DOI:
10.1039/C7AY01118K
(Paper)
Anal. Methods, 2017,
9, 3237-3243
A simple design atomic emission spectrometer combined with multivariate image analysis for the determination of sodium content in urine†
Received
30th April 2017
, Accepted 12th May 2017
First published on 15th May 2017
Abstract
In clinical analysis the determination of alkali metals in biological samples is often performed by flame emission spectroscopy (FES). Despite its good analytical performance for the determination of trace elements, FES apparatus is often cumbersome, expensive, and energy dependent and thus, not applicable for on-site measurements. In an attempt to address these limitations, a simple digital atomic emission spectrometer built from easily available components is proposed in this study for the quantification of sodium in human urine samples. The system was designed to operate in the absence of electrical power supply with a sample consumption per measurement of about 10 μL. It comprises a traditional Bunsen burner with an air-propane flame embedded in a protective box, a silver wire loop for sample introduction and a smartphone camera for digital recording of the signal emitted by the analyte. The collected video frame is subjected to a multivariate image procedure based on multivariate curve resolution – alternating least squares (MCR-ALS) intended to avoid subjectivity in the selection of the region of interest (ROI) used to build the univariate regression model. The developed platform was evaluated in the context of sodium determination in human urine in the range from 40 to 220 mmol L−1. The system displays a linear response (R2 = 0.990) between the color index and sodium content for standard samples with a detection limit of 10.9 mmol L−1. Performance study for intra-day (2.5–4.1%) and inter-day (1.3–2.5%) measurements, performed on three different days, exhibits good repeatability. The homemade digital atomic emission spectrometer was successfully applied to the determination of (spiked) sodium in human urine samples (R2 = 0.942) with recovery that ranged from 94.8 to 110.4% and an averaged mean relative error below 10%. Selectivity study against potassium, calcium and magnesium revealed high tolerance of the method for these interferents.
1. Introduction
Flame emission spectroscopy (FES) is a standard analytical technique1,2 routinely employed in clinical analysis for quantitative determination of alkali elements in complex biological samples such as blood plasma or urine. The attractive feature of FES is its capability to detect multiple elements simultaneously with a high sensitivity.3 The multi-element identification is achieved through the detection of a specific spectral signature, in the form of a discrete emission spectrum. Since the intensity of the emitted light is proportional to the element concentration over several orders of magnitude, FES is a method of choice for a broad range of applications ranging from trace analysis to high concentration level determination.4–8
The accurate determination of sodium content in biological samples is of primary importance in fields such as food chemistry9 or clinical diagnosis.10,11 Owing to its high selectivity, FES usually provides for a fast and reliable quantification in agreement with clinical standards by taking advantage of the characteristic prominent yellow emission from sodium. The performance of FES can nonetheless be altered by the presence of easily ionized elements12–16 leading to biased quantification of low sodium content or trace elements. This situation is mainly encountered in complex samples such as urine, for which the normal sodium level is located in the range 40–220 mmol L−1 per day, where the matrix composition shows great variability from sample to sample. In order to correct for potential interference, methods such as standard additions or internal standards are usually required.17
Commercially available FES systems are usually restricted to specialized laboratories or academic institutes as a consequence of their relatively high cost, bulkiness and energy dependence. In an attempt to apply FES in an on-site mode, efforts have to be devoted to the design of homemade or custom flame emission spectrometers able to minimize each of the above criteria. This strategy is enabled by considering the relatively simple architecture of commercial flame emission spectrometers for which sophisticated parts of the instrument can be easily substituted by simple and cost-effective devices. In practice cumbersome items such as plasma torches can be replaced by more traditional and energy saving light sources based on Bunsen18 or camping burners.19 Likewise the development of embedded systems for which the signal detection is based on a built-in CCD camera20,21 or webcam22 is likely to be more suitable for on-site measurements. It is worth noting that by achieving digital detection, instruments such as photomultipliers and monochromators are discarded in favor of a simpler image processing procedure. Recorded images are decomposed in the RGB (red–green–blue) color system from which a specific mathematic model can be derived to yield appropriate calibration curves.
The conversion of a liquid sample into a fine spray or aerosol is usually a critical step if one wants to achieve a low detection limit23 in FES. In an attempt to substitute expensive commercial systems, several approaches based on 3D-printed19 or custom-made nebulizers24 have been reported. However, despite the efforts made in recent years, sample nebulization still partially require tedious experimental design and/or sophisticated instruments. So far the simplest sample introduction method, as reported by Moraes and co-workers, involves perfume spray bottles and an open-air flame from a Bunsen burner.25 Although this system is suitable for educational purposes, its adaptability to on-site measurement of biological samples is yet questionable. First, spray bottles usually require large liquid volumes which are not always available for particular samples such as blood serum. Second, experiment ergonomics are not optimal as it involves two operators respectively in charge of sample nebulization and flame detection via a smartphone camera, provided that sample spraying is done at an appropriate and fixed distance from the flame to prevent extinguishment. Finally, the digital image-based procedure to define the region of the flame that exhibits the most intense light is set manually and thus it is time-consuming and highly subjective.
In this context, we propose a homemade FES platform for the determination of sodium content in human urine which can potentially operate in an on-site mode. The system is built around a protective box in which an air–propane flame source from a traditional Bunsen burner is embedded. A 10 μL aliquot of the urine sample is introduced via a homemade silver wire loop mounted on a screwdriver and directed toward the flame. A commercial smartphone with a built-in camera is positioned in the front of the box and it captures color changes of the flame through a transparent plastic observation window. Sample introduction and digital recording are achieved simultaneously by a single operator and it takes no more than 10 seconds for each measurement. The recorded signal, in the form of a video frame, is subjected to the special image processing procedure based on multivariate curve resolution – alternating least squares (MCR-ALS).26 In addition to preventing subjectivity in the selection of the ROI, this strategy does not require background correction as image contributions of lower intensity are naturally discarded by the bilinear model assumed by MCR-ALS. The averaged intensity inside the ROI is finally computed and correlated with the known concentration of sodium in calibration urine samples. The derived linear regression model can be further employed for the analysis of new samples.
2. Materials and methods
2.1 Reagents
Sodium chloride, potassium chloride, calcium chloride dihydrate and magnesium chloride hexahydrate (analytical grade – 99.9%) were purchased from Sigma Aldrich. The concentration of standard sodium solutions was adjusted between 40 and 220 mmol L−1 in order to match the reference range considered in clinical analysis. Concentrated solutions of sodium (2.52 mol L−1), potassium (1.05 mol L−1), calcium (0.74 mol L−1) and magnesium (0.42 mol L−1) were prepared from their respective salts in distilled water and used as working solutions for recovery and interference studies.
2.2 Biological samples
Urine samples (n = 22) were collected from healthy volunteers by the Urolithiasis Laboratory (Medical Center of Laboratory Diagnostics, St. Petersburg) and stored in a biomedical fridge at – 25 °C. Following a dilution by a factor 400–800, the content of inorganic cations (calcium, magnesium, sodium, and potassium) was assessed in the range 190–400 nm by using a “Capel 105 M” (Lumex, Russia) capillary electrophoresis system based on the procedure described in ref. 27. All reference data were collected in the Bioanalytical Laboratory CSU “Analytical Spectrometry” (St. Petersburg, Russia). Sodium content in undiluted urine samples was found ranging between 40.6 and 218.3 mmol L−1.
Prior to analysis, raw samples were thawed at room temperature (25 °C) and used without dilution. Spiked samples for recovery studies were prepared by mixing 200 μL of human urine with 10 μL of an appropriate working solution diluted accordingly.
2.3 Development of a homemade atomic emission spectrometer
A scheme of the designed platform for sodium quantification in human urine is shown in Fig. 1. The proposed analytical system is built from cheap and commonly available materials. It comprises a classical Bunsen burner, a thick closing timber box, a screwdriver, a thin silver wire, a transparent CD/DVD plastic case and a commercial smartphone. Assembly of the instrument starts by securing the Bunsen burner on a flat surface. The upper part of the burner (about 1.5 cm) is inserted into the bottom side of the box (vertically oriented) at the barycentre position. The box has the dimensions of 30.5 × 27 × 14 cm (H × L × W) and it is firmly maintained above the flat surface by using homemade blocks placed at each corner. Wood was selected among other materials due to its low thermal conductivity (≈0.12 W m °C−1) in order to prevent heat exposure and minimize skin burning risks. For safety reasons, the flame height is adjusted to less than one third of the box height (about 8 cm). Meanwhile small openings are drilled on top of the box to enable heat removal and avoid wood ignition. Once the burner is switched on, the inner part of the box is secured from potential outside interference such as air streams and/or ambient light by closing the box front door. During the process, the stability of the flame is monitored through an observation window made from a piece of a colorless transparent CD/DVD plastic case fixed with tape on the lower side of the door. The obtained bluish flame is particularly adapted for the atomization of alkali metals28 and features a lower background contribution compared to yellow flames.
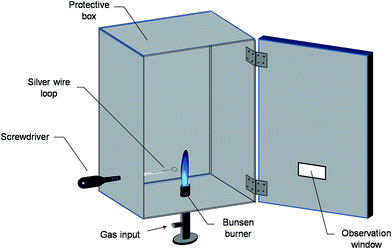 |
| Fig. 1 Scheme of the homemade FES platform built from commonly available materials for the determination of sodium content in urine. | |
The introduction of the sample into the flame is achieved through a thin silver wire mounted with tape on the metallic part of a screwdriver. The end of the wire is manually curved in order to form a circular loop of diameter 3.1 mm. Standardization of the loop form and dimensions is ensured by using a commercial jack male RCA video cable (see Fig. S1 in the ESI†). Prior to measurement, the loop is rinsed three times with distilled water and subsequently immersed in the sample. The screwdriver – silver wire assembly containing the droplet is then inserted into the analytical chamber via a 1 cm hole located on the left side of the box. In order to avoid an early contact with the flame, the sample is first inserted at the angle of approximately 45° towards the left surface of the box. When the screwdriver handle, whose diameter is larger than 1 cm, is in contact with the box the sample holder is put in the 90° position. A wood block placed inside the analytical chamber guide the screwdriver into the optimal alignment position with the flame so that the droplet reaches the upper part of the burner at its center position. The total wire length, the loop included, is about 10.5 cm. The silver loop is carefully washed with distilled water after each measurement and replaced after every 10 measurements to prevent potential damages to the wire. Unlike systems operating via nebulizers or spray bottles, the developed procedure does not require tedious design and has a sample consumption per measurement of about 10 μL.
Throughout the process, the color of the flame is monitored by using a smartphone (Nokia E72 with a 5 M pixel built-in camera, resolution of 480 × 640 pixels) located at the observation window. The capture of the video frame (14 frames per s) starts once the droplet is in contact with the flame and ends as soon as the sample is totally consumed. The average video duration is approximately 10 s. Each sample is measured in triplicate. The subsequent video frame is then transferred to a computer and converted into the AVI format prior to multivariate image analysis.
2.4 Hazards
Switching on the burner inside the box can be potentially dangerous. Therefore a safe distance from the flame should be maintained during the process. Long lighters such as piezoelectric flame gas lighters for kitchen are strongly advised for the sake of safety. Contact with the top of the box should be avoided while performing experiment to prevent skin burning. Although silver is an inert material, its exposure to the flame should not be extended more than 15 seconds, otherwise overheating and melting of the metal might be observed, destroying the loop in the process.
2.5 Multivariate image processing
All video frames were processed in Matlab 7.5 (the MathWorks Ltd, Massachusetts) based on a homemade procedure detailed in the flowchart shown in Fig. 2. The first step consists in uploading the video frame of a particular sample measured in triplicate into the Matlab workspace. For each video sequence a single representative frame (480 × 640 × 3) is selected for analysis. Selection criteria are the presence of highly intense RGB coefficients homogeneously distributed over a significant pixel region and the absence of (saturated) bright pixels. Once selected, the three images (one per replicate) are converted into grayscale images (480 × 640) with double precision. This operation removes hue and saturation from the image while retaining the luminance containing most of the data. The average grayscale image is then computed and subjected to Multivariate Curve Resolution – Alternating Least Squares.26 This is a standard soft-modeling approach intended for the decomposition of a data matrix or image D (480 × 640) into the bilinear model given in eqn (1):where C (480, k) and ST (k, 640) correspond to the k intensity profiles along x and y pixel dimensions of the image, respectively. The profiles are optimized iteratively under constraints by a least squares procedure in order to mimic every physical property which grayscale images are expected to fulfill such as non-negative intensity. Further information about MCR-ALS is available in ref. 29.
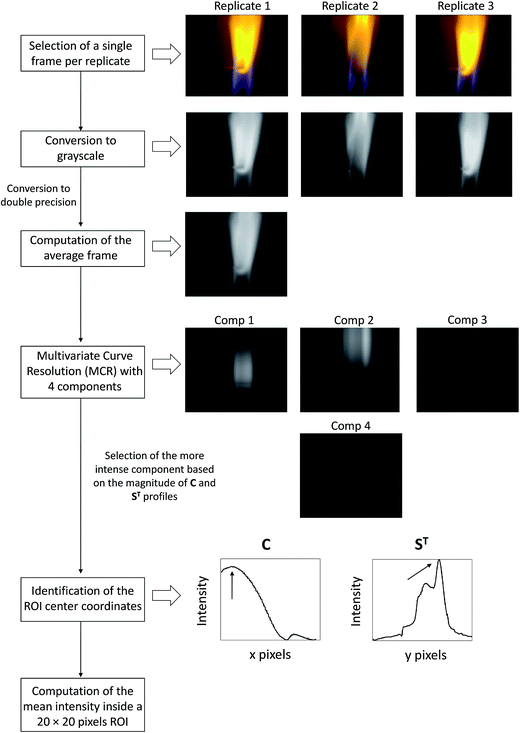 |
| Fig. 2 Flowchart of the multivariate image processing used for the analysis of video frames collected by the developed FES platform. | |
In order to retain only the significant information of the image a 4 component MCR model (k = 4), in agreement with singular values decomposition,30 is built and optimized under non-negativity constraints. For this purpose, the bilinear model shown in eqn (1) can be rewritten as the following eqn (2):
| D = C1·ST1 + C2·ST2 + C3·ST3 + C4·ST4 | (2) |
with each pair of
Ci·
STi profiles corresponding to a new image explaining a particular source of variance contained in the input grayscale image
D. As one can see in
Fig. 2, each
Ci·
STi couple gives access to selective information of the image. This property is exploited to separate information associated with the sample emission from other contributions of lower importance. In this way background correction is not required as the background or other interference can be removed by disregarding
Ci·
STi couples which are not representative of sample emission only. The identification of the
Ci·
STi pair, suitable for sodium determination, is achieved by selecting the optimized
C profile of higher intensity. A further advantage of this method is the possibility to reduce subjectivity in the selection of the region of interest (ROI). The position of the maximum of the
C profile and its corresponding
ST profile gives the center coordinate of the ROI along
x and
y pixel dimensions. Around this center point, the ROI is defined as a 20 × 20 pixel region from which the average grayscale intensity is computed. This intensity is used to build a univariate regression model for the assessment of sodium content in the sample. A detailed example of ROI selection is given in the next section.
3. Results and discussion
3.1 Calibration in model sodium samples
The feasibility of the developed FES platform for the determination of sodium content was first evaluated on a set of 7 standard sodium samples with concentration ranging from 40 to 220 mmol L−1. Following the application of the multivariate image processing described in the previous section, the average grayscale intensity recovered for each standard is plotted against the known concentration of sodium in the sample. The corresponding univariate model, displayed in Fig. 3 (right), exhibits strong correlation (R2 = 0.9904) and is expressed by the equation y = 0.0017x + 0.4008. The mean relative error (5.6%) is within the 10% average tolerance usually considered in clinical analysis. For the sake of clarity, an example of ROI selection in the case of a 130 mmol L−1 standard sample is given for information (see the left panel in Fig. 3).
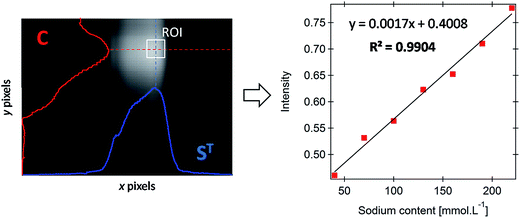 |
| Fig. 3 (Left) illustration of the procedure used to define the ROI for a 130 mmol L−1 standard sodium sample and (right) calibration line obtained for the 7 standard sodium samples in the range 40–220 mmol L−1. | |
The analytical repeatability was studied by collecting four replicate video frames on the same day and on 3 consecutive days for standard sodium samples at 50, 120 and 200 mmol L−1. The intra-day and inter-day repeatability were found satisfactory with coefficients of variation of 3.05 and 1.97%, respectively. The average standard deviation for intra-day and inter-day replicate measurements ranges from 8.96 to 12.35 mmol L−1. Variability in the predicted sodium concentrations is mainly attributed to the size and geometry imperfections of the silver loop, both influencing the repeatability of the sampled volume. For the developed platform the limit of detection (LOD) was evaluated to be 10.87 mmol L−1 based on eqn (3):
|  | (3) |
with
σ the standard deviation of 10 measurements of the flame source (blank) in the absence of the sample and
β the slope of the analytical line displayed in
Fig. 3.
3.2 Calibration for sodium in urine
The performance of the developed FES platform for the analysis of complex biological samples was assessed for a set of 22 human urine samples. The corresponding dependence is shown in Fig. 4 and reveals reasonable linearity (R2 = 0.9420) with a mean relative error of 9.5%. The degradation of the univariate model performance is clearly ascribed to the complex composition of urine samples, more precisely to the variation in the urine composition from sample to sample. In order to rationalize the measurement error, we rely on a more realistic figure of merit defined by eqn (4): | 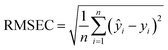 | (4) |
where RMSEC stands for the root mean square error of calibration, and n denotes the size of the calibration set while ŷi and yi represent reference sodium values and the concentration predicted by the calibration model, respectively. Since RMSEC is directly expressed in concentration units, a better insight into the predictive ability of the calibration model can be achieved compared with traditional estimators such as mean relative error. From the calibration line plotted in Fig. 4, the calculated RMSEC value for urinary sodium is 12.66 mmol L−1. This value is approximately twice bigger than the one recovered for standard samples in Fig. 3 (6.54 mmol L−1) which suggests that the system is sensitive to the presence of concomitant species. The most likely source of interference corresponds to chemical elements of low ionization potential which are partially ionized at flame temperature. Free electrons liberated by ionization of elements such as potassium are likely to favor the formation of free sodium atoms leading to an increase in the overall signal.31
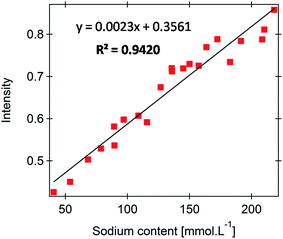 |
| Fig. 4 Calibration for urinary sodium determined from 22 urine samples in the range 40–220 mmol L−1. | |
The extent of interference from easily ionized elements over sodium determination has been investigated by spiking four urine samples with two standard sodium solutions. The recovery study exhibited reasonable performance (94.8–110.4%), in light of the relative simplicity of the developed system, characterized by an average recovery value of 101.3%. This suggests that interference from concomitant species has a little impact on the determination of sodium content in urine. The results are summarized in Table 1.
Table 1 Analytical recovery of sodium in human urine samples
Sodium (mmol L−1) |
Added (mmol L−1) |
Found (mmol L−1) |
Recovered (mmol L−1) |
Recovery (%) |
85.3 |
60.0 |
148.8 |
63.5 |
105.8 |
120.0 |
201.1 |
115.8 |
96.5 |
129.4 |
40.0 |
170.0 |
40.6 |
101.5 |
85.0 |
216.4 |
87.0 |
102.4 |
43.4 |
30.0 |
74.4 |
31.0 |
103.4 |
120.0 |
158.0 |
114.6 |
95.5 |
100.1 |
85.0 |
180.7 |
80.6 |
94.8 |
115.0 |
227.0 |
126.9 |
110.4 |
In an attempt to evaluate the sensitivity of the developed FES platform toward a given concomitant species, a recovery study with a particular emphasis on main interfering species such as potassium, calcium and magnesium was performed. With the criteria of a 95–105% recovery range considered as acceptable, recovery values displayed in Table 2 are all located within the tolerance interval regardless of the employed concomitant. In general, lower levels of potassium, calcium or magnesium are not found to interfere significantly with sodium determination provided that sodium concentration is sufficiently high. When this condition is not fulfilled, as for sample 1 following the addition of a 50 mmol L−1 standard potassium solution, mutual interference between sodium and potassium can be observed as suggested by a lower recovery value below the 95% mark.
Table 2 Analytical recovery of sodium in human urine samples in the presence of potassium, calcium and magnesium
Urine sample |
Sodium (mmol L−1) |
Potassium (mmol L−1) |
Added K (mmol L−1) |
Found (mmol L−1) |
Recovery (%) |
1 |
85.3 |
61.7 |
10.0 |
85.5 |
100.2 |
|
|
50.0 |
80.9 |
94.8 |
2 |
200.3 |
39.1 |
10.0 |
198.6 |
99.1 |
|
|
50.0 |
210.8 |
105.2 |
3 |
182.4 |
71.7 |
10.0 |
179.1 |
98.2 |
|
|
50.0 |
185.4 |
101.6 |
Urine sample |
Sodium (mmol L−1) |
Calcium (mmol L−1) |
Added Ca (mmol L−1) |
Found (mmol L−1) |
Recovery (%) |
4 |
129.2 |
10.8 |
35.0 |
135.3 |
104.7 |
Urine sample |
Sodium (mmol L−1) |
Magnesium (mmol L−1) |
Added Mg (mmol L−1) |
Found (mmol L−1) |
Recovery (%) |
5 |
155.9 |
4.6 |
20.0 |
160.5 |
103.0 |
The results demonstrate that multivariate image analysis applied to the developed FES platform made from cheap and commonly available materials can provide for a reliable quantification of sodium in urine. Since calcium and magnesium levels are usually low in human urine, potassium remains the main source of interference although potassium content was found to produce moderate effect over sodium determination.
4. Conclusion
A cost-effective atomic emission spectrometer built from cheap and commonly available materials has been proposed and evaluated for the determination of sodium content in human urine. The system was developed keeping in mind the possibility to perform experiments at distant locations in an on-site mode. For this purpose the architecture of the spectrometer was built around easily available components along with a battery powered detecting device. These specifications have been fulfilled using a laboratory burner, a protective box, a silver wire for low volume sample introduction and a commercial smartphone built-in camera. The acquired video frame was subjected to a specially designed multivariate image analysis procedure preventing subjectivity in the selection of the ROI. Recovery studies suggest that the presence of concomitant elements with low ionization potential has little or moderate influence over sodium determination. The mean relative error for sodium determination does not exceed 10% and is in good agreement with standard error tolerated in clinical diagnosis. The single element detection of the developed flame emission spectrometer can be tentatively upgraded to multi-element detection by placing an optical-fiber coupled with a USB powered spectrophotometer at the observation window. Based on the reasonable performance of the FES platform, and its apparent modularity, we believe that this system might be a potential candidate for the quantitative analysis of metal elements in complex biological samples in cases when traditional laboratory equipment is not available.
Acknowledgements
B. Debus would like to acknowledge the financial support from St. Petersburg State University PostDoc Grant #12.50.1191.2014. This work was partially financially supported by the Government of Russian Federation, Grant 074-U01. The authors would like to thank Alla Sidorova and Alena Piven from the Bioanalytical Laboratory CSU “Analytical Spectrometry” (St. Petersburg State Polytechnical University, Russia) for their assistance with urine samples.
References
-
J. R. French, U. S. A. E. Commission and L. Oak Ridge National, Determination of Alkali Metal and Alkaline-Earth Elements by Flame Photometry: a Bibliography, Oak Ridge National Laboratory, Oak Ridge, Tenn., 1955 Search PubMed.
- G. M. Hieftje, J. Chem. Educ., 2000, 77, 577 CrossRef CAS.
- E. S. Dipietro, M. M. Bashor, P. E. Stroud, B. J. Smarr, B. J. Burgess, W. E. Turner and J. W. Neese, Sci. Total Environ., 1988, 74, 249–262 CrossRef CAS PubMed.
- D. Bellido-Milla, J. M. Moreno-Perez and M. P. Hernandez-Artiga, Spectrochim. Acta, Part B, 2000, 55, 855–864 CrossRef.
- E. Capuano, G. van der Veer, P. J. J. Verheijen, S. P. Heenan, L. F. J. van de Laak, H. B. M. Koopmans and S. M. van Ruth, J. Food Compos. Anal., 2013, 31, 129–136 CrossRef CAS.
- N. Dilsiz, A. Olcucu and M. Atas, Cell Biochem. Funct., 2000, 18, 259–262 CrossRef CAS PubMed.
- M. S. Epstein and T. A. Rush, Appl. Spectrosc., 1991, 45, 1568–1570 CrossRef CAS.
- E. S. Chaves, T. D. Saint'Pierre, E. J. dos Santos, L. Tormen, V. Bascunana and A. J. Curtius, J. Braz. Chem. Soc., 2008, 19, 856–861 CrossRef CAS.
- G. Ploegaerts, C. Desmet and M. Van krieken, J. Food Compos. Anal., 2016, 45, 66–72 CrossRef CAS.
- R. R. Overman and A. K. Davis, J. Biol. Chem., 1947, 168, 641–649 CAS.
- A. Krejcova, T. Cernohorsky and E. Curdova, J. Anal. At. Spectrom., 2001, 16, 1002–1005 RSC.
- B. Budic, J. Anal. At. Spectrom., 1998, 13, 869–874 RSC.
- D. E. Goodney, J. Chem. Educ., 1982, 59, 875 CrossRef CAS.
- G. N. Havre, Anal. Chim. Acta, 1961, 25, 557–566 CrossRef CAS.
- M. R. Tripkovic and I. D. Holclajtner-Antunovic, J. Anal. At. Spectrom., 1993, 8, 349–357 RSC.
- C. Xie, X. M. Wen, Y. T. Jia and S. P. Sun, Spectrosc. Spectral Anal., 2001, 21, 366–369 CAS.
- A. S. Al-Ammar and R. M. Barnes, Spectrochim. Acta, Part B, 1998, 53, 1583–1593 CrossRef.
- C. N. LaFratta, S. Jain, I. Pelse, O. Simoska and K. Elvy, J. Chem. Educ., 2013, 90, 372–375 CrossRef CAS.
- B. Néel, G. A. Crespo, D. Perret, T. Cherubini and E. Bakker, J. Chem. Educ., 2014, 91, 1655–1660 CrossRef.
- C. S. Seney, K. V. Sinclair, R. M. Bright, P. O. Momoh and A. D. Bozeman, J. Chem. Educ., 2005, 82, 1826–1829 CrossRef CAS.
- A. Y. Nazarenko, Spectrosc. Lett., 2004, 37, 235–243 CrossRef CAS.
- W. Silva Lyra, V. B. dos Santos, A. G. G. Dionízio, V. L. Martins, L. F. Almeida, E. Nóbrega Gaião, P. H. G. D. Diniz, E. C. Silva and M. C. U. Araújo, Talanta, 2009, 77, 1584–1589 CrossRef CAS PubMed.
- S. Groom, G. Schaldach, M. Ulmer, P. Walzel and H. Berndt, J. Anal. At. Spectrom., 2005, 20, 169–175 RSC.
- G. D. Smith, C. L. Sanford and B. T. Jones, J. Chem. Educ., 1995, 72, 438–440 CrossRef CAS.
- E. P. Moraes, N. S. A. da Silva, C. d. L. M. de Morais, L. S. d. Neves and K. M. G. d. Lima, J. Chem. Educ., 2014, 91, 1958–1960 CrossRef CAS.
- C. Ruckebusch and L. Blanchet, Anal. Chim. Acta, 2013, 765, 28–36 CrossRef CAS PubMed.
- A. A. Sidorova and A. V. Grigoriev, J. Anal. Chem., 2012, 67, 478–485 CrossRef CAS.
- G. W. Jones, B. Lewis, J. B. Friauf and G. S. J. Perrott, J. Am. Chem. Soc., 1931, 53, 869–883 CrossRef CAS.
- A. de Juan and R. Tauler, Anal. Chim. Acta, 2003, 500, 195–210 CrossRef CAS.
-
G. H. Golub and C. F. V. Loan, Matrix Computations, John Hopkins University Press, Baltimore,US, 3rd edn, 1996 Search PubMed.
-
C. T. J. Alkemade, Ph.D. Dissertation, University of Utrecht, Utrecht, The Netherlands, 1954.
Footnote |
† Electronic supplementary information (ESI) available. See DOI: 10.1039/c7ay01118k |
|
This journal is © The Royal Society of Chemistry 2017 |