DOI:
10.1039/C7AN00107J
(Paper)
Analyst, 2017,
142, 1891-1899
LILY-lipidome isotope labeling of yeast: in vivo synthesis of 13C labeled reference lipids for quantification by mass spectrometry†
Received
18th January 2017
, Accepted 20th April 2017
First published on 25th April 2017
Abstract
Quantification is an essential task in comprehensive lipidomics studies challenged by the high number of lipids, their chemical diversity and their dynamic range of the lipidome. In this work, we introduce lipidome isotope labeling of yeast (LILY) in order to produce (non-radioactive) isotopically labeled eukaryotic lipid standards in yeast for normalization and quantification in mass spectrometric assays. More specifically, LILY is a fast and efficient in vivo labeling strategy in Pichia pastoris for the production of 13C labeled lipid library further paving the way to comprehensive compound-specific internal standardization in quantitative mass spectrometry based assays. More than 200 lipid species (from PA, PC, PE, PG, PI, PS, LysoGP, CL, DAG, TAG, DMPE, Cer, HexCer, IPC, MIPC) were obtained from yeast extracts with an excellent 13C enrichment >99.5%, as determined by complementary high resolution mass spectrometry based shotgun and high resolution LC-MS/MS analysis. In a first proof of principle study we tested the relative and absolute quantification capabilities of the 13C enriched lipids obtained by LILY using a parallel reaction monitoring based LC-MS approach. In relative quantification it could be shown that compound specific internal standardization was essential for the accuracy extending the linear dynamic range to four orders of magnitude. Excellent analytical figures of merit were observed for absolute quantification for a selected panel of 5 investigated glycerophospholipids (e.g. LOQs around 5 fmol absolute; typical concentrations ranging between 1 to 10 nmol per 108 yeast cell starting material; RSDs <10% (N = 4)).
Introduction
The number of entries in the human metabolome database saw a tremendous increase during the last decade. As a matter of fact, this was primarily due to the high number of newly discovered lipid structures (3800 lipids were added to the database from 2006 to 2009) so that more than 60% of all metabolites within the human metabolome database are annotated lipids.3 Currently, the Lipid Maps Structure Database covers over 40
000 unique lipid structures, steadily growing and highlighting the important biological role of lipids4,5 (http://www.lipidmaps.org/). In analogy to other “omics” disciplines, quantitative information is regarded as essential in lipidomics studies, however methods are not yet routine. In fact, quantification of lipids is highly challenging (regardless whether absolute or relative quantification is addressed) due to (i) the chemical diversity and amphiphilic nature of lipids,6 (ii) occurrence of many lipid isomers and isobars7,8 (iii) high dynamic range of lipid abundance,1,2 (iv) limited stability due to potential oxidation2,9,10 and (v) the lack of suitable lipid standards.2,11 Mass spectrometry has evolved as a state of the art technique for quantitative lipidomics as it provides lipid identification by accurate mass, structural assessment by fragmentation pattern and the possibility of parallel monitoring of a wide range of lipids with high resolution and high mass accuracy.7 The development of standardization strategies remains a topical research theme in the field. Internal standards proved to be valuable tools in both relative as well as absolute quantification. Ideally, compound-specific internal standards in the form of stable-isotope labeled versions of the lipid species of interest are implemented as they provide the same chemical behavior and can be simultaneously treated under identical experimental conditions.12–14 In the best case the concentration of the isotopically labeled standard would be known, a premise that is not fulfilled in most omics-type applications using isotopically labeled standards.15–20 The problem is met by adding isotopically labeled standard in the same amount to samples and standards (absolute quantification) or to different sample groups (relative quantification). Isotopically labeled lipids have to be individually synthesized with the obvious drawback of high costs and elaborate production workflows due to lipid complexity. Up to now these limitations made compound-specific isotope dilution mass spectrometry in lipidomics impractical.2,21
State of the art quantification is carried out by class-specific quantification using non-endogenous lipid standards.22–27 As a prerequisite, the applied class-specific lipid standards have to be unambiguously absent in the sample of interest and display a behavior as similar as possible to the compound of interest in terms of extraction and ionization efficiencies.
In this work, we introduce lipidome isotope labeling of yeast (LILY) for internal standardization in quantitative lipidomics by high resolution mass spectrometry. To tackle the challenge of individual chemical synthesis of isotopically labeled lipid standards, we exploit Pichia pastoris- a well-known cell factory in biotechnology19,28–31 -to produce an uniformly 13C labeled yeast lipidome. Pichia pastoris is an ideal host system as (i) it is an eukaryotic microorganism possessing and generating similar metabolite (and lipid) profiles to mammalian cells, (ii) it can be fermented to very high cell densities increasing product yields and (iii) has a very stable cell wall enabling longer storage periods. In this work, we demonstrate that a complete isotope labeling can be achieved and that the generated standards are suited for relative and absolute quantification in lipidomics studies.
Material and methods
Lipid standards
The lipid standards phosphatidic acid (PA) 16:0/18:1, phosphatidylcholine (PC) 16:0/18:1, phosphatidylethanolamine (PE) 16:0/18:2, phosphatidylinositol (PI) 18:1/16:0, phosphatidylserine (PS) 16:0/18:1 were purchased from Avanti Polar Lipids, Inc. (Alabaster, Alabama, USA). Further reagent and material details are provided in the ESI.†
Fermentation
Yeast fermentation was conducted according to a previously established protocol.19 Briefly, Pichia pastoris cells were grown on 99% 13C6-glucose (Sigma Aldrich, Steinheim, Germany) as sole carbon source to produce an uniformly 13C labeled yeast lipidome. Labeling efficiency was improved by cultivating yeast precultures in shaker flasks prior to the actual inoculation of the fermentor. Fed-batch fermentation was performed for 72 h utilizing a BioFlo 310 Fermenter (New Brunswick™). Moreover, a yeast fermentation of cells grown on natural glucose (Sigma Aldrich, Steinheim, Germany) was conducted under the same fed-batch conditions to obtain a non-labeled yeast lipidome. Cell counting was performed using a cell counting chamber and a transmission microscope (120× magnification) and revealed a cell density of 4 × 1011 cells per liter. Finally, the fermentation broth was transferred into 50 mL falcon tubes and centrifuged for 5 min at 4000 rcf at 4 °C. The supernatant was discarded and the cell pellet was stored at −80 °C until further processing.
Lipid extraction
Harvested yeast cells (corresponding to 4 × 108 cells aliquots) were opened by mechanical disruption facilitating the TissueLyser II (Qiagen, Hilden, Germany) with 3 × 7 min and 30 s min−1 frequency. Yeast lipids were extracted from cell lysate aliquots using the Folch protocol32and blank extractions (N = 3) were carried out with the same equipment. The blank extraction was considered for all identified and quantified lipids presented in the manuscript. For the relative quantification experiment pooled 13C and natural Pichia pastoris aliquots were mixed (N = 4) in defined ratios (12C/13C: 0.02, 0.05, 0.10, 0.14, 0.20, 0.33, 0.5, 1, 2, 3, 5, 7). For the absolute quantification of lipids, a calibration curve was performed by adding the indicated amounts (2 fmol to 20 pmol) of 5 glycerophospholipid standard mixture to the 13C yeast extract matrix. Further, non-labeled yeast extract aliquots were spiked with 13C yeast extract (N = 4) for internal standardization prior to (N = 4) or after Folch extraction (N = 4). Protein pellets of the interphase and the upper methanol phase were collected and protein concentration was determined applying the BCA assay. The lipid containing chloroform phase was dried under nitrogen flow and stored at −80 °C until analysis. Additional sample preparation details are provided in the ESI.†
Lipid identification and quantification
Lipid screening was performed by shotgun analysis infusing the samples via robotic nanoflow ion source TriVersa NanoMate (Advion BioSciences, Ithaca NY, USA) into a Q Exactive Plus instrument (Thermo Fisher Scientific, Bremen, Germany) followed by LipidXplorer analysis.27,33 Comprehensive lipid identification and RP-LC-MS/MS for absolute quantification was conducted using an UltiMate 3000 LC-system (Thermo Fischer Scientific, Darmstadt, Germany) with an Ascentis Express C18 column (150 mm × 2.1 mm, 2.7 μm, Supelco),34 while for the relative quantification experiment a Vanquish UPLC system (Thermo Fischer Scientific, Darmstadt, Germany) was used with an Acquity C18 column (150 mm × 2.1 mm, 1.8 μm, Waters). The LC-system was coupled to a Q Exactive Plus or a Q Exactive HF (Thermo Fisher Scientific, Bremen, Germany) instrument for MS and MS/MS analysis. Instrument parameters, data evaluation and quantification procedures are detailed in the ESI.† The labeling degree was determined by Isopro (Version 3.1).35,36
Results and discussion
Already one decade ago, Pichia pastoris13C labeled glycerophospholipids were applied to lipidomics in direct infusion experiments.37 Here we expand this strategy to the whole yeast lipidome and present the first high resolution, high mass accuracy library of 13C lipid standards by lipidome isotope labeling of yeast (LILY). Pichia pastoris is grown on 13C glucose leading to a mass increment of 1.003 amu per carbon present in the lipid backbone. A significant mass shift for the whole yeast lipidome is introduced i.e.: for PC 34:2 (sum formula: C42H80NO8P) a mass shift of 42.1407 corresponding to 42 13C is observed. Quantification based on in vivo stable isotope labeling techniques were extremely successful in other “omics” disciplines38i.e. proteomics15,18 or metabolomics19,20 offering identical chemical properties and chromatographic behavior (in contrast to deuterated isotopologues39). Despite this success, up to date, to the best of our knowledge, their application in the field of lipidomics is widely unexplored.
Lipidome isotope labeling of yeast (LILY)
Pichia pastoris is an efficient host with high expression levels growing to very high cell densities.28 The yeast is a preferable organism for isotopic labeling in vivo due to its eukaryotic properties, short generation times and the ability to grow on non-complex media such as glucose as sole carbon source.29,40,41 In this study, 13C glucose was selected as carbon source. In fed-batch fermentations, controlled reactor conditions are possible and the substrate is continuously added allowing a high substrate conversion. Fed-batch fermentation with 13C labeled glucose was determined to be ideal for uniformly labeling of the primary metabolome.19,41 Hence, in this work analogous fermentation conditions were applied to produce yeast extracts containing 13C labeled lipids. After fermentation, the cells were harvested and the cell wall mechanically disrupted with glass beads.42,43 Folch extraction32,44 was performed to collect the 13C labeled yeast lipidome in the organic phase. In summary, the workflow introducing LILY encompassed (i) Pichia pastoris fed-batch fermentation using 13C glucose as carbon source followed by cell harvesting, (ii) mechanical disruption with glass beads and (iii) Folch extraction to collect the lipids in the chloroform phase followed by (iv) quantitative lipidomics experiments for 13C lipid characterization(Fig. 1).
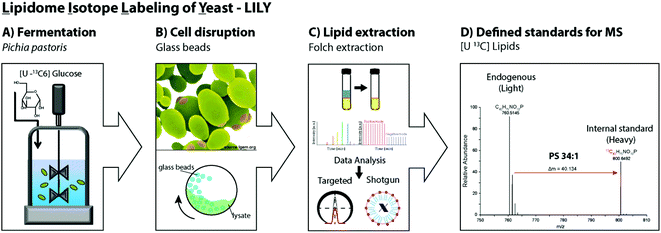 |
| Fig. 1 Schematic workflow of the LILY approach applied in this study. (A) Fed-batch fermentation of Pichia pastoris for 70 h using uniformly 13C labeled glucose. (B) Cell harvesting, yeast cells are mechanically disrupted with glass beads (3 cycles). (C) Lipid extraction using Folch chloroform/methanol (2 : 1) extraction. (D) The uniformly 13C labeled lipid standard is then utilized as internal standard for MS experiments as exemplary shown for the isotopologue pattern of the glycerophospholipid PS 34:1, where a mass shift of 40.134 is introduced by the presented labeling approach. | |
Characterization of 13C lipidome derived from Pichia pastoris
First, the Pichia pastoris lipidome was screened by shotgun analysis followed by a complementary characterization using a reversed-phase LC MS/MS high resolution approach. Selectivity provided by chromatographic dimension was essential for both the assessment of the lipid profile and the enrichment degree of the 13C lipids. Approximately 200 lipids were identified based on accurate mass and fragmentation pattern. Identification was performed via LipidXplorer and manual assignment and led to a lipid list comprising of glycerophospholipids (PA, PE, PC, PG, PI, PS, LysoGP, CL, DMPE), glycerolipids (DAG, TAG) and sphingolipids (Cer, HexCer, IPC, MIPC) in accordance to other Pichia pastoris studies.45–47 In particular, we assigned a 13C fully labeled lipid, by accurate masses of the fully labeled 13C isotopologue of high resolution LC-MS/MS data corresponding to the previously found accurate monoisotopic masses of the non-labeled endogenous lipid (M + 0 isotopologue). As expected for an ideal internal standard, we observed matching retention times of natural abundant lipids and 13C isotopically enriched lipids in Pichia pastoris for all the analyzed compounds (ESI Fig. S1†).
Comparing MS1 spectra obtained from stable-isotope 13C labeled (Fig. 2A) and natural Pichia pastoris (Fig. 2B) fermentations, consistent mass shifted lipid profiles were observed due to the mass increment of 1.003 per carbon present in the lipid backbone in 13C enriched lipids. These mass shifts can also be observed in the MS2 fragmentation pattern as exemplary shown for the glycerophospholipid PA 34:2 (Fig. 2A + B). Moreover, unlabeled and 13C labeled lipid species revealed comparable fragmentation behavior (ESI Fig. S3†). Summed MS1 spectra (from 10.5–12.5 min) display that glycerophospholipids are highly abundant in Pichia pastoris (Fig. 2). Overall, the highest abundant lipids observed in both negative and positive mode (normalized target level: 106–107) in reversed-phase based high resolution MS measurements were glycerophospholipids (PA, PC, PE, PG, PI, PS), with combined fatty acid chain length and unsaturation of 34:2 and 36:2, 36:3, 36:4 as the most abundant(Fig. 2). LysoGP, CL, DAG, TAG, DMPE, Cer, HexCer, IPC and MIPC were present with significant intensities (normalized target level: 103–107). In total, a library of 217 fully 13C labeled lipid species from main lipid classes including glycerophospholipids, glycerolipids and sphingolipids were identified with high confidence due to characteristic MS2 lipid fragmentation, matching retention times and accurate masses (Table 1, ESI Fig. S1,† supporting list S6). Lipid profiles and the relative distribution of the lipid species are consistent with data of Saccharomyces cerevisiae and recently published work in Pichia pastoris.22,45–48 The identification of HexCer is specific for Pichia pastoris compared to Saccharomyces cerevisiae as HexCer biosynthesis is absent in Saccharomyces cerevisiae.49,50 The simultaneous presence of HexCer and Cer in Pichia pastoris provides a more complex model system for mimicking eukaryotic lipid biosynthesis compared to Saccharomyces cerevisiae.50 Notably, lipids comprised of polyunsaturated fatty acids are normally not produced by the yeast.51 Nevertheless, it was shown that the core enzymes involved in lipid metabolism play a major role in cancer cell proliferation, and most of these enzymes are conserved in yeast, highlighting the potential of yeast as interesting model system.52 Moreover, Pichia pastoris is optimally suited for genetic engineering even easier to manipulate than the standard yeast S. cerevisiae due to enhanced vector integration.53 An impressive example is the engineering of the whole glycosylation pathway to mammalian-like hybrid-type N-glycans in Pichia pastoris in order to produce humanized, uniform glycoproteins.54 In our opinion, biotechnological lipid engineering in yeast is a very promising approach to produce a comprehensive library of labeled lipid standards.
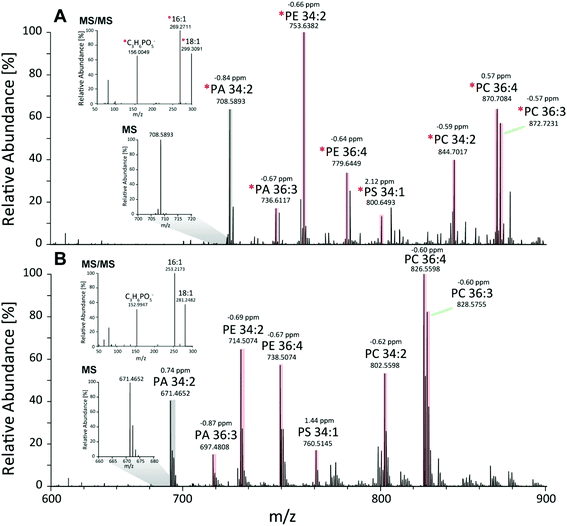 |
| Fig. 2 Comparison of full scan high resolution MS and MS/MS spectra of unlabeled and labeled lipids. Here exemplary RP-LC-MS and MS/MS mass spectra of lipid profiles obtained from natural and 13C isotopically enriched Pichia pastoris fermentations are presented (A) MS1 profile of isotopically enriched yeast recorded in the negative mode from 10.5–12.5 min within the mass range of 600–900 m/z is presented, insets: MS1 isotopic pattern of 13C enriched PA and MS2 fragments of labeled PA (16:1–18:1); (B) MS1 profile non-labeled Pichia pastoris recorded in the negative mode from 10.5–12.5 min within the mass range of 600–900 m/z, insets: MS1 isotopic pattern of natural PA, MS2 fragments of natural PA (16:1–18:1). | |
Table 1 List of identified lipids in Pichia pastoris. The list includes (i) lipid class assignment, (ii) information on positive or negative ion mode in mass spectrometry, (iii) number of identifications (IDs) per lipid class (iv) exemplary lipids from all classes with their (v) calculated natural monoisotopic lipid mass, (vi) 13C analog monoisotopic lipid mass, (vii) mass accuracy comparing observed and calculated 13C monoisotopic mass and (viii) 13C enrichment
Lipid classes |
MS |
Nr. IDs |
Class |
Massmono calc. [m/z] |
13CMassmono calc. [m/z] |
Mass accuracy [ppm] |
13C enrichment [%] |
Example |
Phosphatidic acid |
− |
19 |
PA [16:0–18:1] |
673.4808 |
710.6050 |
−1.3 |
99.8 |
C37H71O8P |
Phosphatidylethanolamine |
− |
21 |
PE [16:0–18:1] |
716.5230 |
755.6539 |
−1.5 |
99.8 |
C39H76NO8P |
Phosphatidylcholine |
− |
28 |
PC [16:0–18:1] |
804.5755 |
846.7164 |
−2.3 |
99.8 |
C42H82NO8P |
Phosphatidylglycerol |
− |
11 |
PG [16:0–18:1] |
747.5176 |
787.6518 |
−1.1 |
99.8 |
C40H77O10P |
Phosphatidylinositol |
− |
15 |
PI [16:0–18:1] |
835.5355 |
878.6807 |
1.9 |
99.7 |
C43H81O13P |
Phosphatidylserine |
− |
10 |
PS [16:0–18:1] |
760.5145 |
800.6493 |
1.5 |
99.8 |
C40H76NO10P |
Lysophospholipids |
− |
15 |
LPE [16:1] |
450.2621 |
471.3325 |
−1.4 |
99.9 |
C21H42NO7P |
Cardiolipin |
− |
10 |
CL [18:2–18:2–18:2–18:2] |
1447.9649 |
1529.2367 |
−1.8 |
99.8 |
C80H140O17P2 |
Dimethyl-phosphatidylethanolamine |
+ |
7 |
DMPE [36:1] |
746.5704 |
787.7082 |
1.5 |
99.7 |
C41H80NO8P |
Diacylglycerol |
+ |
23 |
DAG [16:0–18:1] |
612.5567 |
649.6808 |
0.4 |
99.8 |
C37H80O5 |
Triacylglycerol |
+ |
26 |
TAG [18:0–16:1–18:1] |
874.7858 |
929.9703 |
−2.3 |
99.5 |
C55H100O6 |
Ceramide |
+ |
16 |
Cer 18:0,2/18:0,0 |
568.5669 |
604.6877 |
0.7 |
99.8 |
C36H73O3N |
Hexosyl ceramide |
+ |
3 |
HexCer 18:2,2/18:0,1 |
742.5827 |
784.7238 |
0.3 |
99.8 |
C43H81O9 |
Inositolphosphorylceramide |
− |
7 |
IPC [42:0,4] |
924.6546 |
972.8157 |
−2.4 |
99.8 |
C48H96NO13P |
Mannosylinositolphosphorylceramide |
− |
4 |
MIPC [44:0,4] |
1114.7388 |
1170.9267 |
−0.7 |
99.8 |
C56H110NO18P |
13C labeling degree
High 13C labeling efficiency in the fermentation was achieved by (i) the use of a sole carbon source in a highly labeled form, (ii) the relatively low generation time for cell doubling in Pichia pastoris (∼2 h),28 (iii) a high substrate turnover over the 70 h of fed-batch fermentation and (iv) the use of a closed reactor system preventing contamination during fermentation. Accurate and exact 13C monoisotopic masses were in excellent agreement (within ±3 ppm) for all identified lipids using high resolution mass spectrometry (Table 1). The unlabeled M + 0 isotopologues (corresponding to the isotopologues containing only 12C) were not detected for any lipid species present in the yeast based spike material. In order to assess the 13C labeling degree of the identified lipids, however, all isotopologues and their relative abundances had to be considered. Assuming different 13C enrichment degrees, theoretical isotopologue distributions were calculated for the list of identified lipids (Table 1, supporting list S6) by Isopro.35,36 The calculated distributions were fitted to the experimental data revealing an excellent 13C carbon labeling degree of 99.5–99.8% (ESI Fig. S2†). E.g. for the glycerophospholipid PS 34:2 (H73C40N1O10P1) an 13C enrichment of 99.8% was determined, as revealed by the abundance of 87.4% of the uniformly labeled 13C monoisotopic isotopologue [M + 40] and 7% for the [M + 39] isotopologue (containing 39 13C and 1 12C isotope). The remaining isotopologue fraction of 5.6% was uniformly labeled regarding 13C but contained 15N, 17O or 18O. Due to the high mass shift introduced by the 13C labeling, no spectral overlays with unlabeled species was observed. With this high labeling efficiency our results highlight that Pichia pastoris is an ideal source for the production of uniformly 13C labeled internal standards for quantitative lipidomics.
Relative quantification by 13C-enriched lipids
Compound-specific internal standardization as provided by isotopically labeled internal standards proofed to be beneficial if not a prerequisite for accuracy of quantification in many different omics type applications.15,18,40,55,56 This is the case in both essential quantification tasks, i.e. relative and absolute quantification basically by improving repeatability, extending the linear dynamic range and minimizing the trueness bias. In lipidomics this concept is rather emerging and only rarely applied.
In a first step, we investigated the potential of LILY for relative quantification in lipidomics. The 13C yeast based lipid extract was used as compound-specific scalar addressing fold changes in a proof of principle study on Pichia pastoris. In order to assess analytical figures of merit, such as trueness bias and the repeatability, a dilutions series of (non-labeled endogenous) Pichia pastoris lipid extracts served for preparation of samples with known fold changes. Pooled yeast extract corresponding to approximately 107 cells and phospholipid concentrations in the low μM range were used as reference points and diluted in varying concentrations relative to this reference points. To all samples constant amounts of LILY derived internal standard was added (corresponding to a 1
:
1 ratio compared to the reference points) compensating for matrix effects due to dilution. The dilution series covered approximately 4 orders of magnitude. Since typically observed fold changes in yeast cells are rather low (in the range of 1.5–3)57 we especially focused on small fold changes compared to the reference point (fold changes compared to the reference points were 0.02, 0.05, 0.10, 0.14, 0.20, 0.33, 0.50, 2, 3, 5, 7 corresponding to a dilution factor down to 50 up 7 times concentration compared to the reference points). For a panel of 20 lipids, trueness and measurement precision (N = 4 technical replicates) was studied with and without compound-specific normalization using the isotopically labeled reference lipids (Fig. 3). More specifically, the fold change recoveries of 20 compounds from different lipid classes typically present in yeast (CL, IPC, LPE, PA, PC, PE, PG, PI, PS, MIPC, HexCer, DAG) were monitored by high resolution RP-LC-MS using PRM (CL 70:6, CL 72:6, IPC 42:0,4, LPE 18:0, PA 34:1, PA 36:2, PC 34:2, PC 36:2, PC 38:2, PE 34:1, PE 34:2, PG 34:1, PI 34:1, PI 34:2, PS 34:1, PS 34:2, MIPC 42:0,4, HexCer 35:2, DAG 34:3, DAG 36:2). Fig. 3 shows the resulting plots of fold changes obtained with and without normalization by 13C internal standards. Without internal standardization overall poor precision (RSD ranging from 5–110%) and significant trueness biases (fold change recoveries of 20–95%) were observed (Table in Fig. 3A). For small fold changes of factor 2 and 3, excellent RSDs <10% were observed (N = 4 technical replicates), even without internal standardization (Fig. 3A, supporting list S6). However the trueness of fold change quantification was compromised, average recoveries of as low as 60% were observed for 20 lipids (Table in Fig. 3A, supporting list S6). These experiments clearly show that the accuracy of relative quantification without internal standardization is limited even in ideal cases where the fold change determination was precise and the concentration change only small and hence within the linear dynamic range. By internal standardization applying 13C reference lipids, the quantitative method could be significantly improved in this regard. For all 20 investigated lipids no significant trueness bias was observed over the established concentration differences of 4 orders of magnitude (Fig. 3B). All experiments were carried out using a targeted PRM method based on MS2 (MS2 resolution: 15
000) level quantification (supporting list S6). Comparison of this MS approach to classical high resolution Full-MS (MS1 resolution: 120
000) quantification showed no significant difference (ESI Fig. S4†). For example, PI 34:1 revealed comparable fold change recoveries and precisions assessed by PRM or Full-MS quantification without (PRM- recovery 40–160%, RSD: 2–13%, Full-MS- recovery: 33–140%, RSD: 1–10%) and with 13C internal standardization (PRM- recovery 90–102%, RSD 1–8%, Full-MS- recovery: 100–111%, RSD: 1–5%). Application of normalization by isotopically labeled lipids can successfully correct for all suppression effects as the target fold changes were met (Table in Fig. 3B) highlighting another advantage of internal standardization.
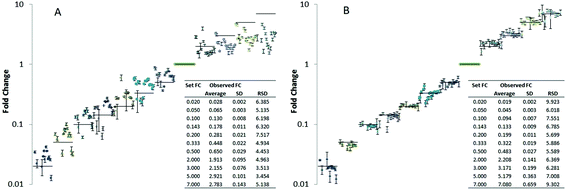 |
| Fig. 3 Trueness of observed fold changes in a dilution experiment on Pichia pastoris lipid extract shown for 20 lipids analyzed by a RP-LC-MS PRM method. Black bars indicate the true fold change set by the relative spike concentrations, table inserts reflect the average across all lipids investigated. (A) Fold change (FC) calculated based on raw peak areas (no normalization), (B) fold change (FC) after lipid specific peak area normalization using the respective 13C labeled lipid analog. | |
Summarizing, the experiments exemplify the pitfalls of relative quantification in established label-free lipidomics approaches.58,59 Matrix effects, ionization suppression and special features of lipids such as concentration dependent micelle formation2 demand internal standardization (regardless whether relative and absolute quantification is addressed). Currently, state of the art quantification comprises class-specific internal standardization, i.e. applying one or two individually synthesized internal standards per lipid class.7,21,22 However, this approach is hampered when using reversed-phase LC-MS to separate different lipid species within one lipid class and therefore, calling for compound-specific quantification. Alternatively, head-group selective HILIC separations60 or direct infusion shotgun approaches7,11,22 use class-specific standardization to overcome this limitation as coelution of all species within one class is obtained. However, for sophisticated lipid sample preparation workflows such as multi-step extractions or additional preconcentration steps, compound-specific isotopologue dilution analysis will remain the method of choice. Through the here proposed LILY strategy more than 200 different lipids become available for compound-specific internal standardization.
Compound-specific absolute quantification of lipids by mass spectrometry
The commonly accepted routine for absolute quantification in lipidomics relies on non-endogenous standards. In this work, we quantified a panel of glycerophospholipids in Pichia pastoris by reversed phase LC high resolution MS using external calibration (by endogenous standards) with internal standardization by LILY derived 13C lipids in analogy to established targeted metabolomics strategies.19,55,56 Out of the 136 identified glycerophospholipids, we selected lipids from five different classes (PA 34:1, PC 34:1, PE 34:2, PI 34:1 and PS 34:1). Pichia pastoris was spiked with 13C yeast extract before lipid extraction (N = 4 biological replicates) and after extraction (N = 4 biological replicates). Matrix-matched calibration was linear (R2 > 0.9987) over four orders of magnitude with the lowest calibration point at 10 nM (corresponding to 0.02 absolute on column) with increased linear dynamic range upon compound-specific normalization (Fig. S5†). Related studies show a comparable linear dynamic range for high resolution mass spectrometric determination of metabolites (depending on the matrix and sample complexity).61,62 A limit of detection (calculated from 3 σ of low-level standards below the linear working range63) of approximately 1 fmol on column (∼for the analyzed glycerophospholipids PA 34:1–0.3 nM; PC 34:1–0.5 nM; PE 34:2–1.1 nM; PI 34:1–1.1 nM; PS 34:1–0.2 nM) was determined leading to limits of quantification around 3 fmol (10 σ). Considering the abundance of quantified species and lipid distribution as well as the number of lipids detected (>200) our data are consistent with data previously published on yeast.2,22
Table 2 summarizes the obtained concentrations (N = 4 individually prepared samples) normalized to the protein content and the cell number of Pichia pastoris. Excellent extraction recoveries as well as procedural repeatabilities were observed. The experiments show the validity of the proposed LILY approach for internal standardization in absolute quantification of lipids.
Table 2 LC-MS quantification of 5 different glycerophospholipids in Pichia pastoris (N = 4 independently prepared samples) calculated based on protein content or starting cell number. Residual standard deviations of the biological replicates as well as recovery of the internal standard added prior and after extraction are shown (Table 2)
Pichia pastoris (N = 4) |
nmol mg−1 protein |
nmol/108 cells |
RSD, % |
Recovery |
PA 34:1 |
15.5 ± 1.4 |
1.16 ± 0.10 |
8.8 |
102 ± 9 |
PC 34:1 |
13.0 ± 1.1 |
0.98 ± 0.09 |
8.7 |
101 ± 9 |
PE 34:2 |
66.2 ± 5.9 |
4.97 ± 0.44 |
8.9 |
105 ± 9 |
PI 34:1 |
12.2 ± 1.1 |
0.92 ± 0.08 |
8.8 |
104 ± 9 |
PS 34:1 |
19.4 ± 1.9 |
1.45 ± 0.14 |
9.6 |
109 ± 11 |
Conclusion
LILY is a workflow to produce (non-radioactive) isotopically labeled eukaryotic lipid standards in yeast for normalization and quantification in mass spectrometric assays. In this work, we introduced a 13C lipid library to enable compound-specific relative and absolute quantification strategies in lipidomics. Pichia pastoris grown on 13C glucose led to the characterization of over 200 uniformly 13C-labeled lipid species achieving a 13C labeling degree of 99.5–99.8%. As proof of concept, accurate determination (average recovery of 100%) of fold changes for 20 lipids present in Pichia pastoris was possible over four orders of magnitude applying compound-specific relative quantification with 13C labeled lipids. Moreover, five glycerophospholipids were absolutely quantified and revealed high precisions (RSDs < 10%) with excellent LODs and LOQs in the fmol range using high resolution LC-MS. Hence, LILY produced lipids can be used for compound-specific relative quantification or for compound-specific normalization in absolute quantification strategies. The presented approach is a cost efficient alternative to chemical synthesis of single isotopically enriched lipid standards as a set of biological relevant eukaryotic lipid species is simultaneously produced. Follow-up studies will demonstrate the quantification potential of isotopically labeled yeast extract for different biological matrices, and focus on upscaling the approach to produce and quantitatively assess high amounts of labeled lipid standard.
Author contributions
The manuscript was written through contributions of all authors. All authors have given approval to the final version of the manuscript.
Conflict of interest
The authors declare no competing financial interest.
Abbreviations
PA | Phosphatidic acid |
PE | Phosphatidylethanolamine |
PC | Phosphatidylcholine |
PG | Phosphatidylglycerol |
PI | Phosphatidylinositol |
PS | Phosphatidylserine |
LysoGPs | Lysophospholipid |
CL | Cardiolipin |
DAG | Diacylglycerol |
TAG | Triacylglycerol |
DMPE | Dimethyl-phosphatidylethanolamine |
Cer | Ceramide |
HexCer | Hexosyl ceramide |
IPC | Inositolphosphorylceramide |
MIPC | Mannosylinositolphosphorylceramide |
PRM | Parallel reaction monitoring |
Acknowledgements
Financial support is derived from the Ministry for Innovation, Science and Research of the Federal State of North Rhine-Westphalia, and by the BMBF. This work is further supported by the Vienna Metabolomics Center (VIME; http://metabolomics.univie.ac.at/), the research platform Chemistry Meets Microbiology and the mass spectrometry center of the University of Vienna. We want to thank all members of the Lipidomics (ISAS) and environmental analysis (University of Vienna) group for continuous support, especially Michaela Schwaiger and Yasin El Abiead for their help with graphical data evaluation.
References
- O. Quehenberger, A. M. Armando, A. H. Brown, S. B. Milne, D. S. Myers, A. H. Merrill, S. Bandyopadhyay, K. N. Jones, S. Kelly, R. L. Shaner, C. M. Sullards, E. Wang, R. C. Murphy, R. M. Barkley, T. J. Leiker, C. R. H. Raetz, Z. Guan, G. M. Laird, D. A. Six, D. W. Russell, J. G. McDonald, S. Subramaniam, E. Fahy and E. A. Dennis, J. Lipid Res., 2010, 51, 3299–3305 CrossRef CAS PubMed.
-
X. Han, Lipidomics: Comprehensive mass spectrometry of lipids, John Wiley & Sons, Hoboken, New Jersey, 2016 Search PubMed.
- D. S. Wishart, C. Knox, A. C. Guo, R. Eisner, N. Young, B. Gautam, D. D. Hau, N. Psychogios, E. Dong, S. Bouatra, R. Mandal, I. Sinelnikov, J. Xia, L. Jia, J. A. Cruz, E. Lim, C. A. Sobsey, S. Shrivastava, P. Huang, P. Liu, L. Fang, J. Peng, R. Fradette, D. Cheng, D. Tzur, M. Clements, A. Lewis, A. de souza, A. Zuniga, M. Dawe, Y. Xiong, D. Clive, R. Greiner, A. Nazyrova, R. Shaykhutdinov, L. Li, H. J. Vogel and I. Forsythei, Nucleic Acids Res., 2009, 37, 603–610 CrossRef PubMed.
- M. Sud, E. Fahy, D. Cotter, A. Brown, E. A. Dennis, C. K. Glass, A. H. Merrill, R. C. Murphy, C. R. H. Raetz, D. W. Russell and S. Subramaniam, Nucleic Acids Res., 2007, 35, 527–532 CrossRef PubMed.
- A. Mullard, Nat. Rev. Mol. Cell Biol., 2008, 9, 92–92 CrossRef CAS.
- A. Shevchenko and K. Simons, Nat. Rev. Mol. Cell Biol., 2010, 11, 593–598 CrossRef CAS PubMed.
- D. Schwudke, K. Schuhmann, R. Herzog, S. Bornstein and A. Shevchenko, Cold Spring Harbor Perspect. Biol., 2011, 3, 1–13 Search PubMed.
- M. Li, L. Yang, Y. Bai and H. Liu, Anal. Chem., 2013, 161–175 Search PubMed.
- B. Fuchs, K. Bresler and J. Schiller, Chem. Phys. Lipids, 2011, 164, 782–795 CrossRef CAS PubMed.
- G. Astarita, A. C. Kendall, E. A. Dennis and A. Nicolaou, Biochim. Biophys. Acta, Mol. Cell Biol. Lipids, 2015, 1851, 456–468 CrossRef CAS PubMed.
- X. Han and R. W. Gross, Mass Spectrom. Rev., 2005, 24, 367–412 CrossRef CAS PubMed.
- S. Dzeletovic, O. Breuer, E. Lund and U. Diczfalusy, Anal. Biochem., 1195, 225, 73–80 CrossRef PubMed.
- D. L. Baker, D. M. Desiderio, D. D. Miller, B. Tolley and G. J. Tigyi, Anal. Biochem., 2001, 292, 287–295 CrossRef CAS PubMed.
- H. Koc, M. Mar, A. Ranasinghe, J. A. Swenberg and S. H. Zeisel, Anal. Chem., 2002, 74, 4734–4740 CrossRef CAS PubMed.
- S. E. Ong and M. Mann, Nat. Protoc., 2006, 1, 2650–2660 CrossRef CAS PubMed.
- C. Guerrero, C. Tagwerker, P. Kaiser and L. Huang, Mol. Cell. Proteomics, 2006, 5, 366–378 CAS.
- B. M. Zee, R. S. Levin, B. Xu, G. LeRoy, N. S. Wingreen and B. A. Garcia, J. Biol. Chem., 2010, 285, 3341–3350 CrossRef CAS PubMed.
- A. F. M. Altelaar, C. K. Frese, C. Preisinger, M. L. Hennrich, A. W. Schram, H. T. M. Timmers, A. J. R. Heck and S. Mohammed, J. Proteomics, 2013, 88, 14–26 CrossRef CAS PubMed.
- S. Neubauer, C. Haberhauer-Troyer, K. Klavins, H. Russmayer, M. G. Steiger, B. Gasser, M. Sauer, D. Mattanovich, S. Hann and G. Koellensperger, J. Sep. Sci., 2012, 35, 3091–3105 CrossRef CAS PubMed.
- K. Klavins, S. Neubauer, A. Al Chalabi, D. Sonntag, C. Haberhauer-Troyer, H. Russmayer, M. Sauer, D. Mattanovich, S. Hann and G. Koellensperger, Anal. Bioanal. Chem., 2013, 405, 5159–5169 CrossRef CAS PubMed.
- K. Yang and X. Han, Metabolites, 2011, 1, 21–40 CrossRef CAS PubMed.
- C. S. Ejsing, J. L. Sampaio, V. Surendranath, E. Duchoslav, K. Ekroos, R. W. Klemm, K. Simons and A. Shevchenko, Proc. Natl. Acad. Sci. U. S. A., 2009, 106, 2136–2141 CrossRef CAS PubMed.
- K. Schuhmann, R. Almeida, M. Baumert, R. Herzog, S. R. Bornstein and A. Shevchenko, J. Mass Spectrom., 2012, 47, 96–104 CrossRef CAS PubMed.
- L. A. Heiskanen, M. Suoniemi, H. X. Ta, K. Tarasov and K. Ekroos, Anal. Chem., 2013, 85, 8757–8763 CrossRef CAS PubMed.
- M. Wang, Y. Huang and X. Han, Rapid Commun. Mass Spectrom., 2014, 28, 2201–2210 CrossRef CAS PubMed.
- M. A. Surma, R. Herzog, A. Vasilj, C. Klose, N. Christinat, D. Morin-Rivron, K. Simons, M. Masoodi and J. L. Sampaio, Eur. J. Lipid Sci. Technol., 2015, 117, 1540–1549 CrossRef CAS PubMed.
- C. Coman, F. A. Solari, A. Hentschel, A. Sickmann, R. P. Zahedi and R. Ahrends, Mol. Cell. Proteomics, 2016, 15, 1453–1466 CAS.
- J. M. Cregg, K. J. Barringer, A. Y. Hessler and K. R. Madden, Mol. Cell. Biol., 1985, 5, 3376–3385 CrossRef CAS PubMed.
- M. J. Wood and E. A. Komives, J. Biomol. NMR, 1999, 13, 149–159 CrossRef CAS PubMed.
- D. Porro, B. Gasser, T. Fossati, M. Maurer, P. Branduardi, M. Sauer and D. Mattanovich, Appl. Microbiol. Biotechnol., 2011, 89, 939–948 CrossRef CAS PubMed.
- C. Rebnegger, A. B. Graf, M. Valli, M. G. Steiger, B. Gasser, M. Maurer and D. Mattanovich, Biotechnol. J., 2014, 9, 511–525 CrossRef CAS PubMed.
- J. Folch, M. Lees and G. H. Sloane Stanley, J. Biol. Chem., 1952, 226, 497–509 Search PubMed.
- R. Herzog, K. Schuhmann, D. Schwudke, J. L. Sampaio, S. R. Bornstein, M. Schroeder and A. Shevchenko, PLoS One, 2012, 7, 15–20 Search PubMed.
- B. Peng and R. Ahrends, J. Proteome Res., 2016, 15, 291–301 CrossRef CAS PubMed.
- J. A. Yergey, Int. J. Mass Spectrom. Ion Phys., 1983, 52, 337 CrossRef CAS.
-
M. Senko, https://sites.google.com/site/isoproms/home, 2009.
- K. Ekroos, I. V. Chernushevich, K. Simons and A. Shevchenko, Anal. Chem., 2002, 74, 941–949 CrossRef CAS PubMed.
- W. Lehmann, Mass Spectrom. Rev., 2017, 36, 58–85 CrossRef CAS PubMed.
- S. S. Iyer, Z.-P. Zhang, G. E. Kellogg and H. T. Karnes, J. Chromatogr. Sci., 2004, 42, 383–387 CAS.
- S. Neubauer, C. Haberhauer-Troyer, K. Klavins, H. Russmayer, M. G. Steiger, B. Gasser, M. Sauer, D. Mattanovich, S. Hann and G. Koellensperger, J. Sep. Sci., 2012, 35, 3091–3105 CrossRef CAS PubMed.
- A. De Ghellinck, H. Schaller, V. Laux, M. Haertlein, M. Sferrazza, E. Maréchal, H. Wacklin, J. Jouhet and G. Fragneto, PLoS One, 2014, 9, 1–9 Search PubMed.
- C. C. Stowers and E. M. Boczko, Yeast, 2007, 24, 533–541 CrossRef CAS PubMed.
- J. D. Stenson, C. R. Thomas and P. Hartley, Chem. Eng. Sci., 2009, 64, 1892–1903 CrossRef CAS.
- N. A. Shaikh, Anal. Biochem., 1994, 216, 313–321 CrossRef CAS PubMed.
- V. A. Ivashov, K. Grillitsch, H. Koefeler, E. Leitner, D. Baeumlisberger, M. Karas and G. Daum, Biochim. Biophys. Acta, Mol. Cell Biol. Lipids, 2013, 1831, 282–290 CrossRef CAS PubMed.
- K. Grillitsch, P. Tarazona, L. Klug, T. Wriessnegger, G. Zellnig, E. Leitner, I. Feussner and G. Daum, Biochim. Biophys. Acta, 2014, 1838, 1889–1897 CrossRef CAS PubMed.
- L. Klug, P. Tarazona, C. Gruber, K. Grillitsch, B. Gasser, M. Trötzmüller, H. Köfeler, E. Leitner, I. Feussner, D. Mattanovich, F. Altmann and G. Daum, Biochim. Biophys. Acta, Mol. Cell Biol. Lipids, 2014, 1841, 215–226 CrossRef CAS PubMed.
- R. Schneiter, B. Brügger, R. Sandhoff, G. Zellnig, A. Leber, M. Lampl, K. Athenstaedt, C. Hrastnik, S. Eder, G. Daum, F. Paltauf, F. T. Wieland and S. D. Kohlwein, Cell, 1999, 146, 741–754 CrossRef CAS.
- A. Singh and M. Del Poeta, Front. Microbiol., 2016, 7, 1–14 Search PubMed.
- P. Ternes, T. Wobbe, M. Schwarz, S. Albrecht, K. Feussner, I. Riezman, J. M. Cregg, E. Heinz, H. Riezman, I. Feussner and D. Warnecke, J. Biol. Chem., 2011, 286, 11401–11414 CrossRef CAS PubMed.
- H. Yazawa, H. Iwahashi, Y. Kamisaka, K. Kimura and H. Uemura, Yeast, 2009, 26, 167–184 CrossRef CAS PubMed.
- K. Natter and S. D. Kohlwein, Biochim. Biophys. Acta, Mol. Cell Biol. Lipids, 2013, 1831, 314–326 CrossRef CAS PubMed.
- R. A. Darby, S. P. Cartwright, M. V. Dilworth and R. M. Bill, Methods Mol. Biol., 2012, 866, 11–23 CAS.
- W. Vervecken, V. Kaigorodov, N. Callewaert, S. Geysens, K. De Vusser and R. Contreras, Appl. Environ. Microbiol., 2004, 70, 2639–2646 CrossRef CAS PubMed.
- R. Guerrasio, C. Haberhauer-Troyer, D. Mattanovich, G. Koellensperger and S. Hann, Anal. Bioanal. Chem., 2014, 406, 915–922 CrossRef CAS PubMed.
- C. Haberhauer-Troyer, M. Delic, B. Gasser, D. Mattanovich, S. Hann and G. Koellensperger, Anal. Bioanal. Chem., 2013, 405, 2031–2039 CrossRef CAS PubMed.
- C. Klose, M. A. Surma, M. J. Gerl, F. Meyenhofer, A. Shevchenko and K. Simons, PLoS One, 2012, 7, 1–11 Search PubMed.
- E. L. Donovan, S. M. Pettine, M. S. Hickey, K. L. Hamilton and B. F. Miller, Diabetol. Metab. Syndr., 2013, 5, 24 CrossRef CAS PubMed.
- S. Priyadarsini, T. B. McKay, A. Sarker-Nag, J. Allegood, C. Chalfant, J.-X. Ma and D. Karamichos, Exp. Eye Res., 2016, 153, 90–100 CrossRef CAS PubMed.
- E. Cifkova, M. Holcapek, M. Lisa, M. Ovcacikova, A. Lycka, F. Lynen and P. Sandra, Anal. Chem., 2012, 84, 10064–10070 CrossRef CAS PubMed.
- X. Liu, Z. Ser and J. W. Locasale, Anal. Chem., 2014, 86, 2175–2184 CrossRef CAS PubMed.
- J. Zhou, H. Liu, Y. Liu, J. Liu, X. Zhao and Y. Yin, Anal. Chem., 2016, 88, 4478–4486 CrossRef CAS PubMed.
-
B. Magnusson and U. Örnemark, Eurachem Guide: The Fitness for Purpose of Analytical Methods – A Laboratory Guide to Method Validation and Related Topics, 2nd edn, 2014 Search PubMed.
Footnotes |
† Electronic supplementary information (ESI) available. See DOI: 10.1039/c7an00107j |
‡ These authors contributed equally. |
|
This journal is © The Royal Society of Chemistry 2017 |