DOI:
10.1039/C7AY01584D
(Paper)
Anal. Methods, 2017,
9, 4783-4789
Objective assessment of SERS thin films: comparison of silver on copper via galvanic displacement with commercially available fabricated substrates†
Received
28th June 2017
, Accepted 1st August 2017
First published on 1st August 2017
Abstract
Many studies report the development of new thin films for surface enhanced Raman scattering (SERS). However, the assessment of these surfaces in terms of their reproducibility for SERS is often subjective and whilst many spectra could and indeed should be reported, very few repeat measurements are typically used. Here, the performance of three SERS thin film substrates is assessed objectively using both univariate and novel multivariate methods. The silver on copper substrate (SoC) was synthesised in-house via galvanic displacement, whilst the other two substrates Klarite and QSERS are commercially available. The reproducibility of these substrates was assessed using rhodamine 6G (R6G) as a probe analyte and seven common vibrational bands that were observed in all R6G spectra were evaluated. In order to be as objective as possible a total of seven different data analysis methods were used to evaluate the surfaces revealing that overall the SoC substrate demonstrates much greater reproducibility when compared to the commercial substrates. Finally, through the collection of large datasets containing 6400 spectra per single substrate we also provide guidelines as to the typical number of spectra that should be collected in order to assess a substrate's performance objectively, and we conclude that this must be a minimum of 180 spectra collected randomly from across the region of interest.
Introduction
Solid-state substrates have been used to facilitate surface enhanced Raman scattering (SERS) since the field's initial conception in 1974.1,2 Since then a wide variety of substrates have been found to enable SERS.3 Although the noble metallic composition of substrates remains a constant, many different types of thin films for SERS have been fabricated including anisotropic metal nanoparticles,4,5 metal films over nanospheres (MFON),6,7 particles grafted onto glass,8,9 porous noble metal films,10–12 nanoparticle arrays,13–17 and metallic fractals,18–20 to name but a few, and other new fabrications are constantly being produced. The methods used to manufacture substrates can often be split into the sub categories, random and engineered,21,22 with nanolithographic techniques being championed as one of the most effective methods of exercising fine control over the substrate's morphology.23,24 The major limitations of using lithographic techniques is the expense of substrate manufacture and the need for specialist instrument operators often making more accessible facile methods such as galvanic displacement is preferred.36–38 Whilst SERS has become a huge area of interest25 and has been successfully applied as a sensitive technique in both chemical and biomedical analysis,26–28 its broader application depends on two factors, activity and reproducibility.29 It is accepted within the field that the perceived lack of reproducibility of SERS signal severely limits its applications.30,31 Nowadays it has become common place to claim very large enhancement effects and low detection limits, including single molecule detection, whilst reproducibility assessment in the majority of cases is avoided. In a 2011 review by Fan and colleagues on the fabrication of substrates for SERS32 the lack of standardization and precisely defined figures of merit within the field is highlighted as a major failing as to why the comparison between systems cannot be accurately implemented; this is also a view echoed here in relation to the publication of reproducibility values and this in the authors' opinion needs to be addressed and reproducibility objectified. To provide effective comparisons it is essential that a unified protocol for the reproducibility assessment of substrates is adopted, resulting in the performance values quoted in articles being fair, un-biased and readily understood, providing researchers with a vital resource for the comparison of novel SERS substrates. Currently there is huge number of methods being used to assess reproducibility. Despite the fact that one can take many repeat measurements from SERS substrates with little expense in terms of cost or labour, the most worrying and paradoxical aspect is the diminishing small number of spectra which some groups deem to be acceptable in order to assess a surfaces performance fully. Needless to say bigger data sets contain much better statistical integrity when assessing a substrate. Another problem with the current methods is the number of analytes interrogated, common chemicals include R6G, crystal violet and benzenethiol; although all of these are may be perfectly acceptable, one analyte alone should be used if comparison values are to be calculated. In this work R6G is used to assess SERS reproducibility across three different substrates – two commercially available (Klarite and QSERS) and one synthesised in-house via galvanic displacement (SoC).33 R6G provides an ideal analyte for this type of analysis because when irradiated with visible light in the absence of a SERS active substrate it exhibits a huge amount of fluorescence, making it a good analyte for certifying SERS activity. This compound has also been readily characterised using SERS by many researchers and so can be consider a ‘gold standard’ analyte. In the present study we report an objective comparison of the three substrates using many univariate and multivariate methods and highlight the need for multiple statistical analyses in order to develop an accurate view of a substrate's performance.
Experimental
Materials
In-house substrate – silver on copper (SoC) substrate materials.
Silver nitrate (99.9999%) was purchased from Sigma Aldrich (Dorset, U.K.) and the copper foil (1 mm thickness) was procured from a high street retailer (Fred Aldous Ltd., Manchester, U.K.). All solvents and chemicals were also obtained from Sigma Aldrich and used as supplied and were of analytical grade.
Commercial substrates.
Two commercial substrates were used in comparison to the SoC substrate. Klarite slides were supplied by Renishaw Diagnostics Limited (Glasgow, U.K.) and QSERS slides were provided by Nanova Inc. (Columbia, United States). Both substrates have been readily characterised using SEM, and are composed of a gold SERS active surface. Klarite consists of an array of carefully optimised inverted pyramids coated in a thin film of gold; the supplied active area is 4 mm × 4 mm. The recommended excitation wavelengths to drive plasmon excitation are either 633 nm or 785 nm and the analytes can be applied to the surface by drop casting, vapour deposition or immersion. The QSERS surface features a mixture of 15 nm and 60 nm gold nanoparticles distributed randomly across a silicon wafer. The dimensions of supplied active area are 5 mm × 5 mm. Although no information is given as to the best excitation wavelength it is assumed that either 633 nm or 785 nm would be ideal.
Methods
Synthesis of SoC substrate.
Copper foil was cut into 2.5 cm × 7.5 cm strips and fixed to a standard microscope slide to generate a more rigid surface. The Cu surface was then cleaned with copious amounts of methanol followed by acetone. 10 μL of 0.1 M AgNO3 aqueous solution was then spotted onto the surface and left to develop for 20 s as described previously.33 Deposition of the nanoparticles was signified by the formation of a grey target on the copper foil. Post deposition, further surface cleaning was carried out using water to remove any residual silver nitrate reagent and copper nitrate product. The substrate was then dried using a warm (35–40 °C) air supply. The deposition was carried out in the same manner at five different positions on the copper foil surface.
Surface characterisation.
The microstructures of all the solid-state substrates were examined using scanning electron microscopy (SEM). The analysis of the Klarite and QSERS substrates was carried out using a FEI Sirion 200 field-emission gun SEM (FEG-SEM) (FEI, Oregon, USA) operating at a voltage of 3 kV. Micrographs of the SoC substrate were generated using a Zeiss Supra 40 VP field-emission gun SEM (FEG-SEM; Carl Zeiss SMT GmBH, Oberkochen, Germany) operating at a voltage of 3 kV.
Deposition of rhodamine 6G.
A 1 × 10−4 M methanolic rhodamine 6G (R6G) was dropcast onto each of the substrates in 10 μL amounts which covered an area of approximately 0.8 cm2 and allowed to air dry. The analyte was applied to five replicates of each substrate. Each sample was analysed within 1 h of being dried.
Instrument setup
Raman mapping.
Raman mapping of the surfaces was carried out using a WITec Alpha 300R confocal Raman instrument (WITec GmbH, Ulm Germany) fitted with a piezo-driven XYZ scan stage. All samples were probed using a laser wavelength of 632.8 nm. The grating was 600 g mm−1 and coupled to a thermoelectrically cooled charge-coupled device. A spectral resolution of 2.7 cm−1 was achieved over a spectral width consisting of 1024 pixels spanning from 130–2900 cm−1. The unfocussed laser power at the sample was measured at ∼1.5 mW. Spectra were acquired across an area measuring 80 μm × 80 μm using an Olympus 100×/0.9 objective. 80 points per line and 80 lines per image were recorded to give a spatial resolution of 1 μm collecting 6400 spectra in total. Each spectrum had an integration time of 0.08 s.
Results and discussion
Substrate characterisation
In order to establish the reproducibility of SERS from SoC substrates we compared multiple batches of these silver thin films with commercially available substrates. Both Klarite and QSERS are readily available and are fabricated from gold rather than silver. This is because these gold thin films are inert compared to silver, which by contrast is readily oxidised and thus must be prepared immediately before use. We chose not to use galvanic displacement of gold on copper due to the fact that we have optimised the SoC substrate which has been synthesised in our labs and known to be very useful for the detection of R6G and illicit materials.33,35
The SEM images of the three SERS substrates are shown in Fig. 1. The SoC substrate (Fig. 1A) appears to be composed of a number of different sized silver deposits which is consistent with our initial syntheses of these substrates,33 whilst the Klarite surface (Fig. 1B) which is constructed from inverted pyramids coated with gold appears highly uniform. These pyramidal structures are ∼1 μm in diameter. Increased magnification of the microstructures (Fig. 1C) allows the rough gold coating to be seen. The QSERS substrate (Fig. 1D) is constructed from gold nanoparticles of varying sizes which are estimated to be 15 nm and 60 nm as stated by the manufacturer.
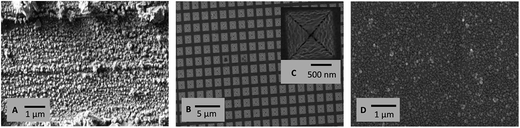 |
| Fig. 1 SEM images of the three SERS substrates are displayed. (A) SoC substrate, (B) Klarite with a magnified pyramidal structure inset (C) and (D) QSERS substrate. | |
Defining common R6G peaks
Five replicate SERS maps were generated on each of the three substrates (Klarite, QSERS and SoC) and exported from instrument manufacturer's software using .DAT files and imported into Matlab (The MathWorks, Inc., Natick, Massachusetts, USA) version 2011a for analysis. Each map consisting of 6400 spectra (80 × 80 pixel, and each pixel contained 1024 data points (wavenumber shifts)) and were initially averaged to elucidate the common R6G peaks, which were present across all 15 data sets. A total of seven common peaks were selected and used for subsequent data analysis, the position of the peaks maxima were at 611 cm−1, 771 cm−1, 1182 cm−1, 1315 cm−1, 1362 cm−1, 1572 cm−1 and 1647 cm−1, and the vibrational assignments for these peaks are given in Table S4.† Although little to no variation in the position of these R6G peaks between the different substrates, if slight shifts were observed these were taken into account when applying analysis methods. The scaled mean spectra from each of the three surfaces Klarite, QSERS and SoC can be seen in Fig. 2, where the red areas in each of the plots highlight the seven common peaks.
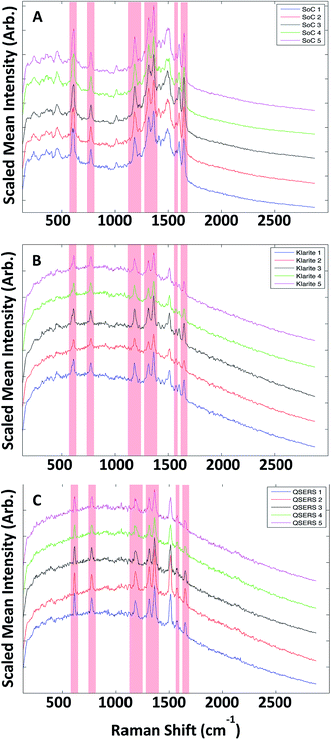 |
| Fig. 2 Staggered plot showing the scaled mean SERS spectra (y-axis = scaled mean intensity, n = 6400) generated on replicates of each of the (A) SoC, (B) Klarite and (C) QSERS substrates. The red shadowed areas show the peaks used for univariate and multivariate data analysis of signal reproducibility. These peaks are positioned at 611 cm−1, 771 cm−1, 1182 cm−1, 1315 cm−1, 1362 cm−1, 1572 cm−1 and 1647 cm−1. Spectra are staggered to allow features to be more easily seen. | |
Extraction of peaks
For each collection the seven common R6G peaks were extracted from all 6400 pixels from each of the 15 maps, to ensure fair assessment of reproducibility across the individual surface and across the five different batches of each of the three substrates.
In order to maintain objectivity the criteria defining the morphology of each peak was kept constant throughout all extractions. Each peak was assigned a maximum as identified earlier. The peak minima however were defined as 3 data points to the left of the maxima corresponding to lower wavenumber shifts and 3 data points to the right corresponding to higher wavenumber shifts. For example the peak at 611 cm−1 would correspond to data point 151 therefore the identified minima are at 148 and 154 corresponding to wavenumbers of 602 cm−1 and 621 cm−1 respectively. Each peak covered an area of ∼20 cm−1. Table S4† shows the peaks maxima and minima, together with their corresponding wavenumbers.
To remove all background contributions the peaks were individually baseline corrected, making certain that the Y values (intensity) at the minima were equal to 0. The two main characteristics of a peak that were used for reproducibility assessment were area and intensity, little preference is shown for either of the two characteristics in Raman or SERS analysis, so to accommodate this data analysis was carried out using calculated values from both. Two methods – trapezoidal integration and sum integration – were used to estimate peak areas. The trapezoidal method (using the TRAPZ.M function in Matlab) calculates the definite integral of a peak by approximating the area contained beneath a curve using a trapezoid, whilst the sum approximation method (also within Matlab) totals the Y values contained within the defined area (i.e., the individual heights of the 7 data points within each peak). Peak intensity was calculated by extracting the Y value which corresponded to the peak maxima. The mean peak areas and intensities together with standard deviations and relative standard deviations calculated from each of the replicate substrates Klarite, QSERS and SoC can be seen in Tables S1–S3a–e.† The results from the individual surfaces show that signal reproducibility is greatest on the QSERS substrate whose lowest mean area (both trapezoidal and sum) RSD across all peaks is calculated to be 33.0% whilst 47.6% is representative of the SoC substrate and 54.6% of the Klarite surface. The RSD of intensity however tells a slightly different story with the SoC substrate appearing the most reproducible with the lowest mean area RSD across all peaks being 53.0%, the second most reproducible thin film was the QSERS substrate (63.0%) with the Klarite surface being most irreproducible with a RSD of 72.8%. To analyse the repeatability of R6G signal between batches of the same substrate, the mean areas calculated using the trapezoidal methodology and intensities were used. The mean RSDs with respect to all peak RSDs calculated across the three substrates are shown in Table 1. The substrate which demonstrates the best batch-to-batch reproducibility (repeatability) based on area is Klarite with an RSD of 13%. The SoC substrate shows the second best reproducibility with an RSD which is slightly higher of 13.5% and QSERS is the least reproducible (16.7%), when intensity is used rather than area. By contrast, a low morphological scores (MS)34 implies a sequence with its major variations is in high frequency domain, which normally means noise. A common value of MS ≤ 0.75 was used on all data sets to ascertain the number of spectra generated on each surface directly relating to noise. The maps in Fig. 3 are generated using the total peak area of the recombined peaks whilst the maps in Fig. S1–S3† show the position of spectra with an MS ≤ or ≥ 0.75. Also present in the figures are plots showing the discrimination of the R6G spectra from the noise on the three surfaces.
Table 1 Mean RSDs calculated across all peak areas and intensities for assessment of batch to batch reproducibility (repeatability) and the calculated mean number of noisy spectra and estimated percentage R6G coverage across all substrate replicates
|
Mean RSDs (%) |
MS ≤ 0.75 |
Peak area (trapezoidal) |
Peak intensity |
Mean number of noisy spectra |
Percentage R6G coverage |
Klarite |
13 |
19.7 |
440 |
93.13 |
QSERS |
16.7 |
21.41 |
338 |
94.72 |
SoC |
13.5 |
17.8 |
5 |
99.92 |
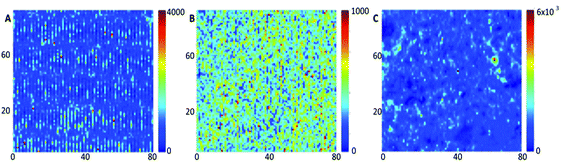 |
| Fig. 3 Example SERS maps generated based on the total peak area of the sum of the 7 R6G peaks. (A) Map representative of Klarite 4 substrate, (B) map representative of QSERS 4 substrate and (C) map representative of SoC 5 substrate. | |
Validation of the MS method was carried out manually by checking the spectra that appear on the MS = 0.75 boundary, this revealed that the discriminatory analysis was very accurate with the assigned noise (MS ≤ 0.75) having no assignable R6G peaks. It was also observed that the peak at 1647 cm−1 assignable to an aromatic C–C stretch was less prevalent in QSERS when compared to Klarite and SoC, the reasoning behind this is unclear but could possibly be due to the molecule residing in a different orientation in comparison to the other two substrates (although there is no direct evidence of this). The average number of non-R6G spectra and estimated surface coverage identified on the five replicates of the three surfaces is shown in Table 1.
Klarite substrates were shown to have the largest number of non-R6G spectra (n = 440) whilst the SoC substrate had the lowest (n = 5). It should be noted however that whilst QSERS had on average only 338 spectra un-assignable to R6G the variation between the number of noise related spectra on each surface was much greater than Klarite or SoC with one surface having only 7 spectra identified as non-R6G whilst another had 828, this could also be due to the lack of control exercised over the substrates synthesis, as mentioned earlier.
Multivariate data analysis was also employed as an extension of univariate methods used to assess substrate reproducibility. Principal components analysis (PCA) was applied to each of the recombined peak datasets followed by the calculation of the data volume across the first 3 PCs (explained below). This was performed in order to assess the dataset distribution. An example PCA plot from the SoC substrate is shown in Fig. S4,† the total explained variance for QSERS across PC1 and PC2 is very low showing that the spectra were not highly correlated.
The average relative standard deviation of volumes across all replicate surfaces is lowest for the SoC substrate (32.8%) showing that the spectra generated from this surface is more highly correlated than the Klarite or QSERS who both have RSDs of ∼61.0%. Euclidean distances were also used to calculate variation across PCs 1–3. To achieve this a mean of all the scores values was calculated and the average distance to each of the individual scores was computed. RSDs were calculated for each substrate, and revealed that the SoC substrate had the lowest RSD of 14.5%, whilst QSERS and Klarite had RSDs of 27.0% and 29.9%, respectively.
It is evident from the data analysis carried out that no one method accurately explains the reproducibility and repeatability of the substrates, therefore using a number of analysis techniques it is possible to build a much more accurate and importantly objective view of a surfaces performance. A traffic light based summary of the results can be seen in Table 2, here each of the analysis methods are listed and the RSDs relating to each substrate are displayed. The highest RSDs calculated for each method are highlighted in red, whilst intermediate and low RSDs are displayed in yellow and green respectively. To add weighting to the colour system, red highlighted RSDs were given a score of 2, yellow 1 and green 0, therefore the lowest total for the colour system represents the most reproducible substrate by comparison. This summary (Table 2) revealed that the SoC substrate produced by far the most reproducible R6G signal overall when compared to Klarite and QSERS.
Table 2 A traffic light based summary of the substrates performance is shown. The red represents the most irreproducible substrate based on the analysis method used, whilst yellow and green highlighting, eludes to the substrates demonstrating intermediate and highest reproducibility. Each colour is given a weighting, allowing the substrates performances to be compared. Red = 2, yellow = 1 and green = 0, hence the substrates with the lowest overall score is deemed the most reproducible
One other important aspect of this work was to ascertain the minimum number of spectra needed give a robust and fair analysis of a substrate's performance. Often in published articles the number of spectra taken to derive a substrates performance is too few, resulting in the quotation of misleading (often optimistically low) RSDs. In all the analysis shown here the RSDs were calculated across all 6400 spectra generated on each replicate surface resulting 32
000 spectra being collected for each substrate set (Klarite, QSERS and SoC). These data sets are exceptionally large and not all groups have the capabilities to collect as many spectra, therefore a smaller number of spectra are needed without the loss of statistical integrity. Initially 20 random spectra were selected from the substrate and the RSD of peak area of the seven common peaks was calculated. This approach was repeated using boot strapping without replacement (1000 iterations) to carry out the random reselection approach. The overall RSD was then estimated for each of the 1000 RSDs of peak area to show the variation in the relative standard deviation as a result of the number of spectra evaluated. The number of random spectra selected was then increased in 20 spectra steps up to 6400 spectra where the RSD converged at 0. The average number of spectra (across all samples) needed to be collected to achieve RSDs on the full RSD (from all spectra in the maps) less than 20%, 15%, 10% and 5% was calculated (Table 3). By carrying out these calculations it can be seen a minimum of 180 spectra is needed to estimate the performance of the substrates with less than 20% expected variation, whilst less than 5% variation necessitates 2040 spectra to be analysed. Clearly experiments showing SERS optimisation should not report 10s of spectra in the analysis as is currently commonly used as reproducibility tests on so few spectra will not be statistically valid.
Table 3 Shows the relationship between the variation in RSD and the number of spectra collected
Variation in RSD |
<20% |
<15% |
<10% |
<5% |
Number of spectra |
180 |
300 |
660 |
2040 |
Conclusion
It has been demonstrated that the SoC substrate synthesised in-house has much better reproducibility overall than two readily available commercial substrates. We have shown that whilst galvanic displacement may not be able to produce uniform morphologies like lithographically produced substrates their reproducibility can be much better. Also, the ability to synthesise the SoC substrate in-house means that SERS can be facilitated at low cost by non-specialist groups, making the technique much more accessible. We have also successfully verified that using only one method of data analysis is insufficient to elucidate a substrate's performance. Here seven different methods have been used to compare the reproducibility of the substrates. Finally, through the collection of large datasets on multiple batches of each substrate replicate it was also possible to generate a guide for other groups as to the acceptable number spectra to collect, to maintain statistical integrity; clearly papers assessing a surface's performance for SERS should measure 100s of spectra rather than the 10s reported in the literature.
Overall, a simple, generalised protocol for the analysis and comparison of SERS substrates has been developed, using R6G as a probe analyte. We recommend that others perform such analyses on their thin films for SERS when reporting reproducibility and that these should be collected from a minimum of 180 spectra, collected randomly from across the surface.
Acknowledgements
The authors thank the RSC and EPSRC for funding S. M.'s Analytical Ph.D. Studentship. R. G. also thanks BBSRC (BB/L014823/1) for financial support of Raman spectroscopy.
References
- M. Fleischmann, P. J. Hendra and A. J. McQuillan, Chem. Phys. Lett., 1974, 26, 163 CrossRef CAS.
- R. P. Van Duyne and D. L. Jeanmaire, J. Electroanal. Chem., 1977, 84, 1 CrossRef.
- M. Moskovits, Rev. Mod. Phys., 1985, 57, 783 CrossRef CAS.
- P. S. Kumar, I. Pastoriza-Santos, B. Rodríguez-Gonzalez, F. J. G. de Abajo and L. M. Liz-Marzan, Nanotechnology, 2008, 19, 015606 CrossRef PubMed.
- C. J. Murphy, T. K. San, A. M. Gole, C. J. Orendorff, J. X. Gao, L. Gou, S. E. Hunyadi and T. Li, J. Phys. Chem. B, 2005, 109, 13857 CrossRef CAS PubMed.
- L. Baia, M. Baia, J. Popp and S. Astilean, J. Phys. Chem. B, 2006, 110, 23982 CrossRef CAS PubMed.
- L. A. Dick, A. D. McFarland, C. L. Haynes and R. P. Van Duyne, J. Phys. Chem. B, 2002, 106, 853 CrossRef CAS.
- M. Fan and A. G. Brolo, Phys. Chem. Chem. Phys., 2009, 11, 7381 RSC.
- S. Bernard, N. Felidj, S. Truong, P. Peretti, G. Levi and J. Aubard, Biopolymers, 2002, 67, 314 CrossRef CAS PubMed.
- Q. M. Yu, P. Guan, D. Qin, G. Golden and P. M. Wallace, Nano Lett., 2008, 8, 1923 CrossRef CAS PubMed.
- J. E. G. J. Wijnhoven, S. J. M. Zevenhuizen, M. A. Hendriks, D. Vanmaekelbergh, J. J. Kelly and W. L. Vos, Adv. Mater., 2000, 12, 888 CrossRef CAS.
- F. Giorgis, E. Descrovi, A. Chiodoni, E. Froner, M. Scarpa, A. Venturello and F. Geobaldo, Appl. Surf. Sci., 2008, 254, 7494 CrossRef CAS.
- S. P. Mulvaney, L. He, M. J. Natan and C. D. Keating, J. Raman Spectrosc., 2003, 34, 163 CrossRef CAS.
- K. C. Grabar, R. G. Freeman, M. B. Hommer and M. J. Natan, Anal. Chem., 1995, 67, 735 CrossRef CAS.
- L. Gunnarsson, E. J. Bjerneld, H. Xu, S. Petronis, B. Kasemo and M. Kall, Appl. Phys. Lett., 2001, 78, 802 CrossRef CAS.
- C. L. Haynes, A. D. McFarland, L. L. Zhao, R. P. Van Duyne, G. C. Schatz, L. Gunnarsson, J. Prikulis, B. Kasemo and M. Kall, J. Phys. Chem. B, 2003, 107, 7337 CrossRef CAS.
- M. A. De Jesus, K. S. Giesfeldt, J. M. Oran, N. A. Abu-Hatab, N. V. Lavrik and M. J. Sepaniak, Appl. Spectrosc., 2005, 59, 1501 CrossRef CAS PubMed.
- K. G. M. Laurier, M. Poets, F. Vermoortele, G. De Cremer, J. A. Martens, H. Uji-I, D. E. De Vos, J. Hofkensa and M. B. J. Roeffaers, Chem. Commun., 2012, 48, 1559 RSC.
- Y. Y. Xia and J. M. Wang, Mater. Chem. Phys., 2011, 125, 267 CrossRef CAS.
- M. I. Stockman, V. M. Shalaev, M. Moskovits, R. Botet and T. F. George, Phys. Rev. B: Condens. Matter Mater. Phys., 1992, 46, 2821 CrossRef.
- A. Gopinath, S. V. Boriskina, B. M. Reinhard and L. Dal Negro, Opt. Express, 2009, 17, 3741 CrossRef CAS PubMed.
- B. Yan, A. Thubagere, W. Ranjith Premasiri, L. D. Ziegler, L. Dal Negro and B. M. Reinhard, ACS Nano, 2009, 3, 1190 CrossRef CAS PubMed.
- M. Kahl, E. Voges, S. Kostrewa, C. Viets and W. Hill, Sens. Actuators, B, 1998, 51, 285 CrossRef CAS.
- A. G. Brolo, E. Arctander, R. Gordon, B. Leathem and K. L. Kavanagh, Nano Lett., 2004, 4, 2015 CrossRef CAS.
- P. L. Stiles, J. A. Dieringer, N. C. Shah and R. P. Van Duyne, Annu. Rev. Anal. Chem., 2008, 1, 601 CrossRef CAS PubMed.
- S. M. Nie and S. R. Emory, Science, 1997, 275, 1102 CrossRef CAS PubMed.
- J. P. Schmidt, S. E. Cross and S. K. Buratto, J. Chem. Phys., 2004, 121, 10657 CrossRef CAS PubMed.
- K. Hering, D. Cialla, K. Ackermann, T. Doerfer, R. Moeller, H. Schneide-wind, R. Mattheis, W. Fritzsche, P. Roesch and J. Popp, Anal. Bioanal. Chem., 2008, 390, 113 CrossRef CAS PubMed.
- M. J. Natan, Faraday Discuss., 2006, 132, 321 RSC.
- M. J. Banholzer, J. E. Millstone, L. D. Qin and C. A. Mirkin, Chem. Soc. Rev., 2008, 37, 885 RSC.
- M. Moskovits, J. Raman Spectrosc., 2005, 36, 485 CrossRef CAS.
- M. Fan, G. F. S. Andrade and A. G. Brolo, Anal. Chim. Acta, 2011, 693, 7 CrossRef CAS PubMed.
- S. Mabbott, I. Larmour, V. Vishnyakov, Y. Xu, D. Graham and R. Goodacre, Analyst, 2012, 137, 2791 RSC.
- H. Shen, L. Stordrange, R. Manne, O. M. Kvalheim and Y. Liang, Chemom. Intell. Lab. Syst., 2000, 51, 37 CrossRef CAS.
- S. Mabbott, A. Eckmann, C. Casiraghi and R. Goodacre, Analyst, 2013, 138, 118 RSC.
- A. Gutés, C. Carraro and R. Maboudian, J. Am. Chem. Soc., 2010, 132, 1476 CrossRef PubMed.
- Y. Lai, W. Pan, D. Zhang and J. Zhan, Nanoscale, 2011, 3, 2134 RSC.
- J. F. Betz, Y. Cheng and G. W. Rubloff, Analyst, 2012, 137, 826 RSC.
Footnote |
† Electronic supplementary information (ESI) available. See DOI: 10.1039/c7ay01584d |
|
This journal is © The Royal Society of Chemistry 2017 |
Click here to see how this site uses Cookies. View our privacy policy here.