,81,83–86 Compared with DC, EVs isolated by SEC have a high yield of biophysically intact EVs although at the expense of dilution.56,86 A single-step plasma EVs isolation using SEC has been published, based on the use of qEV SEC columns, an efficient system to isolate EVs from plasma proteins.60,84 Also, instead of pelleting EVs by UC, as used by some, the application of protein concentrating devices allows the fast concentration of EV fractions.60,84 This provides an efficient means of isolating and concentrating EVs from human plasma, while avoiding some negative issues related to UC as previously mentioned. SEC and ultrafiltration can be coupled to provide higher EVs purity and good recovery rates, while still preserving their biophysical and functional properties.56 Ultrafiltration of cultured media results in higher recovery of EVs after DG purification, and is a faster alternative to UC, whereas the SEC outperforms precipitation of EVs isolated from human plasma.60
3.6 Ultrafiltration (UF)
Filtration-based EVs isolation methods can be used independently or in conjunction with other techniques such as UC. Advantages of filtration-based methods are the ability to use variable sample input volumes, simplicity and low cost. Davies et al. developed a microfluidic filtration system for isolation of EVs directly from unprocessed whole blood55 which allowed the study of very limited amounts of blood from melanoma-bearing mice. After being isolated from a few microliters of blood by this pressure-based device, the EVs were shown to retain their intact morphology and to express Melan A mRNA, a melanoma tumor marker, as well as other classic EV-markers (as evidenced by Western blot of CD9, CD63 and CD81). Interestingly, a direct comparison with EVs isolated by UC was performed, and TEM analysis showed that UC caused many EVs to aggregate and to deform, which did not occur in the filtration-derived EVs, even under pressure.55 A three-step sequential filtration-based protocol allowed the isolation of EVs from larger volumes, such as 150 mL of cell culture conditioned medium.87 The authors conclude that sequential filtration with a 100 nm cut off final filtering step enriches for exosome-sized vesicles (81% compared to 23% for UC), suggesting that UC may lead to co-purification of larger vesicles and/or protein aggregates. UF is more appropriate with volumes in excess of 400 mL due to the higher flow rate, and that EVs loss is only observed with the first 50–100 mL of cultured media.60 Whereas it is far more time efficient than centrifugation methods, taking only 20 minutes to concentrate over 150 mL of sample compared to 2 rounds of UC for 90 minutes each.60 Importantly, UF can have a recovery of up to 80% and may concentrate EVs up to 240-fold.60 This implies that UF-based methods are effective to concentrate EVs. However, UF has some limitations such as it may result into non-specific binding of EVs to membranes35,56 and thus may present some loss of EV yield owing to trapping in filter pores.
3.7 General aspects of EVs isolation methods
Coincident with the EV-TRACK initiative, several groups have compared methodologies to improve the efficiency of the isolation and characterization of EVs. Even being considered gold standard for purification, differential centrifugation can provide technical difficulties to process large volumes of conditioned medium and it has been suggested that repeated UC steps can damage vesicles and reduce yield, impacting proteomic and RNA studies.56 In this sense, the group of Dr Andreas Moeller (QIMR Berghofer Medical Research Institute, Australia) compared UC to UF for the isolation of exosomes from cell culture conditioned medium and concluded that the later provided faster and better recovery.60 They then further compared four alternative methodologies (two precipitation-based methods: ExoQuick and Exo-spin; one SEC-based method: Izon qEV columns; and one DG purification-based method: Opti-Prep), concluding that precipitation-based methods led to higher particle yield but less purity (measured by particle to protein ratio), while qEV columns provided the purest preparations, including also plasma-derived exosomes.
A recent work has compared four exosome isolation protocols (single-step UC) and density-gradient UC-based protocols using iodixanol (Optiprep) and two commercially available precipitation-solution based protocols EXO-Quick (EQ) and total exosome isolation (TEI).88 The results showed Optiprep to be the method of choice in terms of removing EV-associated protein/RNA complexes contaminants, allowing the recovery of exosome-specific proteins and RNA.88 This was reinforced in a clinical study on plasma exosomes where Optiprep gradient centrifugation was the single method capable of removing contaminating plasma proteins.48 Regarding the quality of the RNA, Van Deun et al. found several differences of RNA profiles between the methods, and strongly recommend to validate isolation methods prior to consider exosome-specific content, functions and biomarkers.
Moreover the group of Dr Pieter Vader (University Medical Center Utrecht, The Netherlands) and Samir El Andaloussi (Karolinska Institutet, Stockholm, Sweden), provided evidence that SEC-isolated EVs better retain their functional activities, as compared to UC-isolated EVs.89 They investigated differences in functionality of cardiomyocyte progenitor cell derived EVs isolated using UC and SEC, concluding that SEC-isolated EVs were more efficient in stimulating the migration of endothelial cells, having EV-induced ERK1/2 phosphorylation as a measurement to compare the UC-EVs and SEC-EVs functionality.
Reinforcing the importance of UF the group of Dr An Hendrix (Ghent University, Belgium) compared five commonly used filters for their efficiency to recover exosomes from clinical samples.35 Regenerated cellulose membrane filters, with pores of 10 kDa, showed higher efficiency. They also compared colorimetric and fluorimetric kits to measure EV protein content, and found the Qubit fluorometric assay to be the most sensitive (considering the lower BSA standard of 200 μg mL−1) and the more consistent in terms of quantification among technical and biological replicates. This group also suggested the Optiprep (Sigma Aldrich) to be the best method to isolate EVs and proposed a protocol to remove Optiprep leftovers from EVs. Other efforts have been done by Dr Marca Wauben's group (Utrecht University, The Netherlands) who developed a fluorescence-based flow cytometric high-throughput method for quantitative and qualitative analysis of nanosized cell-derived membrane vesicles (50–100 nm) which cannot be visualized by conventional scatter-based analysis.90,91
In order to ensure how pure are the EVs isolated by any of the possible protocols, the ratio of EVs and non-EV components can be assessed. One of the most used methods is the determination of the number of EVs per microgram of measured proteins in the isolated EVs. EVs purified using sucrose DG from conditioned cell culture media provided highly purified EVs: 3.3 × 1010 particles per μg protein. However, when isolated from biofluids EVs are much more contaminated by proteins giving ratios around 6.5 × 109 particles per μg protein for fresh serum and 1.1 × 109 particles per μg protein for fresh urine.92 This calculation should be interpreted with caution as the ratio depends on the source of the vesicles as well as the method of extraction.88
4 Characterization of the isolated EVs
After isolation, EV populations need to be characterized for intended downstream applications, for which there is a variety of techniques available. This section will discuss some of the available options in light of the instrumental parameters of each technique.
4.1 Electron microscopy (EM)
The use of electron microscopy (EM) has considerably pushed the field of EVs and enabled the discovery of apoptotic vesicles, microvesicles and exosomes. The nanometer-scale resolving power of transmission electron microscopes has, however, its drawbacks, which include lengthy sample preparation, lack of multi-parametric phenotyping, and low throughput capacity. These characteristics make large EM studies rather challenging, with the amount of starting EVs material being particularly critical. Yet, morphological characterization using parameters such lipid and protein compositions, cellular origin, size, density and morphology4 is a key step for classification of isolated EVs. Thus, efforts to mitigate the challenges in the use of electron microscopy to study EVs are most welcome in the field.
One of these common challenges relates to the amount of available material for transmission EM preparation, microtomy and visualisation. Some tissues and body fluids release an abundant amount of vesicles that are easily harvested by differential centrifugation or affinity purification chromatography. However, most often than not, isolated EVs are few and far between; and sample preparation itself can at times cause further loss of material. In an attempt to overcome this issue, recently there have been informal discussions, in conferences and focused meetings, about the use of proteinaceous material (e.g. matrigel, bovine serum albumin, or other inert protein) to ‘encapsulate’ EVs in a rich protein surrounding that is extensively cross-linked during fixation. This is because the protein around EVs, when cross-linked together, will form a firm matrix around the vesicles, protecting them from falling apart. Alternatively, the use of inert polysaccharides (e.g. agarose, agar, methylcellulose) to embed isolated EVs in a small piece of gel prior to EM processing has also been reported by individual laboratories with success.
A routine EM method that has been used with great success for the study of EVs is that of negatively-stained whole mount preparation.4 Here EVs are adsorbed onto a filmed metal grid, chemically fixed and negatively stained prior to observation using a transmission electron microscope. Whole mounts are extremely useful for morphological analysis (e.g. EV size, shape, density), and can be combined with immunolabelling techniques. This method is particularly useful for the identification and localisation of immunological epitopes on the external surface of EVs, whereas EV-internal epitopes are better visualised by positive staining methods.
Nevertheless, the visualisation of EVs by transmission electron microscopy, coupled or not with immunolocalisation techniques, suffers mostly from two major challenges: lack of contrast and preservation (of vesicle morphology and/or molecular epitopes). This is particularly critical when considering the potential of EVs as biomarkers in medical applications. Some whole-mount preparations yield cup-shaped exosomes, which has been suggested to be an artefact caused by sample dehydration.93 Indeed, the preparation steps of chemical fixation, dehydration, observation under vacuum, and electron beam radiation damage could all interfere with an important feature of exosomes, that of size.94,95
Cryo-electron microscopy (cryo-EM) comes at hand here, and hydrated exosomes studied by cryo-EM have been reported as close-to-spherical nanoparticles.96,97 As fixation tends to be physical (cryogenic) rather than chemical, cryo-EM is thought to preserve EVs closer to their native state,98 in particular in terms of their morphology and immunological epitopes used in the identification of vesicle biomarkers. An interesting feature described in cryo-EM of exosomes from prion-infected cells was the presence of a second, internal membrane-bound region of denser core and, in many cases, of hexagonal shape.97 Vesicles derived from human plasma and studied by the same method also show a variety of forms and sizes, suggesting multiple membrane profiles in EVs. Elongated vesicles, empty or not, were also observed, and so were EVs surrounded by smaller spherical structures.99 Yet, because most cryo-EM procedures do not include the use of heavy metals, contrast of the material could be compromised if not for various optical and diffraction techniques – the work of colleagues such as Alain Brisson from the Institut de Chimie & Biologie des Membranes & des Nano-objets (CBMN, Bordeaux, France) in the development of suitable imaging analysis methods, and of diagnostic assays using EVs, holds much promise here.
4.2 Nanoparticle tracking analysis (NTA)
NTA is a light scattering technique that makes use of both laser light scattering and the Brownian motion of particles to obtain the particle size distribution and concentration. This approach takes into consideration the particles’ speed in a medium of known temperature and viscosity and allows the calculation of its hydrodynamic diameter. The first studies of EVs using NTA were published in 2011.100 NTA is currently the most popular quantitative method of EV analysis.52 This is because few other high-throughput techniques have the appropriate resolution for single EV particle analysis. Whilst NTA allows relatively high throughput, and claims to have resolution down to small diameters, its ability to size and determine concentration accurately is however debatable.101 Moreover, when performing NTA measurements, before dilution of samples, attention should be given towards the lack of particulate matter in suspension buffers (usually phosphate buffered saline) to avoid artificial inflation of EV counts. The addition of a detergent such a Triton X to NTA preparations allows for confirmation of EV-lipid moieties. Its fluorescent mode is also capable of providing specific results for labelled particles. In a recent application of fluorescent NTA, it was shown the use of miRNA-specific molecular beacons encapsulated in cationic lipoplex nanoparticles that fuse non-specifically with negatively charged EVs and this allowed the precise quantification of EVs carrying an specific miRNA and how many copies of this miRNA could be found in these EVs.102 This paves the way to future therapeutic applications that rely in the identification/quantification of specific markers.
4.3 Resistive pulse sensing (RPS)
RPS utilises the Coulter principle to determine the absolute diameter and distribution of particles in a suspension in the range of ∼50–10
000 nm.101,103 RPS utilised in the EV field is generally carried out using the qNano (Izon Science Ltd, Christchurch, New Zealand). The qNano consists of two fluid cells separated by a non-conductive membrane. An electric current is passed through a single pore in the membrane and, as particles pass through this pore, a transient attenuation of signal occurs that is approximately proportional to the particle volume. This system is calibrated using beads of a known diameter and concentration.104 Sample volumes in the qNano can be as low as 10 μL. By applying pressure differences between the fluidic cells, pressure-driven flow overcomes the flow produced by diffusion, electrophoresis and electro-osmosis. Disadvantages of this technique include: multiple pore sizes are required to measure the full EV size range; pores are prone to clogging; little phenotypic information regarding the EV's derivation is gained; identifying EVs from similar sized contaminants is not possible.
4.4 Flow cytometry (FCM)
Although mostly used for cellular analysis, FCM is currently one of the most popular techniques used to study EVs, in particular microvesicles.105 Flow cytometers that have been developed for dedicated EV analysis, referred to as dedicated FCM (dFCM), have been shown to be capable of resolving particles consistent with biological vesicles to <30 nm. dFCM are usually conventional flow cytometers that have been customised by a laboratory, although more recently they have also become commercially available.106–108 A persistent concern in FCM is the ability to reliably distinguish between EVs that carry a specific protein marker from those that do not, and thus to accurately measure the proportion of EVs of a certain type. Quantitative and qualitative analysis of EVs populations present within a sample are needed. Although being a pre-requisite for a correct data interpretation, the use of proper controls that robustly validate the test samples and establish background levels are generally missing.109 Fluorescently-stained polystyrene microspheres have been used as a standardisation method for EV analysis, yet their high refractive index compared to EVs means that they cannot reliably be used to directly approximate the size of EVs using FCM scatter parameters. Furthermore, due to FCM collecting light at different angles, scatter resolution cannot be compared using polystyrene microspheres without the use of refractive index normalisation using laser scatter physics modelling.110 Fluorescent sensitivity can be quantified using microspheres that have a known quantity of molecules of equivalent soluble fluorophore (MESF) that are recommended for standardising EV analysis. Reviews dedicated to EV standardisation using FCM can be found elsewhere.105
5 Characterization of EV content
5.1 Characterization of EVs content: RNA
5.1.1 RNA purification.
Several sources of bias due to differences in experimental methodologies may explain discrepancies between results of EV-studies, including EV-RNA related analysis. The two main RNA purification approaches are silica membrane column-based kits and/or organic extraction and precipitation-based kits. One of the earliest studies that systemically compared methods evaluated seven different extraction protocols and concluded that there were major differences in yield, purity and size distribution of the RNAs extracted by the kits.111 Nowadays there is a plethora of commercial kits available from many different companies, specifically tailored for EV isolation and subsequent RNA extraction. Each method has its own peculiarities and attention must be paid when comparing results from different studies, taking into account the EVs and RNA isolation strategies employed. Another important source of bias relates to the possible co-purification of non-vesicular nucleic acids bound to the external surface of EVs. To avoid this and isolate only intra-vesicular RNAs protected by the lipid membrane, ISEV recommends before RNA extraction performing a proteinase treatment first to eliminate protein complexes that may also protect RNAs from enzymatic degradation, followed by RNAse treatment.112 However, residual RNase activity may also damage RNA upon extraction of the luminal contents. Consequently careful consideration of the aim of the study should be first taken into account prior to RNase treatments.
The major drawback of working with EV-derived RNA, especially in the case of those isolated from biofluids, is the poor RNA yield, which is often below the detection limit of current quantification techniques such as fluorimetry (Qubit) and capillary electrophoresis (Bioanalyzer). One possible way of overcoming this limitation is normalizing all samples by the initial biofluid input volume used for extraction, and vacuum-concentrating all the extracted RNA for downstream analysis. Suggestions to improve EV-RNA yield include diluting biological fluid prior to extraction113 and also the observation that more starting material is not necessarily advantageous due to potential over-loading of sample in column based extraction methods.114 Despite the fact that more EVs are present in serum, plasma is more commonly studied due to the absence of EVs released by platelets during the clotting response.115
5.1.2 Microarray analysis.
Microarray technology enabled the seminal discovery in 2007/2008 of RNAs contained in vesicles derived from mast cell lines116 and glioblastoma primary cells as well as patient serum samples.117 These studies used gene expression arrays and first identified thousands of transcripts in EVs, including miRNAs and mRNAs.116,117 The mRNA molecules were shown to be functional, leading to protein production in the recipient cells upon transfer of the EV-cargo.117
5.1.3 Quantitative reverse transcriptase-PCR (qRT-PCR) and PCR arrays.
Similarly, several studies used quantitative real time PCR-based arrays to profile the RNA content of EVs.118–121 This is by far the most widely used method to evaluate and quantify the miRNA content of EVs, due to the low requirements of starting RNA (as little as 1 ng total RNA, using a pre-amplification protocol), the ability to evaluate hundreds of miRNAs of interest and the straightforward analysis of the data that requires simple calculations and no bioinformatics. As most studies that evaluate the RNA-content of EVs aim to identify biomarkers, qRT-PCR is a good, low-cost and reliable tool. The major drawback of this approach is the need defining a priori the list of possible markers, as their evaluation depends on the probes/primers available in the selected platform.
During the qRT-PCR procedure per se, one of the most important factors is the amount of starting molecules. When direct quantification of EVs cannot be performed, the normalization of the input to volume of sample input is recommended.122 Moreover, in an attempt to enhance the amount of amplifiable molecules, pre-amplification methods can be used,123 with no apparent bias. Despite the qRT-PCR approach used (specific probes or non-specific DNA dyes), results can be strongly biased according to the selected normalization method. A major issue is the absence of known endogenous controls to be used for qRT-PCR. In this sense, the Sample and Assay Standards Working Group of the Extracellular RNA Communication Consortium (ERCC), recently suggested the use of spike-in controls to properly normalize the RNA populations43 an approach that has been successfully used.124
5.1.4 Next generation sequencing (NGS).
Recently, the RNA content of EVs from diverse sources has been characterised by next generation sequencing (NGS), allowing a more comprehensive analysis of the EV-RNA repertoire.125–128 The predominating view is that EVs are enriched in small non-coding RNAs, such as miRNAs, tRNAs and miscellaneous structural RNAs such as Y-RNAs, vault RNAs and SRP-RNAs.129 This may be due to the fact that the majority of studies have focused on the small RNA fraction of the transcriptome, by performing either a gel- or bead-based size selection step that limits the analysis to small RNAs (<200 nucleotides).129–132 In this way, researchers have bypassed the issue of sequencing abundant rRNAs, but have consequently missed out on the identification of mRNAs and long RNAs that offer great potential as biomarkers. One study that performed whole transcriptome analysis of EVs derived from breast cancer cell lines found 97% of reads were derived from rRNA and argued that EVs are enriched in rRNA molecules that are fragmented and therefore do not appear as peaks on Bioanalyzer analysis.133 Likewise, in another study with EVs derived from epidermoid and hepatocellular carcinoma cell lines, the authors found 92% rRNA, but as they chose not to perform any size selection step they only analysed RNAs greater than 50 nt, which therefore precluded the identification of miRNA molecules and some other small RNAs.134 In another recent study with EVs isolated from human pleural effusion and plasma, mRNA amplification was performed using oligo dT primers, restricting the analysis to the poly-adenylated fraction of transcriptome.135 An unbiased characterization of the complete vesicular transcriptome is currently lacking, mainly due to methodological constraints that require different library construction protocols for small or long RNA analysis wherein size selections limit the analysis to specific transcript fractions. The limited RNA yield that is obtainable from EVs, particularly those derived from biofluids, oftentimes prohibits the construction of more than one library per sample, not to mention the high costs involved. The depletion of rRNA before sequencing has not been possible also due to limited RNA yield, as the majority of commercial kits require at least 100 ng of RNA input, or has been attempted but was not successful to the fact that fragmented rRNA hinders probe-based capture.133
Crescitelli et al. compared the RNA profiles of apoptotic bodies, microvesicles and exosomes isolated by UC from the conditioned medium of three different cell lines.11 However, authors only undertook a Bioanalyzer analysis, which limits the results to RNA size profiles. This could be misleading as typical 28S and 18S rRNA peaks will not be visible if the rRNAs are present as fragments inside EVs, which has been reported by NGS data.133,134 Nonetheless, Crescitelli et al. found that rRNAs are more prevalent in apoptotic bodies, and increases after TRAIL-induced apoptosis. This observation may perhaps explain the variability of rRNA content of EVs reported in the literature, and highlights the importance of reporting cell viability in studies, when applicable. The variability in quantities of rRNA reported is discussed by Mateescu et al. in an ISEV position paper, and they mention that ribosomes present in extracellular fluids may become associated with EVs during centrifugation and co-isolate at high g-force.136 They conclude that the only way to effectively prove if a particular RNA species is truly inside EVs is by RNAse protection assays after proteinase treatment. Therefore, it is our view that the best description of the whole EV-transcriptome would require the depletion of apoptotic bodies by several steps of lower-speed centrifugations followed by pelleting of EVs at higher g-forces and RNase digestion of intact EVs.
Despite the above-mentioned technical hurdles, NGS is the most comprehensive tool for the analysis of transcriptome, as it allows the unbiased view of all molecule types in a sample and permits not only quantification, but also the identification of mutations, and structural variations such as alternative splicing isoforms, circular-RNAs and translocations.128 However, the limitations of this method include its relatively high-cost, the requirement of large amounts of starting RNA (usually above 100 ng), and the dynamic bioinformatics pipelines to evaluate the results.
5.1.5 Digital droplet PCR (ddPCR).
Digital droplet PCR (ddPCR) is a relatively new technology that allows the absolute quantification of gene expression. By partioning PCR amplifications into thousands of fractions, this methodology allows very sensitive detection of RNA expression levels and DNA variants without the use of standard curves. Recently ddPCR has been applied to the study of plasma-derived exosomal RNA from prostate cancer patients137 and EVs derived from serum and CSF of glioma patients.138 ddPCR is also very useful to validate NGS findings, even more so in the case of EV transcriptome analysis, as standard qRT-PCR depends on normalization to the expression of levels of endogenous controls, and in the case of vesicle-derived RNAs such controls are not ubiquitous.
5.1.6 Bioinformatics analysis of the EV-transcriptome.
After generating the sequences by NGS, the computational analysis is straightforward with no relevant deviations from routine bioinformatics protocols. Normalised count-based differential expression analysis is performed over the RNA sequencing data, after removing artifacts and low quality reads usually using the FastQC139 tool, which is able to report information related to the GC content, the presence of adaptors, over represented k-mers and duplicated reads, PCR artifacts or contaminations. Reads derived from fragments of ribosomal RNAs are usually removed in this step as well. Next, one key aspect is to deal with distinct read-length distribution. To overcome this issue, the alignment step could be performed by using one or the combination of the mapping tools, such as Burrows–Wheeler Aligner (BWA),140 Bowtie2141 or STAR.142 After the alignment is carried out, the mapped reads are used to estimate gene and transcript expression and, the simplest approach is to quantify raw counts of mapped reads using programs such as FeatureCounts143 or HTSeq-count.144 To facilitate transparent comparison of transcript levels both within and between samples it is of utmost importance to use metrics that attempt to normalize for sequencing depth and gene length. The fragments per kilobase of exon per million reads mapped (FPKM)145 and RNASeq by Expectation Maximization (RSEM) software146 are the most used ways to normalize the count data from RNA-seq platforms. The analysis of differentially expressed genes (DEG) follows the above-mentioned steps and the popular methods for DEG are DESeq147 and EdegR148 DGE data can be visualized as MAPplots (log ratio × abundance), Volcano plots (fold change × statistical significance), heatmaps and scatterplots. Further analysis steps include the functional profiling in which DEG are involved. Nevertheless, the characterization of the molecular function requires the availability of functional annotation data for the transcriptome under study. Gene Set Enrichment Analysis (GSEA) is one the most common knowledge-based methods for interpreting genome-wide expression profiles.149 Many of the tasks shown in this section are embedded in the Bioconductor150 a flexible software-engineering environment that enable genome-scale analysis of high-throughput data.
5.2 Characterisation of EVs content: protein
The protein cargo of EVs is cell- and disease-type dependent, and confers particular features to these vesicles influencing their biological properties.71,151,152 Protein components of EVs that are derived from different cell types and biofluids have been widely described using Western blotting, proteomic technologies and fluorescent-activated cell-sorting.153–155
5.2.1 Western blotting.
Western blotting for the so-called EV markers is often used as a confirmation of EVs presence in samples of interest.96,156 Commonly used markers include CD63, Alix, Tsg101 and HSP70.157,158 A common mistake in the literature is the attempt to classify isolated EVs according to the presence of certain markers, in particular the presence of markers such as CD63, to annotate exosomes. There are two major problems with this approach: firstly, most EV isolation methods will isolate a mixed population of exosomes and microvesicles, and secondly, there are no widely accepted, reliable markers to distinguish between these two populations.71 In addition to the fact that there are further sub-populations of EVs within these two subsets. Indeed, Kowal et al. found that CD63 is present in EVs, which are larger than exosomes and that the common exosome markers HSP70, flotillin-1 and actin are present in all EVs sizes.158 Furthermore, they found that other markers, including Tsg101, were present in only a subset of exosome-sized EVs, suggesting that these are not suitable as generic ‘exosome’ markers. One limitation of this study, however, was that it focused only on dendritic cell EVs, and it is impossible to draw conclusions about universal EV markers without more extensive studies. Indeed, Yoshioka et al. found that there was variation in the presence of EV markers such as CD63, flotillin-1 and HSP70 even within different prostate cell lines157 while CD9 and CD81 were uniformly present. The variability among different prostate cell lines may reflect prostate-cancer heterogeneity and it is well known that cancerous cell lines can secrete EVs with different cargo from their non-cancerous counterparts,68,159 yet many commonly used cell lines are cancer cell lines and information taken from these cells taken to infer universal EV-markers will probably be misleading. More research is needed to identify reliable EV markers. However, it remains to be seen whether universal EV markers in fact exist and could be used across all mammalian cells and, furthermore, whether markers will be found to reliably distinguish exosome and microvesicle populations. Showing the relative enrichment of several marker proteins in isolated EVs compared to their corresponding whole cells is probably a good way of confirming the presence of EVs in the absence of better tools.
Another point to note is that most of the common EV markers are also abundant or detectable in whole cells157 so confirming their presence in isolated EVs is not necessarily a good method of demonstrating sample purity. It is therefore essential to also show negative control Western blots96,156 for proteins such as calnexin or histones which can be confidently expected not to be secreted in EVs; showing the absence of abundant whole cell proteins is an important step towards proving that isolated EVs are not contaminated by cell debris.
A more general problem is that all the research discussed above fails to address EV markers in non-mammalian cells, which may have an entirely different set of EV markers even if mammalian markers are present in the genome. EVs are studied from bacteria,160 to algae,161 helminths162 and protozoa163 and have important implications in basic biology. An alternate, unbiased way of showing protein loading and distinct protein composition from whole cells without the need for specific markers is to use protein staining instead of Western blotting, such as silver or SYPRO Ruby staining.164
5.2.2 Proteomics.
Undeniably, mass spectrometry based proteomic analysis has increased our knowledge about the protein content of EVs. In particular, bottom up mass spectrometry-based proteomics has been used worldwide as the strategy of choice. In this approach proteins are extracted from a biological source, digested into peptides that are subsequently separated by 1D or 2D gel electrophoresis (gel-based) or liquid chromatography (gel-free) and analysed by mass spectrometry. Peptide ions are fragmented in the gas phase and their sequence and post-translational modifications (PTMs) can be deduced. Protein quantification can be achieved using different strategies depending on the study aims.165 In particular, shotgun proteomics approach allows a discovery-driven protein identification and quantification where peptide ions are measured and heuristically selected for fragmentation using a data-dependent mode.166 In targeted proteomics, only predetermined peptide ions are selected for fragmentation allowing a hypothesis-driven protein detection and quantification. Several acquisition methods have been implemented for targeted proteomics such as selected reaction monitoring (SRM)167 pseudo selected reaction monitoring (pSRM)168 and parallel reaction monitoring (PRM).169,170 Another approach is the data-independent acquisition in which no precursor ion selection occurs and all precursors are fragmented.171–173 The resulting MS/MS spectra are commonly searched using spectral libraries171 or novel computational frameworks.174 These proteomics strategies have been used in several EVs characterization studies and excellent reviews on EVs isolation and proteomics have been published.175–177 As such, we will focus on the use of mass spectrometry-based protein analysis and proteomics technologies to better understand the more specific differences in the EV proteome such as post-translational modification (phosphorylation and glycosylation).
5.2.3 Post-translational modifications in EVs: a path to explore.
Mass spectrometry analysis allows quantitative protein profile of EVs in different conditions. Still, there are additional layers of protein regulation, such as PTMs that modulate protein structure and function changing its physicochemical characteristics, interaction partners and activity.178
Protein glycosylation is one of most widespread PTMs, which involves the enzymatic attachment of a glycan moiety to a protein, occurring mostly in the ER and Golgi. Indeed, protein glycosylation has been shown to play an important role in cancer, diabetes, neurodegenerative and cardiovascular diseases.179 Several glycan binding proteins were identified in EVs such as CD62, found in isolates from activated platelet5 and several members of the galectin family.180,181
Protein phosphorylation is a very dynamic PTM catalysed by kinases and reversed by phosphatases that regulates several signalling events both extra- and intracellularly. Even though most reports have applied immunodetection techniques to study protein phosphorylation in EVs, Gonzales et al. have described a large-scale phosphoproteomic approach to exosomes isolated from urine that allowed the identification of 14 phosphoproteins, such as AQP2, which was confirmed by immunoblotting.182
Since regulation of cell function is achieved through an intricate network of PTMs, it would be desirable to study several PTMs simultaneously. Although mass spectrometry-based proteomics has allowed the comprehensive identification of PTMs and their crosstalk,183–185 bioinformatics analysis and functional interpretation of multiple PTMs remains a challenge. However, we believe that increasing comprehensive multiple PTMs studies will help shed some light in EVs PTMs cross-talk and burst the discovery of novel signalling mechanisms.
5.3 Characterization of EVs content: lipids
Structurally and functionally, lipids represent an extremely heterogeneous group of molecules, defined by various permutations of head-groups and fatty acid chains, which together define lipid structure, head-group polarity, and hydrophobicity. Due to often limiting amounts of sample available and its high complexity, mass spectrometry-based approaches offer high sensitivity and specificity that is essential for the analysis of EV-lipidome. Modern mass spectrometers offer high resolution and high mass accuracy of survey scan, thereby providing the required accurate mass measurement of the lipid molecular weight. However, partial or complete overlap in the elemental compositions between lipids of different classes imposes a great challenge for their confident identification.186
Lipid content can be analysed from EV pellets reconstituted in aqueous environment after their UC, or from highly concentrated EVs size exclusion chromatography fractions. Typically, the lipid amount used for analysis is expressed relative to protein quantity, and may vary from 100 μg protein equivalent or more.187,188 EV lipids are most commonly extracted using Bligh and Dyer189 and Folch190 liquid–liquid extraction, where lipids are enriched in the lower organic layer of chloroform/methanol/water phase. However, both methods carry a risk of contamination by protein from the upper aqueous phase during the collection of organic phase, which may have deleterious effects to the downstream nLC/LC-MS/MS analysis. Additionally, due to the hydrophobic nature of chloroform, both methods may discriminate extraction of partially polar lipids and additionally challenge their detection. Recently methyl-tert-butyl-ether (MTBE) extraction of lipids was demonstrated to extract almost all lipid classes form highly complex biological samples with equivalent or better recoveries than the “gold standard” Folch extraction.191 To date, lipidomic profiling of EVs derived from different cells,187,192 species193 and biological tissues194,195 have been published, together with recent reviews on lipidomics of EVs.176 Since EVs are cell-derived particles, their lipid composition is related to its parent cell. Eventhough, a direct comparison of the lipid profile between exosomes and plasma membrane of the releasing cells allowed the identification of specific lipid classes that appear to be enriched in the exosomal fraction. Membranes of typical EVs are mainly enriched in fully saturated glycerophospatidylcholines, glycerophospatidylethanolamines and glycerophospatidylserines, that are responsible for membrane rigidity and integrity, and sphingomyelin, cholesterol lipids and ganglioside GM3.196 A study characterized more than 280 lipid species from metastatic prostate cancer cell-line derived exosomes. Exosomes were enriched in glycosphingolipids, sphingomyelin, cholesterol, and phosphatidylserine indicating a particular lipid sorting in the exosome membrane.197 Although lipid content of EVs attracts increasing attention, not much is known about their biological activity. Sphingomyelin is reported to mediate antigenic activity of tumour-derived EVs both in vivo and in vitro.74 Lysophosphatidylcholines in EVs derived from mature dendritic cells appear to act via G-protein coupled receptors and trigger lymphocyte chemotaxis.198
5.4 Characterization of EVs content: metabolites
During EV formation, small metabolites such as sugars, amino acids, nucleotides, different enzymatic cofactors, lipid metabolites and other redox regulatory molecules can be packed into EVs. Sub-nM concentrations of metabolites and low sample amounts impose a great challenge for the metabolomics of EVs, which may be one of the reasons why this type of analysis is only sporadically reported.199–201 Metabolites comprise a broad array of small molecular weight analytes, derived as intermediates and products of enzymatically-driven cellular processes. Due to their smaller size, metabolites are characterized by their lower hydrophobicity compared to lipids. Thus, they are usually enriched via extraction from vesicle pellets with methanol or methanol/water. However, co-extraction of highly abundant lipids may suppress ionization of metabolites of interest and greatly challenge their detection even with the most sensitive targeted mass spectrometric detection. Thus, metabolites of interest are further enriched using solid phase extraction (SPE) cartridges. The choice of SPE chemistry depends solely on the analyte chemical nature and eluting condition. The most common conditions include loading of sample in 10% aqueous methanol (pH 2), hexane washing of hydrophobic lipids, and elution of metabolites in methanolic ethylacetate or butylaceta.199,201 Eluates are further vacuum dried and can be stored at −20 °C, or analysed immediately by mass spectrometry.
Although information of the metabolome of EVs is limited, all published studies to date support the significance of EVs as carriers of important metabolome fingerprints which can be used for defining specific changes in cellular homeostasis. Increasing evidence suggests that, during defined stages of the acute inflammatory process, neutrophil-derived EVs carry specific enzymatically-derived polyunsaturated fatty acid (PUFA) metabolites such as 4-, 7-, 14- and 17-hydroxy-docosahexaenoic acid, 5-, 12-, 15-hydroxy-arachidonic acid, 12-, 15- and 18-hydroxy-eicosapentaenoic acid and others.199,201 These metabolites are of particular importance because they serve as direct precursors of anti-inflammatory and pro-resolving lipid mediators,202 such as resolvins, maresins, protectins and lipoxins. Prostaglandin (PG) rich vesicles trigger PG-dependent intracellular pathways in the host cells.203 Thus, depending on the metabolome cargo, EVs in target cells can induce formation of pro-inflammatory, or anti-inflammatory and pro-resolving lipid mediators, and thereby influence inflammatory outcomes and tissue homeostasis.
Metabolomics is an emerging technique with focus on identification and quantification of a wide variety of small molecules which may be indicative of physiological status in health and disease. Metabolites are produced in vivo, usually via enzymatically-driven processes; thus their circulating levels are very low, and often out of the reach even for the most sensitive detection techniques. Thus, analysis of EV-metabolome relies on a careful and well-designed sample enrichment, and sensitive MS-detection. Recently some authors200 for the first time described methodology for the LC-MS/MS profiling of blood plasma EVs, and EVs derived from cultured cells. Coenzyme Q10, ubiquinone 9,25-hydroxy-hexadehydrovitamin D3, malonyl-CoA, deoxyvitamin D3 and others, are only some of identified metabolites that belong to the wide range of metabolite classes. However, MS-profiling often does not offer the sensitivity necessary for detection of very low abundance metabolites. Targeted mass spectrometry (e.g. multiple reaction monitoring, MRM) provides necessary sensitivity and specificity, however it discriminates detection and identification of those metabolites that are not “targeted” by designed acquisition methods, and cannot be used for wide-band metabolome profiling.204
5.4.1 Bioinformatic tools used in lipidomics and metabolomics.
Comprehensive analysis of large lipidomics and metabolomics data-sets is a challenging and time consuming task. Although significant improvement in the field has been made over the past few years, identification of lipids and metabolites is still not as straightforward as protein identification.205 The LIPID MAPS (http://www.lipidmaps.org) is a free of charge online platform for the analysis of MS data based on their accurate mass measurement.206 Recently, LIPID MAPS have implemented a search tool that uses precursor or product ion lists and matches them with thousands of different lipids with in silico-generated structures or those present in the database. The Human Metabolome Database (http://www.hmdb.ca) is a freely available database that accounts for 42
003 metabolites found in the human body and supports analysis of MS, MS/MS and GC-MS data uploaded in a form of peak lists. LipidXplorer-assisted analysis of large shotgun lipidomics data sets is used routinely for lipid identification, independently of any resource of reference mass spectra, and solely based on assumed molecular fragmentation pathways.207 The software and MFQL library are free for download (https://wiki.mpi-cbg.de/lipidx/Main_Page) and can be used regardless of the mass spectrometer type. It is important to note that the mass resolution and accuracy influence strongly unequivocal lipid identification. Lipid View (ABSciex) and Progenesis QI for small molecules (Waters) are commercially available platforms. Both softwares can use MS and MS/MS that they match against publicly available and in-house databases.
Although many different MS platforms for lipid analyses are available nowadays, analysis of EV-bound metabolites and lipids remains a significant challenge. Very low amount of sample still imposes the greatest challenge for EVs wide-band profiling. To date, both analyses have already advanced our knowledge on biomolecular composition of EVs and helped us better define their structure-to-function relationship. Nevertheless, further advances in lipidomics and metabolomics are necessary to allow application of MS for the analysis of EVs derived from various different sources and biological tissues. The main technical pitfalls of working with EVs and potential ways to avoid them are summarized in Table 1.
Table 1 Potential technical pitfalls, recommendations and remaining challenges according to different techniques of EVs isolation and characterization
|
Sample type/technique |
Potential pitfalls |
Recommendations |
Remaining challenges |
Pre-analytical factors |
Cell culture conditioned medium |
Presence of contaminating bovine vesicles and proteins from FBS leading to misinterpretation of EV quantification/characterisation |
The use of EV-depleted FBS is recommended208 |
Much needed standardisation of protocols by the EV-community; current lack of knowledge regarding serum starvation stress over optimal duration of EVs production, concentration and content |
Use of EV-depleted FBS209 or serum starvation210 may stress cells leading to alterations in EVs concentration and content |
In the case of complete serum removal, cells should be assessed for stress induction that could alter EVs characteristics. Autophagy, apoptosis and necrosis should be monitored |
Blood |
Artificial inflation of EVs counts ex vivo as a result of platelet activation due to blood collection, blood transportation and excessive delay in plasma separation |
Follow guidelines such as those from the International Society on Thrombosis and Haemostasis, including citrated tubes for blood collection, and adequate separation of platelet-free plasma using two rounds of centrifugations immediately after blood collection. Abundant proteins (such as albumin and immunoglobulins) should be depleted |
Difficulty applying rigorous blood collection and plasma separation/storage in a large-scale clinical setting |
Changes in EVs counts/cargo observed are not related to particular disease/phenotype being investigated, but are rather due to other clinical and environmental factors that influence EVs counts such as age, gender, circadian-clock, medications, fasting state, hormonal status, presence of infection, etc.; abundant proteins may hamper the identification of bona fide EV protein cargo |
Compare with a matched control group without disease and try to normalize as much as possible the differences between individuals within a group, controlling for all factors cited |
Unmet need for technological advances that allow disease-specific EVs to be isolated, quantified and characterized separately from all other normal-cell-derived EVs |
Urine |
Membrane-bound proteins could be affected by proteases in the urine; EVs may remain attached to the collection tubes |
Urine should be collected in tubes containing protease-inhibitors; containers should be vortexed to avoid EVs being adhered to tube wall; abundant proteins (such as uromodulin) should be depleted |
Handling of large volume samples and the need to concentrate urine for the adequate isolation of EVs challenges its use in a clinical setting |
EV isolation protocols |
Ultracentrifugation (UC) |
Variability in sedimentation efficiencies, no absolute separation of EVs subpopulations. High centrifugal forces may lead to EVs aggregation and disruption of larger EVs; lower centrifugal forces reduce recovery of smaller EVs |
Low-angle fixed-angle rotors provide better separation efficiency211 and reducing biofluid viscosity prior to centrifugation improves recovery;113,212 protocols need to be adjusted according to the targeted EV-subtypes |
There is a lack of methods to allow the precise assessment of EV damage and the recovery of the full range of EVs |
Co-sedimentation of protein, lipoprotein and RNA aggregates as well as viral particles |
Combine ultracentrifugation with density gradients to further purify samples66 |
Density gradients (DG) |
Sample fractionation leads to EVs being divided into numerous sub-samples |
Ensure higher initial EV counts due to substantial dilution of EVs among the sub-samples |
Ultracentrifugation steps as well as the preparation of the gradient are time consuming; the process is laborious and difficult to be implemented in a clinical setting |
Immunoaffinity |
Concentrated EV samples are required in small volumes |
Spin columns, precipitation and other methods can be used to reduce sample volume |
Clearer definition of EV subpopulations and their characteristic markers. Less aggressive dissociation of EVs from beads to avoid affecting functionality. Minimizing antibody contamination and buffers’ interference |
Markers must be pre-selected by the user |
Characterise markers specific to EVs in the system of interest, it has however been demonstrated that markers vary between EVs of interest213 |
Saturation of beads with abundant, non-EV proteins found in biofluids. Elution of EVs from beads might damage the structure of vesicles. Captured vesicles might not retain functionality after elution. Eluted antibodies or antibody remnants and harsh elution buffers might interfere with downstream analysis |
Coat beads to minimise non-specific adsorption of abundant proteins from serum samples214 |
Commercial kits |
Contaminating non-vesicular molecules (such as protein, protein aggregates, lipids and free nucleic acids) may occur in all isolation methods, but may be more predominant here due to precipitation process |
Evaluate the more prevalent non-vesicular contaminants of the sample under investigation and test kit to establish optimal protocol for experimental aim |
The unknown biochemical basis of many kits restricts our ability to fully understand EV populations extracted and the non-vesicular contaminants |
Potential interference by elution buffers in downstream applications |
Include controls in functional tests checking the effects of kit elution buffers on cells receiving EVs |
Size exclusion chromatography (SEC) |
Inability to concentrate diluted EV sample, which, often requires second step i.e. re-concentration of EVs by ultrafiltration56,80,81,83–86 |
To rapidly concentrate EV fractions the application of protein concentrating devices is recommended |
Concentrating devices need to be standardized in order to allow results to be comparable across studies |
Ultrafiltration (UF) |
Non-specific binding of EVs to column membranes leading to reduced yield owing to trapping in filter pores35,56 |
Ultrafiltration coupled with SEC is recommended for enhanced EV purity, preserving their properties56,60 |
Forcing vesicles through filters might damage larger EVs whereas the use of less force, through gravity or by using less pressure makes the process longer and less adequate in some cases40 |
Characterisation of isolated EVs |
Electron microscopy (EM) |
Dehydration and fixation of EVs during processing may disrupt membranes leading to artefacts |
Use cryo-EM in combination with immunogold labelling, where samples are not dehydrated215 |
Development of new methods both quantifying and characterising EVs. No currently available EM approaches for routine analysis of EVs in a clinical scenario |
Inability to readily discern heterogeneous EV populations |
Use of alternative super-resolution microscopy techniques i.e. PALM216 or STORM217 |
Nanoparticle tracking analysis (NTA)/resistive pulse sensing (RPS) |
Resuspension buffers may contain particles misinterpreted as vesicles |
Include controls to ensure that EV suspension buffers analysed in order to establish a control/background |
Polystyrene bead refractive index not identical to that of EVs. Current issues in discerning clustered from non-clustered vesicles, especially in samples of higher EV-concentration (NTA). Establishment of lower detection limit for RPS |
Accuracy in reporting size and concentration is debatable |
EVs or their contents can be GFP labelled to ensure proper analysis.102 Bead calibration should be used218 |
Flow cytometry (FCM) |
Distinction between single particle positive and negative EV samples |
Use reciprocal dilution to ensure single particle detection or use multicolour and multiplex approaches to resolve individual vesicles219 |
High refractive index of commonly used reference beads compared to EVs |
Scatter resolution |
Use scatter beads of known size and refractive index. Use fluorescence beads with known molecules of equivalent soluble fluorophore (MESF) units220 |
Characterisation of EV contents |
RNA purification |
Low RNA yields |
Normalize all samples by the initial biofluid input volume used for extraction, and vacuum-concentrate extracted RNA for downstream analysis |
Difficulty in eliminating residual RNase that would reduce the available luminal EVs RNA |
DNA contamination in EV RNA samples |
DNase treatment of RNA samples should be performed prior to downstream analysis |
Non-vesicular RNA complexed with proteins may be bound to the outside of the EV membrane |
Use proteinase followed by RNase treatment of intact vesicles to eliminate protein complexes protecting non-vesicular extracellular RNAs from enzymatic degradation221 |
RNA analysis by qRT-PCR |
Low RNA yield, especially from clinical samples, may hamper the quantification and analysis of less abundant RNA populations |
Pre-amplification can enhance the amount of amplifiable molecules with no apparent bias.123 Use spike-in controls to properly normalize the RNA populations.124 If possible, use multiple endogenous controls based on EV-transcriptomic data, or use absolute quantification (by digital PCR, for example) for validation |
The technique is intrinsically limited to a very specific set of pre-selected RNAs, not allowing a broader view of the EV-transcriptome |
RNA analysis by NGS |
Library preparation issues: adapter dimers, ligation and size-selection bias |
Ribosomal RNA (rRNA) depletion may be unsuitable due to low starting input of RNA and presence of fragmented rRNA. Extensive fragmentation of RNA may be used to generate total transcriptome library and analyse small and long RNAs simultaneously128 |
Fragmentation of rRNA species hinders efficient rRNA removal.133 Excessive RNA fragmentation can lead to difficulties in properly mapping the short reads. Comprehensive EV-transcriptional databases are lacking, especially from NGS data derived from clinical samples |
Reduce adapter dimers using specific kits50 or use fragmented RNA to minimize artefactual adapter dimer formation and preclude size selection128 |
Protein analysis |
Co-purification of protein, protein aggregates and lipoprotein contaminants |
Remove abundant proteins such as immunoglobulins by combining ultracentrifugation and protein G agarose bead incubation,222 especially when working with EVs derived from biofluids |
The depletion of contaminant proteins requires a significant mass which is not always available from clinical samples. Proteomics methods need to be developed to accommodate the analysis of reduced mass of protein |
Heterogeneity of protein markers on different EV populations, for example presence of CD63, HSP70, flotillin-1, and actin present in both exosomes and microvesicles |
Use a range of markers or non-marker-specific methods to distinguish differences between cells and EVs such as silver staining or SYPRO Ruby staining164 |
Identification of protein markers capable of differentiating specific EVs subtypes |
Contamination of samples with cellular components |
Non-EVs proteins, such as calnexin, GM130, cytochrome C and histones, should be used as negative controls to ensure purity of isolation158 |
Protein degradation during vesicle manipulation |
Use of protease and phosphatase inhibitors is highly recommended during EV protein extraction |
Protease treatment needs to be standardized according to the biofluid-origin of the EVs under study |
Lipid analysis |
Contamination by proteins during extraction |
Liquid–liquid extraction is preferred method for EV lipid analysis.189,190 Use of internal standards is recommended to ensure high mass accuracy and confident identification. If possible use LC to ensure separation of lipids prior to sensitive MS/MS. MTBE extraction was shown to extract all lipid classes with better recovery than the Folch method191 |
In liquid–liquid extraction protein contamination from the aqueous phase and bias towards extraction of partially polar lipids may occur. Public databases of lipids are still far from complete |
Difficulty in extracting and representing all lipid classes |
Metabolome analysis |
Low metabolite yields and reproducibility (LC/MS) |
Enrich sample using solid phase extraction prior to MS.199,201 Major lipid and protein species should be removed in this process to ensure sensitive MS analysis |
Development of better bioinformatic analysis pipelines for metabolites. Public databases of metabolites are still far from complete |
Cell culture metabolite contamination |
Use of a cell-free medium control223 |
6
In vivo studies of EV-function
The ability to discriminate vesicle populations of interest from the general EV pool in tissue compartments and bodily fluids remains technically challenging. The cellular origins of EVs, their route of administration, their concentration and their mode of targeting significantly impacts on EV bio-distribution.224 However, non-traditional routes of EV administration, such as orally administered EVs have shown uptake via the intestinal mucosal surface and subsequent accumulation in the liver and spleen.225 Irrespective of administration route, a key outcome measure is biodistribution, to trace where exogenously applied EVs go after they have been introduced into an animal.
Fluorescent labelling of cellular proteins have revolutionized in vivo studies of cells. However, similar protein marker tracing of EV through EV-associated proteins like CD63-GFP (green fluorescent protein) have been shown to be an effective detection approach. A fraction of EVs is CD63-positive and further work is required to establish the cellular processing of CD63 on EV populations. Numerous protein markers (CD63, TSG101, Alix and heat shock proteins) are widely reported as associated to EVs but their overall contribution to the total EV population remains contentious. Therefore, utilising these proteins as imaging or labelling moieties requires caution. The nanometer-size scale of EVs presents challenges in studies that require spatiotemporal resolution. In addition, exosomes may be as small as 50 nm in size and tagging them with a 5 nm GFP molecule may significantly affect both their biodistribution, kinetics and their functional effects.
Secondary labelling of isolated EV using lipophilic dyes (DiR (1,1-dioctadecyl-3,3,3,3-tetramethylindotricarbocyanine iodide, D12731, Invitrogen, Life Technologies, PKH26/67 Sigma-Aldrich or Dil Stain (1,1′-dioctadecyl-3,3,3′,3′-tetramethylindocarbocyanine perchlorate (‘DiI’; DiIC18(3))) Thermo)) have shown distribution to the spleen, liver, lungs, pancreas, kidneys and gastrointestinal tract. These lipophilic methods are limited in scope and require the harvest of tissues of interest for analysis, or the use of highly sensitive cameras. Lipophilic dyes may persist in organs and tissues after EV degradation or may associate with other lipids. Injected liposomes that model the EV-population are rapidly cleared from the circulation by the spleen and liver <1 hour after injection, suggesting differences for EV/liposomes clearance based on lipid profiles;226 whereas Gaussia luciferase combined with metabolic biotinylation greatly enhances the spatiotemporal resolution of EV tracing in vivo.227
Whilst labelling and re-applying EVs in vivo enables the investigation of distribution, there is still a paucity of knowledge regarding the downstream effects of these EVs, or even whether the numbers injected are physiologically relevant. Indeed, the rapid generation time of both species, combined with an easily manipulated genome, makes them ideal for studying both the role of EVs in development, as well as the mechanistic underpinnings of their release. It is even possible to study the effect of EVs on stereotypical behaviours in invertebrates; for example Wang et al., 2014228 have shown that ciliary EVs are responsible for the tail-chasing behaviour seen in some worm species.
However, detailed investigations utilising vertebrates are needed to further test the biodistribution of EVs and ascertain the optimal route of administration for target organs or tissues. Rodents, which are traditionally used in preclinical research, provide rapid breeding cycle, complete genome sequence and some physiological similarities to humans. Two key biodistribution studies in rodents were carried out by Xandra Breakefield's and Samir El Andaloussi's group, both showing that by using vertebrate species and specific labelling techniques, it is possible to demonstrate that distribution of EVs is determined by cell source and route of administration.224,227
Tracing experiments to observe EVs biodistribution are important but further emphasis must be placed on the role of EVs in physiological systems. EVs cargo such as miRNAs have shown regulation of physiological barriers such as the blood brain barrier229 to promote tissue invasion and progression230 and descriptions detailing the physiological relevance of EVs at sites of accumulation or deposition are needed.
Functional studies are much more varied and outcome measures are group specific and will largely depend on the scientific question at hand. Despite this, an extremely broad range of disciplines, from basic molecular neuroscience, to complex integrated cardiovascular physiology, are now beginning to study the role of EVs in their model systems.
7 General recommendations for working with EVs
The following general recommendations are made regarding pre-analytical factors, EVs-isolation methods as well as the characterization of EVs and of their content.
7.1 Pre-analytical factors
For human samples, variables such as gender, age, use of medications, circadian-clock, fasting, etc., should be controlled.231,232 For blood-derived EVs, attention should be given to reduce platelet activation and the release of platelet-EVs. In this sense, excessive shaking of collected blood should be avoided and plasma is preferred over serum. Anti-coagulant should be chosen based on the capability to reduce the release of platelet EVs and impact on downstream applications, helping to preserve RNA and/or vesicle integrity for in vitro or in vivo studies: citrated tubes have been suggested for most applications.33 Viscous fluids such as plasma and saliva should be diluted in PBS to improve EV recovery. EVs-depleted FBS, obtained by overnight UC (100
000g), should be preferentially used for cell culture medium.41–43 Non-conditioned medium should always be used as a negative control. For urine samples, it is recommended that protease inhibitors are present in the collection containers to preserve the sample, in addition to extensive vortexing, required to recover the highest amount of vesicles that could remain attached to the tubes during storage.233 If not processed immediately, urine samples should be stored at −80 °C to avoid bacterial contamination. Pathological conditions that affect glomeruli filtration and lead to elevated urine protein loss, together with the high levels of uromodulin, can magnify issues of protein contamination and protein aggregates that make downstream EV-studies challenging. To reduce uromodulin and protein aggregates that interfere with downstream EV-studies we suggest the use of CHAPS (3-[(3-cholamidopropyl)dimethylammonio]-1-propanesulfonic), a mild detergent that preserves protein conformation and enzymatic activity,234 allowing the functional studies of urinary EVs. As a general rule, the characterization of the molecular content of EVs isolated from biofluids will always benefit from depletion of high abundant non-EV proteins. Albumin can be removed from plasma by the use of the Protein Organic Solvent Precipitation (PROSPR) protocol,235 immunoglobulins in CSF can be depleted by protein G agarose beads followed by UC.222
7.2 EV-isolation
The need of robust, standardized and reproducible EVs-isolation methods is an essential requirement. As the EV isolation method employed directly impacts the results obtained, all samples of a study should be processed by the same method. UC, the classic approach for EV-isolation, is not suitable for clinical applications and may disrupt EVs when high g-forces (>100
000g) are used.56 DG is a laborious and time-consuming approach that does not supply high yields but usually provides purer EVs, especially when iodixanol-based gradients are used. In general, we recommend the use of DG when highly purified EVs are required, e.g. for characterization of EVs subtypes, or to determine their nucleic acid or protein content. UF is a good approach to concentrate EVs and it is much faster than UC, but has not been broadly evaluated. For immunocapture we recommend the use of magnetic beads or microfluidics, to improve the interactions between EVs and the capture antibodies. However, it is not currently known if the antibody-binding would affect the functionality or the cargo of the captured EVs or whether it would introduce bias to subpopulations of EVs and non-EVs contaminants. Alternative methods such as chromatography, flow field-flow fractionation, polymer or solvent-based precipitation, immunoaffinity capture and microfluidics are useful methods to avoid the co-isolation of lipoparticles and lipid droplets from biofluids. After isolation the purity of the EVs should be assessed. As a reference, the isolation of EVs using sucrose density ultracentrifugation provided about 3.3 × 1010 EVs per μg protein from conditioned culture media (prostate, breast, bladder and mesothelioma cells) and about 2 × 109 EVs per μg from biofluids (urine and serum from healthy donors).92 To conclude, the selection of the method of choice strongly depends on the type of EVs-source and the downstream analyses and applications.
7.3 EV characterization
NTA is a valuable method for determining the concentration and size distribution profiles of EVs. Due to its non-specific nature, special care should be taken to ensure the particles measured are indeed EVs. We recommend the buffer in which the EV sample are suspended to be analysed separately, to establish a background count. In addition, the use of a lipid dye or a fluorescently labelled EV marker in combination with the NTA fluorescence mode will reduce the impact of non-EV particles. Similarly, efforts should be taken to reduce the contribution of non-EV contaminants when analyzing particles by RPS; this could include measurement of the background particle count present in the EV buffer. For RPS filtration and SEC can prevent clogging and multiple counts are needed to reduce the impact of variability. Furthermore, the size distribution of EVs observed using either whole-mount Electron microscopy EM, NTA or RPS should be compared to check that they yielded similar results. For flow cytometry protocols should be optimised and these parameters checked with routine calibration, as well as the use of robust negative controls, used to minimize the impact of false-positive signals arising from antibodies/protein aggregates. EM is of great help to characterize the EVs and negatively stained whole mount EM is a relatively quick and simple technique that greatly helps evaluating the presence, morphology and size distribution of EVs. Further imaging by cryo-EM is more technically challenging but the ability of this technique to resolve lipid bilayers makes it the most direct way to directly confirm the presence of bona fide membrane-bound vesicles.
7.4 Characterization of EV content
We perceive the major challenge in establishing EV content to be related to the limited amounts of EVs that can be recovered from biofluids. The precise description of the transcriptome of EVs, a mixture of few copies of a complex transcriptome combined with high copies of fragmented ribosomal RNA, is challenging. In order to uncover the total transcriptome of EVs, non-EV RNAs must be removed by RNAse treatment. Following, we recommend the use of Next Generation Sequencing of pre-fragmented EVs-derived RNA that allows the identification and quantification of all RNA biotypes.128 If the aim is to simply describe the EV miRNAs a comprehensive qRT-PCR arrays can be used, but these are limited to a set of pre-defined molecules.
The observation of classic EV-markers in Western blots is used to reinforce that bona fide EVs have been isolated. However, due to the limited knowledge regarding consistent EV-specific proteins, there is no single protein or combination of proteins that can be recommended as universal EVs markers. Nevertheless, we recommend the evaluation and reporting of at least three classic EV-proteins, as their presence or absence may be informative to the EVs characterisation. We also suggest evaluating proteins that are not expected to be present in EVs, as controls of contamination, such as proteins from the endoplasmic reticulum (e.g.: calnexin), Golgi (e.g.: GM130), mitochondria (e.g.: cytochrome C), and nucleus (e.g.: histones).49 The addition of a protease in the presence or absence of a membrane-disrupting detergent should help to establish whether a protein is present inside the EVs, and therefore protected by the EV membrane, or a secreted factor or a co-extracted protein. Finally, the presence or absence of particular proteins should be regarded as supporting evidence, rather than interpreted to mean that the sample definitely contains EVs or a particular EV sub-population. Large-scale shotgun proteomics is the method of choice to characterize more broadly the EVs-content. The application of native mass spectrometry at the protein level characterization and cross-linking, H/D exchange and covalent labelling at the peptide level characterization allows understanding the exosomal protein–protein interaction and structural modulation in different conditions.
LDL and HDL can be co-extracted from plasma by some EV isolation protocols and, due to their content of cholesterol and its fatty acid esters, as well as phospholipids, this can lead to misleading lipid profiles, including suppressing the identification of less abundant EVs lipids. Therefore, before performing the lipidomic profiling of plasma/serum-derived EVs, we recommend the use of size exclusion chromatography for isolation of EVs distinctly from HDL/LDL particles, which might also be helpful to reduce the contamination with other circulating lipids in biological fluids. The chemical diversity of lipids and metabolites compounds requires different extraction methods to be applied for lipids and metabolites. Recently, the promising SIMPLEX protocol was used for the simultaneous isolation of proteins, lipids and metabolites reducing the sample amount needed for a comprehensive biomolecular characterization.236 The combination of different front-end separation techniques such as GC and HPLC using a variety of stationary phases with high resolution and accuracy mass spectrometers will improve the number of identified and quantified lipids and metabolites in EVs.
8 Towards deciphering “EVome” complexity: future directions
EVs are central elements in cell–cell communication and play a key role in the field of liquid biopsies. These two aspects makes their study extremely promising, and future directions include improvements in characterizing their cargo to better define their roles in tissue homeostasis and to better identify the biomarkers that they carry. The full determination of the RNA-content of vesicles will benefit from the fast growing field of genomics, especially from the technologies that handle minute amounts of nucleic acids, including single-cell genomics and barcodes to identify individual molecules. Regarding proteomics, the amount of protein mass has deeply decreased allowing the identification of thousands of proteins from low abundant samples, such as FACS-sorted cells237,238 and fine-needle aspiration biopsies.239 Straightforward sample preparation methods and novel mass spectrometers can improve the sensitivity and quality of protein quantitation.240 Nonetheless, functional validation of EV proteins is needed, linking proteomic information and functional studies and providing greater overview of EV function. Moreover, combining information on multiple omics will help in understanding the complex biological network of EVs.175,241–243
The field of EVs has gained increasing interest and knowledge is rapidly accumulating. This has helped this area to move from bench top science towards translational and clinical research, including in vivo experiments as well as diagnosis. Nevertheless, our perception is that the field is not yet ready for clinical applications. Therefore, we believe it is too early to state that we can confidently interpret EV-data or administer/manipulate EVs for clinical purposes.
Conflicts of interest
There are no conflicts to declare.
Acknowledgements
The authors acknowledge the support received from the British Council/Newton Fund (203855854), Fundação de Amparo à Pesquisa do Estado de São Paulo (2012/24574-3; 2015/50257-3; 2014/26897-0; 2014/06863-3), Associação Beneficente Alzira Denise Hertzog Silva (ABADHS), Conselho Nacional de Desenvolvimento Científico e Tecnológico (441878/2014-8; 202077/2015-2), Brazil; Ricerca Finalizzata (Convenzione no. 172/GR-2011-02350301) from the Ministero della Salute, Italy; Science without Borders/CAPES, Brazil (BEX 7057/15-6). MIR and ED-N are research fellows from CNPq, Brazil.
References
- M. Yáñez-Mó, P. R.-M. Siljander, Z. Andreu, A. B. Zavec, F. E. Borràs, E. I. Buzas, K. Buzas, E. Casal, F. Cappello, J. Carvalho, E. Colás, A. Cordeiro-da Silva, S. Fais, J. M. Falcon-Perez, I. M. Ghobrial, B. Giebel, M. Gimona, M. Graner, I. Gursel, M. Gursel, N. H. H. Heegaard, A. Hendrix, P. Kierulf, K. Kokubun, M. Kosanovic, V. Kralj-Iglic, E.-M. Krämer-Albers, S. Laitinen, C. Lässer, T. Lener, E. Ligeti, A. Linē, G. Lipps, A. Llorente, J. Lötvall, M. Manček-Keber, A. Marcilla, M. Mittelbrunn, I. Nazarenko, E. N. M. Nolte-'t Hoen, T. A. Nyman, L. O'Driscoll, M. Olivan, C. Oliveira, É. Pállinger, H. A. Del Portillo, J. Reventós, M. Rigau, E. Rohde, M. Sammar, F. Sánchez-Madrid, N. Santarém, K. Schallmoser, M. S. Ostenfeld, W. Stoorvogel, R. Stukelj, S. G. Van der Grein, M. H. Vasconcelos, M. H. M. Wauben and O. De Wever, J. Extracell. Vesicles, 2015, 4, 27066 CrossRef PubMed.
- G. Desdín-Micó and M. Mittelbrunn, Cell Adhes. Migr., 2017, 11, 127–134 CrossRef PubMed.
- T. Takeuchi, M. Suzuki, N. Fujikake, H. A. Popiel, H. Kikuchi, S. Futaki, K. Wada and Y. Nagai, Proc. Natl. Acad. Sci. U. S. A., 2015, 112, E2497–E2506 CrossRef CAS PubMed.
-
C. Théry, S. Amigorena, G. Raposo and A. Clayton, Curr Protoc cell Biol, 2006, ch. 3, pp. 3.22.1–3.22.29 Search PubMed.
- H. F. Heijnen, A. E. Schiel, R. Fijnheer, H. J. Geuze and J. J. Sixma, Blood, 1999, 94, 3791–3799 CAS.
- G. K. Atkin-Smith, R. Tixeira, S. Paone, S. Mathivanan, C. Collins, M. Liem, K. J. Goodall, K. S. Ravichandran, M. D. Hulett and I. K. H. Poon, Nat. Commun., 2015, 6, 7439 CrossRef PubMed.
- D. Di Vizio, J. Kim, M. H. Hager, M. Morello, W. Yang, C. J. Lafargue, L. D. True, M. A. Rubin, R. M. Adam, R. Beroukhim, F. Demichelis and M. R. Freeman, Cancer Res., 2009, 69, 5601–5609 CrossRef CAS PubMed.
- M. Nawaz, G. Camussi, H. Valadi, I. Nazarenko, K. Ekström, X. Wang, S. Principe, N. Shah, N. M. Ashraf, F. Fatima, L. Neder and T. Kislinger, Nat. Rev. Urol., 2014, 11, 688–701 CrossRef PubMed.
- J. Kowal, G. Arras, M. Colombo, M. Jouve, J. P. Morath, B. Primdal-Bengtson, F. Dingli, D. Loew, M. Tkach and C. Théry, Proc. Natl. Acad. Sci. U. S. A., 2016, 113, E968–E977 CrossRef CAS PubMed.
- G. Kibria, E. K. Ramos, K. E. Lee, S. Bedoyan, S. Huang, R. Samaeekia, J. J. Athman, C. V. Harding, J. Lötvall, L. Harris, C. L. Thompson and H. Liu, Sci. Rep., 2016, 6, 36502 CrossRef CAS PubMed.
- R. Crescitelli, C. Lässer, T. G. Szabo, A. Kittel, M. Eldh, I. Dianzani, E. I. Buzás and J. Lötvall, J. Extracell. Vesicles, 2013, 2, 1–10 Search PubMed.
- T. R. Lunavat, L. Cheng, D.-K. Kim, J. Bhadury, S. C. Jang, C. Lässer, R. A. Sharples, M. D. López, J. Nilsson, Y. S. Gho, A. F. Hill and J. Lötvall, RNA Biol., 2015, 12, 810–823 CrossRef PubMed.
- J. S. Schorey and S. Bhatnagar, Traffic, 2008, 9, 871–881 CrossRef CAS PubMed.
- S. Yu, H. Cao, B. Shen and J. Feng, Oncotarget, 2015, 6, 37151–37168 Search PubMed.
- A. Hoshino, B. Costa-Silva, T.-L. Shen, G. Rodrigues, A. Hashimoto, M. Tesic Mark, H. Molina, S. Kohsaka, A. Di Giannatale, S. Ceder, S. Singh, C. Williams, N. Soplop, K. Uryu, L. Pharmer, T. King, L. Bojmar, A. E. Davies, Y. Ararso, T. Zhang, H. Zhang, J. Hernandez, J. M. Weiss, V. D. Dumont-Cole, K. Kramer, L. H. Wexler, A. Narendran, G. K. Schwartz, J. H. Healey, P. Sandstrom, K. J. Labori, E. H. Kure, P. M. Grandgenett, M. A. Hollingsworth, M. de Sousa, S. Kaur, M. Jain, K. Mallya, S. K. Batra, W. R. Jarnagin, M. S. Brady, O. Fodstad, V. Muller, K. Pantel, A. J. Minn, M. J. Bissell, B. A. Garcia, Y. Kang, V. K. Rajasekhar, C. M. Ghajar, I. Matei, H. Peinado, J. Bromberg and D. Lyden, Nature, 2015, 527, 329–335 CrossRef CAS PubMed.
- E. M. Mora, S. Álvarez-Cubela and E. Oltra, Int. J. Mol. Sci., 2016, 17, 13 CrossRef PubMed.
- M.-P. Caby, D. Lankar, C. Vincendeau-Scherrer, G. Raposo and C. Bonnerot, Int. Immunol., 2005, 17, 879–887 CrossRef CAS PubMed.
- O. E. Bryzgunova, M. M. Zaripov, T. E. Skvortsova, E. A. Lekchnov, A. E. Grigor'eva, I. A. Zaporozhchenko, E. S. Morozkin, E. I. Ryabchikova, Y. B. Yurchenko, V. E. Voitsitskiy and P. P. Laktionov, PLoS One, 2016, 11, 1–17 Search PubMed.
- Y. Ogawa, M. Kanai-Azuma, Y. Akimoto, H. Kawakami and R. Yanoshita, Biol. Pharm. Bull., 2008, 31, 1059–1062 CAS.
- C. Lässer, V. S. Alikhani, K. Ekström, M. Eldh, P. T. Paredes, A. Bossios, M. Sjöstrand, S. Gabrielsson, J. Lötvall and H. Valadi, J. Transl. Med., 2011, 9, 9 CrossRef PubMed.
- J. C. Akers, V. Ramakrishnan, R. Kim, S. Phillips, V. Kaimal, Y. Mao, W. Hua, I. Yang, C.-C. Fu, J. Nolan, I. Nakano, Y. Yang, M. Beaulieu, B. S. Carter and C. C. Chen, J. Neuro-Oncol., 2015, 123, 205–216 CrossRef CAS PubMed.
- R. Cappellesso, A. Tinazzi, T. Giurici, F. Simonato, V. Guzzardo, L. Ventura, M. Crescenzi, S. Chiarelli and A. Fassina, Cancer Cytopathol., 2014, 122, 685–693 CrossRef CAS PubMed.
- H.-I. Choi, J.-P. Choi, J. Seo, B. J. Kim, M. Rho, J. K. Han and J. G. Kim, Exp. Mol. Med., 2017, 49, e330 CrossRef CAS PubMed.
- L. Li, K. B. Piontek, V. Kumbhari, M. Ishida and F. M. Selaru, J. Visualized Exp., 2016, 7–9 CAS.
- C. Porro, S. Lepore, T. Trotta, S. Castellani, L. Ratclif, A. Battaglino, S. Di Gioia, M. C. Martínez, M. Conese and A. B. Maffione, Respir. Res., 2010, 11, 94 CrossRef PubMed.
- C. J. E. Wahlund, A. Eklund, J. Grunewald and S. Gabrielsson, Front. Cell Dev. Biol., 2017, 5, 39 CrossRef PubMed.
- M. N. Madison, J. L. Welch and C. M. Okeoma, Bio-Protoc., 2017, 7, e2216 Search PubMed.
- A. E. Grigor'eva, S. N. Tamkovich, A. V. Eremina, A. E. Tupikin, M. R. Kabilov, V. V. Chernykh, V. V. Vlassov, P. P. Laktionov and E. I. Ryabchikova, Biomed. Khim., 2016, 62, 99–106 CrossRef PubMed.
- N. S. Barteneva, E. Fasler-Kan, M. Bernimoulin, J. N. H. Stern, E. D. Ponomarev, L. Duckett and I. A. Vorobjev, BMC Cell Biol., 2013, 14, 23 CrossRef CAS PubMed.
- C. C. Pritchard, E. Kroh, B. Wood, J. D. Arroyo, K. J. Dougherty, M. M. Miyaji, J. F. Tait and M. Tewari, Cancer Prev. Res., 2012, 5, 492–497 CrossRef CAS PubMed.
- B. Fendl, R. Weiss, M. B. Fischer, A. Spittler and V. Weber, Biochem. Biophys. Res. Commun., 2016, 478, 168–173 CrossRef CAS PubMed.
- L. Wisgrill, C. Lamm, J. Hartmann, F. Preißing, K. Dragosits, A. Bee, L. Hell, J. Thaler, C. Ay, I. Pabinger, A. Berger and A. Spittler, Cytometry, Part A, 2016, 89, 663–672 CrossRef CAS PubMed.
- R. Lacroix, C. Judicone, P. Poncelet, S. Robert, L. Arnaud, J. Sampol and F. Dignat-George, J. Thromb. Haemostasis, 2012, 10, 437–446 CrossRef CAS PubMed.
- T. Meningher, G. Lerman, N. Regev-Rudzki, D. Gold, I. Z. Ben-Dov, Y. Sidi, D. Avni and E. Schwartz, J. Infect. Dis., 2017, 215, 378–386 Search PubMed.
- G. Vergauwen, B. Dhondt, J. Van Deun, E. De Smedt, G. Berx, E. Timmerman, K. Gevaert, I. Miinalainen, V. Cocquyt, G. Braems, R. Van den Broecke, H. Denys, O. De Wever and A. Hendrix, Sci. Rep., 2017, 7, 2704 CrossRef PubMed.
- L.-G. Liang, M.-Q. Kong, S. Zhou, Y.-F. Sheng, P. Wang, T. Yu, F. Inci, W. P. Kuo, L.-J. Li, U. Demirci and S. Wang, Sci. Rep., 2017, 7, 46224 CrossRef PubMed.
- K. W. Pedersen, B. Kierulf and A. Neurauter, Methods Mol. Biol., 2017, 1660, 65–87 Search PubMed.
- S. I. Gilani, U. D. Anderson, M. Jayachandran, T. L. Weissgerber, L. Zand, W. M. White, N. Milic, M. L. G. Suarez, R. R. Vallapureddy, Å. Nääv, L. Erlandsson, J. C. Lieske, J. P. Grande, K. A. Nath, S. R. Hansson and V. D. Garovic, J. Am. Soc. Nephrol., 2017, 28, 3363–3372 CrossRef PubMed.
- M. I. Zonneveld, A. R. Brisson, M. J. C. van Herwijnen, S. Tan, C. H. A. van de Lest, F. A. Redegeld, J. Garssen, M. H. M. Wauben and E. N. Nolte-'t Hoen, J. Extracell. Vesicles, 2014, 3, 1–12 Search PubMed.
- K. W. Witwer, E. I. Buzás, L. T. Bemis, A. Bora, C. Lässer, J. Lötvall, E. N. Nolte-'t Hoen, M. G. Piper, S. Sivaraman, J. Skog, C. Théry, M. H. Wauben and F. Hochberg, J. Extracell. Vesicles, 2013, 2, 20360 CrossRef PubMed.
- Z. Wei, A. O. Batagov, D. R. F. Carter and A. M. Krichevsky, Sci. Rep., 2016, 6, 31175 CrossRef CAS PubMed.
- G. V. Shelke, C. Lässer, Y. S. Gho and J. Lötvall, J. Extracell. Vesicles, 2014, 3, 1–8 Search PubMed.
- L. C. Laurent, A. B. Abdel-Mageed, P. D. Adelson, J. Arango, L. Balaj, X. Breakefield, E. Carlson, B. S. Carter, B. Majem, C. C. Chen, E. Cocucci, K. Danielson, A. Courtright, S. Das, Z. Y. Abd Elmageed, D. Enderle, A. Ezrin, M. Ferrer, J. Freedman, D. Galas, R. Gandhi, M. J. Huentelman, K. Van Keuren-Jensen, Y. Kalani, Y. Kim, A. M. Krichevsky, C. Lai, M. Lal-Nag, C. D. Laurent, T. Leonardo, F. Li, I. Malenica, D. Mondal, P. Nejad, T. Patel, R. L. Raffai, R. Rubio, J. Skog, R. Spetzler, J. Sun, K. Tanriverdi, K. Vickers, L. Wang, Y. Wang, Z. Wei, H. L. Weiner, D. Wong, I. K. Yan, A. Yeri and S. Gould, J. Extracell. Vesicles, 2015, 4, 26533 CrossRef PubMed.
- Z. Wei, A. O. Batagov, D. R. F. Carter and A. M. Krichevsky, Sci. Rep., 2016, 6, 31175 CrossRef CAS PubMed.
- C. Théry, M. Boussac, P. Véron, P. Ricciardi-Castagnoli, G. Raposo, J. Garin and S. Amigorena, J. Immunol., 2001, 166, 7309–7318 CrossRef.
- Á. M. Lőrincz, C. I. Timár, K. A. Marosvári, D. S. Veres, L. Otrokocsi, Á. Kittel and E. Ligeti, J. Extracell. Vesicles, 2014, 3, 25465 CrossRef PubMed.
- Y. Yuana, A. N. Böing, A. E. Grootemaat, E. van der Pol, C. M. Hau, P. Cizmar, E. Buhr, A. Sturk and R. Nieuwland, J. Extracell. Vesicles, 2015, 4, 29260 CrossRef PubMed.
- H. Kalra, C. G. Adda, M. Liem, C.-S. Ang, A. Mechler, R. J. Simpson, M. D. Hulett and S. Mathivanan, Proteomics, 2013, 13, 3354–3364 CrossRef CAS PubMed.
- J. Lötvall, A. F. Hill, F. Hochberg, E. I. Buzás, D. Di Vizio, C. Gardiner, Y. S. Gho, I. V. Kurochkin, S. Mathivanan, P. Quesenberry, S. Sahoo, H. Tahara, M. H. Wauben, K. W. Witwer and C. Théry, J. Extracell. Vesicles, 2014, 3, 26913 CrossRef PubMed.
- B. Mateescu, E. J. K. Kowal, B. W. M. van Balkom, S. Bartel, S. N. Bhattacharyya, E. I. Buzás, A. H. Buck, P. de Candia, F. W. N. Chow, S. Das, T. A. P. Driedonks, L. Fernández-Messina, F. Haderk, A. F. Hill, J. C. Jones, K. R. Van Keuren-Jensen, C. P. Lai, C. Lässer, I. di Liegro, T. R. Lunavat, M. J. Lorenowicz, S. L. N. Maas, I. Mäger, M. Mittelbrunn, S. Momma, K. Mukherjee, M. Nawaz, D. M. Pegtel, M. W. Pfaffl, R. M. Schiffelers, H. Tahara, C. Théry, J. P. Tosar, M. H. M. Wauben, K. W. Witwer and E. N. M. Nolte-'t Hoen, J. Extracell. Vesicles, 2017, 6, 1286095 CrossRef PubMed.
- EV-TRACK Consortium, J. Van Deun, P. Mestdagh, P. Agostinis, Ö. Akay, S. Anand, J. Anckaert, Z. A. Martinez, T. Baetens, E. Beghein, L. Bertier, G. Berx, J. Boere, S. Boukouris, M. Bremer, D. Buschmann, J. B. Byrd, C. Casert, L. Cheng, A. Cmoch, D. Daveloose, E. De Smedt, S. Demirsoy, V. Depoorter, B. Dhondt, T. A. P. Driedonks, A. Dudek, A. Elsharawy, I. Floris, A. D. Foers, K. Gärtner, A. D. Garg, E. Geeurickx, J. Gettemans, F. Ghazavi, B. Giebel, T. G. Kormelink, G. Hancock, H. Helsmoortel, A. F. Hill, V. Hyenne, H. Kalra, D. Kim, J. Kowal, S. Kraemer, P. Leidinger, C. Leonelli, Y. Liang, L. Lippens, S. Liu, A. Lo Cicero, S. Martin, S. Mathivanan, P. Mathiyalagan, T. Matusek, G. Milani, M. Monguió-Tortajada, L. M. Mus, D. C. Muth, A. Németh, E. N. M. Nolte-'t Hoen, L. O'Driscoll, R. Palmulli, M. W. Pfaffl, B. Primdal-Bengtson, E. Romano, Q. Rousseau, S. Sahoo, N. Sampaio, M. Samuel, B. Scicluna, B. Soen, A. Steels, J. V. Swinnen, M. Takatalo, S. Thaminy, C. Théry, J. Tulkens, I. Van Audenhove, S. van der Grein, A. Van Goethem, M. J. van Herwijnen, G. Van Niel, N. Van Roy, A. R. Van Vliet, N. Vandamme, S. Vanhauwaert, G. Vergauwen, F. Verweij, A. Wallaert, M. Wauben, K. W. Witwer, M. I. Zonneveld, O. De Wever, J. Vandesompele and A. Hendrix, Nat. Methods, 2017, 14, 228–232 CrossRef PubMed.
- C. Gardiner, D. Di Vizio, S. Sahoo, C. Théry, K. W. Witwer, M. Wauben and A. F. Hill, J. Extracell. Vesicles, 2016, 5, 32945 CrossRef PubMed.
- Z. Andreu, E. Rivas, A. Sanguino-Pascual, A. Lamana, M. Marazuela, I. González-Alvaro, F. Sánchez-Madrid, H. de la Fuente and M. Yáñez-Mó, J. Extracell. Vesicles, 2016, 5, 31655 CrossRef PubMed.
-
C. Théry, S. Amigorena, G. Raposo and A. Clayton, Curr Protoc cell Biol, 2006, ch. 3, unit 3.22 Search PubMed.
- R. T. Davies, J. Kim, S. C. Jang, E.-J. Choi, Y. S. Gho and J. Park, Lab Chip, 2012, 12, 5202 RSC.
- J. Z. Nordin, Y. Lee, P. Vader, I. Mäger, H. J. Johansson, W. Heusermann, O. P. B. Wiklander, M. Hällbrink, Y. Seow, J. J. Bultema, J. Gilthorpe, T. Davies, P. J. Fairchild, S. Gabrielsson, N. C. Meisner-Kober, J. Lehtiö, C. I. E. Smith, M. J. A. Wood and S. El Andaloussi, Nanomedicine, 2015, 11, 879–883 CrossRef CAS PubMed.
- G. Raposo and W. Stoorvogel, J. Cell Biol., 2013, 200, 373–383 CrossRef CAS PubMed.
- H. G. Lamparski, A. Metha-Damani, J.-Y. Yao, S. Patel, D.-H. Hsu, C. Ruegg and J.-B. Le Pecq, J. Immunol. Methods, 2002, 270, 211–226 CrossRef CAS PubMed.
- Z. Zhang, C. Wang, T. Li, Z. Liu and L. Li, Oncol. Lett., 2014, 8, 1701–1706 CAS.
- R. J. Lobb, M. Becker, S. W. Wen, C. S. F. Wong, A. P. Wiegmans, A. Leimgruber and A. Möller, J. Extracell. Vesicles, 2015, 4, 27031 CrossRef PubMed.
- B. J. Tauro, D. W. Greening, R. A. Mathias, H. Ji, S. Mathivanan, A. M. Scott and R. J. Simpson, Methods, 2012, 56, 293–304 CrossRef CAS PubMed.
- E. Nolte-'t Hoen, T. Cremer, R. C. Gallo and L. B. Margolis, Proc. Natl. Acad. Sci. U. S. A., 2016, 113, 9155–9161 CrossRef PubMed.
- B. W. Sódar, Á. Kittel, K. Pálóczi, K. V. Vukman, X. Osteikoetxea, K. Szabó-Taylor, A. Németh, B. Sperlágh, T. Baranyai, Z. Giricz, Z. Wiener, L. Turiák, L. Drahos, É. Pállinger, K. Vékey, P. Ferdinandy, A. Falus and E. I. Buzás, Sci. Rep., 2016, 6, 24316 CrossRef PubMed.
- Y. Yuana, J. Levels, A. Grootemaat, A. Sturk and R. Nieuwland, J. Extracell. Vesicles, 2014, 3, 23262 CrossRef PubMed.
- A. Kamińska, M. Platt, J. Kasprzyk, B. Kuśnierz-Cabala, A. Gala-Błądzińska, O. Woźnicka, B. R. Jany, F. Krok, W. Piekoszewski, M. Kuźniewski and E. Ł. Stępień, J. Diabetes Res., 2016, 2016, 5741518 Search PubMed.
- R. Cantin, J. Diou, D. Bélanger, A. M. Tremblay and C. Gilbert, J. Immunol. Methods, 2008, 338, 21–30 CrossRef CAS PubMed.
- S. Mathivanan, J. W. E. Lim, B. J. Tauro, H. Ji, R. L. Moritz and R. J. Simpson, Mol. Cell. Proteomics, 2010, 9, 197–208 CAS.
- K. Koga, K. Matsumoto, T. Akiyoshi, M. Kubo, N. Yamanaka, A. Tasaki, H. Nakashima, M. Nakamura, S. Kuroki, M. Tanaka and M. Katano, Anticancer Res., 2005, 25, 3703–3707 CAS.
- A. Liga, A. D. B. Vliegenthart, W. Oosthuyzen, J. W. Dear and M. Kersaudy-Kerhoas, Lab Chip, 2015, 15, 2388–2394 RSC.
- R. Xu, D. W. Greening, H.-J. Zhu, N. Takahashi and R. J. Simpson, J. Clin. Invest., 2016, 126, 1152–1162 Search PubMed.
- H. Kalra, C. G. Adda, M. Liem, C. S. Ang, A. Mechler, R. J. Simpson, M. D. Hulett and S. Mathivanan, Proteomics, 2013, 13, 3354–3364 CrossRef CAS PubMed.
- C. E. Yoo, G. Kim, M. Kim, D. Park, H. J. Kang, M. Lee and N. Huh, Anal. Biochem., 2012, 431, 96–98 CrossRef CAS PubMed.
- L. Balaj, N. A. Atai, W. Chen, D. Mu, B. A. Tannous, X. O. Breakefield, J. Skog and C. A. Maguire, Sci. Rep., 2015, 5, 10266 CrossRef CAS PubMed.
- C. W. Kim, H. M. Lee, T. H. Lee, C. Kang, H. K. Kleinman and Y. S. Gho, Cancer Res., 2002, 62, 6312–6317 CAS.
- A. Ghosh, M. Davey, I. C. Chute, S. G. Griffiths, S. Lewis, S. Chacko, D. Barnett, N. Crapoulet, S. Fournier, A. Joy, M. C. Caissie, A. D. Ferguson, M. Daigle, M. V. Meli, S. M. Lewis and R. J. Ouellette, PLoS One, 2014, 9, e110443 Search PubMed.
- D. K. Jeppesen, M. L. Hvam, B. Primdahl-Bengtson, A. T. Boysen, B. Whitehead, L. Dyrskjøt, T. F. Orntoft, K. A. Howard and M. S. Ostenfeld, J. Extracell. Vesicles, 2014, 3, 25011 CrossRef PubMed.
- C. Chen, J. Skog, C.-H. Hsu, R. T. Lessard, L. Balaj, T. Wurdinger, B. S. Carter, X. O. Breakefield, M. Toner and D. Irimia, Lab Chip, 2010, 10, 505–511 RSC.
- S. S. Kanwar, C. J. Dunlay, D. M. Simeone and S. Nagrath, Lab Chip, 2014, 14, 1891–1900 RSC.
- D. Taller, K. Richards, Z. Slouka, S. Senapati, R. Hill, D. B. Go and H.-C. Chang, Lab Chip, 2015, 15, 1656–1666 RSC.
- C.-S. Hong, S. Funk, L. Muller, M. Boyiadzis and T. L. Whiteside, J. Extracell. Vesicles, 2016, 5, 29289 CrossRef PubMed.
- T. Baranyai, K. Herczeg, Z. Onódi, I. Voszka, K. Módos, N. Marton, G. Nagy, I. Mäger, M. J. Wood, S. El Andaloussi, Z. Pálinkás, V. Kumar, P. Nagy, Á. Kittel, E. I. Buzás, P. Ferdinandy and Z. Giricz, PLoS One, 2015, 10, e0145686 Search PubMed.
- F. A. W. Coumans, A. R. Brisson, E. I. Buzas, F. Dignat-George, E. E. E. Drees, S. El-Andaloussi, C. Emanueli, A. Gasecka, A. Hendrix, A. F. Hill, R. Lacroix, Y. Lee, T. G. van Leeuwen, N. Mackman, I. Mäger, J. P. Nolan, E. van der Pol, D. M. Pegtel, S. Sahoo, P. R. M. Siljander, G. Sturk, O. de Wever and R. Nieuwland, Circ. Res., 2017, 120, 1632–1648 CrossRef CAS PubMed.
- B. W. Sódar, Á. Kovács, T. Visnovitz, É. Pállinger, K. Vékey, G. Pocsfalvi, L. Turiák and E. I. Buzás, Expert Rev. Proteomics, 2017, 1–18 Search PubMed.
- A. N. Böing, E. van der Pol, A. E. Grootemaat, F. A. W. Coumans, A. Sturk and R. Nieuwland, J. Extracell. Vesicles, 2014, 3, 23430 CrossRef PubMed.
- J. L. Welton, J. P. Webber, L.-A. Botos, M. Jones and A. Clayton, J. Extracell. Vesicles, 2015, 4, 27269 CrossRef PubMed.
- A. Gámez-Valero, M. Monguió-Tortajada, L. Carreras-Planella, M. la Franquesa, K. Beyer and F. E. Borràs, Sci. Rep., 2016, 6, 33641 CrossRef PubMed.
- M. L. Heinemann, M. Ilmer, L. P. Silva, D. H. Hawke, A. Recio, M. A. Vorontsova, E. Alt and J. Vykoukal, J. Chromatogr., A, 2014, 1371, 125–135 CrossRef CAS PubMed.
- J. Van Deun, P. Mestdagh, R. Sormunen, V. Cocquyt, K. Vermaelen, J. Vandesompele, M. Bracke, O. De Wever and A. Hendrix, J. Extracell. Vesicles, 2014, 3, 24858 CrossRef PubMed.
- E. A. Mol, M.-J. Goumans, P. A. Doevendans, J. P. G. Sluijter and P. Vader, Nanomedicine, 2017, 13, 2061–2065 CrossRef CAS PubMed.
- E. J. van der Vlist, E. N. M. Nolte-'t Hoen, W. Stoorvogel, G. J. A. Arkesteijn and M. H. M. Wauben, Nat. Protoc., 2012, 7, 1311–1326 CrossRef PubMed.
- H. B. Steen, Cytometry, Part A, 2004, 57, 94–99 CrossRef PubMed.
- J. Webber and A. Clayton, J. Extracell. Vesicles, 2013, 2, 19861 CrossRef PubMed.
- A. Bobrie and C. Théry, OncoImmunology, 2013, 2, e22565 CrossRef PubMed.
- V. S. Chernyshev, R. Rachamadugu, Y. H. Tseng, D. M. Belnap, Y. Jia, K. J. Branch, A. E. Butterfield, L. F. Pease, P. S. Bernard and M. Skliar, Anal. Bioanal. Chem., 2015, 407, 3285–3301 CrossRef CAS PubMed.
- E. van der Pol, F. Coumans, Z. Varga, M. Krumrey and R. Nieuwland, J. Thromb. Haemostasis, 2013, 11(Suppl 1), 36–45 CrossRef PubMed.
- J. Conde-Vancells, E. Rodriguez-Suarez, N. Embade, D. Gil, R. Matthiesen, M. Valle, F. Elortza, S. C. Lu, J. M. Mato and J. M. Falcon-Perez, J. Proteome Res., 2008, 7, 5157–5166 CrossRef CAS PubMed.
- B. M. Coleman, E. Hanssen, V. A. Lawson and A. F. Hill, FASEB J., 2012, 26, 4160–4173 CrossRef CAS PubMed.
- N. Grigorieff and S. C. Harrison, Curr. Opin. Struct. Biol., 2011, 21, 265–273 CrossRef CAS PubMed.
- Y. Yuana, R. I. Koning, M. E. Kuil, P. C. N. Rensen, A. J. Koster, R. M. Bertina and S. Osanto, J. Extracell. Vesicles, 2013, 2, 1–7 Search PubMed.
- R. A. Dragovic, C. Gardiner, A. S. Brooks, D. S. Tannetta, D. J. P. Ferguson, P. Hole, B. Carr, C. W. G. Redman, A. L. Harris, P. J. Dobson, P. Harrison and I. L. Sargent, Nanomedicine, 2011, 7, 780–788 CrossRef CAS PubMed.
- E. van der Pol, F. A. W. Coumans, A. E. Grootemaat, C. Gardiner, I. L. Sargent, P. Harrison, A. Sturk, T. G. van Leeuwen and R. Nieuwland, J. Thromb. Haemostasis, 2014, 12, 1182–1192 CrossRef CAS PubMed.
- S. Baldwin, C. Deighan, E. Bandeira, K. J. Kwak, M. Rahman, P. Nana-Sinkam, L. J. Lee and M. E. Paulaitis, Nanomedicine, 2017, 13, 765–770 CrossRef CAS PubMed.
- T. Ito, L. Sun, M. A. Bevan and R. M. Crooks, Langmuir, 2004, 20, 6940–6945 CrossRef CAS PubMed.
- S. L. N. Maas, M. L. D. Broekman and J. de Vrij, Methods Mol. Biol., 2017, 1545, 21–33 Search PubMed.
- J. P. Nolan, Curr. Protoc. Cytom., 2015, 73, 13.14.1–13.14.16 Search PubMed.
- W. L. Chandler, W. Yeung and J. F. Tait, J. Thromb. Haemostasis, 2011, 9, 1216–1224 CrossRef CAS PubMed.
- S. Montoro-García, E. Shantsila, E. Orenes-Piñero, M. L. Lozano and G. Y. H. Lip, Thromb. Haemostasis, 2012, 108, 373–383 CrossRef PubMed.
- S. A. Stoner, E. Duggan, D. Condello, A. Guerrero, J. R. Turk, P. K. Narayanan and J. P. Nolan, Cytometry, Part A, 2016, 89, 196–206 CrossRef CAS PubMed.
- R. Hulspas, M. R. G. O'Gorman, B. L. Wood, J. W. Gratama and D. R. Sutherland, Cytometry, Part B, 2009, 76, 355–364 CrossRef PubMed.
- E. van der Pol, M. J. C. van Gemert, A. Sturk, R. Nieuwland and T. G. van Leeuwen, J. Thromb. Haemostasis, 2012, 10, 919–930 CrossRef CAS PubMed.
- M. Eldh, J. Lötvall, C. Malmhäll and K. Ekström, Mol. Immunol., 2012, 50, 278–286 CrossRef CAS PubMed.
- A. F. Hill, D. M. Pegtel, U. Lambertz, T. Leonardi, L. O'Driscoll, S. Pluchino, D. Ter-Ovanesyan and E. N. M. Nolte-'t Hoen, J. Extracell. Vesicles, 2013, 2, 1–8 CAS.
- F. Momen-Heravi, L. Balaj, S. Alian, A. J. Trachtenberg, F. H. Hochberg, J. Skog and W. P. Kuo, Front. Physiol., 2012, 3, 162 CAS.
- D.-J. Kim, S. Linnstaedt, J. Palma, J. C. Park, E. Ntrivalas, J. Y. H. Kwak-Kim, A. Gilman-Sachs, K. Beaman, M. L. Hastings, J. N. Martin and D. M. Duelli, J. Mol. Diagn., 2012, 14, 71–80 CrossRef CAS PubMed.
- C. H. Gemmell, M. V. Sefton and E. L. Yeo, J. Biol. Chem., 1993, 268, 14586–14589 CAS.
- H. Valadi, K. Ekström, A. Bossios, M. Sjöstrand, J. J. Lee and J. O. Lötvall, Nat. Cell Biol., 2007, 9, 654–659 CrossRef CAS PubMed.
- J. Skog, T. Würdinger, S. van Rijn, D. H. Meijer, L. Gainche, M. Sena-Esteves, W. T. Curry, B. S. Carter, A. M. Krichevsky and X. O. Breakefield, Nat. Cell Biol., 2008, 10, 1470–1476 CrossRef CAS PubMed.
- M. P. Hunter, N. Ismail, X. Zhang, B. D. Aguda, E. J. Lee, L. Yu, T. Xiao, J. Schafer, M.-L. T. Lee, T. D. Schmittgen, S. P. Nana-Sinkam, D. Jarjoura and C. B. Marsh, PLoS One, 2008, 3, e3694 Search PubMed.
- L. Manterola, E. Guruceaga, J. Gállego Pérez-Larraya, M. González-Huarriz, P. Jauregui, S. Tejada, R. Diez-Valle, V. Segura, N. Samprón, C. Barrena, I. Ruiz, A. Agirre, A. Ayuso, J. Rodríguez, A. González, E. Xipell, A. Matheu, A. López de Munain, T. Tuñón, I. Zazpe, J. García-Foncillas, S. Paris, J. Y. Delattre and M. M. Alonso, Neuro-Oncology, 2014, 16, 520–527 CrossRef CAS PubMed.
- V. N. Aushev, I. B. Zborovskaya, K. K. Laktionov, N. Girard, M.-P. Cros, Z. Herceg and V. Krutovskikh, PLoS One, 2013, 8, e78649 CAS.
- J. R. Chevillet, Q. Kang, I. K. Ruf, H. A. Briggs, L. N. Vojtech, S. M. Hughes, H. H. Cheng, J. D. Arroyo, E. K. Meredith, E. N. Gallichotte, E. L. Pogosova-Agadjanyan, C. Morrissey, D. L. Stirewalt, F. Hladik, E. Y. Yu, C. S. Higano and M. Tewari, Proc. Natl. Acad. Sci. U. S. A., 2014, 111, 14888–14893 CrossRef CAS PubMed.
- D. Enderle, A. Spiel, C. M. Coticchia, E. Berghoff, R. Mueller, M. Schlumpberger, M. Sprenger-Haussels, J. M. Shaffer, E. Lader, J. Skog and M. Noerholm, PLoS One, 2015, 10, 1–19 Search PubMed.
- L. Moldovan, K. Batte, Y. Wang, J. Wisler and M. Piper, Methods Mol. Biol., 2013, 1024, 129–145 CAS.
- M. J. Marzi, F. Montani, R. M. Carletti, F. Dezi, E. Dama, G. Bonizzi, M. T. Sandri, C. Rampinelli, M. Bellomi, P. Maisonneuve, L. Spaggiari, G. Veronesi, F. Bianchi, P. P. Di Fiore and F. Nicassio, Clin. Chem., 2016, 62, 743–754 CAS.
- K. C. Miranda, D. T. Bond, J. Z. Levin, X. Adiconis, A. Sivachenko, C. Russ, D. Brown, C. Nusbaum and L. M. Russo, PLoS One, 2014, 9, e96094 Search PubMed.
- L. Cheng, X. Sun, B. J. Scicluna, B. M. Coleman and A. F. Hill, Kidney Int., 2014, 86, 433–444 CrossRef CAS PubMed.
- A. Eirin, S. M. Riester, X.-Y. Zhu, H. Tang, J. M. Evans, D. O'Brien, A. J. van Wijnen and L. O. Lerman, Gene, 2014, 551, 55–64 CrossRef CAS PubMed.
- M. G. Amorim, R. Valieris, R. D. Drummond, M. P. Pizzi, V. M. Freitas, R. Sinigaglia-Coimbra, G. A. Calin, R. Pasqualini, W. Arap, I. T. Silva, E. Dias-Neto and D. N. Nunes, Sci. Rep., 2017, 7, 14395 CrossRef PubMed.
- E. N. M. Nolte'T Hoen, H. P. J. Buermans, M. Waasdorp, W. Stoorvogel, M. H. M. Wauben and P. A. C. ‘t Hoen, Nucleic Acids Res., 2012, 40, 9272–9285 CrossRef PubMed.
- X. Huang, T. Yuan, M. Tschannen, Z. Sun, H. Jacob, M. Du, M. Liang, R. L. Dittmar, Y. Liu, M. Liang, M. Kohli, S. N. Thibodeau, L. Boardman and L. Wang, BMC Genomics, 2013, 14, 319 CrossRef CAS PubMed.
- J. E. Freedman, M. Gerstein, E. Mick, J. Rozowsky, D. Levy, R. Kitchen, S. Das, R. Shah, K. Danielson, L. Beaulieu, F. C. P. Navarro, Y. Wang, T. R. Galeev, A. Holman, R. Y. Kwong, V. Murthy, S. E. Tanriverdi, M. Koupenova-Zamor, E. Mikhalev and K. Tanriverdi, Nat. Commun., 2016, 7, 11106 CrossRef CAS PubMed.
- T. Yuan, X. Huang, M. Woodcock, M. Du, R. Dittmar, Y. Wang, S. Tsai, M. Kohli, L. Boardman, T. Patel and L. Wang, Sci. Rep., 2016, 6, 19413 CrossRef CAS PubMed.
- P. Jenjaroenpun, Y. Kremenska, V. M. Nair, M. Kremenskoy, B. Joseph and I. V. Kurochkin, PeerJ, 2013, 1, e201 Search PubMed.
- F. A. Lefebvre, L. P. Benoit Bouvrette, L. Perras, A. Blanchet-Cohen, D. Garnier, J. Rak and É. Lécuyer, Sci. Rep., 2016, 6, 27680 CrossRef CAS PubMed.
- F. A. San Lucas, K. Allenson, V. Bernard, J. Castillo, D. U. Kim, K. Ellis, E. A. Ehli, G. E. Davies, J. L. Petersen, D. Li, R. Wolff, M. Katz, G. Varadhachary, I. Wistuba, A. Maitra and H. Alvarez, Ann. Oncol., 2016, 27, 635–641 CrossRef CAS PubMed.
- B. Mateescu, E. J. K. Kowal, B. W. M. van Balkom, S. Bartel, S. N. Bhattacharyya, E. I. Buzás, A. H. Buck, P. de Candia, F. W. N. Chow, S. Das, T. A. P. Driedonks, L. Fernández-Messina, F. Haderk, A. F. Hill, J. C. Jones, K. R. Van Keuren-Jensen, C. P. Lai, C. Lässer, I. di Liegro, T. R. Lunavat, M. J. Lorenowicz, S. L. N. Maas, I. Mäger, M. Mittelbrunn, S. Momma, K. Mukherjee, M. Nawaz, D. M. Pegtel, M. W. Pfaffl, R. M. Schiffelers, H. Tahara, C. Théry, J. P. Tosar, M. H. M. Wauben, K. W. Witwer and E. N. M. Nolte-'t Hoen, J. Extracell. Vesicles, 2017, 6, 1286095 CrossRef PubMed.
- M. Del Re, E. Biasco, S. Crucitta, L. Derosa, E. Rofi, C. Orlandini, M. Miccoli, L. Galli, A. Falcone, G. W. Jenster, R. H. van Schaik and R. Danesi, Eur. Urol., 2017, 71, 680–687 CrossRef CAS PubMed.
- W. W. Chen, L. Balaj, L. M. Liau, M. L. Samuels, S. K. Kotsopoulos, C. A. Maguire, L. Loguidice, H. Soto, M. Garrett, L. D. Zhu, S. Sivaraman, C. Chen, E. T. Wong, B. S. Carter, F. H. Hochberg, X. O. Breakefield and J. Skog, Mol. Ther.–Nucleic Acids, 2013, 2, e109 CrossRef PubMed.
-
S. Andrews
, FastQC: A quality control tool for high throughput sequence data.
- H. Li and R. Durbin, Bioinformatics, 2009, 25, 1754–1760 CrossRef CAS PubMed.
- B. Langmead and S. L. Salzberg, Nat. Methods, 2012, 9, 357–359 CrossRef CAS PubMed.
- A. Dobin, C. A. Davis, F. Schlesinger, J. Drenkow, C. Zaleski, S. Jha, P. Batut, M. Chaisson and T. R. Gingeras, Bioinformatics, 2013, 29, 15–21 CrossRef CAS PubMed.
- Y. Liao, G. K. Smyth and W. Shi, Bioinformatics, 2014, 30, 923–930 CrossRef CAS PubMed.
- S. Anders, P. T. Pyl and W. Huber, Bioinformatics, 2015, 31, 166–169 CrossRef CAS PubMed.
- A. Mortazavi, B. A. Williams, K. McCue, L. Schaeffer and B. Wold, Nat. Methods, 2008, 5, 621–628 CrossRef CAS PubMed.
- B. Li and C. N. Dewey, BMC Bioinf., 2011, 12, 323 CrossRef CAS PubMed.
- S. Anders and W. Huber, Genome Biol., 2010, 11, R106 CrossRef CAS PubMed.
- M. D. Robinson, D. J. McCarthy and G. K. Smyth, Bioinformatics, 2010, 26, 139–140 CrossRef CAS PubMed.
- A. Subramanian, P. Tamayo, V. K. Mootha, S. Mukherjee, B. L. Ebert, M. A. Gillette, A. Paulovich, S. L. Pomeroy, T. R. Golub, E. S. Lander and J. P. Mesirov, Proc. Natl. Acad. Sci. U. S. A., 2005, 102, 15545–15550 CrossRef CAS PubMed.
- R. C. Gentleman, V. J. Carey, D. M. Bates, B. Bolstad, M. Dettling, S. Dudoit, B. Ellis, L. Gautier, Y. Ge, J. Gentry, K. Hornik, T. Hothorn, W. Huber, S. Iacus, R. Irizarry, F. Leisch, C. Li, M. Maechler, A. J. Rossini, G. Sawitzki, C. Smith, G. Smyth, L. Tierney, J. Y. H. Yang and J. Zhang, Genome Biol., 2004, 5, R80 CrossRef PubMed.
- D. M. Pegtel, L. Peferoen and S. Amor, Philos. Trans. R. Soc. London, Ser. B, 2014, 369, 20130516–20130516 CrossRef PubMed.
- M. Tkach and C. Théry, Cell, 2016, 164, 1226–1232 CrossRef CAS PubMed.
- E.-Y. Lee, D.-S. Choi, K.-P. Kim and Y. S. Gho, Mass Spectrom. Rev., 2008, 27, 535–555 CrossRef CAS PubMed.
- L. Miguet, K. Pacaud, C. Felden, B. Hugel, M. C. Martinez, J.-M. Freyssinet, R. Herbrecht, N. Potier, A. van Dorsselaer and L. Mauvieux, Proteomics, 2006, 6, 153–171 CrossRef CAS PubMed.
- T. Pisitkun, R.-F. Shen and M. A. Knepper, Proc. Natl. Acad. Sci. U. S. A., 2004, 101, 13368–13373 CrossRef CAS PubMed.
- C. Lässer, M. Eldh and J. Lötvall, J. Visualized Exp., 2012, e3037 Search PubMed.
- Y. Yoshioka, Y. Konishi, N. Kosaka, T. Katsuda, T. Kato and T. Ochiya, J. Extracell. Vesicles, 2013, 2, 20424 CrossRef PubMed.
- J. Kowal, G. Arras, M. Colombo, M. Jouve, J. P. Morath, B. Primdal-Bengtson, F. Dingli, D. Loew, M. Tkach and C. Théry, Proc. Natl. Acad. Sci. U. S. A., 2016, 113, E968–E977 CrossRef CAS PubMed.
- S. A. Melo, L. B. Luecke, C. Kahlert, A. F. Fernandez, S. T. Gammon, J. Kaye, V. S. Lebleu, E. A. Mittendorf, J. Weitz, N. Rahbari, C. Reissfelder, C. Pilarsky, M. F. Fraga, D. Piwnica-Worms and R. Kalluri, Nature, 2015, 523, 177–182 CrossRef CAS PubMed.
- Y.-S. Kim, W.-H. Lee, E.-J. Choi, J.-P. Choi, Y. J. Heo, Y. S. Gho, Y.-K. Jee, Y.-M. Oh and Y.-K. Kim, J. Immunol., 2015, 194, 3361–3368 CrossRef CAS PubMed.
- M. Cao, J. Ning, C. I. Hernandez-Lara, O. Belzile, Q. Wang, S. K. Dutcher, Y. Liu and W. J. Snell, eLife, 2015, 4, 5242 Search PubMed.
- J. Sotillo, M. Pearson, J. Potriquet, L. Becker, D. Pickering, J. Mulvenna and A. Loukas, Int. J. Parasitol., 2016, 46, 1–5 CrossRef CAS PubMed.
- I. Cestari, E. Ansa-Addo, P. Deolindo, J. M. Inal and M. I. Ramirez, J. Immunol., 2012, 188, 1942–1952 CrossRef CAS PubMed.
- W. Nakai, T. Yoshida, D. Diez, Y. Miyatake, T. Nishibu, N. Imawaka, K. Naruse, Y. Sadamura and R. Hanayama, Sci. Rep., 2016, 6, 33935 CrossRef CAS PubMed.
- B. Domon and R. Aebersold, Nat. Biotechnol., 2010, 28, 710–721 CrossRef CAS PubMed.
- D. A. Wolters, M. P. Washburn and J. R. Yates, Anal. Chem., 2001, 73, 5683–5690 CrossRef CAS PubMed.
- V. Lange, P. Picotti, B. Domon and R. Aebersold, Mol. Syst. Biol., 2008, 4, 222 CrossRef PubMed.
- S. D. Sherrod, M. V. Myers, M. Li, J. S. Myers, K. L. Carpenter, B. Maclean, M. J. Maccoss, D. C. Liebler and A.-J. L. Ham, J. Proteome Res., 2012, 11, 3467–3479 CrossRef CAS PubMed.
- S. Gallien, E. Duriez, C. Crone, M. Kellmann, T. Moehring and B. Domon, Mol. Cell. Proteomics, 2012, 11, 1709–1723 Search PubMed.
- A. C. Peterson, J. D. Russell, D. J. Bailey, M. S. Westphall and J. J. Coon, Mol. Cell. Proteomics, 2012, 11, 1475–1488 Search PubMed.
- L. C. Gillet, P. Navarro, S. Tate, H. Röst, N. Selevsek, L. Reiter, R. Bonner and R. Aebersold, Mol. Cell. Proteomics, 2012, 11, O111.016717 Search PubMed.
- J. D. Egertson, A. Kuehn, G. E. Merrihew, N. W. Bateman, B. X. MacLean, Y. S. Ting, J. D. Canterbury, D. M. Marsh, M. Kellmann, V. Zabrouskov, C. C. Wu and M. J. MacCoss, Nat. Methods, 2013, 10, 744–746 CrossRef CAS PubMed.
- J. D. Venable, M.-Q. Dong, J. Wohlschlegel, A. Dillin and J. R. Yates, Nat. Methods, 2004, 1, 39–45 CrossRef CAS PubMed.
- C.-C. Tsou, D. Avtonomov, B. Larsen, M. Tucholska, H. Choi, A.-C. Gingras and A. I. Nesvizhskii, Nat. Methods, 2015, 12, 258–264 CrossRef CAS PubMed , 7 p following 264.
- A. Abramowicz, P. Widlak and M. Pietrowska, Mol. BioSyst., 2016, 12, 1407–1419 RSC.
- S. Kreimer, A. M. Belov, I. Ghiran, S. K. Murthy, D. A. Frank and A. R. Ivanov, J. Proteome Res., 2015, 14, 2367–2384 CrossRef CAS PubMed.
- D.-S. Choi, D.-K. Kim, Y.-K. Kim and Y. S. Gho, Mass Spectrom. Rev., 2015, 34, 474–490 CrossRef CAS PubMed.
- O. N. Jensen, Nat. Rev. Mol. Cell Biol., 2006, 7, 391–403 CrossRef CAS PubMed.
- K. W. Moremen, M. Tiemeyer and A. V. Nairn, Nat. Rev. Mol. Cell Biol., 2012, 13, 448–462 CrossRef CAS PubMed.
- C. Looze, D. Yui, L. Leung, M. Ingham, M. Kaler, X. Yao, W. W. Wu, R.-F. Shen, M. P. Daniels and S. J. Levine, Biochem. Biophys. Res. Commun., 2009, 378, 433–438 CrossRef CAS PubMed.
- M. Gonzalez-Begne, B. Lu, X. Han, F. K. Hagen, A. R. Hand, J. E. Melvin and J. R. Yates, J. Proteome Res., 2009, 8, 1304–1314 CrossRef CAS PubMed.
- P. A. Gonzales, T. Pisitkun, J. D. Hoffert, D. Tchapyjnikov, R. A. Star, R. Kleta, N. S. Wang and M. A. Knepper, J. Am. Soc. Nephrol., 2009, 20, 363–379 CrossRef CAS PubMed.
- H. Huang, M. Haar Petersen, M. Ibañez-Vea, P. S. Lassen, M. R. Larsen and G. Palmisano, Mol. Cell. Proteomics, 2016, 15, 3282–3296 CAS.
- G. Palmisano, B. L. Parker, K. Engholm-Keller, S. E. Lendal, K. Kulej, M. Schulz, V. Schwammle, M. E. Graham, H. Saxtorph, S. J. Cordwell and M. R. Larsen, Mol. Cell. Proteomics, 2012, 11, 1191–1202 Search PubMed.
- M. N. Melo-Braga, T. Verano-Braga, I. R. León, D. Antonacci, F. C. S. Nogueira, J. J. Thelen, M. R. Larsen and G. Palmisano, Mol. Cell. Proteomics, 2012, 11, 945–956 CAS.
- D. Schwudke, K. Schuhmann, R. Herzog, S. R. Bornstein and A. Shevchenko, Cold Spring Harbor Perspect. Biol., 2011, 3, a004614 Search PubMed.
- R. Wubbolts, R. S. Leckie, P. T. M. Veenhuizen, G. Schwarzmann, W. Möbius, J. Hoernschemeyer, J.-W. Slot, H. J. Geuze and W. Stoorvogel, J. Biol. Chem., 2003, 278, 10963–10972 CrossRef CAS PubMed.
- K. Laulagnier, C. Motta, S. Hamdi, S. Roy, F. Fauvelle, J.-F. Pageaux, T. Kobayashi, J.-P. Salles, B. Perret, C. Bonnerot and M. Record, Biochem. J., 2004, 380, 161–171 CrossRef CAS PubMed.
- E. G. Bligh and W. J. Dyer, Can. J. Biochem. Physiol., 1959, 37, 911–917 CrossRef CAS PubMed.
- J. Folch, M. Lees and G. H. Sloane Stanley, J. Biol. Chem., 1957, 226, 497–509 CAS.
- V. Matyash, G. Liebisch, T. V. Kurzchalia, A. Shevchenko and D. Schwudke, J. Lipid Res., 2008, 49, 1137–1146 CrossRef CAS PubMed.
- R. A. Haraszti, M. Didiot, E. Sapp, J. Leszyk, S. A. Shaffer, H. E. Rockwell, F. Gao, N. R. Narain, M. DiFiglia, M. A. Kiebish, N. Aronin and A. Khvorova, J. Extracell. Vesicles, 2016, 5, 32570 CrossRef PubMed.
- M. C. Vallejo, E. S. Nakayasu, A. L. Matsuo, T. J. P. Sobreira, L. V. G. Longo, L. Ganiko, I. C. Almeida and R. Puccia, J. Proteome Res., 2012, 11, 1676–1685 CrossRef CAS PubMed.
- P. Del Boccio, F. Raimondo, D. Pieragostino, L. Morosi, G. Cozzi, P. Sacchetta, F. Magni, M. Pitto and A. Urbani, Electrophoresis, 2012, 33, 689–696 CrossRef CAS PubMed.
- X. Gallart-Palau, A. Serra, A. S. W. Wong, S. Sandin, M. K. P. Lai, C. P. Chen, O. L. Kon and S. K. Sze, Sci. Rep., 2015, 5, 14664 CrossRef CAS PubMed.
- D. S. Choi, D. K. Kim, Y. K. Kim and Y. S. Gho, Proteomics, 2013, 13, 1554–1571 CrossRef CAS PubMed.
- A. Llorente, T. Skotland, T. Sylvänne, D. Kauhanen, T. Róg, A. Orłowski, I. Vattulainen, K. Ekroos and K. Sandvig, Biochim. Biophys. Acta, 2013, 1831, 1302–1309 CrossRef CAS.
- C. Subra, K. Laulagnier, B. Perret and M. Record, Biochimie, 2007, 89, 205–212 CrossRef CAS PubMed.
- J. Dalli and C. N. Serhan, Blood, 2012, 120, e60–e72 CrossRef CAS PubMed.
- T. Altadill, I. Campoy, L. Lanau, K. Gill, M. Rigau, A. Gil-Moreno, J. Reventos, S. Byers, E. Colas and A. K. Cheema, PLoS One, 2016, 11, e0151339 Search PubMed.
- J. Dalli and C. Serhan, Microbiol. Spectrum, 2016, 4, 37–54 Search PubMed.
- R. A. Colas, M. Shinohara, J. Dalli, N. Chiang and C. N. Serhan, Am. J. Physiol.: Cell Physiol., 2014, 307, C39–C54 CrossRef CAS PubMed.
- C. Subra, D. Grand, K. Laulagnier, A. Stella, G. Lambeau, M. Paillasse, P. De Medina, B. Monsarrat, B. Perret, S. Silvente-Poirot, M. Poirot and M. Record, J. Lipid Res., 2010, 51, 2105–2120 CrossRef CAS PubMed.
- D. W. Greening, R. Xu, S. K. Gopal, A. Rai and R. J. Simpson, Expert Rev. Proteomics, 2017, 14, 69–95 CrossRef CAS PubMed.
- H. C. Köfeler, A. Fauland, G. N. Rechberger and M. Trötzmüller, Metabolites, 2012, 2, 19–38 CrossRef PubMed.
- E. Fahy, D. Cotter, M. Sud and S. Subramaniam, Biochim. Biophys. Acta, 2011, 1811, 637–647 CrossRef CAS PubMed.
- R. Herzog, D. Schwudke, K. Schuhmann, J. L. Sampaio, S. R. Bornstein, M. Schroeder and A. Shevchenko, Genome Biol., 2011, 12, R8 CrossRef CAS PubMed.
- J. Lötvall, A. F. Hill, F. Hochberg, E. I. Buzás, D. Di Vizio, C. Gardiner, Y. S. Gho, I. V. Kurochkin, S. Mathivanan, P. Quesenberry, S. Sahoo, H. Tahara, M. H. Wauben, K. W. Witwer and C. Théry, J. Extracell. Vesicles, 2014, 3, 26913 CrossRef PubMed.
- G. V. Shelke, C. Lässer, Y. S. Gho and J. Lötvall, J. Extracell. Vesicles, 2014, 3, 24783 CrossRef PubMed.
- K. Aubertin, A. K. A. Silva, N. Luciani, A. Espinosa, A. Djemat, D. Charue, F. Gallet, O. Blanc-Brude and C. Wilhelm, Sci. Rep., 2016, 6, 35376 CrossRef CAS PubMed.
- F. Momen-Heravi, L. Balaj, S. Alian, P.-Y. Mantel, A. E. Halleck, A. J. Trachtenberg, C. E. Soria, S. Oquin, C. M. Bonebreak, E. Saracoglu, J. Skog and W. P. Kuo, Biol. Chem., 2013, 394, 1253–1262 CrossRef CAS PubMed.
- F. Momen-Heravi, L. Balaj, S. Alian, J. Tigges, V. Toxavidis, M. Ericsson, R. J. Distel, A. R. Ivanov, J. Skog and W. P. Kuo, Front. Physiol., 2012, 3, 354 Search PubMed.
- V. R. Minciacchi, A. Zijlstra, M. A. Rubin and D. Di Vizio, Prostate Cancer Prostatic Dis., 2017, 20, 251–258 CrossRef CAS PubMed.
- G. Kim, C. E. Yoo, M. Kim, H. J. Kang, D. Park, M. Lee and N. Huh, Bioconjugate Chem., 2012, 23, 2114–2120 CrossRef CAS PubMed.
- R. Linares, S. Tan, C. Gounou and A. R. Brisson, Methods Mol. Biol., 2017, 1545, 43–54 Search PubMed.
- E. Betzig, G. H. Patterson, R. Sougrat, O. W. Lindwasser, S. Olenych, J. S. Bonifacino, M. W. Davidson, J. Lippincott-Schwartz and H. F. Hess, Science, 2006, 313, 1642–1645 CrossRef CAS PubMed.
- M. J. Rust, M. Bates and X. Zhuang, Nat. Methods, 2006, 3, 793–795 CrossRef CAS PubMed.
- R. A. Dragovic, C. Gardiner, A. S. Brooks, D. S. Tannetta, D. J. P. Ferguson, P. Hole, B. Carr, C. W. G. Redman, A. L. Harris, P. J. Dobson, P. Harrison and I. L. Sargent, Nanomedicine, 2011, 7, 780–788 CrossRef CAS PubMed.
- L. Löf, T. Ebai, L. Dubois, L. Wik, K. G. Ronquist, O. Nolander, E. Lundin, O. Söderberg, U. Landegren and M. Kamali-Moghaddam, Sci. Rep., 2016, 6, 34358 CrossRef PubMed.
- A. Morales-Kastresana, B. Telford, T. A. Musich, K. McKinnon, C. Clayborne, Z. Braig, A. Rosner, T. Demberg, D. C. Watson, T. S. Karpova, G. J. Freeman, R. H. DeKruyff, G. N. Pavlakis, M. Terabe, M. Robert-Guroff, J. A. Berzofsky and J. C. Jones, Sci. Rep., 2017, 7, 1878 CrossRef PubMed.
- A. F. Hill, D. M. Pegtel, U. Lambertz, T. Leonardi, L. O'Driscoll, S. Pluchino, D. Ter-Ovanesyan and E. N. M. Nolte-'t Hoen, J. Extracell. Vesicles, 2013, 2, 22859 CrossRef PubMed.
- J. M. Street, P. E. Barran, C. L. Mackay, S. Weidt, C. Balmforth, T. S. Walsh, R. T. A. Chalmers, D. J. Webb and J. W. Dear, J. Transl. Med., 2012, 10, 5 CrossRef CAS PubMed.
- L. Palomo, E. Casal, F. Royo, D. Cabrera, S. Van-Liempd and J. M. Falcon-Perez, Front. Immunol., 2014, 5, 651 Search PubMed.
- O. P. B. Wiklander, J. Z. Nordin, A. O'Loughlin, Y. Gustafsson, G. Corso, I. Mäger, P. Vader, Y. Lee, H. Sork, Y. Seow, N. Heldring, L. Alvarez-Erviti, C. I. E. Smith, K. Le Blanc, P. Macchiarini, P. Jungebluth, M. J. A. Wood and S. El Andaloussi, J. Extracell. Vesicles, 2015, 4, 26316 CrossRef PubMed.
- M. L. Manca, C. Cencetti, P. Matricardi, I. Castangia, M. Zaru, O. D. Sales, A. Nacher, D. Valenti, A. M. Maccioni, A. M. Fadda and M. Manconi, Int. J. Pharm., 2016, 511, 198–204 CrossRef CAS PubMed.
- T. Smyth, M. Kullberg, N. Malik, P. Smith-Jones, M. W. Graner and T. J. Anchordoquy, J. Controlled Release, 2015, 199, 145–155 CrossRef CAS PubMed.
- C. P. Lai, O. Mardini, M. Ericsson, S. Prabhakar, C. A. Maguire, J. W. Chen, B. A. Tannous and X. O. Breakefield, ACS Nano, 2014, 8, 483–494 CrossRef CAS PubMed.
- J. Wang and M. M. Barr, Cell Mol Neurobiol, 2016, 36, 449–457 CrossRef CAS PubMed.
- N. Tominaga, N. Kosaka, M. Ono, T. Katsuda, Y. Yoshioka, K. Tamura, J. Lötvall, H. Nakagama and T. Ochiya, Nat. Commun., 2015, 6, 6716 CrossRef CAS PubMed.
- A. Bronisz, Y. Wang, M. O. Nowicki, P. Peruzzi, K. Ansari, D. Ogawa, L. Balaj, G. De Rienzo, M. Mineo, I. Nakano, M. C. Ostrowski, F. Hochberg, R. Weissleder, S. E. Lawler, E. A. Chiocca and J. Godlewski, Cancer Res., 2014, 74, 738–750 CrossRef CAS PubMed.
- K. M. Danielson, J. Estanislau, J. Tigges, V. Toxavidis, V. Camacho, E. J. Felton, J. Khoory, S. Kreimer, A. R. Ivanov, P.-Y. Mantel, J. Jones, P. Akuthota, S. Das and I. Ghiran, PLoS One, 2016, 11, e0144678 Search PubMed.
- E. Eitan, J. Green, M. Bodogai, N. A. Mode, R. Bæk, M. M. Jørgensen, D. W. Freeman, K. W. Witwer, A. B. Zonderman, A. Biragyn, M. P. Mattson, N. Noren Hooten and M. K. Evans, Sci. Rep., 2017, 7, 1342 CrossRef PubMed.
- H. Zhou, P. S. T. Yuen, T. Pisitkun, P. A. Gonzales, H. Yasuda, J. W. Dear, P. Gross, M. A. Knepper and R. A. Star, Kidney Int., 2006, 69, 1471–1476 CrossRef CAS PubMed.
- L. Musante, M. Saraswat, E. Duriez, B. Byrne, A. Ravidà, B. Domon and H. Holthofer, PLoS One, 2012, 7, e37279 CAS.
- X. Gallart-Palau, A. Serra, A. S. W. Wong, S. Sandin, M. K. P. Lai, C. P. Chen, O. L. Kon and S. K. Sze, Sci. Rep., 2015, 5, 14664 CrossRef CAS PubMed.
- C. Coman, F. A. Solari, A. Hentschel, A. Sickmann, R. P. Zahedi and R. Ahrends, Mol. Cell. Proteomics, 2016, 15, 1453–1466 CAS.
- S. Di Palma, D. Stange, M. van de Wetering, H. Clevers, A. J. R. Heck and S. Mohammed, J. Proteome Res., 2011, 10, 3814–3819 CrossRef CAS PubMed.
- M. Maurer, A. C. Müller, C. Wagner, M. L. Huber, E. L. Rudashevskaya, S. N. Wagner and K. L. Bennett, J. Proteome Res., 2013, 12, 1040–1048 CrossRef CAS PubMed.
- L. Giusti, P. Iacconi, F. Ciregia, G. Giannaccini, G. L. Donatini, F. Basolo, P. Miccoli, A. Pinchera and A. Lucacchini, J. Proteome Res., 2008, 7, 4079–4088 CrossRef CAS PubMed.
- N. A. Kulak, G. Pichler, I. Paron, N. Nagaraj and M. Mann, Nat. Methods, 2014, 11, 319–324 CrossRef CAS PubMed.
- C. Coman, F. A. Solari, A. Hentschel, A. Sickmann, R. P. Zahedi and R. Ahrends, Mol. Cell. Proteomics, 2016, 15, 1453–1466 CAS.
- J. Tisoncik-Go, D. J. Gasper, J. E. Kyle, A. J. Eisfeld, C. Selinger, M. Hatta, J. Morrison, M. J. Korth, E. M. Zink, Y.-M. Kim, A. A. Schepmoes, C. D. Nicora, S. O. Purvine, K. K. Weitz, X. Peng, R. R. Green, S. C. Tilton, B.-J. Webb-Robertson, K. M. Waters, T. O. Metz, R. D. Smith, Y. Kawaoka, M. Suresh, L. Josset and M. G. Katze, Cell Host Microbe, 2016, 19, 254–266 CAS.
- R. A. Quinn, J. A. Navas-Molina, E. R. Hyde, S. J. Song, Y. Vázquez-Baeza, G. Humphrey, J. Gaffney, J. J. Minich, A. V. Melnik, J. Herschend, J. DeReus, A. Durant, R. J. Dutton, M. Khosroheidari, C. Green, R. da Silva, P. C. Dorrestein and R. Knight, mSystems, 2016, 1, e00038–e00016 CrossRef PubMed.
Footnote |
† Equal contribution. |
|
This journal is © The Royal Society of Chemistry 2018 |
Click here to see how this site uses Cookies. View our privacy policy here.