Electrode–particle impacts: a users guide
Received
14th November 2016
, Accepted 22nd November 2016
First published on 22nd November 2016
Abstract
We present a comprehensive guide to nano-impact experiments, in which we introduce newcomers to this rapidly-developing field of research. Central questions are answered regarding required experimental set-ups, categories of materials that can be detected, and the theoretical frameworks enabling the analysis of experimental data. Commonly-encountered issues are considered and presented alongside methods for their solutions.
1 Introduction
The nano-impact method is a powerful tool that enables the characterisation of individual particulate nano-objects in solution and study of their reactivity. During a typical experiment an electrode under potential control is immersed into a solution containing a freely-diffusing analyte. By virtue of their Brownian motion, the analyte particles (often nanoparticles) will stochastically collide with and impact on the electrode surface where they may for instance adsorb, react, or enable a catalytic reaction. The presence of the analyte can then be recognised in the electrode current and properties of the analyte particle population may be extracted from the measurement.1–6
The general experimental setup for most types of impact experiments involves a three electrode system with a working electrode of micron dimensions (typically an embedded microdisc) held at a potential at which the reaction of interest takes place, an inert counter electrode and a reference electrode (typically a Saturated Calomel Electrode7 (SCE) in aqueous solutions). Supporting electrolyte is used to improve the conductivity of the solution and to compress the double layer at the electrode.8,9 Consequently, the interfacial electric field is confined to within close proximity to the electrode. Current transients are recorded and characteristic ‘spikes’ (blips) or ‘steps’ are observed as a result of collisions of diffusing analyte particles at the electrode. The technique has been applied to a wide range of entities ranging from inorganic/organic nanoparticles10–18 to complex structures such as viruses,19,20 bacteria21,22 and red blood cells.23 In addition voltammograms can be recorded at single particles as demonstrated by Li et al. for the case of hydrogen oxidation on palladium functionalised carbon nanotubes.24
A number of overviews of recent advances in nanoparticle electrochemistry1–4 are available. The present work is intended to complement the excellent review5 by Rees et al. in PCCP with the main aim of highlighting relevant theoretical models, addressing experimental challenges, and providing a guide to experiment design. Initially we describe different types of experimental systems, after which we discuss the theoretical modeling of diffusive nanoparticle transport to an electrode. We then proceed with a discussion of various design features of the experimental set-up, including particle characterization tools, potentiostat requirements, types of electrodes, and the choice of the supporting electrolyte. The final sections provide examples of the systems that have been investigated to date and by an outlook on the future of this promising research field.
1.1 Type of impacts
Modern nano-impact experiments are based on pioneering experiments by Micka25 in Prague as early as 1956 who used mercury electrodes to study insoluble HgS, PbS, CuS and Ag2O particles suspended in water. Micka observed a cathodic peak in a classic damped polarographic wave in a stirred solution which was due to multiple simultaneous collisions of the particles. An example of such polarogram is shown in Fig. 1 and corresponds to the reduction of multiple colliding HgS particles in a stirred solution. Similar observations were later made by Jones and Kaye,26 who investigated the undamped response and observed large, sharp current spikes as a result of multiple simultaneous collisions of carbon particles taking place. The observed undamped polarogram is shown in Fig. 2.
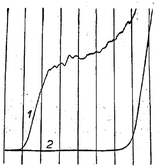 |
| Fig. 1 Damped polarograms of HgS particles in solution as recorded by Micka,25 (1) stirred solution (2) unstirred solution. | |
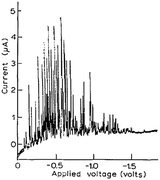 |
| Fig. 2 Undamped polarogram of a stirred suspension of 0.01% activated charcoal in 0.1 M KCl solution as recorded by Jones and Kaye.26 | |
Practical applications of electrode particle electrochemistry were introduced by Moller in 197127 and further advanced by Holland and Feinerman, who developed a device which involved a turntable holding immobilized silver bromide particles and a platinum needle electrode. The turntable was rotated slowly and upon contact with the electrode, silver bromide particles were reduced, thereby allowing the grain size to be determined.28,29 This equipment found significant use in the photographic industry for the characterisation of micron and sub-micron sized AgBr particles. Fig. 3 shows the particle size analyzer device developed and the electrode mount. It is insightful to note that the authors discussed the possibility of studying silver particles using the same technique by reversing the polarity of the electrode.28 Almost 25 years passed before further developments took place. Heyrovsky et al. again in Prague conducted polarographic experiments using particles of TiO2, SnO2 and Fe2O3 and observed the ensemble current response arising from the catalytic reduction of H+ at impacting particles.30–33 In contrast recent work has focused on studying the response of single particle collisions.
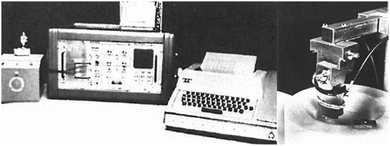 |
| Fig. 3 Automatic particle size analyzer for AgBr particles as designed by Holland and Feinerman.28 | |
Electrode/particle collisions are herein classified into four broad categories depending on the reaction taking place: direct Faradaic, mediated Faradaic, capacitive, and blocking impacts. Various impact events are capable of providing diverse specific information regarding the nature of the impacting particle, the kinetics of the electron transfer,34,35 and the catalytic activity of a given particle. However, in order to understand the nature of relevant physiochemical processes in nano-impacts it is essential to consider the effects of the double layer, the charge transfer mechanisms, and the surface reactions involving the impacting particles. We first provide an overview of the types of impacts by comparing and contrasting capacitive and direct Faradaic impacts, before we proceed to discuss mediated Faradaic and blocking impacts.
1.1.1 Direct Faradaic impacts.
Faradaic impacts involve electron transfer between the particle and the electrode and result in the occurence of a redox reaction: in the case of a direct Faradaic impact the colliding analyte is itself either oxidized or reduced, and in some cases may dissolve. This is in contrast to mediated Faradaic impacts (1.1.3) where the redox state of the impacting analyte is unaltered and the particle serves to mediate a redox reaction of interest (proton reduction or hydrazine oxidation, for example). In 2011, the electrochemical detection and sizing of individual silver nanoparticles was demonstrated.36Fig. 4, schematically depicts the direct Faradaic impact event. Taking the oxidation of silver nanoparticles36 as a model system the electrode reactions may be described by the following equation:
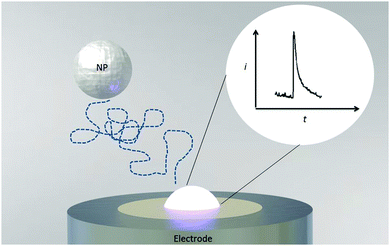 |
| Fig. 4 Schematic diagram of a Faradaic impact. The particle arrives at the electrode by virtue of its Brownian motion and subsequently dissolves at the electrode as a result of electron transfer. The inset shows the characteristic “spike-like” observed current response. | |
If the particle is oxidized (or reduced) completely, then the integral of the current passed during an impact event gives the overall charge transferred, which allows the number of silver atoms contained within a particle to be measured. Typical dissolution behavior is observed for metallic nanoparticles such as silver,37 gold,38,39 nickel40,41 and copper,42 while in other cases the particle can be converted to water soluble compounds (for example reduction of indigo to leuco-indigo13). Assuming the density of the material is not significantly altered from that of the bulk then the particle's individual volume can be directly evaluated. Under the assumption of spherical geometry this allows the effective radius of the particle to be ascertained:
|  | (2) |
where
r is the radius of the nanoparticle,
M is the molar mass,
F is the Faraday constant,
z is the number of electrons transferred per atom in the particle and
ρ is the density of the nanoparticle.
1.1.2 Capacitative impacts.
Capacitative impacts can be separated into two distinct classes, arising either due to the displacement of charge from the electrode–electrolyte interface (double layer) or from the charging of an impacting nanoparticle. In neither process does a redox reaction take place. In order to maintain charge-neutrality the electrons can leave or enter the bulk of the electrode in response to the changes in the ionic charge distribution at the interface. Large capacitive impacts are hence observed for particles featuring large surface areas. Capacitative spikes show a distinct potential dependence with a steady decrease of the spike integral as the applied potential at the working electrode approaches the potential of zero charge (PZC).43,44 Capacitative nano-impacts have been successfully observed for graphene platelets and used to estimate the PZC and the diffusion coefficient.45 The process for the capacitive impact causing the perturbation of an electrode double layer is shown schematically in Fig. 5. The adsorbed particle disrupts the electrode double layer, and after the particle leaves the electrode double layer is restored. Fig. 5 shows a second type of capacitive impact caused by the charging of the impacting particle. It is noted that in principle any particle can show capacitive impacts, including non-conductive ones, due to their ability to alter the double-layer structure at an electrochemical interface with larger particles showing a greater signal.
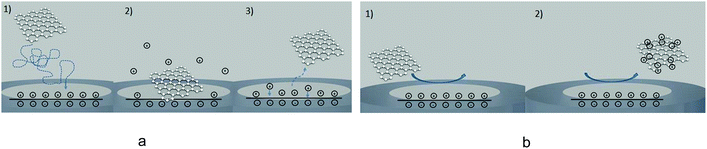 |
| Fig. 5 Two types of capacitive impacts. (a) Initially the impacting particle causes perturbation of the electrode double layer, after the particle leaves the double layer is restored. (b) Impacting particle is charged as it approaches an electrode and leaves the electrode surface. | |
1.1.3 Mediated Faradaic impacts.
Mediated Faradaic impacts rely on the presence of a redox active couple in the solution phase and are therefore also known as a catalytically-amplified impacts. At the chosen electrode potential the reaction does not take place at the electrode due to kinetic limitations, but upon collision with the electrode the nanoparticle adopts the electrode potential and a reaction at its surface is enabled leading to a current spike. The current features can be short- or long lived resulting in either spiked or stepped current responses (see Fig. 6) often reflecting the period of time that the particle is in contact with the electrode. In favorable circumstances the magnitude of the current yields a direct measure of the reaction rate at the individual particle, hence short lived electrochemical events may reflect a particle leaving the electrode surface or a decreases in the catalytic activity of the material as the reaction progresses. Bard et al.46–48 developed this technique using catalytically-active Pt nanoparticles in acidic solution and an inert carbon fiber electrode. For example proton reduction took place whenever a nanoparticle impacted and formed an electrical contact, during which a current plateau was recorded. The characteristic ‘step’ in the chronoamperogram is due to the reaction continuously taking place at the surface of the particle. In contrast for direct Faradaic impacts, the electroactive particle is consumed with time and the current decays throughout the process. Since the initial publication in 2007, the catalytic amplification approach has been extended and applied to a wide range of systems including: iridium oxide,49 DNA-functionalised platinum nanoparticles,50 TiO251 and ruthenium oxide nanoparticles.52 In addition the mediated Faradaic impacts approach has been used by Li et al.24 to monitor the hydrogen oxidation reaction (HOR) on single carbon nanotubes functionalised with palladium nanoparticles. The long residence time of the particle at the electrode allowed the recording of voltammograms of single carbon nanotubes and the observation of current fluctuations due to the movement of the tube.
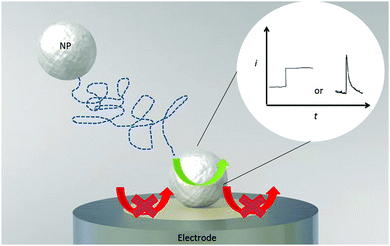 |
| Fig. 6 A schematic diagram of a mediated Faradaic impact. Arrows indicate the catalytic reaction that takes place upon collision of the particle with the electrode. The inset shows the observed step- or spike-like current response. | |
1.1.4 Blocking impacts.
An alternative form of impacts is the observation of electrode blockage by arriving particles. Initial experiments, reported in 2004 and pioneered by Lemay et al.53 demonstrated the amperometric detection of single carboxylated micro- and nano-spheres adsorbing on micro- and nano-electrodes. In such cases the reduction or oxidation of a solution phase redox active species (such as ferrocene methanol) is performed at the detecting electrode under conditions of relatively low supporting electrolyte and in the presence of the solution phase analyte (particle). An impacting non-conductive particle causes a decrease (‘blocking’) in the surface area of the electrode and a corresponding reduction in the diffusion limited current of the redox species is observed, leading to a step in the chronoamperogram.54,55 On the basis of the step height, the size of the impacting particle can be inferred. However, information regarding the concentration of the particles present in solution, from the observed frequency of the steps is complicated by the influence of migration on the mass-transport of the analyte (particles) to the electrode surface.
2 Theory
Experimental data acquired in nano-impact experiments reflects the complex interplay of various physical effects, which most significantly include the particle's mass transport, its charge transfer characteristics at the electrode, and the effect of the employed analogue and digital measurement equipment. Understanding the influence of these key factors is crucial for interpretation of experimental data. In the following we separately review the underlying physical concepts. We commence the section with a discussion of the relevant mass transport mechanisms and an introduction to the most significant theoretical models thereof. These models are then further elucidated from a statistical physics point of view, where we investigate the mass transport with respect to first passage statistics to provide guidelines to experimentalists: in sensing applications, nano-impact experiments can, for instance, be used to determine the concentration of an analyte in solution counting the number of observed impacts in a defined time interval. Since this measured number is non-trivially linked to the mass transport model and subject to stochastic fluctuations, we herein provide look-up tables that immediately link both the analyte concentration and the number of observed impacts for common experimental conditions. Subsequently, we briefly discuss the influence of analogue laboratory equipment on measured data and elucidate related challenges and opportunities in the analysis of spike shapes in chronoamperometric measurements.
2.1 Mass transport
Nano-impact experiments are typically carried out in solutions with high electrolyte concentrations. This is mainly due to two reasons: on the one hand, such electrolyte concentrations elevate the conductivity of the solution to a level that allows good coupling between the solution- and the reference potential throughout the entire bulk. On the other hand, the electrolyte enables the formation of double layers near charged surfaces, which establishes the sharp potential drop at the charged electrode that is required to drive the chemical reactions during the impact. In addition, the formation of double layers effectively screens all electric fields in solution, which crucially influences the mass transport of nano-scaled analytes in the nano-impact method: though the freely-diffusing analyte may frequently be located near charged surfaces, it is not affected by electromigration forces as double layers typically feature thicknesses of only a few Ångströms, if high electrolyte concentrations are present. Furthermore, the influence of convection is negligible in many nano-impact experiments and can be neglected to good approximation. This approximation is on the one side due to the small size of the system and on the other due to the only small change in the density of the analyte during the reaction. Hence, the mass transport of the analyte often does not require solving Nernst–Planck– and Navier–Stokes equation but can be fully described by the diffusion equation |  | (3) |
where c is the concentration of the analyte at the time t and
the position at which c is evaluated, and Dij is the tensor of the diffusion coefficient. For the case of isotropic diffusion, i.e. the diffusion characteristics do not change with the direction of the diffusion and the diffusion coefficient is independent from the diffusing object's position, eqn (3) simplifies to the more commonly known form: |  | (4) |
For a spherical particle the diffusion coefficient D∞ in bulk solution may be estimated via the Stokes–Einstein equation, which was independently developed by Einstein56 and Sutherland:57 |  | (5) |
where kB is the Boltzmann constant, T is the temperature, η is the viscosity and rp is the radius of the diffusing particle. It is however noted, that this diffusion coefficient is only valid in the absence of any diffusion boundaries. Otherwise the diffusion is anisotropic and D has to be evaluated as a tensor rather then a scalar. In particular the diffusion coefficients for diffusion orthogonal and in parallel to a boundary vary with the distance of the diffusing particle from the wall. Solutions for the perpendicular case are provided by Brenner58 and approximated in a convenient form by Bevan et al.:59 | 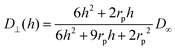 | (6) |
where h is the distance between the edge of the particle and the wall. The case of parallel diffusion was investigated by Faxen60via61 and can be mathematically treated as: | 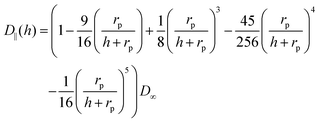 | (7) |
Once found on the basis of eqn (3) or (4), the mass transport equations can be solved via a variety of analytical, computational, and Monte–Carlo methods. Among these analytical approaches as well as computational methods are commonly employed, while particularly finite differences and finite elements are prominent as they provide robust computational tools for this purpose. The modeling of a systems stochastic properties such as its noise characteristics or the first passage statistics of an individual diffusing entity may however be challenging if investigated via analytical approaches, finite differences, or finite elements: in all methods time-dependent concentration profiles are determined, which represent the probability of finding one or multiple diffusing objects at a certain position and time rather than an actual position. While this is acceptable for most electrochemical experiments,9 in single-analyte methods like nano-impacts it is however generally more desirable to model actual analyte pathways to answer questions like: ‘When does the nanoparticle reach the electrode for the first time and what is the related variance?’ or ‘A certain number of nanoparticles were detected in a given time interval. What is their concentration in solution?’. Stochastic information on individual objects may however not be readily available from solutions to the mass transport equations. For this reason, stochastic modeling via random walks62–64 has received growing attention in recent years and turned out to be a particularly useful tool in nano-impacts.65 Rather than concentrations profiles, this computational technique models each object's diffusive pathway individually in accordance to eqn (3) or (4). By this means, sets of obtained pathways can be analysed statistically and the desired stochastic information is revealed.
2.2 Predicting the number of impacts
Modeling the above mass transport equations enables the prediction of the number of impacts or the average frequency of impacts observed in the experiment. The size and type of the electrode, the analyte concentration, the particles size of interest, and the duration of the experiment are herein all parameters that can be optimised to allow the detection of the particle of interest with statistical significance. The number of impacts as function of time then provides valuable information on the transport of the particles to the electrode and can give insight into other processes that may affect the flux towards the electrode such as aggregation, dispersion of the particles, blockage of the electrode, and compete adsorption.
As discussed above, the main mechanism of particle transport in a conventional experiment is diffusion, and therefore the prediction of the number of impacts or the average impact frequency can be first estimated through calculating the diffusion rate towards an electrode for the case of fully dispersed particles.
2.2.1 Bulk diffusion as a first principle.
A rough estimate of the number of impacts as a function of time can be determined by using standard expressions for the diffusive flux of molecular species to an electrode, which neglect the effect of near-wall hindered diffusion.66
Microdisc electrodes.
The frequency of impacts can be evaluated from the diffusive flux to a finite disc electrode. Saito found the steady-state flux towards a disc electrode to be:67where rd is the disc radius and c* is the particle number concentration (particles m−3). However, assuming a steady state can introduce a relatively large error, especially when large particles or short experiments are considered and relatively large disc radii of few micrometre are employed. To overcome these issues, the time-dependent diffusion equation (eqn (4)) can be solved numerically. Shoup and Szabo approximated this numerical solution for the flux to be:68where | f(τ) = 0.7854 + 0.8862τ−1/2 + 0.2146 exp(−0.7823τ−1/2) | (10) |
and τ is a dimensionless time parameter, which is defined as:This expression is widely used and provides an accuracy within 0.6% compared to simulations by Heinze.69
Following that, the number of impacts as a function of time can be estimated by the integration of the diffusive flux towards a disc with respect to t:
| impacts(t) = c*rd3F(τ) | (12) |
Using a Taylor expansion
F(
τ) can be approximated to:
66 |  | (13) |
For the convenience of experimentalists, the calculated data for the number of impacts as function of the disc- and the particle size are provided in
Table 1.
Table 1 Number of impacts at fully adsorbing (a) disc and (b) wire electrodes (l = 1 mm) within a time-interval of 100 s in a solution comprising a particle concentration of 1 pM. The diffusion coefficient for different particles sizes were calculated in accordance with the Stokes–Einstein–Sutherland equation
(a) Disc radius |
0.5 μm |
3.5 μm |
16.5 μm |
Particle radius (nm) |
No. of impacts (non-hindered/hindered) |
5 |
5.93/5.01 |
42.8/42.18 |
228.61/228.5 |
25 |
1.19/0.65 |
8.94/7.16 |
54.2/53.1 |
50 |
0.6/0.23 |
4.61/3.63 |
30.27/28.23 |
150 |
0.2/0.036 |
1.66/0.89 |
12.68/10.07 |
300 |
0.1/0.01 |
0.89/0.32 |
7.54/7.27 |
500 |
0.06/0.003 |
0.57/0.14 |
5.19/4.78 |
1000 |
0.03/0.001 |
0.32/0.043 |
3.12/0.91 |
(b) Wire radius |
1.5 μm |
3.5 μm |
Particle radius (nm) |
No. of impacts (non-hindered/hindered) |
5 |
4848/4751 |
7003/6966 |
25 |
1224/1184 |
1957/1905 |
50 |
687/635 |
1150/1093 |
150 |
285/217 |
512/436 |
300 |
166/99.1 |
316/226 |
500 |
136/50.5 |
224/127 |
1000 |
100.6/17.7 |
144/50 |
2.2.2 Microwire electrodes.
The flux towards a wire is characterised by a slow decay in time and no true steady-state as opposed to the case of a microdisc electrode. Szabo70 approximated the transient flux towards a semi-infinite hemi-cylinder to:where l is the length of the cylinder and f(τ) is: | 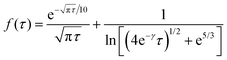 | (15) |
The expression is found to be accurate to within 1.3% and can be conveniently used for our purpose. In the context of impacting particles, the expression of the flux is integrated with respect to time to give the number of impacts as a function of time:66 | impacts(t) = 2πc*lrc2F*(τ), τ = Dt/rc2 | (16) |
where c* is the particle number concentration, l is the length of the cylinder, and rc is the cylinder radius. Note τ from the equation above (eqn (16)) differs by a factor of 4 compared to τ defined in eqn (11). For short τ < 1000, F* is found that: |  | (17) |
while for long τ, F* can be approximated to: |  | (18) |
The calculated number of impacts as function of the wire- and the particle size are also given in Table 1.
2.2.3 Variance and minimum time of detection.
The process of particles arriving from the bulk to the electrode has been shown to follow a Poisson process and its standard deviation can thus be calculated simply as
.71 By setting the equations for number of impacts (eqn (12) and (16)) to unity
impacts(t) = 1, the variance and the standard deviation are hence equally unity.66 This value can provide a practical estimation of the minimum required time for detection for a given set of experiments parameters, which is highly relevant in dilute solutions in the fM region, large particle sizes, and small electrodes below one micrometre.
We can compare the minimum detection time of the two types of electrodes, the wire and the disc electrode, by plotting the minimum detection time as a function of the electrode area. Fig. 7 shows the results for a 10 fM solution as a representative case for very dilute solutions. The thickness of the microwire is fixed to a representative value of 1 μm whilst the length (l) varies with the areas. The lower values for the micro-wire electrode show the possible advantage of using microwires in experiments at ultra-low concentrations and large nanoparticles. Additionally, in the case of ultra-dilute solution of 1 fM concentration of 25 nm particles, a microwire electrode featuring a length of 1 mm and a radius of 1 μm gives a minimum time for detection of approximately 90 s. However, for a microdisc electrode that has the same total surface area of 6.28 × 10−9 m2 (radius of a disc set to 44.7 μm), an impractical value of approximately 660 s is calculated.
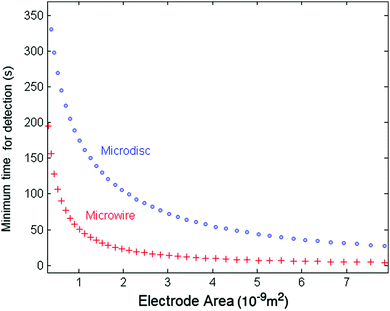 |
| Fig. 7 Minimum measurement time required for the detection of an average of one nanoparticle at a concentration of 10 fM. The particle size is set to 25 nm and the radius of the cylinder is set to 1 μm for all areas. | |
2.2.4 Inclusion of hindered diffusion.
Recently, we have seen that hindered diffusion can have a strong influence on the number of impacts when using micro-sized electrodes,72 which is due to the influence of near-wall hindered diffusion when the characteristic thickness of the diffusion layer is small. In fact, the smaller the electrode size, the thinner the diffusion layer, and hence, the larger the influence of near-wall hindered diffusion.73 The strong effect of hindered diffusion has been also realized and modeled under convective force in rotating disc electrode systems, where the diffusion layer is compressed significantly even for macro-sized electrodes.74
The calculation of heterogeneous diffusion in typical cells often employed for impact studies has been detailed in recent works for the case of a wire electrode and for two-dimensional anisotropic diffusion towards a microdisc73 (see also the diffusion coefficient expressed by eqn (6) and (7)). The diffusion problem can be solved using numerical analysis tools and requires careful modeling and convergence tests.73 However, for the convenience of the experimental researcher, we have calculated the number of impacts expected for the case of a standard concentration of 1 pM and various sizes of common electrode discs (Table 1a) and micro-wire electrodes (Table 1b). The results are shown in Table 1 where they are additionally compared to the calculations without hindered diffusion. It shows that when the ratio of particle- to the electrode size (rp/rd) increases, hindered diffusion becomes more dominant and the number of impacts decreases. The data in the table shows the case of a 1 pM concentration, but can be scaled to include other concentrations. The time can be also approximated by a linear scaling to give a reasonable estimate of the number of impacts in various experiments. The wire results consider a 1 mm wire length but can be scaled as well to cover various wire lengths.
Although the results give a good estimation of the number of impacts expected for diffusive mass transport, in many cases the number of impacts observed in experiments can vary due to various physical reasons. Agglomeration of particles, possible blocking of the electrode, adsorption on the insulating sheath of a microdisc electrode, migration, and convection are all factors that have been shown to have a significant influence on the prediction of the number of impacts in certain systems and should therefore be considered in the interpretation of experimental data. These possible effects are discussed further in Section 3.
2.2.5 Influence of an insulating sheath.
In the case of microdisc electrodes a decrease in the number of impacts from these predicted by diffusion only can be seen due to competitive adsorbing processes. Microdisc electrodes are usually fabricated by insulating a micron sized metal wire in glass or some other non-conducting material, where the sheath (insulator) is usually of the order of a millimetre thick. When analysing the current response, it is usually assumed that the insulating sheath plays no role. In the case of rapidly diffusing molecular solute this is an excellent approximation. However, in the context of nano-particles, this may no longer be the case. If particles adsorb and stick to the insulating surface, the flux of particles to the microdisc electrode is shielded. This shielding effect can change the prediction of the number of impacts even at small rates of adsorption on the insulating sheath. It was shown that in the case of maximum adsorption on the insulating surface, i.e. in the case that any particle which arrives at the surface sticks to it, the steady state flux towards the disc electrode becomes:75 | 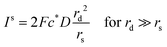 | (19) |
where rd is the disc radius and rs is the insulation radius.
Since the steady state flux towards a disc is given by:
the number of impacts in the case of a fully sticking surface can be roughly estimated as:
| 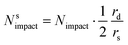 | (21) |
From this expression it is shown that the effect may have a drastic influence in a typical micron-sized disc with an insulating sheath of a millimetre size. This effect should be taken into account when interpreting related experimental data.
2.3 Analysis of current signals
We have seen that in nano-impact experiments, the analyte concentration can be estimated from the frequency of impacts measured, if first passage times are evaluated on the basis of the respective mass transport equations. Aside from that, is there other information that can be extracted from experimental data? Various physiochemical aspects of the impact process are reflected in the measured current spike shape, though they may be masked by the instrument function. The following section first addresses the topic of spike shapes, before the influence of analogue laboratory equipment on the spike shape is investigated.
2.3.1 Spike shapes and sizes.
Early nano-impact papers revealed that the integral of a spike, i.e. the total charge passed, may provide information on the impacting particle's volume. If the particle entirely reacts during the contact with the electrode and a known number of elementary charges is transferred per electroactive unit (for example a silver atom within a silver nanoparticle) within a nanoparticle, the absolute number of such units can be directly concluded from the current measurement. This is for instance the case for the oxidation of some silver nanoparticles, where nano-impact experiments enable measuring a particle populations' size distribution through only a single experiment.36 More recent experimental and theoretical studies further demonstrated that information beyond the concentration and the size distribution can be extracted. Some of these are listed below:
• Dissolution
A common type of nano-impact experiments is the detection of particles that dissolve upon impacting at the electrode, where the adopt the electrode potential and an electro-dissolution process of the formerly stable nanoparticle is enabled. The charge transfer at the electrode is then determined by the propagation of dissolution process, which can either be diffusion- or electron transfer rate-limited. Recent theoretical work76 established and solved related differential equations, which allow the analytical description of expected the spike shape and size.
• Mediated processes
Mediated impacts enable a catalytic reaction between the electrode and analyte in solution, which does not react with the electrode itself, and result in a pulse- or step-like current responses. The duration of the spikes then reflects the residence time of the particle within the tunneling-distance of the electrode, while the spike height provides insight into the particle's catalytic activity: this activity is often limited by the diffusion of analyte towards the particle and the step height can hence be determined by solving the respective mass transport equations. Solutions can be achieved analytically77 or computationally,78 providing the following expression for the steady-state current to a sphere on a flat substrate:
where
n is the number of electrons transferred per analyte molecule,
F is the Faraday constant,
D is the diffusion coefficient of the analyte,
r is the particle radius, and
c* is the analyte concentration. If more complex charge transfer kinetics are present, an analysis alongside the approach
79 by Kahk
et al. can be employed to evaluate reaction rates at the nanoparticle using the potential dependence of the spike current observed.
42
• Electro-doping
A recent technique80 is the electrochemically-driven doping of individual impacting nanoparticles. Herein, the charge transfer between the nanoparticle and the electrode is not determined by diffusion of the analyte in solution but by the mass transport of doping ions inside the nanoparticle, which releases a discrete amount of charge to the electrode for each ion entering it. As the nanoparticle only features a limited uptake capacity and ions may enter or leave the particle at high rates, the current response of the electrode can be modeled on the basis of the mass transport equations of ions inside the nanoparticle, yielding an analytical solution for the spike shape.80
• Surface diffusion
Another rate-determining aspect of the charge transfer between the electrode and the impacting nanoparticle may be the charge diffusion on the particle surface. This is for instance observed at impacting alumina particles modified with catechol, where the reaction rate is determined by the charge diffusion through the layer of adsorbed catechol molecules since the particle itself is insulating.81 The spike shape can then be analytically described by solving the mass transport equations for diffusion on a sphere, which provides a current transient that greatly matches experimental data. Aside from diffusion on a sphere, related equations can be solved for a number of other relevant geometries as recently demonstrated by Eloul and Compton in a computational study.82
2.3.2 Influence of analogue laboratory equipment.
The analysis of current spikes is complicated by the unwanted influences of the electronics used in the experimental set-up: the measured current generally does not describe the charge transfer at the interface as it is theoretically modeled, but provides rather a fingerprint of it transformed by the analogue and digital circuitry. Numerous sources may contribute to the alteration of the initial signal. Usually most significant is the analogue filters that are implemented in the potentiostat to filter noise caused by external sources. These filters are helpful if, for instance, voltammograms are recorded but may sometimes equally remove effects under investigation. Parasitic capacitances in cables, plugs, or similar as well as the analogue-digital converter used in the potentiostat may further alter the signal. The combination of all these influences may ultimately alter the signal in a way that a recorded current spike shape may be entirely different from the actual charge transfer at the interface. A detailed characterisation of the measurement electronics and the understanding thereof is hence crucially required!
The influence of the electronic circuitry on the measured signal can be conveniently characterised via the system's transfer function H, which links the time-dependent input signal x(t) and the circuitry's output signal y(t) as follows:83
| 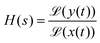 | (23) |
where is the Laplace transform and
s the complex number frequency parameter related to the Laplace transform. If
H(
s) is known,
eqn (23) may then be used to recover the original electrode signal. Depending on the transfer function and the intrinsic noise of the circuitry such recovery may however be obstructed and the desired information may be lost. In many cases, the potentiostat's analogue filters further dominate the transfer function, which may simplify the analysis drastically as it can often be determined.
It is noted that in the context of nano-impact experiments the choice of certain filter types may often be advantageous if the spike integrals are of interest: if for instance Bessel- or Butterworth type filters are used, though the spike shape may be altered significantly, the integral of the spike is nonetheless conserved and can be readily used to determine the size distribution of a particle population if the charge transferred per impact reflects the particle size. To the advantage of experimentalists in nano-impacts, Butterworth- and Bessel filters are commonly employed in potentiostats for other reasons, namely their maximally flat frequency response in the passband and maximally linear phase response, respectively, and are hence often readily available in standard experimental set-ups. Fig. 8 illustrates the responses of these two filter types to a normalised rectangular pulse and demonstrates that the magnitudes of the spike integrals are retained. Even though the response exhibits some ‘overshoot’, all integrals feature a value of one if both positive and negative spike features are considered during the integration.
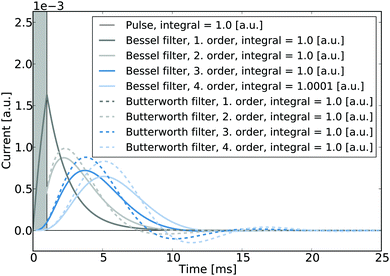 |
| Fig. 8 Responses of various Bessel- and Butterworth filters operated at a cut-off frequency of 100 Hz to a pulse featuring a length of 1 ms and an unity integral. The integrals of the individual filter responses are evaluated in the inset. It is noted that for clarity currents and charges are given in arbitrary units that are normalised to a pulse integral of unity. | |
3 Experimental setup and design
Having established the theoretical understanding of mass transport processes and expected frequencies of impacts, this section provides experimental details, which allow the technique to be applied to a large number of potential usage cases.
3.1 Particle characterization
In order to gain insights into particle systems via the nano-impact method, prior to electrochemical experiments accurate particle characterization is crucial. A wide range of techniques have been developed alongside the fast growth of the nanotechnology field. In this section we briefly discuss particle characterization methods and their applicability in the context of nano-impact experiments.
3.1.1 Electron microscopy.
Transmission and scanning electron microscopy (TEM and SEM) are the most common imaging techniques used for sizing. The resultant image data is easily interpreted, while particularly size- and fine structural data can be obtained through the use of high resolution TEM. The main disadvantage of electron microscopy is the relative high cost and that it is usually performed ex situ and in vacuo. A particular problem for the nanoparticle characterization is aggregation/agglomeration due to sample preparation, which involves the removal of solvent and drying.84 In addition electron microscopy does not provide kinetic information about nanoparticle aggregation or agglomeration; although there are reports of cryo85 and liquid scanning transmission electron microscopy,86 which potentially allow the extraction of kinetic information, such techniques are highly complex and generally cannot be used routinely.
3.1.2 Light scattering techniques.
The most common techniques for nanoparticle sizing are dynamic light scattering (DLS) and nanoparticle tracking analysis (NTA). These techniques involve monochromatic laser source and track variations in light scattering intensity. In the case of DLS ensemble average measurements are taken and the particle size deduced via the correlation function, while NTA allows tracking of scattering of individual nanoparticles. DLS has been extensively used due to its ease of analysis (the process is fully automated), high accuracy for monodisperse samples and the ease and rapidity of sample preparation.87 The main problems arise in the presence of polydisperse particle distribution (large and smaller particles present concurrently) which can cause the resultant distribution to be skewed to larger particle sizes due to their higher scattering intensity leading to erroneous conclusions on the sample. NTA is capable of tracking individual particles and as a result is less susceptible to polydisperse distributions.88 The main challenge for accurate tracking are small weakly scattering nanoparticles (diameter <20 nm, non-metallic). Another challenge for most light scattering techniques is the determination of the geometry of the particles as usually the algorithm operates under the assumption of a spherical geometry although approaches exist to incorporate other geometries.89
3.1.3 Zeta potential measurements.
An important aspect in the design of nano-impact experiments is the surface charge of the particles as it determines their colloidal stability and sometimes electrode–particle interactions.90,91 Unfortunately it is not possible to measure this quantity directly. A charged particle in an ionic environment causes a perturbation in the surrounding ion concentrations.92,93 As a result a double layer surrounding a particle is formed. Within close proximity to the particle surface, the ions are strongly bound and are unable to move meanwhile in an outer diffuse layer ions retain their movement and a disperse dynamic ionic layer is formed.94 When a particle moves due to an electric field the inner Stern layer remains bound, the potential at the boundary of bound and moving ions is known as the zeta potential and can be measured experimentally. Fig. 9 shows a schematic structure of the ionic layers. Most of the experimental measurements are performed through the use of light scattering and electrophoresis. The surface charge of the particle plays an important role in impact experiments which involve migration as the mass transport of the strongly charged particles will be significantly affected.
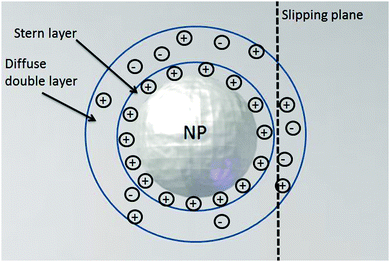 |
| Fig. 9 Schematic diagram of a double layer structure formed at a negatively charged particle in ionic media. | |
3.1.4 Potentiostat requirements.
The currents arising from electrochemical reactions at or of individual nanoparticles are inherently small (often of the order of picoamperes) and commonly comparable to the magnitude of a system's noise. The noise response of an electrode is heavily dependent upon the potentiostatic control and measurement circuitry.95 Consequently, when designing a system for investigating nanoscale electrochemical events the construction of the whole set-up must be considered. Usefully, many of the design requirements of a successful nano-impact system are shared with those of amperometric patch-clamp systems.96 In both cases it is beneficial to place the current amplifier as close to the working electrode as feasible. It is worth noting that with some commercial potentiostats the cables connecting the electrochemical cell to the device are often insufficiently shielded. This problem may, in part, be remedied by placing the entirety of the cables connecting the potentiostat to an electrochemical cell into a Faraday cage.
For an in-house built system the control of the working electrode potential may be adequately achieved using a highly-stabilised adder potentiostat with a good ground.4,97 The primary aim being to ensure the electrode potential is as constant as possible, even relatively small variations in the applied potential can result in a measurable increase of a system's noise. The current-to-voltage converter used to measure the current at the working electrode is another important voltage noise source. Importantly, the converter inherently acts as a low-pass filter (most often designed to be first order) and the output signal of the amplifier may be further filtered prior to digitization of the signal.
3.1.5 Choice of electrode geometry microdisc vs. microwire electrode.
In cases where a system's noise is dominated by the working electrode then, for smaller electrodes, the magnitude of the current fluctuations varies approximately linearly with the square-root of the electrode capacitance.95 In reality for many systems the noise floor of the current amplifier often determines the lower limit of recorded electrochemical response. However in general, smaller electrodes i.e. ones with a smaller capacitances, have improved signal-to-noise ratios allowing better resolution of individual nanoparticle impact events. As a caveat – due to stray capacitances98 and poor electrode sealing99 – the capacitance (and hence electrochemical noise) of a microelectrode may be significantly greater than that predicted solely on the basis of its geometric area. Beyond consideration of the resolution of the current recorded during an individual impact event the impact frequency is another important factor. As the size of an electrode decreases the probability of observing an impact concomitantly lowers. The impact frequency is proportional to the nanoparticle diffusion coefficient and concentration (as discussed fully in Section 2.2).66 This limitation on the frequency can be problematic for the study of large and/or low concentrations of nanoparticles.
From the above discussion it should be clear that working electrode selection, design and fabrication is an important consideration in nano-impact experiments. As an additional constraint, for ‘mediated’ impacts it is imperative that the electrode material be distinctly less active towards the electrochemical reaction of study than the nanomaterial. As a note of caution, although mercury may appear as a prime electrode material for studying catalytic nanoparticle reactions its susceptibility to forming mercurous chloride in chloride containing media can lead to erroneous results.100
To date the vast majority of nano-impact studies have been performed using glass sealed microelectrodes. These electrodes are often sufficient for studying nano-impact events, however, in cases where the nanoparticles irreversibly adsorb to the glass surface then the frequency of observed impacts may be significantly less than predicted.75 In order to overcome these issues of low impact frequency one may either use an array of electrodes101–103 or increase the dimensions of the electrode itself. In the latter case the use of carbon fibre microelectrodes104 have been found to be particularly beneficial in maintaining relatively low electrochemical noise and minimising problems of nanoparticle adsorption to the supporting substrate.
3.1.6 Electrolyte effect.
The main advantages of using high concentrations of supporting electrolyte are the reduced Ohmic drop and the resultant compression of the double layer at the electrode. However the high ionic environment may influence the behaviour of the suspended particles. Many particles have a charged coating and in accordance with Derjaguin, Landau, Varwey, Overbeek theory (DLVO) the presence of electrolyte may cause aggregation or agglomeration due to contraction of the individual double layer surrounding each particle and the associated decreased repulsion.105–108 As a result for the optimal measurement of single entities the experimental conditions require minimal clustering of particles.109,110 For plasmonic particles the effect of the supporting electrolyte on agglomeration or aggregation processes can be conveniently measured using UV-vis spectroscopy by taking UV-vis spectra prior and post introduction of the supporting electrolyte. Aggregation/agglomeration causes broadening of the characteristic plasmonic peak due to increasing size of the particles.111 An alternative method of minimizing agglomeration or aggregation in a strongly ionic environment is to use particles with steric stabilization through use of a polymer coating.112,113
3.1.7 Effect of solution phase impurities.
Many nanoparticle synthesis methods involve the use of organic solvents114 and these solvents may remain as impurities in the final nanoparticle solution.115 Presence of these and other impurities may significantly affect nano-impact experiments due to potential electrode blockage. As a result a solution containing particles of interest may fail to produce the expected current transients. This effect has been evaluated theoretically and demonstrated experimentally using organic indigo nanoparticles in the presence of trace concentrations of acetone.116 As the concentration of acetone reached 250 nM no further collision events were observed consistent with a blocked electrode. By contrast cyclic voltammograms of the molecular probe hexacyanoferrate (K3Fe(CN)6) were not affected by the presence of acetone, thereby highlighting the inherent sensitivity of nano-impact experiments. Blocking is much more significant for nano-impact experiments due to the blocking molecule–nanoparticle size disparity; only a small coverage of blocking species is required in order to prevent the particle from approaching the electrode. By contrast a molecular probe is smaller and a much greater blockage area is required in order to observe any deviations.
3.1.8 Migration effects.
The electrochemical response of solution phase redox active species under conditions of low supporting electrolyte are relatively well understood.117–120 In general migratory effects arise from a buildup of charge in the diffusion layer as caused by the electrochemical reaction. In the majority of cases consideration of the electric field associated with the double layer may be totally neglected. Theoretically these systems can be successfully modeled either through invoking electroneutrality121 or a zero-field approximation.122 In a nanoparticle blocking experiment, a molecular redox probe is reduced or oxidised at an electrode surface under conditions of low supporting electrolyte.123 It is this build-up of charge in the diffusion layer that is used to ‘attract’ nanoparticles to the electrode surface thereby increasing the impact frequency. Upon impact of the nanoparticle the electrode becomes blocked and the magnitude of the current associated with the molecular species decreases. Although the use of migration to enhance the frequency of impacts is analytically beneficial, gaining quantitative information regarding the nanoparticle concentration is challenging.124 A distinct advantage of this technique however is that the nanoparticle of study does not need to be electroactive or conducting.
Beyond the use of electrochemically induced electrophoresis of nanoparticles, the nano-impact method provides a new route by which electrostatic interactions with the double layer may be probed. As discussed above for a redox active molecular species, migratory effects primarily arise due to a build-up of charge in the diffusion layer and are not predominantly related to the double layer. For a nano-impact experiment where the events are stochastic and the electrochemical products of the nanoparticle process are diffusionally isolated, the frequency of nanoparticle impacts with an electrode can be used to study the possible influence of the double layer on the nanoparticle. Through the investigation of the reduction of organic indigo nanoparticles under conditions of decreasing ionic strength it was demonstrated in this specific case that the decrease in the electrochemical driving force associated with the greater extent of the double layer is a more dominant effect than the migratory exclusion of the nanoparticles from the double layer.125 Due to the instability of nanoparticles towards agglomeration and aggregation under conditions of high supporting electrolyte or in the presence of multi-valent ions, the influence of low electrolyte concentrations upon the observed electrochemical response of the system is an important consideration.
3.1.9 Forced convection.
For samples with extremely low concentrations of species of interest the time scales needed to observe impacts may be impractically long even when the electrodes with increased surface area are used. Another approach of increasing frequency of impacts apart from migration is the use of forced convection in order to compress the diffusion layer at the electrode. Opallo et al.126 used a glassy carbon rotating disk electrode (RDE) to increase the adsorption of injected catalytically active gold nanoparticles and were able to demonstrate characteristic steps for electrocatalytic oxidation of glucose. Murray et al. extended the impact approach to the ensemble response on an RDE using 4 nm Fe3O4 nanoparticles. The bulk arrival of nanoparticles allowed a cyclic voltammogram composed of multiple collision events to be recorded.127 Yoo et al. have employed magnetically guided microparticles for detection of silver nanoparticles in a microfluidics flow cell.128 Alligrant and co-workers have employed a microband microfluidic electrode to increase the collision rate of nanoparticles for mediated Faradaic impacts and thereby enhanced limit of detection.129 A femtomolar limit of detection has been achieved for direct Faradaic impacts through use of in-house designed flow cell.130 In general use of forced convection provides an opportunity for sensor development due to online detection capabilities and improved limits and timescales of detection.
3.2 What information can be extracted from impacts?
A diverse range of information can be extracted through the study of nano-impacts. This section briefly summarizes the range of properties which can be investigated and is followed by a description of some of the particular systems which have been studied to date.
3.2.1 Sizing.
Sizing of nanomaterials is crucial for efficient applications and rational system design.131,132 Initial studies of direct Faradaic impacts have demonstrated the ability to size a large variety of nanoparticle materials.11 Silver nanoparticles of a radius of 3.1 nm have been sized97 corresponding to a transfer of 7000–8000 electrons highlighting the sensitivity of the technique. In contrast to that particles as large as 100 nm have been sized.11 The results indicate that a wide range of sizes are covered by the nano-impact technique. In addition unlike common light scattering techniques such as dynamic light scattering (DLS) samples with diverse ranges of sizes (large and small particles) can be sized as the technique is unaffected by increased light scattering caused by the presence of the large particles.
3.2.2 Nanoparticle shape.
In addition to the ability to size spherical nanoparticles the nano-impact technique has been extended to non-spherical particles. For the case of non-spherical particles with well-defined geometries (for example nanorods17 or icosahedra102) knowledge of the surface area of a particle is required in order to obtain important geometric information such as the aspect ratio. In practice this can be achieved through monolayer functionalisation of a particle with a suitable redox active molecule such as 4-nitrothiophenol,17 which allows the measurement of the surface area of individual particles. Reduction of the 4-nitrothiophenol monolayer when a particle impacts the electrode allows to accurately determine the surface area of the particle and, in combination with the volumetric information obtained via direct Faradaic oxidation of the particle, the geometry can be determined.
3.2.3 Agglomeration and aggregation.
For many applications understanding of agglomeration or aggregation phenomena is critical as it can have a significant effect on the mass transport and physical properties of a particulate system.133–135 We note we use the IUPAC definition136 of an aggregate and an agglomerate. According to this definition aggregation is defined as comprising strongly bonded individual particles, and the clustering process is irreversible. Agglomeration corresponds to the process when the dispersed particles are held together by weaker physical or chemical interactions and the whole process is reversible. One of the key advantages of the nano-impact technique is that it is inherently an in situ technique and as a result agglomeration or aggregation processes can be probed. The capability of sizing nanoparticle aggregates has been demonstrated by Ellison et al.137 who showed good agreement between the size of distribution of aggregates and light scattering techniques. Further nano-impact experiments have allowed reversible agglomeration to be distinguished from irreversible aggregation for large (d = 100 nm) citrate-capped silver nanoparticles and revealed presence of monomers even in the media of high ionic strength.109 Similar behavior was observed for the case of the reduction of bismuth oxide and was used as an efficient way for the formation of nano-arrays.138 Stevenson et al. highlighted the importance of aggregation for mediated Faradaic impacts and outlined conditions for successful experimental design.110
3.3 Examples of systems investigated via nano-impacts method
3.3.1 Metallic nanoparticles.
Metallic nanoparticles are amongst the most-commonly synthesized particles and their physical properties are highly size-dependent.139,140 Nano-impacts have been shown to be able to quantify the size of a wide range of metallic nanoparticles including: silver,37 gold,38,39 nickel40,41 and copper.42 The particles used in such experiments were independently sized using electron microscopy and light scattering techniques and excellent agreement was observed between the different techniques, highlighting the quantitative sizing abilities of the nano-impact technique.
3.3.2 Core–shell and alloy systems.
Core–shell nanoparticles have significant potential in catalysis and industrial applications due to their multifunctional capabilities provided by different materials comprising the core and the shell.141 However sizing such materials possesses challenges often requiring time-consuming electron microscopy coupled with energy dispersive X-ray spectroscopy (EDX). Light scattering techniques can provide the overall size of the particles but are insensitive to their composition. Nano-impacts has been successfully applied to core shell systems of AuAg nanoparticles.16 By stepping the electrode potential to different values where first the shell and then both core and shell are oxidised the shell thickness has been determined and the corresponding size of the core.
Alloy nanoparticles have tuneable properties such as the plasmonic response based on the composition and as a result are also subject of research interest. Cyclic voltammetry has been used to characterize ensemble properties of such systems.142 The nano-impacts technique has been extended by Tschulik et al. to 14 nm Ag–Au nanoparticles and allowed to determine the composition of individual particles and revealed an alloy stabilization effect on the oxidation potential of silver of a single nanoparticle.143
3.3.3 Organic and biological particles.
Organic nanoparticles are prominent candidates for drug delivery in biomedical field144 and have found a wide use in photonic applications.145 Unlike metallic particles they are difficult to size via traditional means such as electron microscopy due to aggregation/agglomeration upon drying. Light scattering techniques can provide bulk size information but can be adversely affected by the presence of large particles.88 As a result the nano-impacts technique holds the important advantage of being able to size individual droplets or particles and provide information of the size distribution of the sample.14,18,146–151 Initial proof of concept study involved the reduction of indigo to leuco-indigo nanoparticles, excellent agreement was observed between the nano-impacts and DLS.13 Another striking example of the versatility of the nano-impact technique of the organic particles is the study of oxygen reduction reaction mediated by a single nano-droplet containing vitamin B12. The mechanistic understanding of the process was established by varying the potential and investigating the observed average charge and establishing the number of electrons transferred.147 Organic nanoparticles encompass a wide range of structures and nano-impacts have been successfully applied to micelles152 and liposomes151 further extending the applicability of the technique.
In addition to artificial organic structures recent works have demonstrated the use of nano-impacts for the determination of the presence of biological entities including viruses,19,20 bacteria21,22 and red blood cells.23
3.3.4 Metal oxides/metal halides.
Nano-impacts are capable of sizing various metal oxides (iron oxide,153–155 bismuth oxide138) and reveal complex pH dependent phenomena. In addition nano-impacts revealed the importance of reversible agglomeration which takes place at the electrode surface. For bismuth oxide system as large clusters approach the electrode cluster dissociate into individual monomers since the latter diffuse more quickly and rapidly reach the electrode where they react. This may lead to an apparent non-observation of large particle sizes as only predominantly monomers are observed.156
3.3.5 Tracking Ostwald ripening.
Nano-impacts in conjunction with transmission electron microscopy have also been successfully applied to tracking of photochemical mechanism of nanoparticle growth and revealed key growth stages of triangular silver prisms.157 The key advantages of the technique being the ability to analyze large number of particles and reveal information regarding the overall population of the particles with minimal sample preparation.
3.3.6 Detection of enzymes via nano-impacts.
Understanding enzymatic activity lies at the heart of biology. While there are reliable tools readily-available for measuring the activity of an ensemble of enzymes, the characterisation individual enzymes still remains challenging – and of particular interest in fundamental research. In order to address this issue, various methods are under development, of which fluorescence-based measurements currently are among the most prominent approaches. In this context and to complement other methods, nano-impact experiments of individual enzymes appear promising at least at first sight, as they may open up new routes towards single-enzyme measurements. Compared to many other approaches, the nano-impact approach offers several advantages: the enzyme is characterised in its ‘natural’ environment without being previously immobilised or molecularly tagged, the method is cheaper than a fluorescence set-up, and the experimental set-up is significantly more compact.
The idea of single-enzyme impact was recently pursued by two publications: in early 2016 Sekretaryova et al. claimed the detection of individual laccase enzymes via the catalytic reduction of oxygen by the impacting enzyme at a microdisc electrode.158 Soon after, Han et al. observed current spikes at a nanoelectrode that were interpreted as impacts of individual enzymes revealed through the reaction of horse radish peroxidase catalysed hydrogen peroxide.159
The exact interpretation of these results remains open to debate and in particular the possibility of direct electron transfer to single enzymes.160 Without doubt there will be more works on this topic soon!
4 Conclusions and outlook
The field of particle–electrode collisions is an ever-growing research discipline opening up a wide span of potential applications. Among these, a novel direction of nano-impact research may be single enzyme activity, which potentially provides insight into the enzyme reaction mechanism and kinetics at an unprecedented level of detail. Further applications may include the monitoring of the activity of nanomotors, as previous research has already demonstrated the applicability of the technique to micromotors.161 In addition it is likely that the direction of the future research will involve concurrent electrochemistry and microscopy in order to further probe the physical processes taking place building on initial studies.162–165
Aside from that, we note the recent assessment of Gooding,15 who speculates that particularly the upcoming analysis of complex biological objects via the nano-impact technique will have significant impact on the future developments in this field. According to Gooding ‘this [method] has potential to allow us to better understand heterogeneity and to develop new diagnostic devices based on single cell electrochemistry’.15 Recent developments23 in the detection of individual biological cells may inspire other researchers to investigate single cell behaviour and to develop novel diagnostic tools.
Acknowledgements
The research leading to these results has received partial funding from the European Research Council under the European Union Seventh Framework Programme (FP/2007-2013)/ERC Grant Agreement no. [320403]. We thank Dr Lior Sepunaru and Dr Giorgia Zampardi for helpful discussions.
References
- W. Cheng and R. G. Compton, TrAC, Trends Anal. Chem., 2014, 58, 79–89 CrossRef CAS.
- M. V. Mirkin, T. Sun, Y. Yu and M. Zhou, Acc. Chem. Res., 2016 DOI:10.1021/acs.accounts.6b00294.
- Y. Wang, X. Shan and N. Tao, Faraday Discuss., 2016 10.1039/C6FD00180G.
- C. Batchelor-McAuley, E. Kätelhön, E. O. Barnes, R. G. Compton, E. Laborda and A. Molina, ChemistryOpen, 2015, 4, 224–260 CrossRef CAS PubMed.
- P. H. Robbs and N. V. Rees, Phys. Chem. Chem. Phys., 2016, 35, 583–592 Search PubMed.
- M. Pumera, ACS Nano, 2014, 8, 7555–7558 CrossRef CAS PubMed.
- M. G. Banus, Science, 1941, 93, 601–602 CAS.
-
A. Bard and L. Faulkner, Electrochemical methods: fundamentals and applications, Wiley, New York, 2nd edn, 2001 Search PubMed.
-
R. G. Compton and C. E. Banks, Understanding Voltammetry, Imperial College Press, 2nd edn, 2010 Search PubMed.
- B. Haddou, N. V. Rees and R. G. Compton, Phys. Chem. Chem. Phys., 2012, 14, 13612 RSC.
- T. R. Bartlett, S. V. Sokolov and R. G. Compton, ChemistryOpen, 2015, 4, 600–605 CrossRef CAS PubMed.
- C. S. Lim, S. M. Tan, Z. Sofer and M. Pumera, ACS Nano, 2015, 9, 8474–8483 CrossRef CAS PubMed.
- W. Cheng, X.-F. Zhou and R. G. Compton, Angew. Chem., 2013, 52, 12980–12982 CrossRef CAS PubMed.
- X.-F. Zhou, W. Cheng and R. G. Compton, Nanoscale, 2014, 6, 6873–6878 RSC.
- J. J. Gooding, Angew. Chem., Int. Ed., 2016 DOI:10.1002/anie.201606459.
- L. R. Holt, B. J. Plowman, N. P. Young, K. Tschulik and R. G. Compton, Angew. Chem., Int. Ed., 2016, 55, 397–400 CrossRef CAS PubMed.
- B. J. Plowman, N. P. Young, C. Batchelor-McAuley and R. G. Compton, Angew. Chem., Int. Ed., 2016, 55, 7002–7005 CrossRef CAS PubMed.
- B.-K. Kim, J. Kim and A. J. Bard, J. Am. Chem. Soc., 2015, 137, 2343–2349 CrossRef CAS PubMed.
- J. E. Dick, A. T. Hilterbrand, A. Boika, J. W. Upton and A. J. Bard, Proc. Natl. Acad. Sci. U. S. A., 2015, 112, 5303–5308 CrossRef CAS PubMed.
- L. Sepunaru, B. J. Plowman, S. V. Sokolov, N. P. Young and R. G. Compton, Chem. Sci., 2016, 7, 3892–3899 RSC.
- L. Sepunaru, K. Tschulik, C. Batchelor-McAuley, R. Gavish and R. G. Compton, Biomater. Sci., 2015, 3, 816–820 RSC.
- J. Y. Lee, B.-K. Kim, M. Kang and J. H. Park, Sci. Rep., 2016, 6, 30022 CrossRef PubMed.
- L. Sepunaru, S. V. Sokolov, J. Holter, N. P. Young and R. G. Compton, Angew. Chem., Int. Ed., 2016, 55, 9768–9771 CrossRef CAS PubMed.
- X. Li, C. Batchelor-McAuley, S. A. I. Whitby, K. Tschulik, L. Shao and R. G. Compton, Angew. Chem., Int. Ed., 2016, 55, 4296–4299 CrossRef CAS PubMed.
- K. Micka, Collect. Czech. Chem. Commun., 1956, 21, 647–651 CrossRef CAS.
- I. Jones and R. Kaye, J. Electroanal. Chem. Interfacial Electrochem., 1969, 20, 213–221 CrossRef CAS.
- G. Moller, Chimia, 1971, 25, 29 Search PubMed.
- A. Holland and J. Sawers, Photogr. Sci. Eng., 1973, 17, 295–298 CAS.
- A. Holland and A. Feinerman, J. Appl. Photogr. Eng., 1982, 8, 165–167 Search PubMed.
- M. Heyrovsky and J. Jirkovsky, Langmuir, 1995, 11, 4288–4292 CrossRef CAS.
- M. Heyrovsky, J. Jirkovsky and M. Struplova-Bartackova, Langmuir, 1995, 11, 4300–4308 CrossRef CAS.
- M. Heyrovsky, J. Jirkovsky and M. Struplova-Bartackova, Langmuir, 1995, 11, 4309–4312 CrossRef CAS.
- M. Heyrovsky, J. Jirkovsky and B. R. Mueller, Langmuir, 1995, 11, 4293–4299 CrossRef CAS.
- H. Wu, Q. Lin, C. Batchelor-McAuley and R. G. Compton, ChemElectroChem, 2016, 3, 1478–1483 CrossRef CAS.
- X. Li, C. Lin, C. Batchelor-McAuley, E. Laborda, L. Shao and R. G. Compton, J. Phys. Lett., 2016, 7, 1554–1558 CAS.
- Y.-G. Zhou, N. V. Rees and R. G. Compton, Angew. Chem., Int. Ed., 2011, 50, 4219–4221 CrossRef CAS PubMed.
- N. V. Rees, Y.-G. Zhou and R. G. Compton, ChemPhysChem, 2011, 12, 1645–1647 CrossRef CAS PubMed.
- Y.-G. Zhou, N. V. Rees, J. Pillay, R. Tshikhudo, S. Vilakazi and R. G. Compton, Chem. Commun., 2012, 48, 224–226 RSC.
- D. Qiu, S. Wang, Y. Zheng and Z. Deng, Nanotechnology, 2013, 24, 505707 CrossRef PubMed.
- Y.-G. Zhou, B. Haddou, N. V. Rees and R. G. Compton, Phys. Chem. Chem. Phys., 2012, 14, 14354 RSC.
- E. J. E. Stuart, Y.-G. Zhou, N. V. Rees and R. G. Compton, RSC Adv., 2012, 2, 6879 RSC.
- B. Haddou, N. V. Rees and R. G. Compton, Phys. Chem. Chem. Phys., 2012, 14, 13612 RSC.
- C. E. Banks, N. V. Rees and R. G. Compton, J. Phys. Chem. B, 2002, 106, 5810–5813 CrossRef CAS.
- N. V. Rees, C. E. Banks and R. G. Compton, J. Phys. Chem. B, 2004, 108, 18391–18394 CrossRef CAS.
- J. Poon, C. Batchelor-McAuley, K. Tschulik and R. G. Compton, Chem. Sci., 2015, 6, 2869–2876 RSC.
- X. Xiao and A. J. Bard, J. Am. Chem. Soc., 2007, 129, 9610–9612 CrossRef CAS PubMed.
- X. Xiao, F.-R. F. Fan, J. Zhou and A. J. Bard, J. Am. Chem. Soc., 2008, 130, 16669–16677 CrossRef CAS PubMed.
- H. S. Ahn and A. J. Bard, Angew. Chem., Int. Ed., 2015, 54, 13753–13757 CrossRef CAS PubMed.
- S. J. Kwon, F.-R. F. Fan and A. J. Bard, J. Am. Chem. Soc., 2010, 132, 13165–13167 CrossRef CAS PubMed.
- S. J. Kwon and A. J. Bard, J. Am. Chem. Soc., 2012, 134, 10777–10779 CrossRef CAS PubMed.
- A. Fernando, S. Parajuli and M. A. Alpuche-Aviles, J. Am. Chem. Soc., 2013, 135, 10894–10897 CrossRef CAS PubMed.
- M. Kang, D. Perry, Y.-R. Kim, A. W. Colburn, R. A. Lazenby and P. R. Unwin, J. Am. Chem. Soc., 2015, 137, 10902–10905 CrossRef CAS PubMed.
- B. M. Quinn, P. G. van't Hof and S. G. Lemay, J. Am. Chem. Soc., 2004, 126, 8360–8361 CrossRef CAS PubMed.
- E. Lebègue, C. M. Anderson, J. E. Dick, L. J. Webb and A. J. Bard, Langmuir, 2015, 31, 11734–11739 CrossRef PubMed.
- J. E. Dick, C. Renault and A. J. Bard, J. Am. Chem. Soc., 2015, 137, 8376–8379 CrossRef CAS PubMed.
- A. Einstein, Ann. Phys., 1905, 322, 549–560 CrossRef.
- W. Sutherland, Philos. Mag., 1905, 9, 781–785 CrossRef CAS.
- H. Brenner, Chem. Eng. Sci., 1961, 16, 242–251 CrossRef CAS.
- M. A. Bevan and D. C. Prieve, J. Chem. Phys., 2000, 113, 1228–1236 CrossRef CAS.
- H. Faxen, Ark. Mat., Astron. Fys., 1923, 17, 27 Search PubMed.
- A. Goldman, R. Cox and H. Brenner, Chem. Eng. Sci., 1967, 22, 637–651 CrossRef CAS.
- P. S. Singh, E. Kätelhön, K. Mathwig, B. Wolfrum and S. G. Lemay, ACS Nano, 2012, 6, 9662–9671 CrossRef CAS PubMed.
- E. Kätelhön, K. J. Krause, P. S. Singh, S. G. Lemay and B. Wolfrum, J. Am. Chem. Soc., 2013, 135, 8874–8881 CrossRef PubMed.
- E. Kätelhön, K. J. Krause, K. Mathwig, S. G. Lemay and B. Wolfrum, ACS Nano, 2014, 8, 4924–4930 CrossRef PubMed.
- E. Kätelhön and R. G. Compton, Chem. Sci., 2014, 5, 4592–4598 RSC.
- S. Eloul, E. Kätelhön, C. Batchelor-McAuley, K. Tschulik and R. G. Compton, J. Electroanal. Chem., 2015, 755, 136–142 CrossRef CAS.
- Y. Saito, Rev. Polarogr., 1968, 15, 177–187 CrossRef CAS.
- D. Shoup and A. Szabo, J. Electroanal. Chem. Interfacial Electrochem., 1982, 140, 237–245 CrossRef CAS.
- J. Heinze, J. Electroanal. Chem. Interfacial Electrochem., 1981, 124, 73–86 CrossRef CAS.
- A. Szabo, D. K. Cope, D. E. Tallman, P. M. Kovach and R. Wightman, J. Electroanal. Chem. Interfacial Electrochem., 1987, 217, 417–423 CrossRef CAS.
- S. Eloul, E. Kätelhön, C. Batchelor-McAuley, K. Tschulik and R. G. Compton, J. Phys. Chem. C, 2015, 119, 14400–14410 CAS.
- S. Eloul and R. G. Compton, J. Phys. Lett., 2016, 4317–4321 CAS.
- S. Eloul, E. Kätelhön and R. G. Compton, Phys. Chem. Chem. Phys., 2016, 50, 4219–4221 Search PubMed.
- S. V. Sokolov, E. Kätelhön and R. G. Compton, J. Phys. Chem. C, 2016, 120, 10629–10640 CAS.
- S. Eloul and R. G. Compton, ChemElectroChem, 2014, 1, 917–924 CrossRef CAS.
- E. Kätelhön, E. E. Tanner, C. Batchelor-McAuley and R. G. Compton, Electrochim. Acta, 2016, 199, 297–304 CrossRef.
- P. Bobbert, M. Wind and J. Vlieger, Phys. A, 1987, 141, 58–72 CrossRef.
- I. Streeter and R. G. Compton, J. Phys. Chem. C, 2007, 111, 18049–18054 CAS.
- J. M. Kahk, N. V. Rees, J. Pillay, R. Tshikhudo, S. Vilakazi and R. G. Compton, Nano Today, 2012, 7, 174–179 CrossRef CAS.
- E. Kätelhön, A. Feng, W. Cheng, S. Eloul, C. Batchelor-McAuley and R. G. Compton, J. Phys. Chem. C, 2016, 120, 17029–17034 Search PubMed.
- Q. Lin and R. G. Compton, J. Phys. Chem. C, 2015, 119, 23463–23469 CAS.
- S. Eloul and R. G. Compton, J. Phys. Chem. C, 2015, 119, 27540–27549 CAS.
-
I. N. Bronstein, K. A. Semendjajew, G. Musiol and H. Mühlig, Taschenbuch der Mathematik, 2008, vol. 23, p. 1216 Search PubMed.
-
D. B. Williams and C. B. Carter, Transmission Electron Microscopy, Springer US, Boston, MA, 2009 Search PubMed.
- P. L. Stewart, Wiley Interdiscip. Rev.: Nanomed. Nanobiotechnol., 2016 DOI:10.1002/wnan.1417.
- E. A. Ring and N. de Jonge, Micron, 2012, 43, 1078–1084 CrossRef CAS PubMed.
-
K. Schmitz, An Introduction to Dynamic Light Scattering by Macromolecules, Elsevier, 2006, vol. 24 Search PubMed.
- V. Filipe, A. Hawe and W. Jiskoot, Pharm. Res., 2010, 27, 796–810 CrossRef CAS PubMed.
- R. Pecora, J. Nanopart. Res., 2000, 2, 123–131 CrossRef CAS.
- M. Kaszuba, J. Corbett, F. M. Watson and A. Jones, Philos. Trans. R. Soc., A, 2010, 368, 4439–4451 CrossRef CAS PubMed.
- G. V. Lowry, R. J. Hill, S. Harper, A. F. Rawle, C. O. Hendren, F. Klaessig, U. Nobbmann, P. Sayre and J. Rumble, Environ. Sci.: Nano, 2016, 3, 953–965 RSC.
- W. R. Fawcett, J. Solid State Electrochem., 2011, 15, 1347–1358 CrossRef CAS.
- H. Gerischer, Berichte der Bunsengesellschaft für physikalische Chemie, 1966, 70, 765–766 Search PubMed.
- D. C. Grahame, Chem. Rev., 1947, 41, 441–501 CrossRef CAS PubMed.
- J. Yao and K. D. Gillis, Analyst, 2012, 137, 2674 RSC.
-
B. Sakmann and E. Neher, Single-Channel Recording, Springer US, Boston, MA, 1995 Search PubMed.
- C. Batchelor-McAuley, E. Kätelhön, E. O. Barnes, R. G. Compton, E. Laborda and A. Molina, ChemistryOpen, 2015, 4, 224–260 CrossRef CAS PubMed.
- D. O. Wipf, A. C. Michael and R. Wightman, J. Electroanal. Chem. Interfacial Electrochem., 1989, 269, 15–25 CrossRef CAS.
- K. R. Wehmeyer and R. Wightman, J. Electroanal. Chem. Interfacial Electrochem., 1985, 196, 417–421 CrossRef CAS.
- T. R. Bartlett, C. Batchelor-McAuley, K. Tschulik, K. Jurkschat and R. G. Compton, ChemElectroChem, 2015, 2, 522–528 CrossRef CAS.
- K. J. Krause, A. Yakushenko and B. Wolfrum, Anal. Chem., 2015, 87, 7321–7325 CrossRef CAS PubMed.
- S. V. Sokolov, C. Batchelor-McAuley, K. Tschulik, S. Fletcher and R. G. Compton, Chem. – Eur. J., 2015, 21, 10741–10746 CrossRef CAS PubMed.
- S. Fletcher and M. D. Horne, Electrochem. Commun., 1999, 1, 502–512 CrossRef CAS.
- J. Ellison, C. Batchelor-McAuley, K. Tschulik and R. G. Compton, Sens. Actuators, B, 2014, 200, 47–52 CrossRef CAS.
- J. Lyklema, H. P. Van Leeuwen and M. Minor, Adv. Colloid Interface Sci., 1999, 83, 33–69 CrossRef CAS.
- E. M. V. Hoek and G. K. Agarwal, J. Colloid Interface Sci., 2006, 298, 50–58 CrossRef CAS PubMed.
- S. Torkzaban, S. A. Bradford and S. L. Walker, Langmuir, 2007, 23, 9652–9660 CrossRef CAS PubMed.
-
G. Trefalt and M. Borkovec, Overview of DLVO theory, 2014, http://www.colloid.ch/dlvo (accessed November 2016).
- S. V. Sokolov, K. Tschulik, C. Batchelor-McAuley, K. Jurkschat and R. G. Compton, Anal. Chem., 2015, 87, 10033–10039 CrossRef CAS PubMed.
- D. A. Robinson, A. M. Kondajji, A. D. Castañeda, R. Dasari, R. M. Crooks and K. J. Stevenson, J. Phys. Lett., 2016, 7, 2512–2517 CAS.
- N. T. K. Thanh and Z. Rosenzweig, Anal. Chem., 2002, 74, 1624–1628 CrossRef CAS PubMed.
- C. Lourenco, M. Teixeira, S. Simões and R. Gaspar, Int. J. Pharm., 1996, 138, 1–12 CrossRef CAS.
- M. K. Corbierre, N. S. Cameron, M. Sutton, S. G. J. Mochrie, L. B. Lurio, A. Rühm and R. B. Lennox, J. Am. Chem. Soc., 2001, 123, 10411–10412 CrossRef CAS PubMed.
- L. Wu, A. Mendoza-Garcia, Q. Li and S. Sun, Chem. Rev., 2016, 116, 10473–10512 CrossRef CAS PubMed.
- M. Niederberger, G. Garnweitner, J. Buha, J. Polleux, J. Ba and N. Pinna, J. Sol–Gel Sci. Technol., 2006, 40, 259–266 CrossRef CAS.
- E. Kätelhön, W. Cheng, C. Batchelor-McAuley, K. Tschulik and R. G. Compton, ChemElectroChem, 2014, 1, 1057–1062 CrossRef.
- S. Feldberg, Electrochem. Commun., 2000, 2, 453–456 CrossRef CAS.
- K. B. Oldham and A. M. Bond, J. Electroanal. Chem., 2001, 508, 28–40 CrossRef CAS.
- W. Hyk and Z. Stojek, Anal. Chem., 2005, 77, 6481–6486 CrossRef CAS PubMed.
- S. R. Belding, J. G. Limon-Petersen, E. J. F. Dickinson and R. G. Compton, Angew. Chem., Int. Ed., 2010, 49, 9242–9245 CrossRef CAS PubMed.
- E. J. F. Dickinson, J. G. Limon-Petersen and R. G. Compton, J. Solid State Electrochem., 2011, 15, 1335–1345 CrossRef CAS.
- I. Streeter and R. G. Compton, J. Phys. Chem. C, 2008, 112, 13716–13728 CAS.
- J. H. Park, A. Boika, H. S. Park, H. C. Lee and A. J. Bard, J. Phys. Chem. C, 2013, 117, 6651–6657 CAS.
- A. Boika, S. N. Thorgaard and A. J. Bard, J. Phys. Chem. B, 2013, 117, 4371–4380 CrossRef CAS PubMed.
- K. Tschulik, W. Cheng, C. Batchelor-McAuley, S. Murphy, D. Omanović and R. G. Compton, ChemElectroChem, 2015, 2, 112–118 CrossRef CAS.
- J. Dolinska, M. Jonsson-Niedziolka, V. Sashuk and M. Opallo, Electrochem. Commun., 2013, 37, 100–103 CrossRef CAS.
- J. J. P. Roberts, J. A. Westgard, L. M. Cooper and R. W. Murray, J. Am. Chem. Soc., 2014, 136, 10783–10789 CrossRef CAS PubMed.
- J. J. Yoo, J. Kim and R. M. Crooks, Chem. Sci., 2015, 6, 6665–6671 RSC.
- T. M. Alligrant, M. J. Anderson, R. Dasari, K. J. Stevenson and R. M. Crooks, Langmuir, 2014, 30, 13462–13469 CrossRef CAS PubMed.
- S. V. Sokolov, T. R. Bartlett, P. Fair, S. Fletcher and R. G. Compton, Anal. Chem., 2016, 88, 8908–8912 CrossRef CAS PubMed.
- W. J. Stark, Angew. Chem., Int. Ed., 2011, 50, 1242–1258 CrossRef CAS PubMed.
- W. J. Stark, P. R. Stoessel, W. Wohlleben and A. Hafner, Chem. Soc. Rev., 2015, 44, 5793–5805 RSC.
- R. Prasher, W. Evans, P. Meakin, J. Fish, P. Phelan and P. Keblinski, Appl. Phys. Lett., 2006, 89, 143119 CrossRef.
- Y. Wei, S. Han, J. Kim, S. Soh and B. A. Grzybowski, J. Am. Chem. Soc., 2010, 132, 11018–11020 CrossRef CAS PubMed.
- S. V. Sokolov, E. Kätelhön and R. G. Compton, J. Electroanal. Chem., 2015 DOI:10.1016/j.jelechem.2016.01.023.
-
A. McNaught and A. Wilkinson, IUPAC. Compendium of Chemical Terminology, Blackwell Scientific Publications, Oxford, 2nd edn, 1997 Search PubMed.
- J. Ellison, K. Tschulik, E. J. E. Stuart, K. Jurkschat, D. Omanović, M. Uhlemann, A. Crossley and R. G. Compton, ChemistryOpen, 2013, 2, 69–75 CrossRef CAS PubMed.
- T. R. Bartlett, S. V. Sokolov, J. Holter, N. Young and R. G. Compton, Chem. – Eur. J., 2016, 22, 7408–7414 CrossRef CAS PubMed.
- J.-T. Lue, J. Phys. Chem. Solids, 2001, 62, 1599–1612 CrossRef CAS.
- V. H. Grassian, J. Phys. Chem. C, 2008, 112, 18303–18313 CAS.
- M. B. Gawande, A. Goswami, T. Asefa, H. Guo, A. V. Biradar, D.-L. Peng, R. Zboril and R. S. Varma, Chem. Soc. Rev., 2015, 44, 7540–7590 RSC.
- B. J. Plowman, B. Sidhureddy, S. V. Sokolov, N. P. Young, A. Chen and R. G. Compton, ChemElectroChem, 2016, 3, 1039–1043 CrossRef CAS.
- E. N. Saw, V. Grasmik, C. Rurainsky, M. Epple and K. Tschulik, Faraday Discuss., 2016, 29, 27–35 Search PubMed.
- D. Horn and J. Rieger, Angew. Chem., Int. Ed., 2001, 40, 4330 CrossRef CAS.
- K. K. Ng and G. Zheng, Chem. Rev., 2015, 115, 11012–11042 CrossRef CAS PubMed.
- B.-K. Kim, A. Boika, J. Kim, J. E. Dick and A. J. Bard, J. Am. Chem. Soc., 2014, 136, 4849–4852 CrossRef CAS PubMed.
- W. Cheng and R. G. Compton, Angew. Chem., Int. Ed., 2015, 54, 7082–7085 CrossRef CAS PubMed.
- A. Feng, W. Cheng and R. G. Compton, Chem. Sci., 2016, 318, 62–64 Search PubMed.
- A. Feng, W. Cheng, J. Holter, N. Young and R. G. Compton, Chem. – Eur. J., 2016, 22, 6981–6986 CrossRef CAS PubMed.
- X.-F. Zhou, W. Cheng and R. G. Compton, Angew. Chem., 2014, 126, 12795–12797 CrossRef.
- W. Cheng and R. G. Compton, ChemElectroChem, 2016 DOI:10.1002/celc.201600396.
- H. S. Toh and R. G. Compton, Chem. Sci., 2015, 6, 5053–5058 RSC.
- K. Tschulik, B. Haddou, D. Omanović, N. V. Rees and R. G. Compton, Nano Res., 2013, 6, 836–841 CrossRef CAS.
- K. Shimizu, S. V. Sokolov and R. G. Compton, Colloid Interface Sci. Commun., 2016, 13, 19–22 CrossRef CAS.
- K. Shimizu, K. Tschulik and R. G. Compton, Chem. Sci., 2016, 7, 1408–1414 RSC.
- S. V. Sokolov, E. Kätelhön and R. G. Compton, J. Electroanal. Chem., 2016 DOI:10.1016/j.jelechem.2016.01.023.
- T. R. Bartlett, S. V. Sokolov, B. J. Plowman, N. P. Young and R. G. Compton, Nanoscale, 2016 10.1039/C6NR05740C.
- A. N. Sekretaryova, M. Y. Vagin, A. P. F. Turner and M. Eriksson, J. Am. Chem. Soc., 2016, 138, 2504–2507 CrossRef CAS PubMed.
- L. Han, W. Wang, J. Nsabimana, J.-W. Yan, B. Ren and D. Zhan, Faraday Discuss., 2016 10.1039/C6FD00061D.
- E. Kätelhön, L. Sepunaru, A. Karyakin and R. Compton, ACS Catal., 2016 DOI:10.1021/acscatal.6b02633.
- J. G. S. Moo and M. Pumera, ACS Sens., 2016, 1, 949–957 CrossRef CAS.
- C. Batchelor-McAuley, A. Martinez-Marrades, K. Tschulik, A. N. Patel, C. Combellas, F. Kanoufi, G. Tessier and R. G. Compton, Chem. Phys. Lett., 2014, 597, 20–25 CrossRef CAS.
- V. Brasiliense, P. Berto, C. Combellas, G. Tessier and F. Kanoufi, Acc. Chem. Res., 2016, 49, 2049–2057 CrossRef CAS PubMed.
- V. Brasiliense, A. N. Patel, A. Martinez-Marrades, J. Shi, Y. Chen, C. Combellas, G. Tessier and F. Kanoufi, J. Am. Chem. Soc., 2016, 138, 3478–3483 CrossRef CAS PubMed.
- C. Batchelor-McAuley, C. A. Little, S. V. Sokolov, E. Kätelhön, G. Zampardi and R. G. Compton, Anal. Chem., 2016 DOI:10.1021/acs.analchem.6b03524.
|
This journal is © the Owner Societies 2017 |
Click here to see how this site uses Cookies. View our privacy policy here.