DOI:
10.1039/C7AN01346A
(Critical Review)
Analyst, 2018,
143, 60-80
Recent advances in the use of microfluidic technologies for single cell analysis
Received
13th August 2017
, Accepted 25th October 2017
First published on 26th October 2017
Abstract
The inherent heterogeneity in cell populations has become of great interest and importance as analytical techniques have improved over the past decades. With the advent of personalized medicine, understanding the impact of this heterogeneity has become an important challenge for the research community. Many different microfluidic approaches with varying levels of throughput and resolution exist to study single cell activity. In this review, we take a broad view of the recent microfluidic developments in single cell analysis based on microwell, microchamber, and droplet platforms. We cover physical, chemical, and molecular biology approaches for cellular and molecular analysis including newly emerging genome-wide analysis.
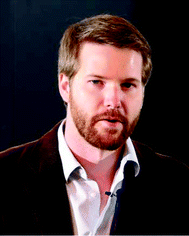 Travis W. Murphy | Travis Murphy received his B.S. in chemical engineering with honors from Virginia Tech in 2014. He is now a Ph.D. candidate in chemical engineering at Virginia Tech with Professor Chang Lu. His research interests include developing high-throughput microfluidic technologies as well as downstream integration and automation of microfluidic systems specifically in the area of epigenomic analysis. |
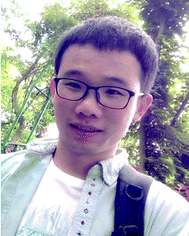 Qiang Zhang | Qiang Zhang is currently a PhD student in the Department of Chemical Engineering at Virginia Tech. He obtained his B.S. and M.S. from Qingdao University. His research interests include droplet microfluidics, bisulfite sequencing, and single-cell analysis. |
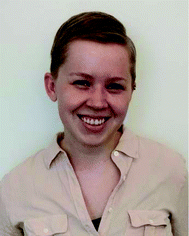 Lynette B. Naler | Lynette Naler is a graduate student in chemical engineering at Virginia Tech. Lynette obtained her B.S. in Chemical and Biomolecular Engineering and B.A. in Computer Science from Johns Hopkins University in 2014. She then spent two years as a research fellow at the National Institute on Aging. Lynette is currently a member of Prof. Chang Lu's group, where she is working on a microfluidic technology for the study of epigenetics using low-input samples. |
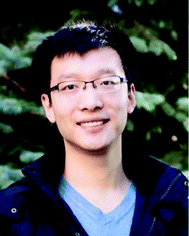 Sai Ma | Dr Sai Ma is a postdoctoral associate at Broad Institute of MIT and Harvard. He obtained his B.S. in Chemistry from Zhejiang University in 2011. He received his PhD in Biomedical Engineering from Virginia Tech in 2017 where his research in Dr Chang Lu's group focused on developing microfluidic technologies for comprehensive genomic and epigenomic analysis. These technologies have been used to study neurological disease and brain development. After completing his PhD, he joined Broad Institute and started working with Dr Jason Buenrostro and Dr Aviv Regev, where he currently focuses on the development of high-throughput biological tools to systematically understand single cells. |
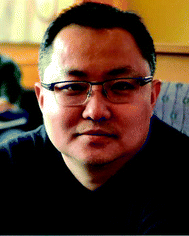 Chang Lu | Dr Chang Lu is a Fred W. Bull Professor of Chemical Engineering at Virginia Tech. Dr Lu obtained his B.S. in Chemistry with honors from Peking University in 1998 and his PhD in Chemical Engineering from University of Illinois at Urbana-Champaign in 2002. He then spent 2 years as a postdoctoral associate in Applied Physics of Cornell University. His research has been in the general area of development of microfluidic technologies for molecular manipulation and analysis. Dr Lu received a Wallace Coulter Foundation Early Career Award, a NSF CAREER Award, and a VT Dean award for research excellence, among a number of other honors. |
1. Introduction
Microfluidic technologies are able to easily improve sensitivity, reduce cost, and minimize operator influence in the operation of biological assays, and therefore have been widely applied to a number of different fields. Microfluidics has the advantage of working with picoliter to nanoliter volumes of solution that help to reduce sample loss and the cost of reagents. Additionally, they are highly automatable with the ability to be multiplexed to create high-throughput assays. These features of microfluidics make it an ideal platform to analyze the heterogeneity of single cells.1,2
Microfluidics takes different forms and shapes. There are two primary forms of microfluidics: channel microfluidics and droplet microfluidics. Channel microfluidic systems utilize microscale channels and chambers that allow for all flow to be in the laminar regime. The laminar flow allows for highly reproducible and well understood flow patterns within the microfluidic structures. These devices are typically manufactured using polydimethylsiloxane (PDMS), etched glass, or silicon which is then bonded to glass. Many channel microfluidic technologies make use of multi-layer soft lithography, which allows the use of a channel for sample flows and a layer that consists of valves to manipulate the sample flow through the use of an applied pressure.3,4 In contrast, droplet microfluidics utilizes the immiscibility of water and oil to create pico- and nanoliter scale droplet microreactors.5,6 The ease and speed of generation combined with a simple encapsulation of single cells by using a dilute suspension makes it the ideal high-throughput technology for single cell analysis.7,8 Individual droplets can be transported, merged, mixed, and divided using on-chip processes.5 Additionally, the generation of unique barcodes in single droplets makes pooling samples for data analysis much simpler.9–12 Digital microfluidics (DMF) is a subset of droplet microfluidics, also known as electrowetting on dielectric (EWOD), which is a different technological approach for developing lab-on-chip systems.13,14 EWOD systems are made up of separate surfaces that are able to change hydrophobicity when applied with an electric field. An array of these surfaces allow for the movement and manipulation of droplets of solutions.
Single cell analysis has been gaining attention and popularity in recent years. There is a known heterogeneity to exist within a population of seemingly identical cells.15,16 This is particularly important when primary cell samples from lab animals and patients are concerned. For this reason, it is important to study individual cells to understand the complex biology of the heterogeneous population. These minute differences in cellular activities could be essential in the development of personalized medicine and disease research. The ability to analyze a population of cells to isolate drug resistant cells for further analysis is one of the most important applications for developing effective therapeutic methods.17
The methods for single cell analysis are broad and include everything from measuring the physical properties of cells, to protein analysis, deciphering cell signaling, and DNA/RNA sequencing. Using these examinations it is possible to make previously unknown breakthroughs by looking at rare tumor cells such as circulating tumor cells.18,19 It can additionally be used to study cancer stem cells in order to understand the disease progression and make more effective chemotherapeutics.20,21
Earlier developments in single cell analysis began primarily with cytometric analysis of single cells, rapidly screening fluorescently labeled cells in a flow.22,23 As the field has evolved, microfluidics allowed for a much wider range of analysis that would not be economical or feasible using a traditional platform. For example, further developments in single cell proteomic analysis were achieved through the controlled breakage of single cells and further analysis of their contents.24,25
This review of microfluidic single cell analysis will cover recent developments, ranging from single cell sample collection to various examination methods (physical, chemical, and molecular/nucleic acid analyses). These works were conducted on various platforms that enabled high throughput (flow cytometry, microarrays, microwells, and droplets).
2. Single cell manipulation and isolation
The first step in any sort of single cell analysis is collecting single cell samples for examination. Conventional and commonly-used single cell isolation methods include serial dilution, fluorescence activated cell sorting (FACS), and laser capture microdissection (LCM). Serial dilution and micro-pipetting rely on manual micro-manipulation of single cells with the advantages of being cheap and easy to perform and the disadvantages of being labor-consuming and low throughput. They have been used to isolate cells for single cell culture26 and genomic analysis.27,28 Commercial FACS is an automatic single cell sorting and analysis system with high throughput and accuracy, which could sort or isolate single cells into tubes or microwells for downstream applications.29,30 LCM is another commercial system, which employs a laser to precisely cut and isolate specific single cells from tissues through direct microscopic visualization.31
Currently, various microfluidic systems have been developed for single cell isolation and analysis based on a variety of basic micro-manipulation techniques, including hydrodynamic cell traps, valve traps, electrical traps, optical traps, droplets, and microwells. Other techniques for single cell sorting exist, such as acoustic32–35 and magnetic sorting.36–39 These microfluidic methods have a number of inherent advantages over the conventional methods mentioned previously, such as downstream integration, automation, cost reduction, and a reduced risk of sample contamination.
2.1. Hydrodynamic cell traps
The most common method of collecting single cells for analysis is by using a hydrodynamic trap. A hydrodynamic cell trap is simply a system where the cell is removed from the flow of a cell suspension after being stopped by microscale structures (such as U-shaped structure) or hydrodynamic tweezers for examination. Hydrodynamic tweezers usually require specific and complex fluid flow profiles to trap single cells or particles40,41 and have been reviewed previously.42
U-shaped traps.
These cell traps consist of one or more positive U-shaped structures within a flow cell that capture single cells from a bulk solution. One of the earliest single cell U-shaped traps was demonstrated by Di Carlo et al. for isolating about 100 individual HeLa cells to observe their growth and adherence within 24 h (Fig. 1A).43 This type of U-shaped structure has also been utilized to perform fluorescence analysis of intracellular calcium of a single cardiac myocyte44 and study single cell enzyme kinetics for HeLa, 293T, and Jurkat cells.45 U-shaped traps have been further modified by adding small drainage channels into the capture structure, which would allow the fluid to flow through before trapping a cell.46,47 Compared to the unmodified U-shaped traps, it was reported that this improvement helps reduce the amount of stress placed on the cell, to enable the analysis of more fragile cells.46 In order to reduce the number of empty structures without compromising on loading time, Chung et al.48 and Yesilkoy et al.49 designed a hydrodynamic trap array that splits the flow cell into individual channels, and by utilizing channel focusing with small capture structures with drainage channels, was able to quickly trap single cells using only gravity driven flow, ultimately achieving ∼70% trapping efficiency and >90% fill factors. Recently, Zhang et al. integrated U-shaped traps into a hand-held single cell pipet (hSCP) device for rapid and highly-efficient isolation of single cells from cell suspensions into well plates, Petri dishes, or tubes for further analysis.50
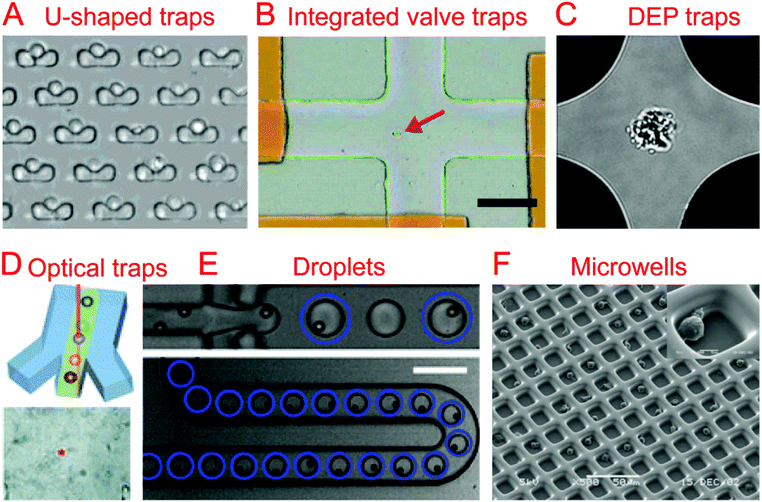 |
| Fig. 1 Microfluidics-based single-cell isolation methods. (A) U-shaped traps. Reprinted with permission from D. D. Carlo et al., Anal. Chem., 2006, 78, 4925–4930. Copyright 2006 American Chemical Society. (B) Integrated valve traps. Reprinted with permission from J. W. Hong et al., Nat. Biotechnol., 2004, 22, 435–439. Copyright 2004 Macmillan Publishers Ltd. (C) DEP traps. Reprinted with permission from U. C. Schroder et al., Anal. Chem., 2013, 85, 10717–10724. Copyright 2013 American Chemical Society. (D) Optical traps. Reprinted with permission from A. Y. Lau et al., Lab Chip, 2008, 8, 1116–1120. Copyright 2008 The Royal Society of Chemistry. (E) Droplets. Reprinted with permission from J. F. Edd et al., Lab Chip, 2008, 8, 1262–1264. Copyright 2008 The Royal Society of Chemistry. (F) Microwells. Reprinted with permission from A. Revzin et al., Lab Chip, 2005, 5, 30–37. Copyright 2005 The Royal Society of Chemistry. | |
Bypass-channel traps.
While the drainage channels are parallel to the main flow channel in U-shaped traps, bypass-channel traps usually consist of bypass channels with drainage channels perpendicular to the main flow channel. This allows them to not only trap single cells at the bypass channels, but to also perform physical manipulation of the cell once it enters the capture structure. Yamaguchi et al. demonstrate a microfluidic system consisting of a parallel capture channel and a buffer channel to capture multiple single cells for analysis and culture.51 The system used a micro-pocketed main channel with drainage channels in each micro-pocket connected to the buffer channel. The system was able to capture and release cells based on the difference between flow rates of the capture and buffer channels. Similar structures have been developed to enable high-efficiency single cell trapping.52–54 Chung et al. further optimized the geometry structures of the traps and the hydrodynamic resistances of the channels, achieving the trapping of 4000 single cells in 4.5 mm2 within 30 seconds as well as with high efficiency (>95%).55 Hong et al. integrated this cell trap into a microfluidic cell co-culture platform in which each trapping unit could sequentially capture two different cells via changing fluidic resistance. Using this platform, the interaction between mouse embryonic fibroblasts (MEF) and mouse embryonic stem cells (mESC) at the single cell level has been demonstrated.56
2.2. Integrated valve traps
Trap structures can come from the top or bottom of microchannels, such as traps supplied by integrated valves (i.e. Quake valves). Hong et al. integrated parallel valves into a microfluidic chip to achieve the steps from cell isolation to purified DNA recovery, in which single cells were trapped at the closed intersection of two microchannels (Fig. 1B).57 Ottesen et al. further developed a microfluidic digital PCR chip integrated with 1176 parallel chambers, and these chambers could be individually isolated by closing valves to trap single cells for performing independent PCR.58 These integrated valve traps can be used to perform multiple on chip processes, from single cell isolation to single cell lysis. It can also include different kinds of single cell analyses, such as Multiple Displacement Amplification (MDA),59–61 digital PCR (dPCR),62 whole-transcriptome analysis,63 and protein analysis.64
2.3. Electrical traps
In the case when a cell is very sensitive to physical stresses placed on the membrane, electrical methods of cell capture can be utilized. Toriello et al. developed a microfluidic system that consisted of a flow cell with a series of gold electrodes throughout. The gold electrodes were covered in an oxide layer, leaving an array of 16 μm2 exposed gold pads, which were capable of binding to the single cells flowed through the system that are labeled with a thiol functional group.65
In order to electrically capture cells without the use of cell labeling, it becomes necessary to utilize the dielectrophoretic (DEP) force that is generated when a nonuniform electric field acts on a particle. Das et al. demonstrated that DEP electrodes coated on a microscope slide could trap different types of cells directly from a cell suspension on the slide.66 In terms of single cell trapping, Jaeger et al. employed negative DEP (nDEP) to trap a single yeast cell without contact in an electric field cage for a single cell proliferation study.67 Similarly, Thomas et al. developed concentric ring nDEP traps to hold the selected cell in place while repelling other cells in the flow system.68 DEP traps have been further coupled with downstream cell detection methods. Single cell Raman spectra (SCRS) could be obtained by employing DEP to trap specific single cells for Raman detection (Fig. 1C).69–71 In addition, immobilizing a single HeLa cell by DEP for impedance measurement has been demonstrated by Chen et al. using DEP as a medium for both liquid manipulation and cell capture on a digital microfluidic device.72
2.4. Optical traps
Optical tweezers, formed by a highly focused laser beam, offer a contactless trapping force able to hold a single cell in a fluidic medium. Xie et al. demonstrated the trapping of single living red blood cells, yeast cells, and bacterial cells using low-power optical tweezers for Raman spectroscopy acquisition.73,74 Furthermore, optical tweezers have been integrated into microfluidic systems to allow label-free identification and sorting of single cells. Specific single cells were first identified using Raman spectra and then trapped and moved from the cell suspension (Fig. 1D).75–77 A combination of optical tweezers and DEP, termed optoelectronic tweezers (OET), has been developed to manipulate single cells.78 Huang et al. designed an optoelectronic tweezer based microfluidic system that can optically select a specific cell from the population and move it to a separate channel. Once all of the channels are loaded with single cells, the cells can be flushed off chip for further analysis.79
2.5. Droplets
Droplet technologies are becoming increasingly popular with their ability to quickly and cheaply encapsulate single cells and provide an ideal micro-reactor.7,8 Single cell capture technologies for droplet systems typically consist of generating droplets from a statistically dilute suspension of cells.80 With the advantage of high throughput, droplets have been utilized to perform a variety of biological assays at the single cell level, including single cell cultivation,81–83 electroporation,84 antibody detection,85 enzyme screening,86,87 drug screening,88 PCR,89,90 RNA sequencing (RNA-seq),9,11 whole-genome amplification,91,92 and chromatin immunoprecipitation for sequencing (ChIP-seq).10
The difficulty that arises from using a statistically dilute suspension of cells is the inherent variability of the droplet contents. In order to produce high quality single cell droplet studies it is important to maximize the number of droplets containing a single cell, while minimizing empty droplets and droplets containing multiple cells. Passive methods relying on optimized channel designs have been developed to improve the rate of single cell droplets in all generated droplets.93–96 Chabert et al. presented a microfluidic device for single cell droplet encapsulation and hydrodynamic sorting for high reliability. The system took advantage of the Rayleigh-Plateau instability in a flow constriction to form the droplets, where they were sorted by hydrodynamic forces. The system was capable of sorting 70–80% of the encapsulated cells with low incidence of multiple cell encapsulation and even fewer empty droplets. If integrated with other droplet technologies, this system could improve the quality of data while reducing the operating cost per cell.95 Another method to reduce the variability of single cell encapsulation in droplet based systems is to improve the efficiency of single cell loading. Edd et al. demonstrated a microfluidic system capable of altering the single cell loading efficiency of generated droplets by utilizing inertial sorting to generate two trains of single cells in the loading channel (Fig. 1E). This self-organization of cells allows for an almost doubled fraction of droplets containing single cells when compared to a Poisson distribution.96 Kemna et al. introduced Dean forces to order cells in microchannels before being encapsulated into droplets. With the aid of the cell ordering, ∼80% of the formed droplets contain single cells without compromising cell viability.94
Active methods to obtain single cell droplets are based on sorting single cell droplets from other generated droplets. We developed a droplet sorting platform that integrated a fluorescence signal processing system and a solenoid valve, which could identify the number of cells in an individual droplet and sort droplets containing a specific number of cells.97 This platform demonstrated high efficiency and accuracy for sorting droplets with fluorescent beads or lightly stained cells. Similarly, Wu et al. demonstrated an active droplet sorter which used hydrodynamic gated injection that was formed by a pressure difference among different microchannels. A single cell droplet rate of 94.1% has been obtained after droplet sorting.98 Recently, Zhang et al. presented a droplet microfluidic platform that prepared single cell samples of the type of one-cell-in-one-tube. This platform included cell-encapsulated droplet generation, single cell droplet manual identification, sorting by solenoid valve suction force, and dispensing from a microfluidic chip into collection tubes via a capillary embedded in the microchannel.99 Single cell analyses including cultivation, real-time quantitative PCR, reverse transcription PCR, and MDA for sequencing have been demonstrated based on single cell samples prepared by this platform.
2.6. Microwells
Single cell isolation through the use of microwells uses either a specially sized well, a modified surface, and/or a statistically dilute suspension of cells to seed single cell microwells. Relying on microfabrication technology, parallel microwells can be easily made and enable high-throughput single cell analysis.100 Chin et al. demonstrated the fabrication of ∼10
000 SU-8 microwells on a glass coverslip and their application for long-term and quantitative analysis of single cell stem cell proliferation.101 The strategies of cell recovery from microwells have been further developed in other studies.102–104 Guo et al. developed an array of conical nanopores integrated in a microfluidic device that could not only selectively trap and isolate cyanobacteria from a mixed population containing chlamydomonas, but also release the trapped bacteria when the flow was inverted.103 In order to improve single cell capture efficiency, Faenza et al. developed a microwell platform coupled with DEP, in which DEP would provide focusing and patterning of single cells in microwells. Deterministic, not statistical, patterning of cells was used in this platform, achieving a high cell trapping yield (>83%).105 Huang et al. demonstrated the use of reverse conical microwells in conjunction with the application of a centrifugal force, which allowed for 90% occupancy in only a few seconds.106 In addition to single cell trapping function, microwells could also act as microreactors for further single cell examination.107,108
Surface modification has been utilized for cell trapping without complicated fabrication of cell-sized microwells.109–111 Lin et al. made an array of hydrophilic patterns on a hydrophobic glass substrate, which would rapidly form discrete aqueous droplets bearing seeded single Escherichia coli bacterial cells.109 These cell-seeded aqueous droplets have been further used as reaction chambers for real-time PCR of single cells.111 By combining the use of a surface modification with specifically sized micro-wells, it is possible to improve the efficiency and have a more defined geometry. Revzin et al. reported a polyethylene glycol (PEG) microwell array modified with a T-cell specific anti-CD5 antibody to immobilize single T-lymphocytes, achieving 95% occupancy (Fig. 1F).112 Similarly, Chen et al. developed a microfluidic microwell array coated with a cell specific aptamer that was capable of isolating and capturing single cells from a mixture.113 Lin et al. combined surface modification with hydrodynamic cell traps to adhere cells to a protein micro-pattern in a controlled manner. The use of a removable hydrodynamic trap allowed for controllable paired positioning of cells at a higher frequency than random seeding would allow.114
3. Physical analysis
3.1. Deformability
Many techniques that differentiate between cells of different types, phenotypes, and cell states require the use of fluorescent markers or antibodies, which can change the physiology of the single cell. For this reason, it is useful to study the mechanical properties of the membrane for differentiating cell states. A variety of microfluidic devices and perturbation techniques have been developed to characterize the deformability of cells and have been summarized previously,115 including a constriction channel,116–118 fluid stress,118,119 an optical stretcher,120,121 electro-deformation,122,123 electroporation,124 and microfluidic pipette aspiration.125,126
Lincoln et al. demonstrated a deformability-based flow cytometry system by coupling an optical stretcher with a microfluidic device, which achieved classification of red blood cells (RBCs) and polymorphonuclear cells (PMNs) based on their optical deformability.127 A biophysical flow cytometry technique has been developed by Rosenbluth et al. to detect differences between normal and aberrant blood cells based on their transit times flowing through microfluidic capillary microchannels.128 Similarly, Gabriele et al. developed a microfluidic device that consisted of a parallel analysis channel and a bypass channel.129 The analysis channel contained a series of constrictions and expansions which could differentiate cells by the amount of time it took to pass through the constriction structure. Hur et al. developed a microfluidic system capable of separation of single cells based on their deformability without the use of markers.130 The deformability could be indirectly quantified by studying the equilibrium position of the cells in a focusing channel before they were separated into different populations. This approach could be applied at high throughput and is of great use when differentiating phenotypically similar cells with different deformabilities.
In order to better characterize the mechanical deformability of single cells it becomes important to induce greater shear stress in the system. Gossett et al. described a microfluidic system capable of high-throughput analysis of single cell deformability.131 Using a cross shaped channel and driving high flow rates from opposite sides, single cells were deformed at great strain rates. The deformation was observed and analyzed allowing for the markerless classification of single cells into different disease states. Tse et al. utilized their previous microfluidic stretching system to also perform high-throughput markerless classification of single cells (Fig. 2A).132 The system was able to differentiate between various cell types with high confidence. This was useful in differentiating malignant cancer cells from a population. Improving upon their previous work, Dudani et al. developed a microfluidic hydrodynamic cell stretching platform which was able to perform two different stretching measurements at different strain rates.133 This provided complementary data sets for the more accurate classification of mechanical phenotypes.
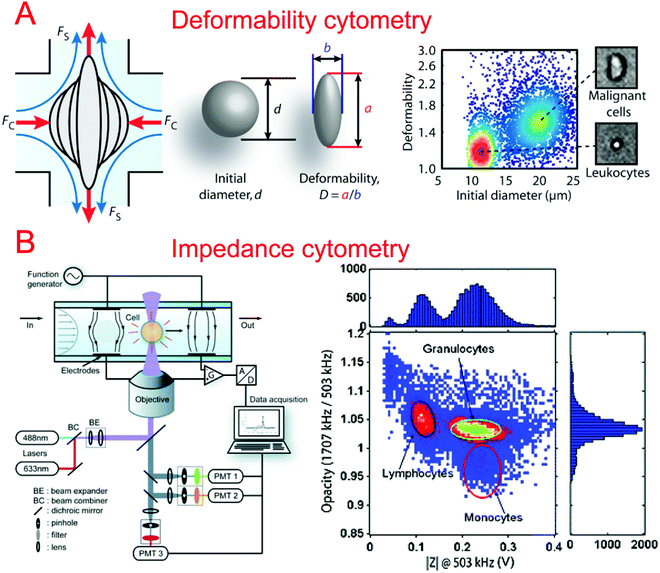 |
| Fig. 2 Microfluidics-based physical analysis of single cells. (A) Single-cell deformability cytometry. Reprinted with permission from H. T. K. Tse et al., Sci. Transl. Med., 2013, 5, 212ra163–212ra163. Copyright 2013 The American Association for the Advancement of Science. (B) Single-cell impedance cytometry. Reprinted with permission from D. Holmes et al., Lab Chip, 2009, 9, 2881–2889. Copyright 2009 The Royal Society of Chemistry. | |
We developed a microfluidics-based electroporative flow cytometry (EFC) platform to capture swelling of cells during electroporation, which provided a new method to study single-cell deformability/biomechanics.124 Otto et al. demonstrated a real-time deformability cytometry technique without using shear stresses and pressure gradients.134 Instead, a microchannel constriction induced cell deformation, which was detected by a CMOS camera for continuous and real-time image acquisition. The concept of studying mechanical deformability can be applied further to non-mammalian cell types. Lei et al. developed a flow focusing microfluidic device capable of classifying microalgae into different phenotypes based on mechanical and cytometric analysis under a multitude of on-chip culture conditions.135
3.2. Impedance
Impedance cytometry is an invaluable tool in differentiating cells in a clinical setting. The ability to produce highly automated systems for differentiating important cell types can have a positive effect on point-of-care medicine. Sohn et al. reported a capacitance cytometry that could measure the capacitance of cells as they flowed through the electrodes one by one.136 They found a linear relationship between the DNA content and the capacitance of a eukaryotic cell. Gawad et al. developed a coplanar electrode-based microfluidic device for impedance measurements of single cells or particles with different sizes or types.137 During their study, they also compared three types of electrode geometries using simulations. Single cell and particle impedance cytometry has experienced rapid growth in recent years (Fig. 2B).138–142 New developments have focused on implementing rapid and high-throughput examination,143–145 high sensitivity,143,146–148 multi-modal measurements,149–151 and clinical field applications.152–159 Bashir's group has demonstrated a series of studies in HIV/AIDS diagnostics based on microfluidic impedance cell counting.160,161 Ang et al. described a microfluidic flow cytometer with high-sensitivity graphene transistors capable of sensing malaria infected red blood cells.153 The flow cell contained a graphene transistor functionalized with CD36 receptors to selectively capture malaria infected cells. The conductance was then measured before the cell was released. The system was able to differentiate between two different phases of malaria infection with high confidence. Islam et al. developed a method of detection and electrical profiling of single tumor cells from whole blood in a microfluidic device.154 Tumor cells were found to have a higher translocation time when using nanotextured channels while most blood cells were largely unaffected. Based on translocation time and peak amplitude of the electrical measurements, cancer cells were easily identified from whole blood. Hong et al. demonstrated a microfluidic trap-and-measure device capable of performing single-cell electrical impedance spectroscopy in order to differentiate cell types using different voltages.156 Using their system, they were able to distinguish between 4 different cell types and cancer pathological states. Similarly, Zhou et al. described hydrodynamic trapping of single cells and the use of electrodes for impedance spectroscopy.155 They could quantitatively analyze the differentiation of mESCs at different time points. This system could also be adapted to study dynamic changes in the properties of cells over a long period of time.
3.3. Migration
The study of cell migration dynamics is crucial in understanding invasive and malignant diseases such as cancer. Zheng et al. demonstrated a microfluidic platform for cell migration analysis at single cell resolution.162 The system utilized large micromechanical valves inside a channel, which when actuated created a cell free area after the channel was loaded with cells. When the valve was opened, the system could be used to study the migration of the cells into these open areas. Unfortunately, cells’ in vivo migration in three dimensions could not be measured using Zheng's device. To study this, Nguyen et al. developed a multiplexed microfluidic system for electrical cell–substrate impedance sensing.163 The system was able to investigate 2D and 3D cell migration in addition to identifying cellular activities using electrical parameters on a single cell level. In order to study the competing migration between two different cell species, Zheng et al. developed a microfluidic system for co-culture of two cell types with 16 parallel cultures.162 The system had a valve separating the two cell cultures. When the physical separation between the populations was removed, this allowed for migration and infiltration studies.
3.4. Other physical parameters
Exploring the physical characteristics such as the shape and volume of cells, Lee et al. utilized their quartz nanopillar device to isolate single circulating tumor cells from whole blood.164 The cells were then examined using laser scanning cytometry to determine cell shape or circularity. Riordon et al. developed a microfluidic device to measure the volume of a single cell during cell growth in suspension by controllably passing the cell back and forth over a set of electrodes.165 Investigating the weight of intracellular components, Olcum et al. demonstrated a microfluidic system capable of weighing nanoparticles.166 This was applied to single cell analysis of an exome self-assembled nanoparticle mass which could be used to characterize cell types.
4. Chemical analysis
4.1. Electrophoresis
Capillary electrophoresis (CE) coupled with laser-induced fluorescence (LIF) has been widely used to analyze cellular contents.167–169 McClain et al. integrated CE into a microfluidic device, in which single cells were lysed at the lysis intersection and the cell lysate was separated in the channel by the application of a high voltage.170 The fluorescently labeled cellular contents subsequently underwent LIF detection. We further optimized the chip design to obtain different field intensities at the lysis124,171 and electrophoresis sections, which could alleviate local Joule heating caused by high field intensity (Fig. 3A).172 Instead of electrical lysis, Phillips et al. integrated laser-generated cavitation bubble based cell lysis into a microfluidic electrophoresis chip.173 The device was able to achieve robust and continuous single cell content analysis. Instead of LIF, chemiluminescence detection (CL) was coupled with microchip electrophoresis to detect glutathione (GSH) in single human red blood cells with about 100 times greater sensitivity.174 In addition, western blotting has also been coupled with CE to allow multiplexed protein detection.175 A high throughput of 38 cells per minute has been achieved by introducing sheath-flow streams to focus labeled cells.176 This was used to analyze reduced GSH and reactive oxygen species in single erythrocytes. Cells trapped in microwells have also been subjected to automated and high-throughput CE analysis.177,178 Microfluidic CE has also been used for the analysis of neurotransmitters in single rat pheochromocytoma (PC 12) cells179 and peptidase activity in single tumor cells,180 in addition to the analysis of small-molecule metabolites,181 sphingosine kinase activity,182 and Akt activity in single cells.183
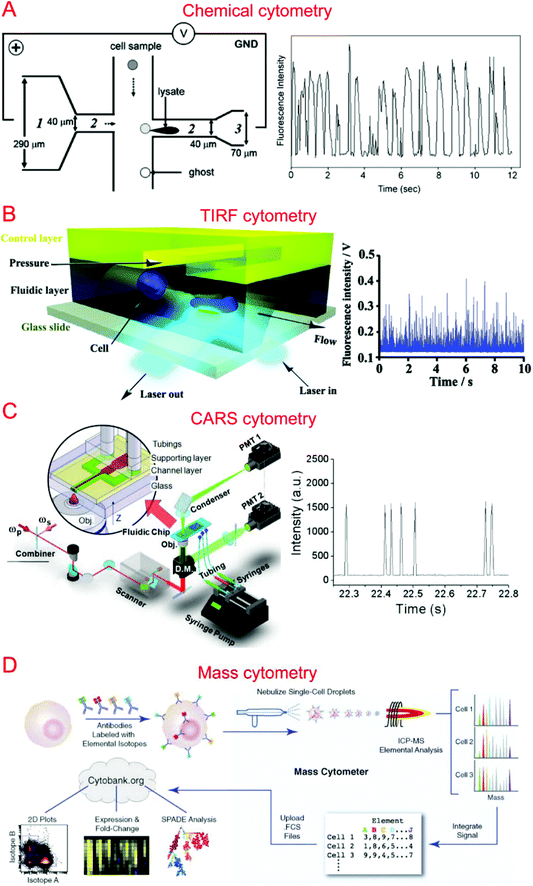 |
| Fig. 3 Microfluidics-based chemical analysis of single cells. (A) Chemical cytometry. Reprinted with permission from H.-Y. Wang et al., Chem. Commun., 2006, 33, 3528–3530. Copyright 2006 The Royal Society of Chemistry. (B) TIRF (Total Internal Reflection Fluorescence) flow cytometry. Reprinted with permission from J. Wang et al., Anal. Chem., 2008, 80, 9840–9844. Copyright 2008 American Chemical Society. (C) CARS (Coherent Anti-Stokes Raman Spectroscopy) cytometry. Reprinted with permission from H.-W. Wang et al., Opt. Express, 2008, 16, 5782–5789. Copyright 2008 Optical Society of America. (D) Mass cytometry. Reprinted with permission from S. C. Bendall et al., Science, 2011, 332, 687–696. Copyright 2011 The American Association for the Advancement of Science. | |
4.2. Flow cytometry
Flow cytometry based on detecting fluorescence emitted from flowing single cells has been widely used to study single cell intracellular calcium,44,184,185 proteins and cytokines,22,186,187 DNA content,188 and apoptosis.189,190 We have developed specialized flow cytometric technologies on microfluidic platforms for applications other than quantification of fluorescent species. For instance, we combined electroporation with flow cytometry, denoted Electroporative Flow Cytometry (EFC), in a microfluidic device to study the translocation of a protein-tyrosine kinase to the plasma membrane in B cells.191 EFC took advantage of the fact that the rate of electroporation-based release of intracellular molecules depends on their subcellular locations (e.g. a protein in the nucleus is harder to release than the same protein of cytosolic localization during electroporation, which breaches the plasma membrane). Thus the protein subcellular localization quantitatively correlated with the amount of protein loss during electroporation (or other release methods). Other than examining translocation to the cell membrane, we also used it to study NF-κB nucleocytoplasmic transport at the single cell level.187,192 We also developed total internal reflection fluorescence flow cytometry (TIRF-FC) which could be utilized to quantitatively examine events at the plasma membrane region by using a TIRF setup and a microfluidic constriction region where cells were forced to be in close contact with the substrate surface (Fig. 3B).193 TIRF-FC was run at a throughput of ∼100–150 cells per second and used to detect protein translocations194 and examine endocytosis-based uptake of quantum dots into cells.195
Baumgarth et al. reported a multicolor flow cytometer, which could detect eleven colors of fluorescence plus two scattered light parameters on each single cell.196 Krutzik et al. developed a fluorescent cell barcoding (FCB) technique which enabled cells to be labeled with multiple fluorophores of different intensities.22 This allowed them to achieve high-throughput flow cytometry while measuring fifteen or more fluorescence parameters of single cells.23
The first microfabricated fluorescence-activated cell sorter (μFACS) was developed by Fu et al.197 Miniaturized flow cytometry has been integrated in a microfluidic device by Kruger et al. for FACS.198 Since then, different kinds of microfluidic techniques have been used to separate target cells after being identified by fluorescence. For instance, Holmes et al. demonstrated a high-speed microparticle sorting microfluidic platform that employed DEP to deflect fluorescent particles in the microchannel.199 Wang et al. utilized the optical force for sorting of HeLa cells expressing a fluorescent protein to achieve fluorescence-activated microfluidic cell sorting.200 Chiou's group developed a pulsed laser triggered fluorescence activated cell sorter (PLACS), which could achieve a throughput of 20
000 cells per s with 37% sorting purity and >90% purity at a throughput of 1500 cells per s.201 Hydrodynamic switching originating from pneumatic microvalves,202 off-chip valves,203 or piezoelectric (PZT) actuators204 has also been employed to isolate single cells after fluorescence detection.
4.3. Raman spectroscopy
Raman spectroscopy that provides information on chemical signature is a valuable tool for the analysis of single cells. Single cell Raman spectroscopy (SCRS) provides a wealth of information for the classification of cell types,205–208 physiological states,209–212 and environmental conditions.213–215 These have been reviewed previously.216,217
By integrating SCRS-based analysis into microfluidic systems, Raman cell counting, Raman flow cytometry, and Raman-activated cell sorting (RACS) have been developed. Li et al. showed that 12C- and 13C-cyanobacterial cells could be counted in a microfluidic device by using resonance Raman spectra to improve the weak spontaneous Raman signal of single cells.218 Nolan et al. pioneered the method of surface-enhanced Raman spectroscopy (SERS) flow cytometry.219,220 For instance, Sebba et al. developed a high-throughput SERS flow cytometry technique with nanoparticles labeled with one of three chromophores: oxazine 170, thionin, or malachite green.220 This allowed for SERS detection within submillisecond time. Collaborating with Ji-xin Cheng's group, we incorporated laser-scanning coherent anti-Stokes Raman spectroscopy (CARS) detection into a microfluidic device (i.e. microfluidic CARS flow cytometry).221 We demonstrated its use to measure the size distribution of adipocytes harvested from mice fat tissue (Fig. 3C).221 Subsequent works coupled multiplexed CARS with flow cytometry to reach a large spectral range by exciting multiple Raman transitions.222,223 Stimulated Raman spectroscopy (SRS) could significantly improve Raman detection sensitivity. SRS flow cytometry allowed 200
000 Raman spectra to be acquired within one second and the throughput could achieve 11
000 particles per second.224 Using SRS flow cytometry, Zhang et al. have demonstrated the classification of 3T3-L1 cells based on their differentiation states.224
Other than Raman flow cytometry, RACS has also been developed to identify and isolate target cells from a cell suspension based on their Raman spectra.71,75,76,225 Lau et al. developed an optofluidic RACS platform by using optical tweezers to trap single cells for the acquisition of Raman spectra before moving the cell of interest into a collection channel.76 Zhang et al. reported a more automated RACS system with cell sorting of higher throughput, in which they used positive dielectrophoresis (pDEP) to trap single cells for Raman acquisition.71 The target cells were then isolated in a collection chamber using solenoid valve suction.
4.4. Mass spectroscopy
Different types of mass spectroscopy have been used in the field of single cell analysis, including single probe mass spectrometry,226 matrix-assisted laser desorption/ionization mass spectrometry (MALDI-MS),227,228 secondary ion mass spectrometry (SIMS),229 electrospray ionization mass spectrometry (ESI-MS),230 and combined methodologies.231 Utilizing microfluidic technology, a high-density microarray for mass spectrometry (MAMS) was produced by Urban et al. for single cell analysis using MALDI-MS. Furthermore, Xie et al. utilized a microfluidic frame and a microporous membrane to selectively place single cells in a microwell array on an indium tin oxide coated glass slide. The array of cells were processed using MALDI-MS to study phospholipids in the single cells.232 In addition, Mellors et al. demonstrated a microfluidic system integrating capillary electrophoresis and electrospray ionization-mass spectrometry.233 The system electrically lysed single cells, separated the cellular contents in the electrophoretic channel and passed the separated contents to the electrospray emitter to be characterized by mass spectrometry.233 Using inkjet single cell printing, Chen et al. were able to profile lipid fingerprints of single cells using probe electrospray ionization mass spectrometry.234
In the past decade, single cell mass cytometry, or cytometry by time of flight (CyTOF), has seen dramatic improvement for multi-parameter analysis of cells.235–238 This technique was first reported by Bandura et al., who used an inductively coupled plasma time-of-flight mass spectrometry to conduct the simultaneous measurement of 20 antigens in single cells of leukemia cell lines and leukemia patient samples.239 Recently, Porpiglia et al. reported that CyTOF could simultaneously measure up to 50 parameters in single cell analysis.240 Using time-of-flight secondary ion mass spectrometry with a single cell array, Huang et al. were able to study phenotypic alterations through cell apoptosis.241 CyTOF has been widely used in single cell analysis by Nolan's group (Fig. 3D)242–244 as well as other groups.240,245
5. Molecular biology analysis
5.1. Immunoassays
Microwells.
Microwell-based single cell immunoassay was a microengraving-based method first developed by Love et al.246 After loading cells into a microwell array engraved on a PDMS slab, the array was bound to a slide of glass coated with antigen. The primary antibody produced by the cells was captured onto the substrate and a fluorescently labeled secondary antibody was added. The secondary antibody targeted the primary antibody and indicated the amount of antigen-specific antibody secreted by single cells. This method was used to characterize the antibodies produced by primary B cells in terms of specificity, isotype, and apparent affinity at the single cell level.247 The cytokine secretion from single cells has also been analyzed.204,248–250 Combined with the cellular barcoding technique, the microengraving method achieved the simultaneous analysis of thousands of single cells.251
Herr's group developed a microwell-based single cell western blotting method that detected single cell proteins with high specificity.252–254 The method integrated the multiple processes of single cell loading in microwells, on-chip lysis, gel electrophoresis, blotting, and antibody probing. Whole cell imaging was further incorporated into single cell western blotting to demonstrate simultaneous phenotypic and proteomic analysis of single cells.255
Microchambers.
Eyer et al. integrated enzyme-linked immunosorbent assays (ELISA) into a microchamber. This allowed for single cell capture, lysis, and analysis of proteins, secondary messengers, or metabolites. The system has been demonstrated to quantify the enzyme GAPDH in single U937 cells and HEK 293 cells.256 Sun et al. demonstrated a microfluidic cell array chip for immunocytochemistry, termed microfluidic image cytometry (MIC). They achieved multiparametric single cell measurements (simultaneous quantification of four proteins).257,258 There were many other methods for analyzing protein expression259 and spatial distribution260 in single cells using fluorescence.
Protein barcodes are multiple strips of antibodies capable of capturing specific proteins. When coupled with fluorescence detection, they provided a simple method for quantifying proteins for a number of loci. A single-cell barcode chip was developed by Heath's group for quantitative and multiplexed analysis of intracellular proteins from single cells isolated in microchambers containing an antibody barcode array. Shin et al. first developed a method for patterning microfluidic platforms using DNA microarrays which were subsequently converted into antibody arrays for protein capture and quantification.261 Using this method, they were able to develop a microfluidic system for capturing single cells and profiling their protein secretions.262 Lu et al. expanded upon previous work, developing a highly paralleled protein barcode assay for secretomic analysis of single cells.263 Using 14-protein barcodes in a microchamber array (>5000 microchambers) allowed for the simultaneous analysis of thousands of single cells.263 Elitas et al. further improved the system to allow for the detection of up to 16 different proteins.264 Finally, Lu et al. expanded upon their barcode secretomic analysis platform by multiplexing the protein barcodes with multiple fluorescence signals.265 This enabled analysis of up to 45 different proteins at the single cell level in a highly multiplexed assay (Fig. 4A). Many groups have demonstrated the use of protein barcoding for high-throughput single cell protein analysis.266 Shi et al. developed a microfluidic system for profiling signaling pathways that integrated single cell chambers and a protein detecting antibody barcode, which could detect and quantify nine proteins released from lysed single cells.64 Ma et al. demonstrated an assay for high-throughput secretomic analysis at the single cell level. The system used antibody barcodes in over 1000 microchambers and allowed for the simultaneous analysis of up to 10 proteins.267 Deng et al. created a microfluidic system to isolate circulating tumor cells (CTCs) from whole blood using ssDNA encoded antibodies to absorb CTCs. These complexes were then captured on a functionalized surface in the microfluidic device. After purification, the CTCs were loaded onto a single cell barcode chip for fluorescent protein analysis.53
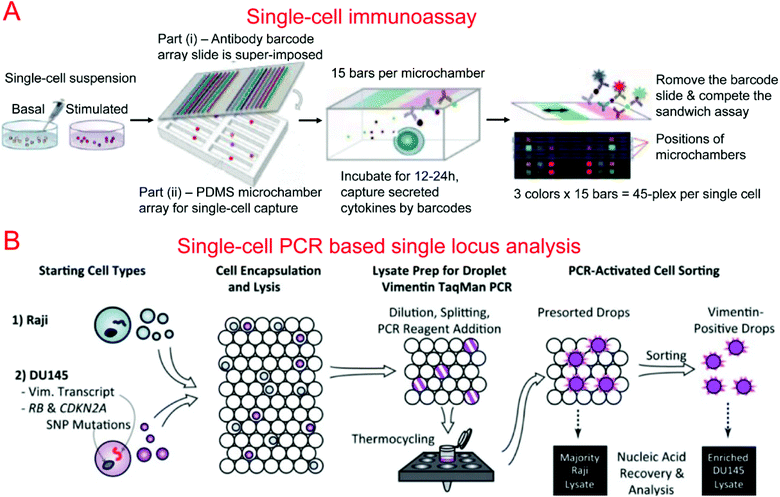 |
| Fig. 4 Single cell analysis based on immunoassays and PCR. (A) Single-cell immunoassay. Reprinted with permission from Y. Lu et al., Proc. Natl. Acad. Sci. U. S. A., 2015, 112, E607–E615. Copyright 2015 National Academy of Sciences. (B) PCR-based single-locus analysis. Reprinted with permission from D. J. Eastburn et al., Nucleic Acids Res., 2014, 42, e128. Copyright 2014 Oxford University Press. | |
Droplets.
There are a number of reports of immunoassays performed in microfluidic droplets. Konry et al. demonstrated a droplet-based approach to detect protein secretions by co-encapsulating secreting T cells with capture-antibody-modified microspheres and a fluorescently labeled secondary antibody. Using this method, IL-10 cytokine secretion in single CD4 + CD25+ regulatory T cells has been detected.268 Akbari et al. developed a droplet-based heterogeneous single cell immunoassay that could be used to screen cells producing antigen-specific antibodies at the single cell level.269 By using digital microfluidics, Ng et al. created an automated system for cell culture, stimulation, and immunochemistry study of single cells. The digital microfluidic system allowed for very short pulsing of stimuli and subsequent examination of the phosphorylation state of platelet-derived growth factor and the signaling protein Akt.270
5.2. PCR-based single-locus analysis
PCR-based assays target specific genes in the single cell transcriptome, genome and epigenome. These assays have been performed on single cells trapped in microwells,271 microchambers,272,273 and droplets.89,274,275 Gong et al. demonstrated a microwell-based protocol for single cell detection of gene expression by single step RT-PCR of mRNA transcripts. The system deposited a dilute cell suspension with a PCR mix into a microwell array for on-chip PCR and fluorescence detection.271 Zhang et al. developed a microarray with 900 microwells that was capable of performing fully integrated liquid phase purification of DNA or RNA and qPCR of single bacterial cells. This microarray achieved 87% and 71% single cell positive amplification for DNA and RNA, respectively.276 This platform was also employed to investigate bacterial adhesion to single host cells.276 With hydrophilic-surface-based single cell trapping, Shi et al.111 and Zhu et al.277 demonstrated real-time PCR assay of single bacterial cells and reverse transcription quantitative PCR (RT-qPCR) assay of single Huh-7 cells, respectively. White et al. developed a fully integrated device consisting of a series of pneumatic-valve-formed microchambers to capture single cells, which were then washed, lysed, and reverse transcribed. A PCR mix was loaded into the chambers for real time qPCR to measure the gene expression.272 White et al. then expanded upon their previous high-throughput platform by adding a digital PCR assay instead of the qPCR chamber, reaching 204
000 PCR reactions per run. This approach made it possible to provide direct quantification of mRNA transcripts and microRNAs on a high-throughput platform.62
Kumaresan et al. reported a droplet-based microfluidic platform that could generate droplets containing single cells, primer-attached beads, and a PCR mix for the PCR analysis of a specific gene in single cells.275 Combined with fluorescence microscopy analysis, single cell agarose droplet PCR has been used to digitally detect rare pathogens with high sensitivity and throughput278 and transcriptional profiling of single mammalian cells with a throughput of ∼50
000 individual cells per run.279 It was reported that CE could also be coupled with single cell droplet PCR for the analysis of short tandem repeat (STR) in forensic identification.89 Combining single-cell droplet PCR with DEP-based droplet sorting, PCR-activated cell sorting (PACS) was able to separate droplets containing target nucleic acids within single cells after PCR.274 This was utilized for the enrichment of genomes and transcripts of prostate cancer cells (Fig. 4B). PACS has further been used to sort rare microbes90 or specific viruses280,281 based on their genomes from a mixed population without requiring cultivation.
5.3. Transcriptomic, genomic and epigenomic analysis
We have recently written a review specifically on microfluidics-based whole-genome analysis assays that included transcriptomic, genomic and epigenomic analysis of single cells.282 Thus we will only highlight some of the most important works on omic analysis of single cells here.
RNA sequencing (RNA-seq) provides the ability to classify the entirety of the transcriptome of a single cell. Streets et al. developed a multiplexed assay to capture single cells, perform lysis, reverse transcription, polyA tailing, primer digestion, and second strand cDNA synthesis.63 Other steps such as amplification, purification, library preparation, and sequencing were all performed off-chip. This device utilized multiple chambers to increase the reaction volume with every step to achieve proper concentrations and produce such high quality cDNA for sequencing that the majority of the bulk transcriptome could be recreated using the transcriptomes of 10 single cells. Using mESCs, this method was able to detect an average of 8000 genes per cell, which was approximately 65% of the bulk samples detected.63 A commercially available Fluidigm C1 microchamber-based platform has also been widely used for RNA-seq. It can perform 96 parallel assays of single cell capture, lysis, reverse transcription, and preamplification.283 The platform has been utilized to study RNA sequencing for a number of different biological outcomes such as understanding the cellular taxonomy of neurons,284 cell reprogramming,284 genetic causes of diseases,285 and constructing lineage hierarchies.283
Microwells have also been utilized as reactors to process single cells in preparation for RNA sequencing. DeKosky et al. fabricated 170
000 wells of 125 pl volume within a PDMS slide for containing single cells and poly(dT) magnetic beads. After annealing, the beads were collected and emulsified for cDNA synthesis. Sequencing was performed after linkage PCR.286 Fan et al. demonstrated that barcoded beads loaded into microwells were able to distinguish each single cell and each transcript copy.287 By this method, one could examine a few thousand cells simultaneously with high sensitivity. Bose et al. integrated the microwells in a microfluidic platform, which allowed for easy reagent loading and diffusion-based fluid exchange.288 Furthermore, by using sealing oil, this system was able to reduce crosstalk between samples by physically segregating the microwells during lysis and mRNA hybridization. The average sample using this method detected a mean of 635 genes per cell using human cancer cell lines. Similarly, a more automated microwell platform has been developed for single cell RNA-seq.289 Recently, a portable and low-cost platform, named Seq-Well, was reported for massively parallel single cell RNA-seq, in which ∼86
000 sub-nanoliter wells within a slide of PDMS could achieve ∼95% single-bead loading efficiency and 80% single cell capture efficiency.290 A semipermeable membrane was used to seal the microwells that would trap biological macromolecules within the microwells while enabling rapid solution exchange.
Two microfluidic droplet-based RNA-seq devices have been reported in 2015. Macosko et al. utilized nanoliter droplets to combine single cells together with barcoded beads for the analysis of all mRNAs from a single cell, termed Drop-seq.9 Barcoded beads have been carefully designed with a priming site for downstream PCR and sequencing, a cell barcode sequence for identifying different cells, a Unique Molecular Identifier (UMI) for distinguishing different mRNA transcripts, and an oligo-dT sequencing for the capture of mRNAs (Fig. 5A). Drop-seq has been used to characterize transcriptomes and find 39 distinct cell populations with a high throughput.9 Instead of barcoded beads, Klein et al. reported using barcoded hydrogel microspheres co-encapsulated in droplets with single cells and a RT mix, termed inDrop.11 The hydrogel microspheres contained a T7 promoter, a sequencing primer, barcodes, a synthesis adaptor, UMI, and a poly-T primer. It has been demonstrated to profile the whole transcriptome of mESCs with a throughput of over 10
000 cells per run.11
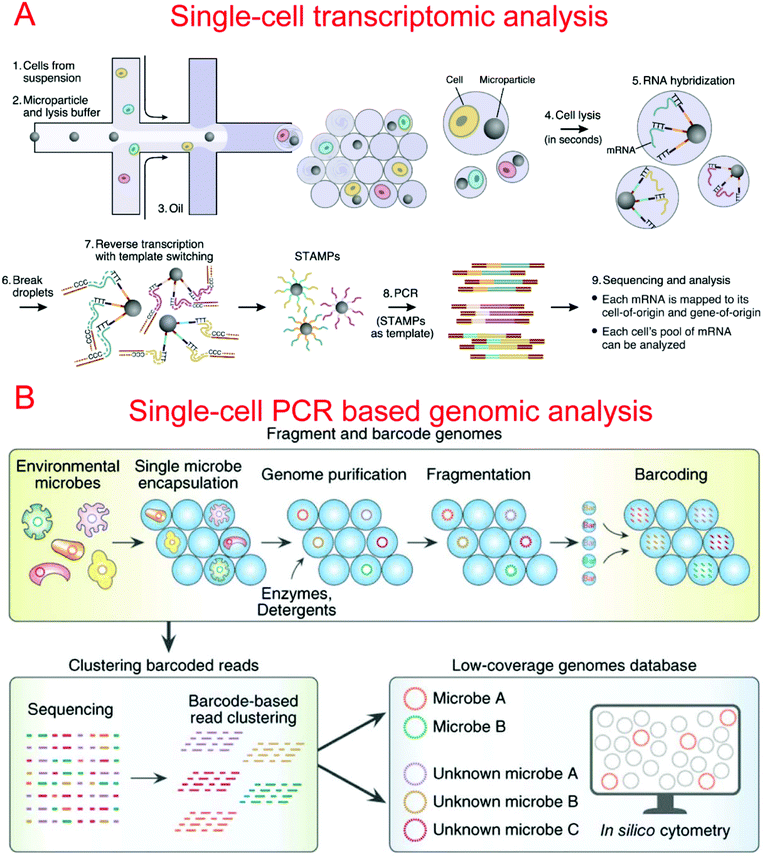 |
| Fig. 5 Single-cell transcriptomic and genomic analysis. (A) Single-cell transcriptomic analysis. Reprinted with permission from E. Z. Macosko et al., Cell, 2015, 161, 1202–1214. Copyright 2015 Elsevier. (B) Single-cell genomic analysis. Reprinted with permission from F. Lan et al., Nat. Biotechnol., 2017, 35, 640–646. Copyright 2017 Macmillan Publishers Ltd. | |
It is often advantageous to perform whole-genome amplification in order to study the DNA of a single cell. Multiple displacement amplification (MDA) is a non-PCR-based method for whole-genome amplification which has been known to generate less nonspecific amplification artifacts compared with PCR amplification. The first microfluidic single cell MDA was reported by Marcy et al., in which multiple single cell processing was achieved by utilizing microchambers formed by integrated valves.59 Single E. coli MDA was performed with a small volume of 60 nl that could reduce background amplification and improve amplification efficiency. This platform was further used to amplify and analyze genomes of uncultivated, rare single microbial cells,291 as well as single sperm cells.61 Instead of MDA, Yu et al. developed a microfluidic device that was able to perform whole genome amplification utilizing multiple annealing and looping based amplification cycles (MALBEC). After single cell isolation, lysis, MALBEC preamplification, and MALBEC, PCR was performed, achieving lower contamination and improved amplification uniformity.292
By using a commercially available liquid dispensing platform, Leung et al. created nanoliter droplets on a planar substrate as single cell MDA reactors. The method was able to achieve up to 80% coverage at the single cell level.293 Gole et al. developed a microwell displacement amplification system (MIDAS) to perform a single cell MDA reaction within 12 nl volume, achieving highly uniform genome coverage and low amplification bias.107 By reducing the amplification volume even further, Fu et al. demonstrated single cell whole genome amplification with reduced amplification bias while utilizing MDA.92 This was done by encapsulating fragments of genomic DNA in picoliter droplets which could then be isothermally amplified to saturation.92 Recently, Lan et al. developed an ultra-high-throughput droplet-microfluidic platform to prepare single-cell genomes for sequencing, which achieved single-cell genomic sequencing (SiC-seq) at >50
000 cells per run and the sequencing of large populations of single cells (Fig. 5B).294
Microfluidic epigenomic analysis has just been emerging. We developed microfluidic oscillatory washing based chromatin immunoprecipitation (MOWChIP-seq) to examine genome-wide histone modifications using as few as 100 cells.295 Our technology used a packed bed of beads and oscillatory washing to achieve adsorption of ChIP DNA with high efficiency approaching the theoretical limit. Drop-ChIP was the first microfluidic single cell ChIP-seq tool.10 Chromatin fragments from single cells were encapsulated and processed in droplets to tag unique barcodes to chromatin from individual cells. Then ChIP was conducted at the population level. Drop-ChIP was able to reveal variation among cells. However, the downside was that each cell only yielded about 1000 reads, which were not enough to provide a high resolution of genome-wide features.
6. Conclusions
Many microfluidic technologies have been developed over the past decade for the analysis of single cells. These devices have become increasingly integrated, high-throughput, and sensitive. Future generations of microfluidic single cell tools will trend towards high-throughput and multi-modal assays with full workflow integration and limited operator interaction. We predict that molecular biology assays will be a significant point of growth in the near future, given their direct relevance to clinical assessment and decision-making. With the decreasing cost associated with next-generation sequencing, single-cell omic analysis will also attract substantial interest and development. The application of these single cell technologies will enable the analysis and profiling of various cell and tissue types in the human body, as well as yielding improved understanding and resolution of cell signaling pathways and phenotypic/genotypic changes. Finally, there will likely be great strides made in the field of personalized and precision medicine with the widespread availability of single cell profiling technologies, specifically with regard to rare cell types such as circulating tumor cells and cancer stem cells.
Conflicts of interest
There are no conflicts to declare.
Acknowledgements
We acknowledge support from National Institutes of Health grants HG008623, CA214176, EB017235, and HG009256.
References
- G. M. Whitesides, Nature, 2006, 442, 368–373 CrossRef CAS PubMed.
- J. El-Ali, P. K. Sorger and K. F. Jensen, Nature, 2006, 442, 403–411 CrossRef CAS PubMed.
- T. Thorsen, S. J. Maerkl and S. R. Quake, Science, 2002, 298, 580–584 CrossRef CAS PubMed.
- M. A. Unger, H.-P. Chou, T. Thorsen, A. Scherer and S. R. Quake, Science, 2000, 288, 113 CrossRef CAS PubMed.
- S. Y. Teh, R. Lin, L. H. Hung and A. P. Lee, Lab Chip, 2008, 8, 198–220 RSC.
- D. Belder, Angew. Chem., Int. Ed., 2005, 44, 3521–3522 CrossRef CAS PubMed.
- H. N. Joensson and H. Andersson Svahn, Angew. Chem., Int. Ed., 2012, 51, 12176–12192 CrossRef CAS PubMed.
- M. T. Guo, A. Rotem, J. A. Heyman and D. A. Weitz, Lab Chip, 2012, 12, 2146–2155 RSC.
- E. Z. Macosko, A. Basu, R. Satija, J. Nemesh, K. Shekhar, M. Goldman, I. Tirosh, A. R. Bialas, N. Kamitaki, E. M. Martersteck, J. J. Trombetta, D. A. Weitz, J. R. Sanes, A. K. Shalek, A. Regev and S. A. McCarroll, Cell, 2015, 161, 1202–1214 CrossRef CAS PubMed.
- A. Rotem, O. Ram, N. Shoresh, R. A. Sperling, A. Goren, D. A. Weitz and B. E. Bernstein, Nat. Biotechnol., 2015, 33, 1165–1172 CrossRef CAS PubMed.
- A. M. Klein, L. Mazutis, I. Akartuna, N. Tallapragada, A. Veres, V. Li, L. Peshkin, D. A. Weitz and M. W. Kirschner, Cell, 2015, 161, 1187–1201 CrossRef CAS PubMed.
- F. Lan, J. R. Haliburton, A. Yuan and A. R. Abate, Nat. Commun., 2016, 7, 11784 CrossRef CAS PubMed.
- M. Abdelgawad and A. R. Wheeler, Adv. Mater., 2009, 21, 920–925 CrossRef CAS.
- K. Choi, A. H. Ng, R. Fobel and A. R. Wheeler, Annu. Rev. Anal. Chem., 2012, 5, 413–440 CrossRef CAS PubMed.
- F. Buettner, K. N. Natarajan, F. P. Casale, V. Proserpio, A. Scialdone, F. J. Theis, S. A. Teichmann, J. C. Marioni and O. Stegle, Nat. Biotechnol., 2015, 33, 155–160 CrossRef CAS PubMed.
- J. P. Junker and A. van Oudenaarden, Cell, 2014, 157, 8–11 CrossRef CAS PubMed.
- W. M. Weaver, P. Tseng, A. Kunze, M. Masaeli, A. J. Chung, J. S. Dudani, H. Kittur, R. P. Kulkarni and D. Di Carlo, Curr. Opin. Biotechnol, 2014, 25, 114–123 CrossRef CAS PubMed.
- D. T. Miyamoto, R. J. Lee, S. L. Stott, D. T. Ting, B. S. Wittner, M. Ulman, M. E. Smas, J. B. Lord, B. W. Brannigan, J. Trautwein, N. H. Bander, C. L. Wu, L. V. Sequist, M. R. Smith, S. Ramaswamy, M. Toner, S. Maheswaran and D. A. Haber, Cancer Discovery, 2012, 2, 995–1003 CrossRef CAS PubMed.
- S. L. Stott, R. J. Lee, S. Nagrath, M. Yu, D. T. Miyamoto, L. Ulkus, E. J. Inserra, M. Ulman, S. Springer, Z. Nakamura, A. L. Moore, D. I. Tsukrov, M. E. Kempner, D. M. Dahl, C. L. Wu, A. J. Iafrate, M. R. Smith, R. G. Tompkins, L. V. Sequist, M. Toner, D. A. Haber and S. Maheswaran, Sci. Transl. Med., 2010, 2, 25ra23 Search PubMed.
- D. A. Lawson, N. R. Bhakta, K. Kessenbrock, K. D. Prummel, Y. Yu, K. Takai, A. Zhou, H. Eyob, S. Balakrishnan, C. Y. Wang, P. Yaswen, A. Goga and Z. Werb, Nature, 2015, 526, 131–135 CrossRef CAS PubMed.
- L. Wen and F. Tang, Genome Biol., 2016, 17, 71 CrossRef PubMed.
- P. O. Krutzik and G. P. Nolan, Nat. Methods, 2006, 3, 361–368 CrossRef CAS PubMed.
- P. O. Krutzik, J. M. Crane, M. R. Clutter and G. P. Nolan, Nat. Chem. Biol., 2008, 4, 132–142 CrossRef CAS PubMed.
- B. Huang, H. Wu, D. Bhaya, A. Grossman, S. Granier, B. K. Kobilka and R. N. Zare, Science, 2007, 315, 81–84 CrossRef CAS PubMed.
- G. D. Jeffries, R. M. Lorenz and D. T. Chiu, Anal. Chem., 2010, 82, 9948–9954 CrossRef CAS PubMed.
- S. Lindstrom, M. Eriksson, T. Vazin, J. Sandberg, J. Lundeberg, J. Frisen and H. Andersson-Svahn, PLoS One, 2009, 4, e6997 Search PubMed.
- K. Zhang, A. C. Martiny, N. B. Reppas, K. W. Barry, J. Malek, S. W. Chisholm and G. M. Church, Nat. Biotechnol., 2006, 24, 680–686 CrossRef CAS PubMed.
- K. Kurimoto, Y. Yabuta, Y. Ohinata and M. Saitou, Nat. Protoc., 2007, 2, 739–752 CrossRef CAS PubMed.
- N. E. Potter, L. Ermini, E. Papaemmanuil, G. Cazzaniga, G. Vijayaraghavan, I. Titley, A. Ford, P. Campbell, L. Kearney and M. Greaves, Genome Res., 2013, 23, 2115–2125 CrossRef CAS PubMed.
- P. Dalerba, T. Kalisky, D. Sahoo, P. S. Rajendran, M. E. Rothenberg, A. A. Leyrat, S. Sim, J. Okamoto, D. M. Johnston, D. Qian, M. Zabala, J. Bueno, N. F. Neff, J. Wang, A. A. Shelton, B. Visser, S. Hisamori, Y. Shimono, M. van de Wetering, H. Clevers, M. F. Clarke and S. R. Quake, Nat. Biotechnol., 2011, 29, 1120–1127 CrossRef CAS PubMed.
- V. Espina, J. D. Wulfkuhle, V. S. Calvert, A. VanMeter, W. D. Zhou, G. Coukos, D. H. Geho, E. F. Petricoin and L. A. Liotta, Nat. Protoc., 2006, 1, 586–603 CrossRef CAS PubMed.
- X. Y. Ding, P. Li, S. C. S. Lin, Z. S. Stratton, N. Nama, F. Guo, D. Slotcavage, X. L. Mao, J. J. Shi, F. Costanzo and T. J. Huang, Lab Chip, 2013, 13, 3626–3649 RSC.
- T. Franke, S. Braunmuller, L. Schmid, A. Wixforth and D. A. Weitz, Lab Chip, 2010, 10, 789–794 RSC.
- C. W. Shields, C. D. Reyes and G. P. Lopez, Lab Chip, 2015, 15, 1230–1249 RSC.
- P. Li, Z. M. Mao, Z. L. Peng, L. L. Zhou, Y. C. Chen, P. H. Huang, C. I. Truica, J. J. Drabick, W. S. El-Deiry, M. Dao, S. Suresh and T. J. Huang, Proc. Natl. Acad. Sci. U. S. A., 2015, 112, 4970–4975 CrossRef CAS PubMed.
- D. Huh, J. H. Bahng, Y. Ling, H. H. Wei, O. D. Kripfgans, J. B. Fowlkes, J. B. Grotberg and S. Takayama, Anal. Chem., 2007, 79, 1369–1376 CrossRef CAS PubMed.
- N. Xia, T. P. Hunt, B. T. Mayers, E. Alsberg, G. M. Whitesides, R. M. Westervelt and D. E. Ingber, Biomed. Microdevices, 2006, 8, 299–308 CrossRef CAS PubMed.
- N. Pamme and C. Wilhelm, Lab Chip, 2006, 6, 974–980 RSC.
- A. E. Saliba, L. Saias, E. Psychari, N. Minc, D. Simon, F. C. Bidard, C. Mathiot, J. Y. Pierga, V. Fraisier, J. Salamero, V. Saada, F. Farace, P. Vielh, L. Malaquin and J. L. Viovy, Proc. Natl. Acad. Sci. U. S. A., 2010, 107, 14524–14529 CrossRef CAS PubMed.
- V. H. Lieu, T. A. House and D. T. Schwartz, Anal. Chem., 2012, 84, 1963–1968 CrossRef CAS PubMed.
- M. Tanyeri and C. M. Schroeder, Nano Lett., 2013, 13, 2357–2364 CrossRef CAS PubMed.
- A. Karimi, S. Yazdi and A. M. Ardekani, Biomicrofluidics, 2013, 7, 21501 CrossRef CAS PubMed.
- D. Di Carlo, L. Y. Wu and L. P. Lee, Lab Chip, 2006, 6, 1445–1449 RSC.
- X. Li and P. C. Li, Anal. Chem., 2005, 77, 4315–4322 CrossRef CAS PubMed.
- D. Di Carlo, N. Aghdam and L. P. Lee, Anal. Chem., 2006, 78, 4925–4930 CrossRef CAS PubMed.
- F. Shen, X. Li and P. C. Li, Biomicrofluidics, 2014, 8, 014109 CrossRef PubMed.
- H. Chen, J. Sun, E. Wolvetang and J. Cooper-White, Lab Chip, 2015, 15, 1072–1083 RSC.
- J. Chung, Y. J. Kim and E. Yoon, Appl. Phys. Lett., 2011, 98, 123701 CrossRef PubMed.
- F. Yesilkoy, R. Ueno, B. X. Desbiolles, M. Grisi, Y. Sakai, B. J. Kim and J. Brugger, Biomicrofluidics, 2016, 10, 014120 CAS.
- K. Zhang, X. Han, Y. Li, S. Y. Li, Y. Zu, Z. Wang and L. Qin, J. Am. Chem. Soc., 2014, 136, 10858–10861 CrossRef CAS PubMed.
- Y. Yamaguchi, T. Arakawa, N. Takeda, Y. Edagawa and S. Shoji, Sens. Actuators, B, 2009, 136, 555–561 CrossRef CAS.
- D. Jin, B. Deng, J. X. Li, W. Cai, L. Tu, J. Chen, Q. Wu and W. H. Wang, Biomicrofluidics, 2015, 9, 014101 CrossRef CAS PubMed.
- Y. Deng, Y. Zhang, S. Sun, Z. Wang, M. Wang, B. Yu, D. M. Czajkowsky, B. Liu, Y. Li, W. Wei and Q. Shi, Sci. Rep., 2014, 4, 7499 CrossRef CAS PubMed.
- B. de Wagenaar, J. T. Berendsen, J. G. Bomer, W. Olthuis, A. van den Berg and L. I. Segerink, Lab Chip, 2015, 15, 1294–1301 RSC.
- K. Chung, Y. Kim, J. S. Kanodia, E. Gong, S. Y. Shvartsman and H. Lu, Nat. Methods, 2011, 8, 171–176 CrossRef CAS PubMed.
- S. Hong, Q. Pan and L. P. Lee, Integr. Biol., 2012, 4, 374–380 RSC.
- J. W. Hong, V. Studer, G. Hang, W. F. Anderson and S. R. Quake, Nat. Biotechnol., 2004, 22, 435–439 CrossRef CAS PubMed.
- E. A. Ottesen, J. W. Hong, S. R. Quake and J. R. Leadbetter, Science, 2006, 314, 1464–1467 CrossRef CAS PubMed.
- Y. Marcy, T. Ishoey, R. S. Lasken, T. B. Stockwell, B. P. Walenz, A. L. Halpern, K. Y. Beeson, S. M. Goldberg and S. R. Quake, PLoS Genet., 2007, 3, 1702–1708 CAS.
- H. C. Fan, J. Wang, A. Potanina and S. R. Quake, Nat. Biotechnol., 2011, 29, 51–57 CrossRef CAS PubMed.
- J. Wang, H. C. Fan, B. Behr and S. R. Quake, Cell, 2012, 150, 402–412 CrossRef CAS PubMed.
- A. K. White, K. A. Heyries, C. Doolin, M. Vaninsberghe and C. L. Hansen, Anal. Chem., 2013, 85, 7182–7190 CrossRef CAS PubMed.
- A. M. Streets, X. Zhang, C. Cao, Y. Pang, X. Wu, L. Xiong, L. Yang, Y. Fu, L. Zhao, F. Tang and Y. Huang, Proc. Natl. Acad. Sci. U. S. A., 2014, 111, 7048–7053 CrossRef CAS PubMed.
- Q. Shi, L. Qin, W. Wei, F. Geng, R. Fan, Y. S. Shin, D. Guo, L. Hood, P. S. Mischel and J. R. Heath, Proc. Natl. Acad. Sci. U. S. A., 2012, 109, 419–424 CrossRef CAS PubMed.
- N. M. Toriello, E. S. Douglas and R. A. Mathies, Anal. Chem., 2005, 77, 6935–6941 CrossRef CAS PubMed.
- C. M. Das, F. Becker, S. Vernon, J. Noshari, C. Joyce and P. R. Gascoyne, Anal. Chem., 2005, 77, 2708–2719 CrossRef CAS PubMed.
- M. S. Jaeger, K. Uhlig, T. Schnelle and T. Mueller, J. Phys. D: Appl. Phys., 2008, 41, 175502 CrossRef.
- R. S. Thomas, P. D. Mitchell, R. O. Oreffo and H. Morgan, Biomicrofluidics, 2010, 4, 022806 CrossRef PubMed.
- A. F. Chrimes, K. Khoshmanesh, S. Y. Tang, B. R. Wood, P. R. Stoddart, S. S. Collins, A. Mitchell and K. Kalantar-zadeh, Biosens. Bioelectron., 2013, 49, 536–541 CrossRef CAS PubMed.
- U. C. Schroder, A. Ramoji, U. Glaser, S. Sachse, C. Leiterer, A. Csaki, U. Hubner, W. Fritzsche, W. Pfister, M. Bauer, J. Popp and U. Neugebauer, Anal. Chem., 2013, 85, 10717–10724 CrossRef PubMed.
- P. Zhang, L. Ren, X. Zhang, Y. Shan, Y. Wang, Y. Ji, H. Yin, W. E. Huang, J. Xu and B. Ma, Anal. Chem., 2015, 87, 2282–2289 CrossRef CAS PubMed.
- N. C. Chen, C. H. Chen, M. K. Chen, L. S. Jang and M. H. Wang, Sens. Actuators, B, 2014, 190, 570–577 CrossRef CAS.
- C. Xie, M. A. Dinno and Y. Q. Li, Opt. Lett., 2002, 27, 249–251 CrossRef PubMed.
- C. G. Xie, Y. Q. Li, W. Tang and R. J. Newton, J. Appl. Phys., 2003, 94, 6138–6142 CrossRef CAS.
- C. Xie, D. Chen and Y. Q. Li, Opt. Lett., 2005, 30, 1800–1802 CrossRef PubMed.
- A. Y. Lau, L. P. Lee and J. W. Chan, Lab Chip, 2008, 8, 1116–1120 RSC.
- S. Dochow, C. Krafft, U. Neugebauer, T. Bocklitz, T. Henkel, G. Mayer, J. Albert and J. Popp, Lab Chip, 2011, 11, 1484–1490 RSC.
- P. Y. Chiou, A. T. Ohta and M. C. Wu, Nature, 2005, 436, 370–372 CrossRef CAS PubMed.
- K. W. Huang, Y. C. Wu, J. A. Lee and P. Y. Chiou, Lab Chip, 2013, 13, 3721–3727 RSC.
- S. Koster, F. E. Angile, H. Duan, J. J. Agresti, A. Wintner, C. Schmitz, A. C. Rowat, C. A. Merten, D. Pisignano, A. D. Griffiths and D. A. Weitz, Lab Chip, 2008, 8, 1110–1115 RSC.
- B. L. Wang, A. Ghaderi, H. Zhou, J. Agresti, D. A. Weitz, G. R. Fink and G. Stephanopoulos, Nat. Biotechnol., 2014, 32, 473–478 CrossRef CAS PubMed.
- H. Lu, O. Caen, J. Vrignon, E. Zonta, Z. El Harrak, P. Nizard, J. C. Baret and V. Taly, Sci. Rep., 2017, 7, 1366 CrossRef PubMed.
- F. Chen, Y. Zhan, T. Geng, H. Lian, P. Xu and C. Lu, Anal. Chem., 2011, 83, 8816–8820 CrossRef CAS PubMed.
- Y. Zhan, J. Wang, N. Bao and C. Lu, Anal. Chem., 2009, 81, 2027–2031 CrossRef CAS PubMed.
- L. Mazutis, J. Gilbert, W. L. Ung, D. A. Weitz, A. D. Griffiths and J. A. Heyman, Nat. Protoc., 2013, 8, 870–891 CrossRef CAS PubMed.
- S. L. Sjostrom, Y. Bai, M. Huang, Z. Liu, J. Nielsen, H. N. Joensson and H. Andersson Svahn, Lab Chip, 2014, 14, 806–813 RSC.
- A. Zinchenko, S. R. Devenish, B. Kintses, P. Y. Colin, M. Fischlechner and F. Hollfelder, Anal. Chem., 2014, 86, 2526–2533 CrossRef CAS PubMed.
- E. Brouzes, M. Medkova, N. Savenelli, D. Marran, M. Twardowski, J. B. Hutchison, J. M. Rothberg, D. R. Link, N. Perrimon and M. L. Samuels, Proc. Natl. Acad. Sci. U. S. A., 2009, 106, 14195–14200 CrossRef CAS PubMed.
- T. Geng, R. Novak and R. A. Mathies, Anal. Chem., 2014, 86, 703–712 CrossRef CAS PubMed.
- S. W. Lim, T. M. Tran and A. R. Abate, PLoS One, 2015, 10, e0113549 Search PubMed.
- K. Leung, H. Zahn, T. Leaver, K. M. Konwar, N. W. Hanson, A. P. Page, C. C. Lo, P. S. Chain, S. J. Hallam and C. L. Hansen, Proc. Natl. Acad. Sci. U. S. A., 2012, 109, 7665–7670 CrossRef CAS PubMed.
- Y. Fu, C. Li, S. Lu, W. Zhou, F. Tang, X. S. Xie and Y. Huang, Proc. Natl. Acad. Sci. U. S. A., 2015, 112, 11923–11928 CrossRef CAS PubMed.
- T. Jing, R. Ramji, M. E. Warkiani, J. Han, C. T. Lim and C. H. Chen, Biosens. Bioelectron., 2015, 66, 19–23 CrossRef CAS PubMed.
- E. W. Kemna, R. M. Schoeman, F. Wolbers, I. Vermes, D. A. Weitz and A. van den Berg, Lab Chip, 2012, 12, 2881–2887 RSC.
- M. Chabert and J. L. Viovy, Proc. Natl. Acad. Sci. U. S. A., 2008, 105, 3191–3196 CrossRef CAS PubMed.
- J. F. Edd, D. Di Carlo, K. J. Humphry, S. Koster, D. Irimia, D. A. Weitz and M. Toner, Lab Chip, 2008, 8, 1262–1264 RSC.
- Z. Cao, F. Chen, N. Bao, H. He, P. Xu, S. Jana, S. Jung, H. Lian and C. Lu, Lab Chip, 2013, 13, 171–178 RSC.
- L. Wu, P. Chen, Y. Dong, X. Feng and B. F. Liu, Biomed. Microdevices, 2013, 15, 553–560 CrossRef CAS PubMed.
- Q. Zhang, T. Wang, Q. Zhou, P. Zhang, Y. Gong, H. Gou, J. Xu and B. Ma, Sci. Rep., 2017, 7, 41192 CrossRef CAS PubMed.
- C. Liu, J. Liu, D. Gao, M. Ding and J. M. Lin, Anal. Chem., 2010, 82, 9418–9424 CrossRef CAS PubMed.
- V. I. Chin, P. Taupin, S. Sanga, J. Scheel, F. H. Gage and S. N. Bhatia, Biotechnol. Bioeng., 2004, 88, 399–415 CrossRef CAS PubMed.
- Y. Tokimitsu, H. Kishi, S. Kondo, R. Honda, K. Tajiri, K. Motoki, T. Ozawa, S. Kadowaki, T. Obata, S. Fujiki, C. Tateno, H. Takaishi, K. Chayama, K. Yoshizato, E. Tamiya, T. Sugiyama and A. Muraguchi, Cytometry, Part A, 2007, 71, 1003–1010 CrossRef PubMed.
- P. Guo, E. W. Hall, R. Schirhagl, H. Mukaibo, C. R. Martin and R. N. Zare, Lab Chip, 2012, 12, 558–561 RSC.
- M. Bocchi, L. Rambelli, A. Faenza, L. Giulianelli, N. Pecorari, E. Duqi, J. C. Gallois and R. Guerrieri, Lab Chip, 2012, 12, 3168–3176 RSC.
- A. Faenza, M. Bocchi, E. Duqi, L. Giulianelli, N. Pecorari, L. Rambelli and R. Guerrieri, Anal. Chem., 2013, 85, 3446–3453 CrossRef CAS PubMed.
- L. Huang, Y. Chen, Y. Chen and H. Wu, Anal. Chem., 2015, 87, 12169–12176 CrossRef CAS PubMed.
- J. Gole, A. Gore, A. Richards, Y. J. Chiu, H. L. Fung, D. Bushman, H. I. Chiang, J. Chun, Y. H. Lo and K. Zhang, Nat. Biotechnol., 2013, 31, 1126–1132 CrossRef CAS PubMed.
- D. K. Wood, D. M. Weingeist, S. N. Bhatia and B. P. Engelward, Proc. Natl. Acad. Sci. U. S. A., 2010, 107, 10008–10013 CrossRef CAS PubMed.
- L. I. Lin, S. H. Chao and D. R. Meldrum, PLoS One, 2009, 4, e6710 Search PubMed.
- K. Jang, Y. Xu, Y. Tanaka, K. Sato, K. Mawatari, T. Konno, K. Ishihara and T. Kitamori, Biomicrofluidics, 2010, 4, 32208 CrossRef PubMed.
- X. Shi, L. I. Lin, S. Y. Chen, S. H. Chao, W. Zhang and D. R. Meldrum, Lab Chip, 2011, 11, 2276–2281 RSC.
- A. Revzin, K. Sekine, A. Sin, R. G. Tompkins and M. Toner, Lab Chip, 2005, 5, 30–37 RSC.
- Q. Chen, J. Wu, Y. Zhang, Z. Lin and J. M. Lin, Lab Chip, 2012, 12, 5180–5185 RSC.
- L. Lin, Y. S. Chu, J. P. Thiery, C. T. Lim and I. Rodriguez, Lab Chip, 2013, 13, 714–721 RSC.
- Y. Zheng and Y. Sun, Micro Nano Lett., 2011, 6, 327–331 CAS.
- J. P. Shelby, J. White, K. Ganesan, P. K. Rathod and D. T. Chiu, Proc. Natl. Acad. Sci. U. S. A., 2003, 100, 14618–14622 CrossRef CAS PubMed.
- W. G. Lee, H. Bang, H. Yun, J. Lee, J. Park, J. K. Kim, S. Chung, K. Cho, C. Chung, D. C. Han and J. K. Chang, Lab Chip, 2007, 7, 516–519 RSC.
- H. W. Hou, Q. S. Li, G. Y. Lee, A. P. Kumar, C. N. Ong and C. T. Lim, Biomed. Microdevices, 2009, 11, 557–564 CrossRef CAS PubMed.
- Y. Katsumoto, K. Tatsumi, T. Doi and K. Nakabe, Int. J. Heat Fluid Flow, 2010, 31, 985–995 CrossRef.
-
B. Lincoln, F. Wottawah, S. Schinkinger, S. Ebert and J. Guck, in Methods Cell Biol, Academic Press, 2007, vol. 83, pp. 397–423 Search PubMed.
- F. Lautenschlager, S. Paschke, S. Schinkinger, A. Bruel, M. Beil and J. Guck, Proc. Natl. Acad. Sci. U. S. A., 2009, 106, 15696–15701 CrossRef PubMed.
- L. A. MacQueen, M. D. Buschmann and M. R. Wertheimer, J. Micromech. Microeng., 2010, 20, 065007.
- J. Chen, M. Abdelgawad, L. Yu, N. Shakiba, W.-Y. Chien, Z. Lu, W. R. Geddie, M. A. S. Jewett and Y. Sun, J. Micromech. Microeng., 2011, 21, 054012.
- N. Bao, Y. Zhan and C. Lu, Anal. Chem., 2008, 80, 7714–7719 CrossRef CAS PubMed.
-
W. Kim and A. Han, A Micro-Aspirator Chip Using Vacuum Expanded Microchannels for High-Throughput Mechanical Characterization of Biological Cells, in The 14th International Conference on Miniaturized Systems for Chemistry and Life Sciences (μTAS 2010), Groningen, The Netherlands, 2010, pp. 253–255 Search PubMed.
- X. Y. Liu, Y. F. Wang and Y. Sun, IEEE Trans. Autom. Sci. Eng., 2009, 6, 536–543 CrossRef.
- B. Lincoln, H. M. Erickson, S. Schinkinger, F. Wottawah, D. Mitchell, S. Ulvick, C. Bilby and J. Guck, Cytometry, Part A, 2004, 59, 203–209 CrossRef PubMed.
- M. J. Rosenbluth, W. A. Lam and D. A. Fletcher, Lab Chip, 2008, 8, 1062–1070 RSC.
- S. Gabriele, M. Versaevel, P. Preira and O. Theodoly, Lab Chip, 2010, 10, 1459–1467 RSC.
- S. C. Hur, N. K. Henderson-MacLennan, E. R. McCabe and D. Di Carlo, Lab Chip, 2011, 11, 912–920 RSC.
- D. R. Gossett, H. T. Tse, S. A. Lee, Y. Ying, A. G. Lindgren, O. O. Yang, J. Rao, A. T. Clark and D. Di Carlo, Proc. Natl. Acad. Sci. U. S. A., 2012, 109, 7630–7635 CrossRef CAS PubMed.
- H. T. Tse, D. R. Gossett, Y. S. Moon, M. Masaeli, M. Sohsman, Y. Ying, K. Mislick, R. P. Adams, J. Rao and D. Di Carlo, Sci. Transl. Med., 2013, 5, 212ra163 CrossRef PubMed.
- J. S. Dudani, D. R. Gossett, H. T. Tse and D. Di Carlo, Lab Chip, 2013, 13, 3728–3734 RSC.
- O. Otto, P. Rosendahl, A. Mietke, S. Golfier, C. Herold, D. Klaue, S. Girardo, S. Pagliara, A. Ekpenyong, A. Jacobi, M. Wobus, N. Topfner, U. F. Keyser, J. Mansfeld, E. Fischer-Friedrich and J. Guck, Nat. Methods, 2015, 12, 199–202 CrossRef CAS PubMed , 194 p following 202.
- C. Lei, T. Ito, M. Ugawa, T. Nozawa, O. Iwata, M. Maki, G. Okada, H. Kobayashi, X. Sun, P. Tiamsak, N. Tsumura, K. Suzuki, D. Di Carlo, Y. Ozeki and K. Goda, Biomed. Opt. Express, 2016, 7, 2703–2708 CrossRef PubMed.
- L. L. Sohn, O. A. Saleh, G. R. Facer, A. J. Beavis, R. S. Allan and D. A. Notterman, Proc. Natl. Acad. Sci. U. S. A., 2000, 97, 10687–10690 CrossRef CAS PubMed.
- S. Gawad, L. Schild and P. H. Renaud, Lab Chip, 2001, 1, 76–82 RSC.
- H. Morgan, D. Holmes and N. G. Green, Curr. Appl. Phys., 2006, 6, 367–370 CrossRef.
- K. Cheung, S. Gawad and P. Renaud, Cytometry, Part A, 2005, 65, 124–132 CrossRef PubMed.
- T. Sun and H. Morgan, Microfluid. Nanofluid., 2010, 8, 423–443 CrossRef CAS.
- D. Holmes, D. Pettigrew, C. H. Reccius, J. D. Gwyer, C. van Berkel, J. Holloway, D. E. Davies and H. Morgan, Lab Chip, 2009, 9, 2881–2889 RSC.
- J. Chen, C. Xue, Y. Zhao, D. Chen, M. H. Wu and J. Wang, Int. J. Mol. Sci., 2015, 16, 9804–9830 CrossRef CAS PubMed.
- N. Haandbaek, S. C. Burgel, F. Heer and A. Hierlemann, Lab Chip, 2014, 14, 369–377 RSC.
- A. El Hasni, C. Schmitz, K. Bui-Göbbels, P. Bräunig, W. Jahnen-Dechent and U. Schnakenberg, Sens. Actuators, B, 2017, 248, 419–429 CrossRef CAS.
- Z. Zhu, O. Frey, F. Franke, N. Haandbaek and A. Hierlemann, Anal. Bioanal. Chem., 2014, 406, 7015–7025 CrossRef CAS PubMed.
- Y. Zhao, D. Chen, H. Li, Y. Luo, B. Deng, S. B. Huang, T. K. Chiu, M. H. Wu, R. Long, H. Hu, J. Wang and J. Chen, Biosens. Bioelectron., 2013, 43, 304–307 CrossRef CAS PubMed.
- X. Guo, R. Zhu and X. Zong, Analyst, 2015, 140, 6571–6578 RSC.
- Y. Zheng, E. Shojaei-Baghini, C. Wang and Y. Sun, Biosens. Bioelectron., 2013, 42, 496–502 CrossRef CAS PubMed.
- J. Chen, Y. Zheng, Q. Tan, Y. L. Zhang, J. Li, W. R. Geddie, M. A. Jewett and Y. Sun, Biomicrofluidics, 2011, 5, 14113 CrossRef PubMed.
- J. Chen, Y. Zheng, Q. Tan, E. Shojaei-Baghini, Y. L. Zhang, J. Li, P. Prasad, L. You, X. Y. Wu and Y. Sun, Lab Chip, 2011, 11, 3174–3181 RSC.
- D. Kim, E. Choi, S. S. Choi, S. Lee, J. Park and K.-S. Yun, Jpn. J. Appl. Phys., 2010, 49, 127002.
- U. Hassan and R. Bashir, Lab Chip, 2014, 14, 4370–4381 RSC.
- P. K. Ang, A. Li, M. Jaiswal, Y. Wang, H. W. Hou, J. T. Thong, C. T. Lim and K. P. Loh, Nano Lett., 2011, 11, 5240–5246 CrossRef CAS PubMed.
- M. Islam, M. M. Bellah, A. Sajid, M. R. Hasan, Y. T. Kim and S. M. Iqbal, Sci. Rep., 2015, 5, 13031 CrossRef CAS PubMed.
- Y. Zhou, S. Basu, E. Laue and A. A. Seshia, Biosens. Bioelectron., 2016, 81, 249–258 CrossRef CAS PubMed.
- J. L. Hong, K. C. Lan and L. S. Jang, Sens. Actuators, B, 2012, 173, 927–934 CrossRef CAS.
- C. Petchakup, K. H. H. Li and H. W. Hou, Micromachines, 2017, 8, 87 CrossRef.
- D. Holmes and H. Morgan, Anal. Chem., 2010, 82, 1455–1461 CrossRef CAS PubMed.
- J. S. McGrath, C. Honrado, D. Spencer, B. Horton, H. L. Bridle and H. Morgan, Sci. Rep., 2017, 7, 2601 CrossRef CAS PubMed.
- N. N. Watkins, S. Sridhar, X. Cheng, G. D. Chen, M. Toner, W. Rodriguez and R. Bashir, Lab Chip, 2011, 11, 1437–1447 RSC.
- U. Hassan, N. N. Watkins, C. Edwards and R. Bashir, Lab Chip, 2014, 14, 1469–1476 RSC.
- C. Zheng, L. Zhao, G. Chen, Y. Zhou, Y. Pang and Y. Huang, Anal. Chem., 2012, 84, 2088–2093 CrossRef CAS PubMed.
- T. A. Nguyen, T. I. Yin, D. Reyes and G. A. Urban, Anal. Chem., 2013, 85, 11068–11076 CrossRef CAS PubMed.
- S. K. Lee, G. S. Kim, Y. Wu, D. J. Kim, Y. Lu, M. Kwak, L. Han, J. H. Hyung, J. K. Seol, C. Sander, A. Gonzalez, J. Li and R. Fan, Nano Lett., 2012, 12, 2697–2704 CrossRef CAS PubMed.
- J. Riordon, M. Nash, W. Jing and M. Godin, Biomicrofluidics, 2014, 8, 011101 CrossRef PubMed.
- S. Olcum, N. Cermak, S. C. Wasserman, K. S. Christine, H. Atsumi, K. R. Payer, W. Shen, J. Lee, A. M. Belcher, S. N. Bhatia and S. R. Manalis, Proc. Natl. Acad. Sci. U. S. A., 2014, 111, 1310–1315 CrossRef CAS PubMed.
- S. Chen and S. J. Lillard, Anal. Chem., 2001, 73, 111–118 CrossRef CAS PubMed.
- E. R. Mainz, D. S. Serafin, T. T. Nguyen, T. K. Tarrant, C. E. Sims and N. L. Allbritton, Anal. Chem., 2016, 88, 7786–7792 CrossRef CAS PubMed.
- G. D. Meredith, C. E. Sims, J. S. Soughayer and N. L. Allbritton, Nat. Biotechnol., 2000, 18, 309–312 CrossRef CAS PubMed.
- M. A. McClain, C. T. Culbertson, S. C. Jacobson, N. L. Allbritton, C. E. Sims and J. M. Ramsey, Anal. Chem., 2003, 75, 5646–5655 CrossRef CAS PubMed.
- H. Y. Wang and C. Lu, Anal. Chem., 2006, 78, 5158–5164 CrossRef CAS PubMed.
- H. Y. Wang and C. Lu, Chem. Commun., 2006, 3528–3530 RSC.
- K. S. Phillips, H. H. Lai, E. Johnson, C. E. Sims and N. L. Allbritton, Lab Chip, 2011, 11, 1333–1341 RSC.
- S. Zhao, X. Li and Y. M. Liu, Anal. Chem., 2009, 81, 3873–3878 CrossRef CAS PubMed.
- S. Jin, M. D. Furtaw, H. Chen, D. T. Lamb, S. A. Ferguson, N. E. Arvin, M. Dawod and R. T. Kennedy, Anal. Chem., 2016, 88, 6703–6710 CrossRef CAS PubMed.
- C. X. Xu and X. F. Yin, J. Chromatogr., A, 2011, 1218, 726–732 CrossRef CAS PubMed.
- P. J. Marc, C. E. Sims, M. Bachman, G. P. Li and N. L. Allbritton, Lab Chip, 2008, 8, 710–716 RSC.
- A. J. Dickinson, P. M. Armistead and N. L. Allbritton, Anal. Chem., 2013, 85, 4797–4804 CrossRef CAS PubMed.
- B. Shi, W. Huang and J. Cheng, Electrophoresis, 2007, 28, 1595–1600 CrossRef CAS PubMed.
- M. L. Kovarik, P. K. Shah, P. M. Armistead and N. L. Allbritton, Anal. Chem., 2013, 85, 4991–4997 CrossRef CAS PubMed.
- Q. Li, P. Chen, Y. Fan, X. Wang, K. Xu, L. Li and B. Tang, Anal. Chem., 2016, 88, 8610–8616 CrossRef CAS PubMed.
- A. J. Dickinson, S. A. Hunsucker, P. M. Armistead and N. L. Allbritton, Anal. Bioanal. Chem., 2014, 406, 7027–7036 CrossRef CAS PubMed.
- E. R. Mainz, Q. Wang, D. S. Lawrence and N. L. Allbritton, Angew. Chem., Int. Ed., 2016, 55, 13095–13098 CrossRef CAS PubMed.
- A. R. Wheeler, W. R. Throndset, R. J. Whelan, A. M. Leach, R. N. Zare, Y. H. Liao, K. Farrell, I. D. Manger and A. Daridon, Anal. Chem., 2003, 75, 3581–3586 CrossRef CAS PubMed.
- T. Xu, C. W. Li, X. Yao, G. Cai and M. Yang, Anal. Biochem., 2010, 396, 173–179 CrossRef CAS PubMed.
- N. Srivastava, J. S. Brennan, R. F. Renzi, M. Wu, S. S. Branda, A. K. Singh and A. E. Herr, Anal. Chem., 2009, 81, 3261–3269 CrossRef CAS PubMed.
- Z. N. Cao, S. Geng, L. W. Li and C. Lu, Chem. Sci., 2014, 5, 2530–2535 RSC.
- J. Dolezel, J. Greilhuber and J. Suda, Nat. Protoc., 2007, 2, 2233–2244 CrossRef CAS PubMed.
- C. Riccardi and I. Nicoletti, Nat. Protoc., 2006, 1, 1458–1461 CrossRef CAS PubMed.
- D. Wlodkowic, S. Faley, M. Zagnoni, J. P. Wikswo and J. M. Cooper, Anal. Chem., 2009, 81, 5517–5523 CrossRef CAS PubMed.
- J. Wang, N. Bao, L. L. Paris, H. Y. Wang, R. L. Geahlen and C. Lu, Anal. Chem., 2008, 80, 1087–1093 CrossRef CAS PubMed.
- J. Wang, B. Fei, Y. Zhan, R. L. Geahlen and C. Lu, Lab Chip, 2010, 10, 2911–2916 RSC.
- J. Wang, N. Bao, L. L. Paris, R. L. Geahlen and C. Lu, Anal. Chem., 2008, 80, 9840–9844 CrossRef CAS PubMed.
- J. Wang, B. Fei, R. L. Geahlen and C. Lu, Lab Chip, 2010, 10, 2673–2679 RSC.
- J. Wang, Y. Zhan, N. Bao and C. Lu, Lab Chip, 2012, 12, 1441–1445 RSC.
- N. Baumgarth and M. Roederer, J. Immunol. Methods, 2000, 243, 77–97 CrossRef CAS PubMed.
- A. Y. Fu, C. Spence, A. Scherer, F. H. Arnold and S. R. Quake, Nat. Biotechnol., 1999, 17, 1109–1111 CrossRef CAS PubMed.
- J. Krüger, K. Singh, A. O'Neill, C. Jackson, A. Morrison and P. O'Brien, J. Micromech. Microeng., 2002, 12, 486–494.
- D. Holmes, M. E. Sandison, N. G. Green and H. Morgan, IEE Proc. Nanobiotechnol., 2005, 152, 129–135 CrossRef CAS PubMed.
- M. M. Wang, E. Tu, D. E. Raymond, J. M. Yang, H. Zhang, N. Hagen, B. Dees, E. M. Mercer, A. H. Forster, I. Kariv, P. J. Marchand and W. F. Butler, Nat. Biotechnol., 2005, 23, 83–87 CrossRef CAS PubMed.
- T. H. Wu, Y. Chen, S. Y. Park, J. Hong, T. Teslaa, J. F. Zhong, D. Di Carlo, M. A. Teitell and P. Y. Chiou, Lab Chip, 2012, 12, 1378–1383 RSC.
- S.-Y. Yang, S.-K. Hsiung, Y.-C. Hung, C.-M. Chang, T.-L. Liao and G.-B. Lee, Meas. Sci. Technol., 2006, 17, 2001.
- A. Wolff, I. R. Perch-Nielsen, U. D. Larsen, P. Friis, G. Goranovic, C. R. Poulsen, J. P. Kutter and P. Telleman, Lab Chip, 2003, 3, 22–27 RSC.
- N. Varadarajan, B. Julg, Y. J. Yamanaka, H. Chen, A. O. Ogunniyi, E. McAndrew, L. C. Porter, A. Piechocka-Trocha, B. J. Hill, D. C. Douek, F. Pereyra, B. D. Walker and J. C. Love, J. Clin. Invest., 2011, 121, 4322–4331 CAS.
- J. W. Chan, A.
P. Esposito, C. E. Talley, C. W. Hollars, S. M. Lane and T. Huser, Anal. Chem., 2004, 76, 599–603 CrossRef CAS PubMed.
- W. E. Huang, R. I. Griffiths, I. P. Thompson, M. J. Bailey and A. S. Whiteley, Anal. Chem., 2004, 76, 4452–4458 CrossRef CAS PubMed.
- V. Ranc, J. Srovnal, L. Kvitek and M. Hajduch, Analyst, 2013, 138, 5983–5988 RSC.
- C. Xie, J. Mace, M. A. Dinno, Y. Q. Li, W. Tang, R. J. Newton and P. J. Gemperline, Anal. Chem., 2005, 77, 4390–4397 CrossRef CAS PubMed.
- A. Avetisyan, J. B. Jensen and T. Huser, Anal. Chem., 2013, 85, 7264–7270 CrossRef PubMed.
- D. Berry, E. Mader, T. K. Lee, D. Woebken, Y. Wang, D. Zhu, M. Palatinszky, A. Schintlmeister, M. C. Schmid, B. T. Hanson, N. Shterzer, I. Mizrahi, I. Rauch, T. Decker, T. Bocklitz, J. Popp, C. M. Gibson, P. W. Fowler, W. E. Huang and M. Wagner, Proc. Natl. Acad. Sci. U. S. A., 2015, 112, E194–E203 CrossRef CAS PubMed.
- E. Brauchle, S. Thude, S. Y. Brucker and K. Schenke-Layland, Sci. Rep., 2014, 4, 4698 CrossRef PubMed.
- H. G. Schulze, S. O. Konorov, N. J. Caron, J. M. Piret, M. W. Blades and R. F. Turner, Anal. Chem., 2010, 82, 5020–5027 CrossRef CAS PubMed.
- T. Wang, Y. Ji, Y. Wang, J. Jia, J. Li, S. Huang, D. Han, Q. Hu, W. E. Huang and J. Xu, Biotechnol. Biofuels, 2014, 7, 58 CrossRef PubMed.
- T. J. Moritz, D. S. Taylor, C. R. Polage, D. M. Krol, S. M. Lane and J. W. Chan, Anal. Chem., 2010, 82, 2703–2710 CrossRef CAS PubMed.
- K. Ramser, J. Enger, M. Goksor, D. Hanstorp, K. Logg and M. Kall, Lab Chip, 2005, 5, 431–436 RSC.
- Q. Zhang, P. Zhang, H. Gou, C. Mou, W. E. Huang, M. Yang, J. Xu and B. Ma, Analyst, 2015, 140, 6163–6174 RSC.
- J. W. Chan and D. K. Lieu, J. Biophotonics, 2009, 2, 656–668 CrossRef CAS PubMed.
- M. Li, P. C. Ashok, K. Dholakia and W. E. Huang, J. Phys. Chem. A, 2012, 116, 6560–6563 CrossRef CAS PubMed.
- J. P. Nolan, E. Duggan, E. Liu, D. Condello, I. Dave and S. A. Stoner, Methods, 2012, 57, 272–279 CrossRef CAS PubMed.
- D. S. Sebba, D. A. Watson and J. P. Nolan, ACS Nano, 2009, 3, 1477–1484 CrossRef CAS PubMed.
- H. W. Wang, N. Bao, T. L. Le, C. Lu and J. X. Cheng, Opt. Express, 2008, 16, 5782–5789 CrossRef CAS PubMed.
- C. H. Camp Jr., S. Yegnanarayanan, A. A. Eftekhar and A. Adibi, Opt. Lett., 2011, 36, 2309–2311 CrossRef PubMed.
- C. H. Camp Jr., S. Yegnanarayanan, A. A. Eftekhar, H. Sridhar and A. Adibi, Opt. Express, 2009, 17, 22879–22889 Search PubMed.
- C. Zhang, K. C. Huang, B. Rajwa, J. J. Li, S. Q. Yang, H. N. Lin, C. S. Liao, G. Eakins, S. H. Kuang, V. Patsekin, J. P. Robinson and J. X. Cheng, Optica, 2017, 4, 103–109 Search PubMed.
- Y. Wang, Y. Ji, E. S. Wharfe, R. S. Meadows, P. March, R. Goodacre, J. Xu and W. E. Huang, Anal. Chem., 2013, 85, 10697–10701 CrossRef CAS PubMed.
- N. Pan, W. Rao, N. R. Kothapalli, R. Liu, A. W. Burgett and Z. Yang, Anal. Chem., 2014, 86, 9376–9380 CrossRef CAS PubMed.
- S. S. Rubakhin, W. T. Greenough and J. V. Sweedler, Anal. Chem., 2003, 75, 5374–5380 CrossRef CAS PubMed.
- Y. Schober, S. Guenther, B. Spengler and A. Rompp, Anal. Chem., 2012, 84, 6293–6297 CrossRef CAS PubMed.
- S. G. Ostrowski, M. E. Kurczy, T. P. Roddy, N. Winograd and A. G. Ewing, Anal. Chem., 2007, 79, 3554–3560 CrossRef CAS PubMed.
- S. A. Hofstadler, J. C. Severs, R. D. Smith, F. D. Swanek and A. G. Ewing, Rapid Commun. Mass Spectrom., 1996, 10, 919–922 CrossRef CAS PubMed.
- M. K. Passarelli, A. G. Ewing and N. Winograd, Anal. Chem., 2013, 85, 2231–2238 CrossRef CAS PubMed.
- W. Xie, D. Gao, F. Jin, Y. Jiang and H. Liu, Anal. Chem., 2015, 87, 7052–7059 CrossRef CAS PubMed.
- J. S. Mellors, K. Jorabchi, L. M. Smith and J. M. Ramsey, Anal. Chem., 2010, 82, 967–973 CrossRef CAS PubMed.
- F. Chen, L. Lin, J. Zhang, Z. He, K. Uchiyama and J. M. Lin, Anal. Chem., 2016, 88, 4354–4360 CrossRef CAS PubMed.
- M. H. Spitzer and G. P. Nolan, Cell, 2016, 165, 780–791 CrossRef CAS PubMed.
- Z. B. Bjornson, G. P. Nolan and W. J. Fantl, Curr. Opin. Immunol., 2013, 25, 484–494 CrossRef CAS PubMed.
- E. W. Newell and Y. Cheng, Nat. Immunol., 2016, 17, 890–895 CrossRef CAS PubMed.
- S. C. Bendall, G. P. Nolan, M. Roederer and P. K. Chattopadhyay, Trends Immunol., 2012, 33, 323–332 CrossRef CAS PubMed.
- D. R. Bandura, V. I. Baranov, O. I. Ornatsky, A. Antonov, R. Kinach, X. Lou, S. Pavlov, S. Vorobiev, J. E. Dick and S. D. Tanner, Anal. Chem., 2009, 81, 6813–6822 CrossRef CAS PubMed.
- E. Porpiglia, N. Samusik, A. T. Van Ho, B. D. Cosgrove, T. Mai, K. L. Davis, A. Jager, G. P. Nolan, S. C. Bendall, W. J. Fantl and H. M. Blau, Nat. Cell Biol., 2017, 19, 558–567 CrossRef CAS PubMed.
- L. Huang, Y. Chen, L. T. Weng, M. Leung, X. Xing, Z. Fan and H. Wu, Anal. Chem., 2016, 88, 12196–12203 CrossRef CAS PubMed.
- S. C. Bendall, E. F. Simonds, P. Qiu, A. D. Amirel, P. O. Krutzik, R. Finck, R. V. Bruggner, R. Melamed, A. Trejo, O. I. Ornatsky, R. S. Balderas, S. K. Plevritis, K. Sachs, D. Pe'er, S. D. Tanner and G. P. Nolan, Science, 2011, 332, 687–696 CrossRef CAS PubMed.
- G. K. Behbehani, S. C. Bendall, M. R. Clutter, W. J. Fantl and G. P. Nolan, Cytometry, Part A, 2012, 81, 552–566 CrossRef PubMed.
- E. R. Zunder, E. Lujan, Y. Goltsev, M. Wernig and G. P. Nolan, Cell Stem Cell, 2015, 16, 323–337 CrossRef CAS PubMed.
- E. Lujan, E. R. Zunder, Y. H. Ng, I. N. Goronzy, G. P. Nolan and M. Wernig, Nature, 2015, 521, 352–356 CrossRef CAS PubMed.
- J. C. Love, J. L. Ronan, G. M. Grotenbreg, A. G. van der Veen and H. L. Ploegh, Nat. Biotechnol., 2006, 24, 703–707 CrossRef CAS PubMed.
- C. M. Story, E. Papa, C. C. Hu, J. L. Ronan, K. Herlihy, H. L. Ploegh and J. C. Love, Proc. Natl. Acad. Sci. U. S. A., 2008, 105, 17902–17907 CrossRef CAS PubMed.
- Q. Han, E. M. Bradshaw, B. Nilsson, D. A. Hafler and J. C. Love, Lab Chip, 2010, 10, 1391–1400 RSC.
- A. J. Torres, R. L. Contento, S. Gordo, K. W. Wucherpfennig and J. C. Love, Lab Chip, 2013, 13, 90–99 RSC.
- A. J. Torres, A. S. Hill and J. C. Love, Anal. Chem., 2014, 86, 11562–11569 CrossRef CAS PubMed.
- Y. J. Yamanaka, G. L. Szeto, T. M. Gierahn, T. L. Forcier, K. F. Benedict, M. S. Brefo, D. A. Lauffenburger, D. J. Irvine and J. C. Love, Anal. Chem., 2012, 84, 10531–10536 CrossRef CAS PubMed.
- A. J. Hughes, D. P. Spelke, Z. Xu, C. C. Kang, D. V. Schaffer and A. E. Herr, Nat. Methods, 2014, 11, 749–755 CrossRef CAS PubMed.
- J. Vlassakis and A. E. Herr, Anal. Chem., 2015, 87, 11030–11038 CrossRef CAS PubMed.
- T. A. Duncombe, C. C. Kang, S. Maity, T. M. Ward, M. D. Pegram, N. Murthy and A. E. Herr, Adv. Mater., 2016, 28, 327–334 CrossRef CAS PubMed.
- C. C. Kang, J. M. Lin, Z. Xu, S. Kumar and A. E. Herr, Anal. Chem., 2014, 86, 10429–10436 CrossRef CAS PubMed.
- K. Eyer, S. Stratz, P. Kuhn, S. K. Kuster and P. S. Dittrich, Anal. Chem., 2013, 85, 3280–3287 CrossRef CAS PubMed.
- J. Sun, M. D. Masterman-Smith, N. A. Graham, J. Jiao, J. Mottahedeh, D. R. Laks, M. Ohashi, J. DeJesus, K. Kamei, K. B. Lee, H. Wang, Z. T. Yu, Y. T. Lu, S. Hou, K. Li, M. Liu, N. Zhang, S. Wang, B. Angenieux, E. Panosyan, E. R. Samuels, J. Park, D. Williams, V. Konkankit, D. Nathanson, R. M. van Dam, M. E. Phelps, H. Wu, L. M. Liau, P. S. Mischel, J. A. Lazareff, H. I. Kornblum, W. H. Yong, T. G. Graeber and H. R. Tseng, Cancer Res., 2010, 70, 6128–6138 CrossRef CAS PubMed.
- K. Kamei, M. Ohashi, E. Gschweng, Q. Ho, J. Suh, J. Tang, Z. T. For Yu, A. T. Clark, A. D. Pyle, M. A. Teitell, K. B. Lee, O. N. Witte and H. R. Tseng, Lab Chip, 2010, 10, 1113–1119 RSC.
- A. Salehi-Reyhani, J. Kaplinsky, E. Burgin, M. Novakova, A. J. deMello, R. H. Templer, P. Parker, M. A. Neil, O. Ces, P. French, K. R. Willison and D. Klug, Lab Chip, 2011, 11, 1256–1261 RSC.
- J. Shen, Y. Zhou, T. Lu, J. Peng, Z. Lin, L. Huang, Y. Pang, L. Yu and Y. Huang, Lab Chip, 2012, 12, 317–324 RSC.
- Y. S. Shin, H. Ahmad, Q. Shi, H. Kim, T. A. Pascal, R. Fan, W. A. Goddard 3rd and J. R. Heath, ChemPhysChem, 2010, 11, 3063–3069 CrossRef CAS PubMed.
- Y. S. Shin, F. Remacle, R. Fan, K. Hwang, W. Wei, H. Ahmad, R. D. Levine and J. R. Heath, Biophys. J., 2011, 100, 2378–2386 CrossRef CAS PubMed.
- Y. Lu, J. J. Chen, L. Mu, Q. Xue, Y. Wu, P. H. Wu, J. Li, A. O. Vortmeyer, K. Miller-Jensen, D. Wirtz and R. Fan, Anal. Chem., 2013, 85, 2548–2556 CrossRef CAS PubMed.
- M. Elitas, K. Brower, Y. Lu, J. J. Chen and R. Fan, Lab Chip, 2014, 14, 3582–3588 RSC.
- Y. Lu, Q. Xue, M. R. Eisele, E. S. Sulistijo, K. Brower, L. Han, A. D. Amir el, D. Pe'er, K. Miller-Jensen and R. Fan, Proc. Natl. Acad. Sci. U. S. A., 2015, 112, E607–E615 CrossRef CAS PubMed.
- L. Huang, S. A. Michael, Y. Chen and H. Wu, Chem. – Asian J., 2017, 12, 1680–1691 CrossRef CAS PubMed.
- C. Ma, R. Fan, H. Ahmad, Q. Shi, B. Comin-Anduix, T. Chodon, R. C. Koya, C. C. Liu, G. A. Kwong, C. G. Radu, A. Ribas and J. R. Heath, Nat. Med., 2011, 17, 738–743 CrossRef CAS PubMed.
- T. Konry, M. Dominguez-Villar, C. Baecher-Allan, D. A. Hafler and M. L. Yarmush, Biosens. Bioelectron., 2011, 26, 2707–2710 CrossRef CAS PubMed.
- S. Akbari and T. Pirbodaghi, Lab Chip, 2014, 14, 3275–3280 RSC.
- A. H. Ng, M. D. Chamberlain, H. Situ, V. Lee and A. R. Wheeler, Nat. Commun., 2015, 6, 7513 CrossRef CAS PubMed.
- Y. Gong, A. O. Ogunniyi and J. C. Love, Lab Chip, 2010, 10, 2334–2337 RSC.
- A. K. White, M. VanInsberghe, O. I. Petriv, M. Hamidi, D. Sikorski, M. A. Marra, J. Piret, S. Aparicio and C. L. Hansen, Proc. Natl. Acad. Sci. U. S. A., 2011, 108, 13999–14004 CrossRef CAS PubMed.
- A. M. Thompson, A. Gansen, A. L. Paguirigan, J. E. Kreutz, J. P. Radich and D. T. Chiu, Anal. Chem., 2014, 86, 12308–12314 CrossRef CAS PubMed.
- D. J. Eastburn, A. Sciambi and A. R. Abate, Nucleic Acids Res., 2014, 42, e128 CrossRef PubMed.
- P. Kumaresan, C. J. Yang, S. A. Cronier, R. G. Blazej and R. A. Mathies, Anal. Chem., 2008, 80, 3522–3529 CrossRef CAS PubMed.
- R. Zhang, H. Q. Gong, X. D. Zeng and C. C. Sze, Anal. Bioanal. Chem., 2013, 405, 4277–4282 CrossRef CAS PubMed.
- Y. Zhu, Y. X. Zhang, W. W. Liu, Y. Ma, Q. Fang and B. Yao, Sci. Rep., 2015, 5, 9551 CrossRef PubMed.
- Z. Zhu, W. Zhang, X. Leng, M. Zhang, Z. Guan, J. Lu and C. J. Yang, Lab Chip, 2012, 12, 3907–3913 RSC.
- D. J. Eastburn, A. Sciambi and A. R. Abate, Anal. Chem., 2013, 85, 8016–8021 CrossRef CAS PubMed.
- S. T. Lance, D. J. Sukovich, K. M. Stedman and A. R. Abate, Virol J., 2016, 13, 201 CrossRef PubMed.
- S. W. Lim, S. T. Lance, K. M. Stedman and A. R. Abate, J. Virol. Methods, 2017, 242, 14–21 CrossRef CAS PubMed.
- S. Ma, T. W. Murphy and C. Lu, Biomicrofluidics, 2017, 11, 021501 CrossRef PubMed.
- B. Treutlein, D. G. Brownfield, A. R. Wu, N. F. Neff, G. L. Mantalas, F. H. Espinoza, T. J. Desai, M. A. Krasnow and S. R. Quake, Nature, 2014, 509, 371–375 CrossRef CAS PubMed.
- O. Gokce, G. M. Stanley, B. Treutlein, N. F. Neff, J. G. Camp, R. C. Malenka, P. E. Rothwell, M. V. Fuccillo, T. C. Sudhof and S. R. Quake, Cell Rep., 2016, 16, 1126–1137 CrossRef CAS PubMed.
- J. R. Priest, C. Gawad, K. M. Kahlig, J. K. Yu, T. O'Hara, P. M. Boyle, S. Rajamani, M. J. Clark, S. T. Garcia, S. Ceresnak, J. Harris, S. Boyle, F. E. Dewey, L. Malloy-Walton, K. Dunn, M. Grove, M. V. Perez, N. F. Neff, R. Chen, K. Maeda, A. Dubin, L. Belardinelli, J. West, C. Antolik, D. Macaya, T. Quertermous, N. A. Trayanova, S. R. Quake and E. A. Ashley, Proc. Natl. Acad. Sci. U. S. A., 2016, 113, 11555–11560 CrossRef CAS PubMed.
- B. J. DeKosky, G. C. Ippolito, R. P. Deschner, J. J. Lavinder, Y. Wine, B. M. Rawlings, N. Varadarajan, C. Giesecke, T. Dorner, S. F. Andrews, P. C. Wilson, S. P. Hunicke-Smith, C. G. Willson, A. D. Ellington and G. Georgiou, Nat. Biotechnol., 2013, 31, 166–169 CrossRef CAS PubMed.
- H. C. Fan, G. K. Fu and S. P. Fodor, Science, 2015, 347, 1258367 CrossRef PubMed.
- S. Bose, Z. Wan, A. Carr, A. H. Rizvi, G. Vieira, D. Péer and P. A. Sims, Genome Biol., 2015, 16, 120 CrossRef PubMed.
- J. Yuan and P. A. Sims, Sci. Rep., 2016, 6, 33883 CrossRef CAS PubMed.
- T. M. Gierahn, M. H. Wadsworth Ii, T. K. Hughes, B. D. Bryson, A. Butler, R. Satija, S. Fortune, J. C. Love and A. K. Shalek, Nat. Methods, 2017, 14, 395–398 CrossRef CAS PubMed.
- Y. Marcy, C. Ouverney, E. M. Bik, T. Losekann, N. Ivanova, H. G. Martin, E. Szeto, D. Platt, P. Hugenholtz, D. A. Relman and S. R. Quake, Proc. Natl. Acad. Sci. U. S. A., 2007, 104, 11889–11894 CrossRef CAS PubMed.
- Z. Yu, S. Lu and Y. Huang, Anal. Chem., 2014, 86, 9386–9390 CrossRef CAS PubMed.
- K. Leung, A. Klaus, B. K. Lin, E. Laks, J. Biele, D. Lai, A. Bashashati, Y. F. Huang, R. Aniba, M. Moksa, A. Steif, A. M. Mes-Masson, M. Hirst, S. P. Shah, S. Aparicio and C. L. Hansen, Proc. Natl. Acad. Sci. U. S. A., 2016, 113, 8484–8489 CrossRef CAS PubMed.
- F. Lan, B. Demaree, N. Ahmed and A. R. Abate, Nat. Biotechnol., 2017, 35, 640–646 CrossRef CAS PubMed.
- Z. Cao, C. Chen, B. He, K. Tan and C. Lu, Nat. Methods, 2015, 12, 959–962 CrossRef CAS PubMed.
Footnote |
† These two authors have contributed equally to this work. |
|
This journal is © The Royal Society of Chemistry 2018 |