DOI:
10.1039/C5NR08944A
(Feature Article)
Nanoscale, 2016,
8, 9919-9943
How should the completeness and quality of curated nanomaterial data be evaluated?†
Received
16th December 2015
, Accepted 26th April 2016
First published on 27th April 2016
Abstract
Nanotechnology is of increasing significance. Curation of nanomaterial data into electronic databases offers opportunities to better understand and predict nanomaterials’ behaviour. This supports innovation in, and regulation of, nanotechnology. It is commonly understood that curated data need to be sufficiently complete and of sufficient quality to serve their intended purpose. However, assessing data completeness and quality is non-trivial in general and is arguably especially difficult in the nanoscience area, given its highly multidisciplinary nature. The current article, part of the Nanomaterial Data Curation Initiative series, addresses how to assess the completeness and quality of (curated) nanomaterial data. In order to address this key challenge, a variety of related issues are discussed: the meaning and importance of data completeness and quality, existing approaches to their assessment and the key challenges associated with evaluating the completeness and quality of curated nanomaterial data. Considerations which are specific to the nanoscience area and lessons which can be learned from other relevant scientific disciplines are considered. Hence, the scope of this discussion ranges from physicochemical characterisation requirements for nanomaterials and interference of nanomaterials with nanotoxicology assays to broader issues such as minimum information checklists, toxicology data quality schemes and computational approaches that facilitate evaluation of the completeness and quality of (curated) data. This discussion is informed by a literature review and a survey of key nanomaterial data curation stakeholders. Finally, drawing upon this discussion, recommendations are presented concerning the central question: how should the completeness and quality of curated nanomaterial data be evaluated?
1. Introduction
The technological application of engineered nanomaterials, known as “nanotechnology”,1–3 is of increasing significance.4–6 Nanomaterials are commonly defined as materials comprising (a majority of) constituent particles with at least one (external) dimension in the nanoscale (1–100 nanometres) range.1,7–11 Nanomaterials have been used or considered for use in a wide variety of areas such as electronics, consumer products, agrochemicals and medical applications.2,5,6,12–15 However, concerns have been raised regarding the potential effects of nanomaterials on the environment and on human health.4,6,14–16 The study of the properties and behaviour of nanomaterials is within the domain of “nanoscience”, encompassing fields such as “nanoinformatics”, “nanochemistry”, “nanomedicine” and “nanotoxicology”.
The design of novel nanomaterials with desirable properties and acceptable safety profiles, as well as the appropriate regulation of both new and existing nanomaterials, relies upon nanoscience researchers (both experimentalists and computational modellers), risk assessors, regulators and other relevant stakeholders having access to the necessary data and metadata.
These data should be sufficiently complete, including their associated metadata, and of acceptable quality to render them fit for their intended purpose e.g. risk assessment. However, defining what one means by data which are “sufficiently complete” and of “acceptable quality” is non-trivial in general and is arguably especially challenging for the nanoscience area.
The current paper is part of a series of articles9,17 that address various aspects of nanomaterial data curation, arising from the Nanomaterial Data Curation Initiative (NDCI), where curation is defined as a “broad term encompassing all aspects involved with assimilating data into centralized repositories or sharable formats”.9 A variety of nanomaterial data resources, holding different kinds of data related to nanomaterials in a variety of formats, currently exist. Many of these were recently reviewed.9,18,19 The number of nanomaterial data resources is expected to increase as a result of ongoing research projects.4,19
An overview of the articles planned for the NDCI series was presented in Hendren et al.9 At the time of writing, an article on curation workflows17 was published and articles dedicated to curator responsibilities, data integration and metadata were at various stages of development. The current paper addresses the question of how to evaluate the degree to which curated nanomaterial data are “sufficiently complete” and of “acceptable quality”. In order to address this central question, the current paper addresses a number of key issues: (1) what the terms data completeness and quality mean; (2) why these issues are important; (3) the specific requirements for nanomaterial data and metadata intended to support the needs of specific stakeholders; (4) how to most appropriately score the degree of completeness and quality for a given nanomaterial data collection. The abstract meaning of data completeness and quality in a range of relevant disciplines is reviewed and the importance of these concepts to the area of nanomaterial data curation is explained. An overview of existing approaches for characterising the degree of completeness and quality of (curated) nanomaterial data is presented, with a focus on those currently employed by curated nanomaterial data resources. Approaches to evaluating data completeness and quality in mature disciplines are also reviewed, with a view to considering how the relatively young discipline of nanoscience could learn from these disciplines. However, as is also discussed, there are specific challenges associated with nanomaterial data which affect assessment of their completeness and quality. Drawing upon the discussion of these issues, the current paper concludes with a set of recommendations aimed at promoting and, in some cases, establishing best practice regarding the manner in which the completeness and quality of curated nanomaterial data should be evaluated.
The snapshot of current practice, discussion of key challenges and recommendations were informed via a review of the published literature as well as responses to a survey distributed amongst a variety of stakeholders associated with a range of nanomaterial data resources. The survey and responses can be found in the ESI,† along with an overview of the nanomaterial data resources managed by these stakeholders – with a focus on how they address the issues related to data completeness and quality. The perspectives of individuals involved in a variety of nanomaterial data resources were captured via this survey. However, the resources for which respondents agreed to participate in this survey should not be seen as comprehensive.9,18,19
For the purposes of the survey, the Nanomaterial Data Curation Initiative (NDCI) identified 24 data resources that addressed various nanomaterial data types: from cytotoxicity test results to consumer product information. Some of the identified resources were exclusively focussed on nanomaterial data, whereas others were broader databases holding some data for nanomaterials. Representatives of the 24 data resources were contacted by the NDCI and, in total, 12 liaisons, corresponding to nine (38%) of the 24 nanomaterial data resources, responded to the NDCI data completeness and quality survey. Some of the nine resources incorporated primary experimental data, whilst others were exclusively populated via literature curation. Some of these were in-house resources, whilst others were publicly available via the internet. The median experience of the survey respondents was 5 years in the nanomaterial data curation field, 10.5 years in the wider nanoscience field, and 5.5 years in the broader data curation field.
The rest of this paper is organised as follows. Section 2 reviews the meaning of data completeness and quality, in abstract terms, and then explains the importance of these issues in the context of nanomaterial data curation. Section 3 reviews existing proposals for characterising the completeness and quality of (curated) nanomaterial data. Section 4 reviews approaches for evaluating (curated) data completeness and quality which are employed in mature fields. Section 5 then discusses the key challenges associated with nanomaterial data which need to be taken into account when evaluating their completeness and quality. Section 6 presents the recommendations for evaluating curated nanomaterial data completeness and quality.
2. The meaning and importance of data completeness and quality
The importance of data completeness and quality is made clear by explaining what these concepts mean and their implications for a range of important issues. (Data completeness and quality are hereafter referred to as Key concept 1 and Key concept 3, with full descriptions presented in Tables 1 and 3, respectively.) The precise meanings of these concepts and the issues with which they are related are defined somewhat differently in the varied fields which are relevant to nanomaterial data curation e.g. informatics, toxicology and risk assessment. Nonetheless, it is possible to provide broad and flexible definitions which encompass a variety of perspectives.
Table 1 Key concept 1: data completeness. Broad and flexible definition employed for reviewing prior work
The completeness of data and associated metadata may be considered a measure of the availability of the necessary, non-redundant (meta)data for a given entity e.g. a nanomaterial or a set of nanomaterials in the context of nanoscience. However, there is no definitive consensus regarding exactly how data completeness should be defined in the nanoscience, or wider scientific, community.9,20–24 Indeed, metadata availability may be considered an issue distinct from data completeness.20,21 |
Data completeness may be considered to include, amongst other kinds of data and metadata, the extent of nanomaterial characterisation, both physicochemical and biological, under a specified set of experimental conditions and time points. It may also encompass the degree to which experimental details are described, as well as the availability of raw data, processed data, or derived data from the assays used for nanomaterial characterisation. Data completeness may be considered to be highly dependent upon both the questions posed of the data and the kinds of data, nanomaterials and applications being considered. Data completeness may be defined in terms of the degree of compliance with a minimum information checklist (Table 2). However, when estimating the degree of data completeness, it should be recognised that this will not necessarily be based upon consideration of all independent variables which determine, say, a given result obtained from a particular biological assay. This is especially the case when data completeness is assessed with respect to a predefined minimum information checklist (Table 2). Precise definitions of completeness may evolve in tandem with scientific understanding. |
Broad and flexible definitions of data completeness and quality are presented in Tables 1 and 3 respectively. These reflect the different and sometimes inconsistent definitions presented, either implicitly or explicitly, in the literature, during discussions amongst the co-authors and by respondents to the NDCI data completeness and quality survey. (The perspectives of the survey respondents are presented in the ESI.† Literature definitions of data completeness9,20–24 and quality9,20–23,25,26 are provided in ESI Tables S3 and S5† respectively.)
Section 6.1.1 proposes that more precise definitions be adopted by the nanoscience community. These more precise definitions are generally consistent with the definitions presented in Tables 1 and 3, but some issues incorporated into those broad and flexible definitions are deemed out of scope. However, the definitions provided in Tables 1 and 3 encompass the range of different perspectives encountered when preparing this paper. Hence, these definitions serve as a reference point for the purpose of reviewing existing approaches to evaluating data completeness and quality in sections 3, 4 and ESI S2.†
The following discussion expands upon the broad and flexible definitions presented in Tables 1 and 3. The importance of these concepts for nanomaterial data curation, and the issues with which they are commonly associated, is explained with reference to the nanoscience literature.
Data completeness may be considered a measure of the availability of the necessary, non-redundant data and associated metadata for a given entity (e.g. a nanomaterial). (Some scientists consider the availability of “metadata” to be a separate issue to data completeness.)20,21 The term “metadata” is broadly defined as “data which describes data”27 or “data about the data”.28 Defining exactly what is meant by “data” as opposed to “metadata” is challenging. For example, physicochemical characterisation data may be considered metadata associated with a biological datum obtained from testing a given nanomaterial in some assay.3 However, precisely delineating “data” and “metadata” lies beyond the scope of the current article. In this article, data and metadata are collectively referred to as “(meta)data”.
Generally, data completeness assesses the extent to which experimental details are described and associated experimental results are reported. One means of assessing the degree of completeness compliance is to employ a minimum information checklist. (This concept is referred to hereafter as Key Concept 2 and a broad and flexible definition is presented in Table 2. Literature definitions28,29 are presented in ESI Table S4.†) However, one may also draw a distinction between data which are truly complete and data which are compliant with a minimum information checklist. The checklist may simply specify the most important, but not the only important, (meta)data. For example, in the case of nanomaterial physicochemical characterisation, measurement of a large number of properties might be considered necessary for complete characterisation but not truly essential to achieve all study goals. These properties might be distinguished from “priority” or “minimum” properties which are “essential” to determine.3
Table 2 Key concept 2: minimum information checklist. Broad and flexible definition employed for reviewing prior work
Minimum information checklists might otherwise be referred to as minimum information standards, minimum information criteria, minimum information guidelines or data reporting guidelines etc.28,29 These checklists define a set of data and metadata which “should” be reported – if available – by experimentalists and/or captured during data curation. Again, the precise set of data and metadata which “should” be reported may be considered to be highly dependent upon both the questions posed of the data and the kinds of data, nanomaterials and applications being considered. There are two possible interpretations of the purpose of these checklists: (1) they should be used to support assessment of data completeness (Table 1); (2) data should be considered unacceptable if they are not 100% compliant with the checklist. |
The degree of data completeness, insofar as this refers to description of the necessary experimental details and availability of (raw) data, needs to be evaluated in a range of different nanoscience contexts. Firstly, it impacts the extent to which data are – and can be verified to be – reproducible.30–33 Reproducibility32–34 is contingent upon the degree to which the tested nanomaterial is identified and the experimental protocols, including the precise experimental conditions, are described.35 Given the context dependence of many properties which may identify nanomaterials, these two issues are interrelated. This is because nanomaterial identification, if based on physicochemical measurements, is not meaningful unless the corresponding experimental protocols are adequately described.3,36–40
Providing sufficient (meta)data to ensure the nanomaterial being considered is identified, to the degree required, is also inherently important to achieve the goals of “uniqueness” and “equivalency”.41 Establishing “uniqueness” means determining that nanomaterial A is different from B.41 Establishing “equivalency” means determining that nanomaterial A is – essentially – the same as B.41 Achieving “uniqueness” allows so-called “conflicting” results to be resolved.3 Achieving “equivalency” allows for data integration (e.g. to interrogate relationships between different kinds of data) using data reported for the same, or functionally equivalent, nanomaterial in different studies.
Physicochemical characterisation also assists with explaining observed differences in (biological) effects.3 Indeed, it facilitates the development of computational models for (biological) activity, based on the physicochemical properties as explanatory variables. Modelling of nanomaterial effects may entail the development of nanomaterial quantitative structure activity relationships (QSARs) – termed “nano-QSARs”,42 nanoscale structure–activity relationships (“nanoSARs”)43 and quantitative nanostructure–activity relationships (“QNARs”)44 – or “grouping” and “read-across” predictions for nanomaterial biological activity.44,45 Reporting of the experimental details associated with the generation of a given biological or physicochemical measurement facilitates assessment of whether data from different sources might be combined for modelling, given the potential trade-off between dataset size and heterogeneity.46,47
Data quality may be considered a measure of the potential usefulness, clarity, correctness and trustworthiness of data. Some data quality assessment proposals23,35,48 may talk interchangeably about the quality of data, datasets (or “data sets”), studies and publications. However, subsets of data from a given source (e.g. a dataset, study report or journal article) may be considered to be of different quality, depending upon exactly how data quality is defined and assessed.49 For example, the cytotoxicity data reported in a publication might be considered of different quality compared to the genotoxicity data. As another example, the data obtained for a single nanomaterial using a single assay might be considered of higher quality than the data obtained for a different nanomaterial and/or assay.
Whilst the quality of individual data points is an important issue, data points which – viewed in isolation – may be considered of insufficient quality to be useful may possibly be useful when used in combination with other data. For example, toxicity data which are evaluated as less reliable might be combined via a “weight-of-evidence” approach.35 As another example, in the context of statistical analysis, large sample sizes may partially offset random measurement errors.50 However, the importance of the reliability of the original data which are to be combined cannot be overlooked in either context.23,50
According to some definitions, data quality may be partly assessed based upon the relevance of the data for answering a specific question.27,48 Similarly, data completeness may also be considered highly context dependent. Here, the specific context refers to the kinds of data, the kinds of nanomaterials, the kinds of applications and the kinds of questions that need to be answered by a particular end user of the data. In other words, the degree to which the data are complete may be contingent upon “the defined [business] information demand”.27
None of the preceding discussion addresses the key question of how exactly to evaluate data completeness or quality for (curated) nanomaterial data. This question will be addressed in subsequent sections of the current paper.
3. Existing proposals for evaluating nanomaterial data completeness and quality
A plethora of proposals has been presented for assessing data completeness and quality in the nanoscience area. Because it would not be practical to comprehensively list and discuss all existing proposals in the current work, the following discussion (sections 3.1 and 3.2) aims to be illustrative of the different proposals which have been developed – with an emphasis on the most recent and those which are employed by the maintainers of specific curated nanomaterial data resources. Examples are taken from the published literature as well as the responses to the survey which informed the current article. A summary of the evaluation schemes, if any, employed by each of the data resources represented by the respondents to the survey is provided in the ESI.†
3.1. An overview of nanomaterial data completeness proposals
Considerable attention has been paid to identifying the minimum set of physicochemical parameters for which it is anticipated that nanomaterials with similar values for these parameters would exhibit similar effects in biological (e.g. toxicological) tests or clinical studies.3 Here, “physicochemical parameters” refers to the characteristics/properties relevant for the description of a nanomaterial such as chemical composition, shape, size and size distribution statistics. A number of lists exist, including the well-known MINChar Initiative Parameters List, proposed in 2008.60 Earlier efforts to provide minimum characterisation criteria for nanomaterials included the work carried out by the prototype Nanoparticle Information Library (NIL).61–63 The prototype NIL was developed in 2004 to illustrate how nanomaterial data could be organised and gave examples of what physicochemical parameters, along with corresponding information regarding synthesis and characterisation methodology, might be included for nanomaterial characterisation (see the ESI† for further details). In 2012, Stefaniak et al. identified and carefully analysed 28 lists (published between 2004 and 2011) which proposed “properties of interest” (for risk assessment), from which 18 lists of “minimum” – or, in their terms, “priority” – properties were discerned.3 These authors summarised the properties found on these lists and the corresponding frequency of occurrence across all lists. Other lists39,64–69 of important physicochemical parameters have been published subsequent to the analysis of Stefaniak et al.3
Arguably, within nanoscience, less attention70 has been paid to the question of which additional experimental details (e.g. the cell density,71 number of particles per cell,72 cell line used, passage number used or exposure medium constituents73,74 in cell-based in vitro assays) need to be recorded. It is important to note that many of the physicochemical characteristics which define the identity of a nanomaterial are highly dependent upon experimental conditions such as the pH and biological macromolecules found in the suspension medium.36,39,40 Nonetheless, some lists which specify key experimental details that should be reported (in addition to key physicochemical parameters) do exist.3,60,64,66,75,76 Indeed, it should be noted that some lists focused on the minimum physicochemical parameters which should be reported also suggest certain experimental conditions such as “particle concentration”3 and “media”60 should be reported. (Here, the potential ambiguity as to what is considered a physicochemical parameter for a nanomaterial sample and what is considered an experimental condition should be noted: “particle concentration”3 and “pH”77 may be considered either as physicochemical properties or important experimental conditions.)36 Other proposals, such as the caNanoLab data availability standard,78 go further and stipulate that other (meta)data, such as characterisation with respect to specific biological endpoints, should be made available.
Key international standards bodies, the Organisation for Economic Co-operation and Development (OECD) and the International Standards Organisation (ISO), have also made recommendations regarding physicochemical parameters and other experimental variables which should be reported for various kinds of experimental studies of nanomaterials.79–85 Notable reports include the “Guidance Manual for the Testing of Manufactured Nanomaterials: OECD Sponsorship Programme”80 which stipulates physicochemical parameters and biological endpoints which needed to be assessed, as part of the OECD's “Safety Testing of a Representative Set of Manufactured Nanomaterials” project, and a guidance document on sample preparation and dosimetry,81 which highlights specific experimental conditions, associated with stepwise sample preparation for various kinds of studies, that should be reported.
Many of the proposals cited above are not associated with a specific curated nanomaterial data resource, although some which were intended as recommendations for experimentalists (e.g. the MINChar Initiative Parameters List)60 have been used as the basis for curated data scoring schemes.78 Examples of proposals which are specifically used as the basis of a scoring scheme, partly or wholly based upon data completeness, for curated nanomaterial data include those employed by the Nanomaterial Registry,39,86,87 caNanoLab78 as well as the MOD-ENP-TOX and ModNanoTox projects (see ESI†).
Some proposals draw a distinction between broader completeness criteria (see Table 1) and what may be considered “minimum information” criteria (see Table 2). For example, within the MOD-ENP-TOX project (see ESI†) a set of minimum physicochemical parameters were required to be reported within a publication in order for it to be curated: composition, shape, crystallinity and primary size. Additional physicochemical parameters (such as surface area) were deemed important for the data to be considered complete. This is in keeping with many proposals reviewed by Stefaniak et al.,3 which drew a distinction between “properties of interest” and “minimum” (or “priority”) properties, as well as publications proposing increasing characterisation requirements within a tiered approach to nanosafety assessment.67,68
Some proposals have also stressed the context dependence of completeness definitions. For example, the ModNanoTox project proposed (see ESI†) that certain physicochemical parameters and experimental metadata were only relevant for certain kinds of nanomaterials: crystal phase was considered crucial for TiO2 nanoparticles but less important for CeO2 nanoparticles, in keeping with an independent review of the literature emphasising the importance of crystal phase data for TiO2 nanomaterials specifically.68 Recent publications have also stressed the importance of characterisation requirements depending upon the type of nanomaterials studied and otherwise being relevant for the specific study.68,88,89
Indeed, in contrast to the proposals discussed above which define specific (meta)data requirements, the developers of the Center for the Environmental Implications of NanoTechnology (CEINT) NanoInformatics Knowledge Commons (CEINT NIKC) data resource90–92 have proposed that data completeness be calculated on a use-case-specific basis i.e. with respect to the (meta)data which a given database query aims to retrieve. For example, a researcher interested in the die off rate of fish due to nanomaterial exposure would need mortality data at multiple time points, whereas a researcher interested in mortality after, say, one week would only need data at a single time point.
3.2. An overview of nanomaterial data quality assessment proposals
Various schemes for scoring/categorising nanomaterial data (in part) according to their quality have been proposed in recent years. Because data completeness (see Table 1) and quality (see Table 3) may be considered highly interrelated, a number of these schemes are strongly based upon consideration of (meta)data availability. One of the simplest schemes, presented by Hristozov et al.,93 assessed the reliability of toxicity data in nanomaterial databases based purely upon the availability of basic provenance metadata: data were considered “unusable”, or “unreliable”, where a result from a study is not accompanied by a “properly cited reference”. Significantly more sophisticated schemes exist which take into account the availability of a variety of additional (meta)data such as the availability of certain physicochemical data and experimental details concerning biological assay protocols. One such sophisticated scheme is the iteratively developed DaNa “Literature Criteria Checklist”75,76 used to assess the quality of a given published study concerning a given nanomaterial for the purpose of preventing low quality scientific findings from being integrated within the DaNa knowledge base.94–96
Table 3 Key concept 3: data quality. Broad and flexible definition employed for reviewing prior work
Data quality may be considered a measure of the potential usefulness, clarity, correctness and trustworthiness of data and datasets. However, there is no definitive consensus regarding exactly how data quality should be defined in the nanoscience, or wider scientific, community.9,20–23,25,26 |
Data quality may be considered dependent upon the degree to which the meaning of the data is “clear” and the extent to which the data are “plausible”.48 In turn, this may be considered to incorporate (aspects of) data completeness (Table 1). For example, data quality may be considered23 to be (partly) dependent upon the “reproducibility” of data31–34 and the extent to which data are reproducible and their reproducibility can be assessed will partly depend upon the degree of data completeness in terms of the, readily accessible, available metadata and raw data.30,35 As well as “reproducibility”, data quality may be considered to incorporate a variety of related issues. These issues include systematic and random “errors” in the data,32,33 data “precision” (which may be considered33 related to notions such as “repeatability”32–35 or “within-laboratory reproducibility”),33 “accuracy” and “uncertainty”.20,23,25,27,32,33,35,51–55 (As indicated by the cited references, different scientists may provide somewhat different definitions for these concepts. These concepts may be considered in a qualitative or quantitative sense.) Data quality may also be considered to be dependent upon the “relevance” of the data for answering a specific question, although data “relevance” might be considered an entirely distinct issue from data quality.23,48 In the context of data curation, not only the quality of the original experimental data needs to be considered but also quality considerations associated with curated data. Quality considerations associated with curation include the probability of transcription errors56 and possibly57 whether a given dataset, structured according to some standardised format (e.g. XML based),58 was compliant with the rules of the applicable standardised format (e.g. as documented via an XML schema).59 Such compliance, amongst other possible aspects of data quality, could be determined using validation software. |
Indeed, some existing nanomaterial quality proposals go beyond merely considering data completeness, but are also concerned with whether the experimental protocols were carried out appropriately. For example, Lubinski et al.47 proposed an extension of the Klimisch framework48 for evaluating the reliability of nanotoxicology, or nano-physicochemical, data which was considered, in part, to depend upon compliance with Good Laboratory Practice (GLP)97 and standardised test protocols. Other assessment schemes, such as the scheme employed by the DaNa75,76,94–96 project (see ESI†), take account of whether biological results were affected by assay interference.98–107 Indeed, application of the DaNa “Literature Criteria Checklist”75,76 entails making a range of judgements regarding the quality of the nanomaterial data which go beyond mere consideration of data completeness (see ESI†). Likewise, Simkó et al. proposed a range of criteria for evaluating in vitro studies, including clearly specified criteria for the statistical “quality of study”.108
Some, but not all, proposals for quality assessment of nanomaterial data have sought to assign a categorical or numeric score to express the quality of the nanomaterial data. One such scheme, which assigns a qualitative score, was proposed by Lubinski et al.47 Likewise, the “Data Readiness Levels” scheme proposed by the Nanotechnology Knowledge Infrastructure (NKI) Signature Initiative51 assigns any kind of data – i.e. not necessarily generated for nanomaterials – to one of seven, ranked categories denoting their “quality and maturity”. In contrast, the following schemes assign numeric quality scores and were specifically designed to evaluate nanomaterial data curated into a specific data resource. The Nanomaterial Registry,109,110 assigns normalised, numeric “compliance” scores to each nanomaterial record in the database based upon its associated measurements, corresponding to the physicochemical characteristics specified in the “minimal information about nanomaterials (MIAN)”, which are designed to capture the “quality and quantity” of the physicochemical characterisation performed for that nanomaterial.39,86,87 The MOD-ENP-TOX and ModNanoTox curated nanomaterial data resources also developed quality scoring schemes which assign numeric scores (see ESI†).
One notion of data quality (see Table 3) might be based on validation of dataset files, according to their data content or compliance with format specifications, using specialist software tools. (This is further discussed in section 4, with examples from mature fields.) In the nanoscience area, the validation tools111 developed within the MODERN E.U. FP7 project,112 used to validate ISA-TAB-Nano datasets based on their compliance with the ISA-TAB-Nano specification,113–115 were, to the best of the authors’ knowledge, the only such tools available at the time of writing which were specifically developed for validating curated nanomaterial datasets.
4. Lessons which can be learned from mature fields
In order to improve the means via which the completeness and quality of (curated) nanomaterial data are currently evaluated, it is worth considering the lessons which may be learned from “mature” fields.
A variety of different minimum information checklists or reporting guidelines (see Table 2) have been proposed in different areas of the life sciences. These are increasingly being used by publishers to assess the suitability of submitted publications.116–118 The seminal Minimum Information About a Microarray Experiment (MIAME) reporting guidelines were proposed over a decade ago to describe the minimum information required for microarray data to be readily interpreted and for results obtained from analysis of these data to be independently verified,116,119 which may be achieved if the results are reproducible. In under a decade, this standard was widely accepted and most scientific journals adopted these guidelines as a requirement for publication of research in this area, with authors being obliged to deposit the corresponding MIAME-compliant microarray data in recognised public repositories.116 A variety of similar guidelines116 were subsequently developed for other life science technologies (e.g. proteomics)120 or studies (e.g. toxicology121 and molecular bioactivity studies).122 The BioSharing project and online resource,123–126 originally founded as the MIBBI Portal in 2007,28 serves to summarise proposed “reporting guideline” standards and promote their development and acceptance. Clearly, the BioSharing online resource might be used to link to the various minimum information checklists that have been (implicitly) developed within the nanoscience domain (see section 3.1), thereby raising awareness of them and facilitating their comparison and further development. It is also possible that some of the recommendations made regarding experimental (meta)data in the (non-nanoscience specific) reporting guidelines linked to via the BioSharing website may also be applicable to (specific sub-domains of) the nanoscience area.
The Standard Reference Data Program of the U.S. National Institute of Standards and Technology (NIST)127 has supported the evaluation of data in many areas of science and technology. Typically, data are not only curated but also evaluated from three perspectives: documentation of the identification and control of the independent variables governing a measurement; the consistency of measurement results with the laws of nature; and through comparison with similar measurements. Over the years it has become clear that, as new phenomena are identified and measured, it takes years – if not decades – to truly identify and understand how to control a measurement. Consequently, initial experiments produce data that primarily provide guidance for future experiments rather than be recognised as definitive properties. Feedback from the evaluation efforts to the experimental community is critical for improving the quality of data.
Chirico et al.53 recently described how NIST data resources and computational tools can be and are being used to improve the quality of thermophysical and thermochemical data submitted for publication within the context of a collaborative effort between NIST and five key journals.
Because uncertainty may be considered a key aspect (Table 3), or even the key aspect,25,52 of data quality evaluation, the approaches to characterising uncertainty proposed by ISO,25,52 NIST32 and SCENIHR23 merit consideration.
The concept of data quality has received considerable attention within the toxicology and risk assessment communities and a number of proposals for assessing the quality of data, studies or publications have been published.23,48,128–132 A number of these were reviewed in Ågerstrand et al.133 and Przybylak et al.49 Arguably the most well-known is the framework proposed by Klimisch et al.48 for categorising the reliability (see ESI Table S5† literature definition 3.4) of toxicology data, or a toxicology study test report or publication. The Klimisch categories are widely employed within regulatory toxicology.24,49,132,134
Since the original work of Klimisch et al.48 lacked detailed criteria for assigning their proposed reliability categories, the ToxRTool program131,135 was proposed as a means of improving the transparency and consistency with which these categories were assigned. The program assigns a reliability category based upon the score obtained after answering a set of “yes/no” questions. However, it is interesting to note that neither GLP nor test guideline compliance is explicitly considered by the ToxRTool when assessing reliability (although these issues are considered when evaluating “relevance”) – even though these were deemed key indicators of reliable data in the original work of Klimisch et al.48 Recently, an extension to the ToxRTool program was developed by Yang and co-workers.136 Their approach took the following issues into account: (1) an assessor might feel that a given ToxRTool criterion was only partially met, rather than it being possible to simply answer “yes/no” for that question; (2) an assessor might be unsure of the most appropriate answer to a given question. Hence, their approach, based on fuzzy arithmetic, allows toxicity data to be assigned to multiple reliability categories with different degrees of satisfaction.
Consideration of these different approaches to evaluating data quality raises some important questions which arguably need to be taken into account when designing a scheme for assessing the quality of nanosafety data or, where applicable, nanoscience data in general.
1. To what extent should quality be assessed on the basis of considering data completeness as opposed to making judgements regarding the data such as the “soundness and appropriateness of the methodology used”23 or, equivalently, whether or not a method was “acceptable”?48
2. More specifically, should data be considered most reliable48 when they were generated according to Good Laboratory Practice (GLP),97 or some other “audited scheme”23 and according to standardised test protocols,133 such as those presented in OECD Test Guidelines or by ISO? The appropriateness of adherence to standardised test protocols is especially relevant for testing of nanomaterials (see section 5.11). It may also be argued that, even for conventional chemicals, data which were not generated according to standardised test protocols and/or GLP are not necessarily less reliable.48,132,137
3. To what extent should a data quality assessment scheme be prescriptive as opposed to allowing for flexibility based upon expert judgement? Whilst a scheme which is more prescriptive offers the advantage of promoting transparency and consistency23,131 in the assigned quality scores (or categories), flexibility based upon allowing for expert judgement may still be necessary.23
4. Should the outcome of the quality assessment be expressed numerically? Beronius et al.132 have argued that this risks implying an undue level of scientific certainty in the final quality assessment. However, using a qualitative scheme based on certain criteria being met in order for data to be assigned to a particular category would fail to assign partial credit to data meeting a subset of those criteria. Furthermore, as illustrated by the ToxRTool approach,131,135 a numeric score might be mapped onto a qualitative category for ease of interpretation.
5. How can the community best characterise uncertainty to provide a clearer understanding of data quality?
The preceding discussion concerns proposals which might be applied by a human expert for the purposes of assessing data completeness and quality in various domains. In principle, where these schemes are sufficiently prescriptive, rather than relying on subjective expert judgement they could be applied programmatically i.e. via parsing a structured electronic dataset or database using specialist software.
Indeed, various validation software programs have been developed to validate electronic datasets, based on standardised file formats, according to a range of criteria. For example, validation programs have been developed to validate different kinds of biological (meta)data reported in XML-based58,59,138 or ISA-TAB139,140 formats and, more specifically, raw sequence and sequence alignment data141–144 reported in FastQ142–144 or Binary Alignment/Map (BAM) format.145 Validation software146,147 was also developed for crystallographic data reported in the crystallographic information file (CIF) format.148
As well as checking format compliance, some of these validation programs may also be used to enforce compliance with (implicit) minimum information checklists.138,149 For example, The Cancer Genome Atlas (TCGA)150 validation software checks certain fields to ensure they are not “null” (unknown) or missing, as well as carrying out various other data quality checks for errors and inconsistencies.138 Software used to validate sequence data may carry out data quality assessment via calculating a variety of metrics, including those which are indicative of different kinds of possible errors/biases/artefacts generated during measurement/analysis or possible contamination of the analysed samples.142–144
All of these software programs are potentially relevant to automatically validating nanomaterial characterisation and/or biological data. The ISA-TAB format151–153 was recently extended via the development of ISA-TAB-Nano113–115 to better capture nanomaterial (meta)data, so the ISA-Tools139,140 software might be extended to validate ISA-TAB-Nano datasets. (As is discussed in section 3.2, some software for validating ISA-TAB-Nano files already exists.)111,115 Validation software for CIF files is arguably of particular relevance to building quantitative structure–activity relationships (QSARs), or quantitative structure–property relationships (QSPRs), for nanomaterials. Crystallographic data has been used to calculate descriptors for nano-QSAR (or nano-QSPR) models of inorganic oxide nanoparticle activities (or properties) in various recent studies.42,154,155
5. Key challenges
Important challenges are associated with nanomaterial data which need to be taken into account when evaluating their completeness and quality. To some extent, a number of these issues are taken into account in a subset of the existing proposals for evaluating nanomaterial data (see section 3). Other challenges relate to limitations of (some) of these existing evaluation proposals. The key challenges are summarised in Table 4 and explained in the remainder of section 5.
Table 4 The key challenges which impact completeness and quality evaluations of (curated) nanomaterial data
Challenge no. |
Brief description |
5.1 |
Uncertainty regarding the most biologically significant variables |
5.2 |
Dependence of many physicochemical properties on experimental conditions |
5.3 |
Potential time dependence of physicochemical properties |
5.4 |
Problems expressing dosimetry in biological assays |
5.5 |
Possible redundancy in physicochemical data |
5.6 |
Batch-to-batch variability of nanomaterials |
5.7 |
Context dependency of (meta)data requirements |
5.8 |
Lack of clarity in some existing checklists |
5.9 |
Artefacts in biological studies related to nanomaterials |
5.10 |
Misinterpretations in biological studies |
5.11 |
Uncertainty regarding standardised test guidelines |
5.12 |
Reduced relevance of some standard assays |
5.13 |
Problems with analysis of environmental samples |
5.1. Uncertainty regarding the most biologically significant variables
A key challenge associated with defining minimum information criteria for nanomaterials is that the current understanding of the independent variables, such as nanomaterial physicochemical properties and other experimental variables, which contribute most significantly to the variability in the outputs of biological assays is arguably insufficient.3,41,68–70,89,105,156 Understanding which of the physicochemical properties are most correlated to biological effects is hampered by the dependence of many of these properties on experimental conditions (section 5.2), time (section 5.3), dosimetry uncertainty (section 5.4), possible redundancy in physicochemical data (section 5.5), the potential for artefacts in biological studies related to the presence of nanomaterials (section 5.9) and possible confounding factors (section 5.10).
5.2. Dependence of many physicochemical properties on experimental conditions
Many, but not necessarily all, physicochemical parameters may change significantly depending upon the dispersion (suspension) medium and any additives (e.g. dispersant aids)37i.e. many physicochemical characterisation data obtained under pristine conditions (e.g. dispersed in water) may differ greatly from those determined for the nanomaterial dispersed in the medium, plus additives, used for biological testing.36–40,157 This variability makes it difficult to find correlations between the physicochemical properties and the outcome of biological assays. No straightforward relationship can be expected to exist when these properties are measured under pristine conditions, or conditions which otherwise differ from biologically relevant conditions, even if a simple correlation exists when the physicochemical properties are measured under biologically relevant conditions. For example, a recent study found the positive zeta potential values measured in physiological saline (pH 5.6) exhibited good linear correlation with acute lung inflammogenicity, but not the negative values measured in more basic (pH 7.4) media.157 Other experimental conditions which may significantly affect physicochemical properties include sample processing details such as sonication steps.37
As well as making it harder to discern which physicochemical parameters are most important to measure and document, this challenge has the following implications for data completeness. Firstly, a careful description of the various factors which could affect physicochemical properties is required36,38,40,81 in order to establish “uniqueness” and “equivalency”41 based upon physicochemical characterisation. Secondly, measurement of many physicochemical characteristics under biologically relevant conditions, as is considered best practice,38 should assist with explaining biological results or developing structure–activity relationships.
5.3. Potential time dependence of physicochemical properties
Many nanomaterial characteristics may change over time, depending upon their environment and processing protocols, such as their state of agglomeration,40,81 their “corona”158–160 of adsorbed (biological) molecules40,161 and even primary particle characteristics such as chemical composition (e.g. via dynamic speciation)162,163 or morphology.37 Some of these changes may be reversible,159,164 whilst other processes may give rise to irreversible transformations or “aging”165 (“ageing”).166 These time dependent changes in physicochemical properties can give rise to changes in their biological effects.166
The first implication for data completeness is that temporal metadata,166 along with corresponding processing (e.g. sonication)37 and storage history166 details, are important to capture. Secondly, because “ageing” may have transformed the physicochemical characteristics responsible for biological activity, data for biological studies of nanomaterials might not be considered complete if key physicochemical characteristics were not measured at time points corresponding to biological testing.166
5.4. Problems expressing dosimetry in biological assays
The most appropriate dose metric to use in biological studies of nanomaterials is unclear and may depend upon the kind of nanomaterial being considered.167 Nonetheless, it is generally accepted77,81,167,168 that mass based concentrations and doses are less appropriate and that dose metrics based on the total surface area or number of particles should be considered: the use of mass based concentration units may give misleading indications as to the rank order of toxicity for different nanomaterials.77
Thus, the use of an inappropriate dose (or concentration) metric may be considered to adversely affect the clarity, hence the quality (see Table 3), of nanomaterial biological data. Since additional physicochemical data are required for conversion of the mass based concentration (or dose) units (e.g. surface area measurements or density measurements, depending upon the approach employed),36,77,81,168 this issue also has implications for the minimum information criteria which might be proposed for nanomaterial data. N.B. Different approaches for estimating surface area based dose units, based upon different physicochemical measurements, have distinct advantages and disadvantages: geometric estimates of surface area may be based upon simplistic assumptions regarding particle geometry and fail to take account of porosity, whilst surface area measurements under dry conditions may not reflect the accessible surface area under biological conditions.36,168
An additional problem is that the nominal, administered concentration (or dose) may not correspond to the concentration (or dose) delivered to the site of biological action.101,168–170 Hence, additional data completeness considerations for aquatic toxicity tests include measurements of exposure levels over the course of the experiment and data quality concerns arise regarding whether the experimental methods employed to quantify nanomaterials in complex media are appropriate (see section 5.13).101,169
5.5. Possible redundancy in physicochemical data
As discussed in section 5.4, different kinds of physicochemical data may be required to estimate surface area based dose units, depending upon the approach employed i.e. this is one source of potential redundancy in physicochemical characterisation requirements. However, as is also discussed in section 5.4, even ignoring other rationales for obtaining the same physicochemical data, the different strengths and weaknesses of alternative surface area based dosimetry approaches mean these data cannot be said to be completely interchangeable. The interrelatedness between nanomaterial physicochemical properties44,68,154 also means that, in principle, extensive lists of “essential” properties3 may call for excessive characterisation that is both a burden for experimentalists and curators. However, the degree of interrelatedness between physicochemical properties may not mean that some properties are entirely interchangeable and, furthermore, the relationships between different properties – especially if measured under different conditions – are arguably hard to discern.68 Indeed, investigating which properties correlate might be hampered by synthesis challenges5 which may be associated with producing systematically varied nanomaterial libraries.171
Given the lack of complete interchangeability and problems associated with determining correlations in physicochemical properties, reducing the necessary physicochemical characterisation data based on potential redundancy remains a challenge. Furthermore, a challenge which arises as a consequence of these correlations is that it may be difficult to interpret the effect of changing a given property upon biological activity (hence, the importance of measuring that property) without this being confounded by variation in other physicochemical parameters.
5.6. Batch-to-batch variability of nanomaterials
The issue of batch-to-batch variability, i.e. variability in the properties of nominally identical nanomaterials obtained via repetitions of nominally the same synthesis, is a key challenge which is particularly significant for industrially produced nanomaterials.5,38,172 The implications for data completeness are arguably that the batch identity of a given nanomaterial (as denoted via its “batch identifier”,38,173 “lot number”38,173or “manufacturer lot identifier”)174 should be documented, to establish nanomaterial “equivalency”,41 even for nanomaterials which are nominally the same e.g. which have the same trade name. However, since not all nanomaterial synthesis procedures may exhibit the same degree of batch-to-batch variability,5,38,172 the importance of these metadata may depend upon the kind of synthesis procedure. Indeed, the kind of synthesis route may be considered important metadata to curate174 for this reason and because it may implicitly convey (biologically relevant) information regarding chemical composition.175
5.7. Context dependency of (meta)data requirements
Data and metadata requirements may depend upon the experimental scenario and intended use of the data i.e. the specific context. Not all (meta)data are relevant for all experimental scenarios. For example, not all physicochemical parameters are applicable to all kinds of nanomaterials and those physicochemical parameters which contribute most significantly to nanomaterial effects may vary according to the kind of nanomaterial, their intended application and the specific effect of interest.3,68,69,83,88 Likewise, not all of the key experimental variables which (most) affect the outcome of biological testing will necessarily be common to all kinds of biological assays.105 For example, whether cytochalasin-B is employed during a micronucleus assay, which may be used to evaluate the genotoxicity of nanomaterials,6,176 can significantly affect the results.176,177 However, this experimental variable is not relevant for other genotoxicity tests.6,176 Moreover, in practice, different stakeholders will have different objectives i.e. the properties and experimental metadata which are important may vary between disciplines and user communities, or even within the same disciplines and communities the information requirements may vary according to the specific questions posed of the data.41
Hence, enforcing a single set of “minimum information” criteria could lead to some existing data being unnecessarily deprecated due to a lack of completeness even though the existing (meta)data are sufficient for specific purposes.89 For example, consider toxicological assessment of a commercially available nanomaterial with limited batch-to-batch variability,5,38,172 assessed during different studies at essentially the same point in its life-cycle or which is not significantly affected by “aging”.165 For such a nanomaterial, its trade name (“X”) might be considered a sufficiently unique identifier i.e. one can suppose that essentially the same material is being referred to in different studies of “X” or that the samples being assessed do not cause significantly different biological effects for the endpoint(s) of interest. If these data were simply being used to determine whether material “X” could cause a given set of effects (as determined in different studies), enforcing a requirement for adherence to a “minimum information checklist” in terms of physicochemical characterisation3 might be considered unnecessarily stringent i.e. in this context, detailed physicochemical characterisation might not be required to establish “equivalency”.41 Conversely, if a nano-QSAR modeller wished to generalise from these data (e.g. to build a relationship between physicochemical characteristics and a given adverse effect), then batch-specific physicochemical characterisation might be considered much more important.
In light of the context dependence discussed here and the evolving state of nanoscience (e.g. challenge 5.1), those utilising stringent “minimum information” schemes should anticipate that their criteria are not necessarily applicable in all contexts and are likely to be superseded as the field develops, instruments improve, and current hypotheses are exhausted. However, the underlying informational value of current and past data may nevertheless remain intact.
5.8. Lack of clarity in some existing checklists
Many existing proposals regarding important physicochemical data specify characteristics which are very broadly defined, rather than a specific set of measurements,3 making it unclear to researchers as to which measurements should be made. For example, many lists propose that the “agglomeration” or “aggregation” state be determined.3 However, a variety of different measurements (such as number of primary particles per aggregate or agglomerate, as might be quantified via the “average agglomeration number”,178 or assessment of particle size distributions under different conditions) might be considered to assess this.36,179
A related issue is that two protocols which are nominally measuring the same parameter (such as “average size”), may actually be providing different kinds of information that are not directly comparable.3,36,38,180 Different measurement techniques, such as transmission electron microscopy (TEM) and dynamic light scattering (DLS), employ different principles and assumptions to estimate “size” and may be measuring different aspects of “size” (e.g. “height above a flat substrate” or “hydrodynamic diameter”).3,180,181 Some techniques (e.g. TEM) may be used to estimate the “size” of agglomerates, aggregates or the primary particles, depending upon how the raw data are analysed,37,182 and different kinds of “average size” may be obtained using the same technique.36,180,181
The implications for data completeness are that (1) recommendations for specific kinds of physicochemical data, or clear guidance regarding acceptable alternatives, should be provided and (2) corresponding metadata regarding the measurement technique, the characterisation protocol and a precise description of the kind of statistical estimate produced (e.g. arithmetic mean of the number distribution vs. volume distribution)36 are important to capture.
5.9. Artefacts in biological studies related to nanomaterials
A growing body of literature has raised concerns regarding various artefacts which may affect the reliability of biological assessment of nanomaterials.70,98,99,101–107,183–186 These artefacts mean that the measurements obtained may not entirely correspond to the biological phenomena which the studies are trying to detect. For example, various kinds of nanomaterial “interference” with commonly used in vitro (cell-based) toxicity assays have been noted which may lead to overestimation or underestimation of toxicity.70,98,99,102–107,183–186 In in vivo aquatic toxicity studies, nanomaterials adhering to the surface of organisms may inhibit movement – leading to overestimation of mortality.101,187
An immediate implication for evaluating the quality of (curated) nanomaterial data is the need to evaluate the possibility for artefacts (e.g. interference). This is complicated by the fact that assay interference may be dependent upon the specific combination of assay, nanomaterial and tested concentration.98,106,185,186 Indeed, the possible dependency of assay interference on specific physicochemical characteristics106,185 may be another factor to take into account when evaluating completeness and quality.
Various recommendations have been made in the experimental literature for detecting and, in some cases, correcting for possible assay interference.104–106,184,185 In spite of this, analysis by Ong et al.,185 using a sample size of 200 papers for each year, suggested that around 95% (90%) of investigations using colorimetric or fluorescent-based assays published in 2010 (2012) failed to experimentally assess the potential for nanomaterial interference or, at least, failed to explicitly state that such potential interferences had been ruled out experimentally.
5.10. Misinterpretations in biological studies
As well as artefacts which lead to erroneous estimations of toxicity, a variety of additional factors may lead to erroneous interpretation of the cause of the toxicity observed when testing nanomaterials.104 For example, a failure to experimentally determine the presence of different kinds of impurities (e.g. endotoxin contamination, solvent contamination, metals) may lead to the observed toxicity being wrongly attributed to the nominal nanomaterial.104
The implications for data completeness are that thorough characterisation of the nanomaterial, including with respect to these key impurities, needs to be carried out when studying the biological effects of nanomaterials in order to meet the following objectives: (1) unless the nanomaterial identity is otherwise clear (see section 5.7), to associate a specific nanomaterial identity with the observed biological activity; (2) if desired, to ensure that any mechanistic interpretation of the biological effect is correct. Lack of clarity in the meaning of the data, such as failure to correctly identify which specific nanomaterial was tested in an assay, can also be considered to affect data quality (see Table 3).
5.11. Uncertainty regarding standardised test guidelines
An initial review188 of the applicability of the OECD test guidelines to nanomaterials – developed as standardised test protocols for conventional, small molecule chemicals101,188 – concluded that many (but not all) of these were applicable to nanomaterials in principle, if coupled with additional guidance documents regarding nanospecific issues.81,188 A related question is the requirement for OECD test guidelines for parameters which are specifically important for nanomaterials.68,189 However, these issues were still not fully resolved as of the time of writing.68,79,169,179,189–191 Also, at the time of writing, some standardised protocols for nanomaterial assessment with respect to a variety of endpoints were under development by ISO.82,192 Nonetheless, some recent articles in the nanotoxicology literature have strongly advocated the use of OECD test guidelines, or other standardised protocols, to evaluate nanomaterials.103,193,194
Clearly, if the use of established standardised protocols cannot be assured to address all of the concerns raised regarding the quality of nanomaterial data (e.g. the artefacts discussed in section 5.9), this has implications as to whether adherence to existing standardised protocols should be considered an indicator of high quality data, as supposed by some existing data quality evaluation schemes discussed in sections 3 and 4,47,48 compared to a novel protocol which may have been specifically designed to address these concerns. Indeed, it is, in principle, possible that the use of some existing standardised tests might miss novel endpoints or be based upon assumptions regarding the mode of action that are not applicable to some nanomaterials. For example, the use of “omics” methods in nanotoxicology is advocated due their ability to capture novel modes of action.195 However, the extent, if any, to which nanomaterials can cause novel harm, act via genuinely novel modes of action – or even exhibit novelty in the underlying45 mechanisms of action and/or structure activity relationships – has recently been debated.14,89,167,196–199
5.12. Reduced relevance of some standard assays
Another potential problem with some toxicity tests when applied to nanomaterials, as compared to testing of small molecules, is that they might be of reduced relevance for assessment of possible human health effects. For example, the Ames genotoxicity test and cytotoxicity tests, based on bacterial cell cultures, might be inappropriate for nanomaterials as bacterial sensitivity to nanomaterials may be significantly reduced compared to human cells,176,200 due to reduced uptake as a result of the cell wall and lack of endocytosis for bacterial cells.176 However, it should be noted that Holden et al.201 have suggested that bacterial studies may still be relevant to assessing potential nanomaterial impacts on human health, at least in terms of indirect effects following environmental release.
Reduced relevance for human health effects assessment is sometimes considered to be a data quality issue (see ESI Table S5† literature definition 3.4).48
5.13. Problems with analysis of environmental samples
The analysis of engineered nanomaterials, along with their derivatives, in environmental samples provides important information for risk assessment.202 The engineered nanomaterials first need to be detected, followed by quantification of their concentration and determination of their physicochemical properties.202 In particular, quantification of their concentration provides a direct means of validating the predictions of fate and transport models.203 However, obtaining reliable data on engineered nanomaterials in environmental samples remains challenging.202,203 In part, this reflects the need to make measurements at or below the detection limits for many analytical techniques. For example, many analytical techniques (e.g. dynamic light scattering) have detection limits101,203–206 which are too high to detect concentrations as low as those expected for engineered nanomaterials in environmental samples.101,203,205 Recently, single particle inductively coupled plasma mass spectrometry (SP-ICP-MS) has been advocated as a possible solution which would allow detection of realistic environmental concentrations and, in combination with additional information or assumptions, simultaneous measurement of particle size distributions.202,203,205 However, SP-ICP-MS is not without its limitations,202,203 including composition dependent size detection limits.205 (Indeed, detection of small particles is noted to be a problem with many analytical techniques due to their detection limits and/or low sensitivity for smaller particles.)206,207 In addition to these challenges, it has been argued that the most serious remaining problem with analysis of engineered nanomaterials in environmental samples is discriminating engineered from naturally occurring nanomaterials.203
The key challenges highlighted in this section emphasise the difficulties associated with generating sufficiently complete and high quality nanomaterial data. Consideration of these challenges is critical when evaluating the completeness and quality of (curated) nanomaterial data.
6. Recommendations for promoting and improving upon established best practice
The following recommendations are designed to promote established best practice or improve the manner in which the completeness and quality of curated nanomaterial data are evaluated. Many of these recommendations are also applicable to evaluating the completeness and quality of nanomaterial data reported in, say, the published literature prior to curation. They were informed by the preceding discussions regarding the meaning and importance of data completeness and quality (section 2), existing proposals for evaluating the completeness and quality of (curated) nanomaterial data (section 3), lessons which can be learned from mature fields (section 4) and the key challenges associated with nanomaterial data (section 5). These recommendations were developed by the authors of the current publication and were informed by the responses to the Nanomaterial Data Curation Initiative (NDCI) survey on data completeness and quality. (Full details of the recommendations made by specific survey respondents may be found in the ESI.†) However, they should not be considered to provide a definitive road-map for progress in this area which is endorsed by all authors and survey respondents. Rather, they summarise options for promoting best practice or improving the evaluation of the completeness and quality of curated nanomaterial data.
These recommendations are divided into five categories: terminology recommendations (section 6.1), specific (meta)data requirements (section 6.2), computational tool focused recommendations (section 6.3), strategic recommendations (section 6.4), and recommendations regarding the role specific organisations and scientific communities could play in advancing the manner in which the completeness and quality of curated nanomaterial data are evaluated (section 6.5).
To allow the reader to get a quick overview, the recommendations are merely summarised in the main text of the article. An in-depth discussion of these recommendations, including caveats, is provided in section S4 of the ESI.†
6.1. Terminology recommendations
It is proposed that the following definitions of terms (Table 5) should be adopted across the nanoscience community. The particular context in which these terms are explained is nanomaterial data curation. However, the definitions and many of the accompanying notes are relevant to the wider nanoscience, or broader scientific, community. These definitions build upon the broad and flexible definitions of (curated) data completeness (Table 1) and quality (Table 3) presented in section 2. The new definitions are generally consistent with the definitions presented in section 2. However, some issues incorporated into those broad and flexible definitions are deemed out of scope. For example, it is proposed that the relevance of the data for a particular purpose should be considered related to data completeness rather than quality.
Table 5 Recommendations regarding terminology concerning (curated) data completeness and quality in the nanoscience area
Recommendation no. |
Brief description |
Comment |
6.1.1 |
Specific definitions of completeness and quality are recommended to the nanoscience community. |
These definitions are not restricted for use within the nanoscience domain. Formal agreement on terms should proceed via ISO or some other standardisation body. |
The broad and flexible definitions (section 2) were appropriate for reviewing prior work as they ensured that different perspectives were not deemed out of scope. However, for the sake of greater clarity, the following, specific definitions are recommended to the community. This greater clarity will aid consideration of the practical recommendations presented in the remainder of this article.
6.1.1. Specific definitions of completeness and quality are recommended to the nanoscience community.
The terms data completeness and quality should be considered to be related but should not be used interchangeably. Guidance notes which further clarify the following definitions are presented in the detailed discussion of these terminology recommendations in ESI S4.†
Data completeness.
This is a measure of the extent to which the data and metadata which serve to address a specific need are, in principle, available.
Data quality.
This is a measure of the degree to which a single datum or finding is clear and the extent to which it, and its associated metadata, can be considered correct.
These abstract definitions are further clarified by Fig. 1, which illustrates the kinds of (meta)data requirements for data to be assessed as sufficiently complete and of acceptable quality. A more detailed discussion of specific (meta)data requirements is provided in section 6.2.
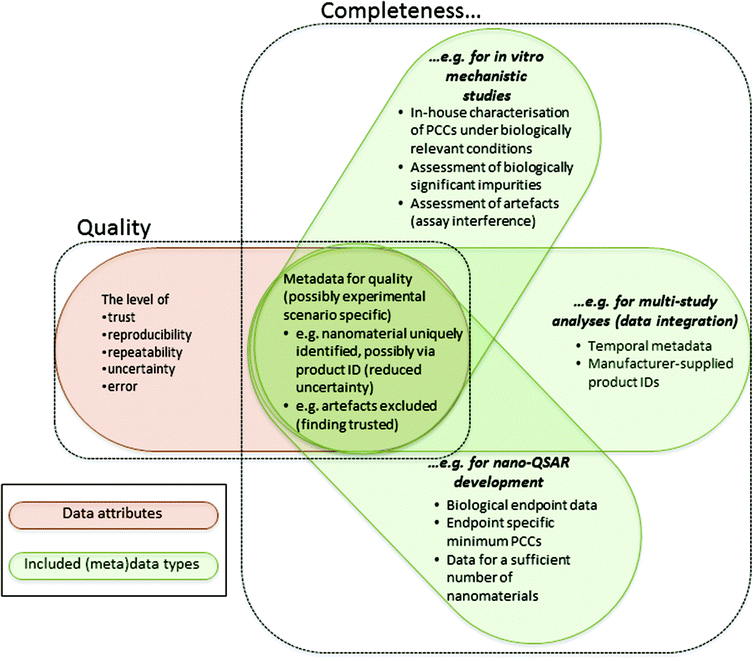 |
| Fig. 1 The quality and completeness of (curated) nanomaterial data are viewed as overlapping, yet distinct, concepts. This figure illustrates various contexts, meaning the experimental scenario and intended use of the data, and the kinds of (meta)data which may be required to assess those data as being sufficiently complete and of acceptable quality. N.B. (1) PCCs is an abbreviation for physicochemical characteristics. (2) The concept of data completeness applies to a set of data and their associated metadata. Hence, the number of data points of a specific kind (e.g. number of nanomaterials screened in a cytotoxicity assay) may be a completeness criterion in specific contexts if a given number of data points are required to achieve a specific aim. (3) In contrast, the concept of data quality applies to a single datum (i.e. a single data point) or a single “finding”, taking into account its associated metadata. A “finding” might be a conclusion derived from analysis of a set of raw or processed data and the “metadata” associated with that finding might include these data. (4) The dependence of both completeness and quality upon metadata is not entirely for the same reasons. For example, metadata (e.g. related to the nanomaterial identity and experimental conditions) are required to determine the relevance of the data for answering a specific question. The relevance of data for answering a specific question affects the completeness of the data, since only relevant data should be counted when evaluating completeness, but not the quality of a datum or finding. In addition, metadata are required to make the meaning of the datum or finding clear, reducing uncertainty in a qualitative sense and facilitating reproducibility, and to assess the level of trust, reproducibility, repeatability, uncertainty and error. All of these issues affect the quality of a datum or finding. However, the quality of a datum or finding does not directly affect the completeness of the data. (5) The context determines the (meta)data required for completeness. Whilst quality is not dependent upon the intended use of the data, the specific (meta)data required for quality assessment may be dependent upon the experimental scenario. For example, specific kinds of (meta)data will be required in specific in vitro studies to assess assay interference and, hence, assess the error in a given datum. (6) The examples in this figure are by no means exhaustive or, necessarily, minimum requirements. The example contexts and their requirements are not necessarily mutually exclusive. For example, a nano-QSAR might be developed via integrating data across multiple in vitro mechanistic studies. (7) Where examples are provided in this figure of specific metadata which might be required for data completeness in different contexts, it should be recalled that the availability of these metadata could also affect the quality of individual data points or findings. | |
6.2. Specific (meta)data requirements
6.2.1. Specific (meta)data highlighted by the NDCI survey.
The Nanomaterial Data Curation Initiative (NDCI) survey on data completeness and quality asked respondents to suggest the different kinds of (meta)data required in order for nanomaterial data to be considered sufficiently complete and of sufficient quality.
They were further asked to consider whether these (meta)data were only important in specific contexts and to identify those (meta)data they felt were most important to capture. The aim here was to capture recommendations even if they went beyond the (meta)data considered when curating the nanomaterial data resource for which they were acting as a liaison. (See the ESI† for further details.)
Some survey respondents emphasised that their responses were not intended to be a comprehensive summary of all (meta)data and considerations which would need to be taken into account in order to assess the completeness and quality of curated nanomaterial data. Rather, their responses to these questions highlighted issues (e.g. nanomaterial ageing) which they considered to be given insufficient attention. Some respondents kindly provided detailed lists of (meta)data and comments regarding additional considerations required for completeness and quality assessment. Some of these responses also gave some consideration to the relative importance and context/use-case dependence of certain kinds of (meta)data requirements.
The recommendations regarding physicochemical data which should be provided were generally in keeping with the kinds of physicochemical data recommended as being important in the lists analysed by Stefaniak et al.3 As well as physicochemical data, many kinds of metadata were also highlighted as being important for data to be determined to be sufficiently complete and/or of sufficient quality. Metadata recommendations were concerned with various issues, including experimental conditions, protocols and techniques, as well as data provenance, nanomaterial synthesis and experimental error.
Based on the survey responses and the literature review which informed the current article, a definitive list of all necessary (meta)data cannot be made. Neither can a definitive set of lists presenting all (meta)data requirements for different scenarios be made. Nonetheless, some key recommendations may be made.
6.2.2. Key recommendations regarding specific (meta)data.
Table 6 presents key recommendations concerning specific kinds of (meta)data which are important to capture in various curated nanomaterial data collections. ESI S4† explains these recommendations in detail.
Table 6 Key recommendations regarding specific (meta)data which are important for nanomaterials
Recommendation no. |
Brief description |
When is this important? |
6.2.2.1 |
For many physicochemical properties, in-house determination, including under biologically relevant exposure conditions, is recommended. |
In principle, whenever reporting physicochemical data from biological studies. However, the caveats documented in the detailed discussion of this recommendation, in the ESI, should be noted. |
6.2.2.2 |
Temporal metadata are particularly important to capture. |
In principle, when reporting data from any experimental study |
6.2.2.3 |
(Meta)data allowing for assessment of possible artefacts are required. |
In principle, when reporting data from any biological study |
6.2.2.4 |
(Meta)data related to experimental errors and uncertainty are required. |
In principle, when reporting data from any experimental study |
6.2.2.5 |
Data identifying (biologically significant) impurities are important. |
In principle, when reporting data from any experimental study |
6.2.2.6 |
Various manufacturer supplied IDs should be recorded. |
In principle, when trying to integrate data from different experimental studies |
6.2.2.7 |
Sufficient metadata should be provided to precisely identify any measured data. |
When reporting data from any experimental study |
6.2.2.8 |
Provenance metadata are essential. |
For all curation efforts |
6.2.2.9 |
Data regarding the surface composition and structure/morphology are important. |
In principle, when reporting data from any experimental study. N.B. The surface composition and structure/morphology may arise due to a ligand shell/layer. |
It should be noted that these recommendations are not a comprehensive list of all kinds of (meta)data which need to be captured in curated nanomaterial data collections. Rather, they are designed to emphasise key issues which are not always captured in existing minimum information checklists (section 3.1) or quality assessment schemes (section 3.2) for (curated) nanomaterial data. Additional (meta)data requirements might be determined via consulting existing proposals (see sections 3.1 and 3.2). Indeed, the need to consult existing recommendations is a key strategic recommendation (recommendation 6.4.1).
However, the possible dependence of (meta)data requirements upon the kinds of data and intended use of those data must be remembered (see section 5.7). This consideration is applicable, in principle, to the existing proposals (see sections 3.1 and 3.2) as well as the recommendations in Table 6. To some extent, the context dependence of the recommendations is indicated in Table 6. The discussion of these recommendations in ESI S4† considers this context dependence in greater depth.
6.3. Computational recommendations
Table 7 presents recommendations regarding how computational tools might be developed to support evaluation of the completeness and quality of curated nanomaterial data. Some of these recommendations concern existing nanoinformatics resources, whilst other computational tools may need to be developed de novo.
Table 7 Computational recommendations to support evaluation of the completeness and quality of curated nanomaterial data
Recommendation no. |
Brief description |
Comment |
6.3.1 |
Computational tools for assessment of completeness and quality should be developed. |
Careful consideration of the extent to which completeness and quality assessment could be automated using these tools is required and may be contingent upon progress towards recommendation 6.3.2. Recommendation 6.3.3 is also pertinent here. |
6.3.2 |
Standard templates for data exchange should be developed based upon the ISA-TAB-Nano specification. |
Some early work towards this objective has already been carried out. The required templates are likely to be scenario specific. |
6.3.3 |
Nanomaterial data resources providing completeness and quality scores should allow end-users to customise these based upon their own requirements. |
The scoring systems should include the ability to customise and select the criteria upon which the degree of data completeness (in terms of fitness for purpose), or quality, is defined and provide the decision process and justification involved in this. The potential need to customise data completeness scoring primarily stems from the dependency of completeness on the use-case. The potential need to customise data quality scoring primarily stems from the lack of universal standards as to quality determination. |
6.4. Strategic recommendations
The proposals in Table 8 should be considered in order to develop scientific strategies for improving the manner in which the completeness and quality of nanomaterial data are evaluated in future work.
Table 8 Recommended strategies to improve the manner in which the completeness and quality of nanomaterial data are evaluated in future work
Recommendation no. |
Brief description |
Comment |
6.4.1 |
Proposals for minimum information and data quality requirements could be informed via expert consensus, building upon existing proposals. |
Recommendations 6.4.1, 6.4.2 and 6.4.3 are not mutually exclusive. |
6.4.2 |
Proposals for minimum information and data quality requirements could be informed via targeted experimental studies. |
Recommendations 6.4.1, 6.4.2 and 6.4.3 are not mutually exclusive. |
6.4.3 |
Proposals for minimum information requirements could be informed via data mining. |
Recommendations 6.4.1, 6.4.2 and 6.4.3 are not mutually exclusive. |
6.4.4 |
To reduce redundancy in physicochemical characterisation requirements, further modelling (or experimental) efforts targeting the interrelatedness of different physicochemical characteristics are required. |
|
6.5. Institutional and community level recommendations
Table 9 summarises recommendations regarding initiatives which could be undertaken by various organisations, in collaboration with the wider nanoscience community, to improve the manner in which the completeness and quality of nanomaterial data are evaluated.
Table 9 Recommendations regarding actions which should be considered by various organisations to improve the manner in which the completeness and quality of nanomaterial data are evaluated
Recommendation no. |
Brief description |
Comment |
6.5.1 |
Work to develop and promote acceptance of minimum information checklists, data quality assessment schemes and related resources should be carried out in collaboration with suitable organisations with a global reach. |
Ongoing effort to support adoption and implementation will also be required, including by data curators. |
7. Conclusions
The curation of nanomaterial data into electronic resources is crucial to realise the potential of nanotechnology to deliver benefits to society whilst having acceptable impacts upon human health and the environment. In order for these data to be fit for their intended purposes, they need to be sufficiently complete and of acceptable quality. Hence, appropriate evaluation of the quality and completeness of curated nanomaterial data is essential even if, in practice, analysis and conclusions may need to be drawn from imperfect data: such an evaluation can inform awareness of the limitations of any work based upon the available data. Any such evaluation needs to take account of the issues related to the completeness and quality of the underlying experimental data as well as additional issues related to their curation such as transcription errors. However, carrying out this evaluation in practice is non-trivial. There are different perspectives as to exactly what these terms mean as well as different proposals as to how exactly the degree of completeness and quality of (curated) nanomaterial data should be evaluated in practice. After reviewing various existing proposals in light of broad and flexible definitions of these concepts, which accommodate the varying range of perspectives, more precise definitions are recommended to the nanoscience community. None of the existing proposals reviewed herein is perfect. A variety of challenges exist which impede appropriate evaluation of the completeness and quality of nanomaterial data. These challenges include the need to appropriately take account of the dependency of nanomaterial properties on their processing and storage history (i.e. time dependency), artefacts associated with biological testing of nanomaterials and incomplete understanding of which physicochemical properties and other experimental variables most significantly impact the effects of nanomaterials. In addition, the data requirements are likely to be dependent upon the precise experimental scenario (e.g. type of nanomaterials) and stakeholder requirements (e.g. regulatory decisions regarding a single nanomaterial vs. computational modelling). Some lessons might be learned from work in mature fields, such as the possibility of developing appropriate software tools to facilitate the efficient and transparent evaluation of (curated) experimental data. In the nanoscience domain, automated evaluation of data completeness and quality might best be supported via further development of nascent nanoinformatics resources. Common data collection templates based upon the ISA-TAB-Nano data exchange specification are envisaged. These will likely need to be adapted to the specific data requirements of different experimental scenarios and stakeholder objectives. The development of these resources will require community driven consensus regarding nanomaterial data requirements, which will best be supported by appropriate organisations and initiatives with an international reach. This article is one outcome of just such an initiative, the Nanomaterial Data Curation Initiative (NDCI), as reflected in the wide range of contributors and stakeholders who provided a variety of perspectives which informed the current work and resulted in a variety of recommendations to promote best practice and improve evaluation of the completeness and quality of (curated) nanomaterial data. An overview of the perspectives of these different stakeholders is presented in the ESI† of the current article.
Acknowledgements
RLMR is grateful for funding from the European Union Seventh Framework Programme (FP7/2007–2013) under grant agreement #309837 (NanoPUZZLES project). IL is grateful for funding from the European Union Seventh Framework Programme (FP7/2007–2013) under grant agreement #310451 (NanoMILE project). RP is grateful for funding from the European Union Seventh Framework Programme (FP7/2007–2013) under grant agreement #309666 (PreNanoTox – Predictive toxicology of engineered nanoparticles – project). PH and HV are grateful for funding from the European Union Seventh Framework Programme (FP7/2007–2013) under grant agreement #310715 (MOD-ENP-TOX project). WP is grateful for the support obtained within the RIVM sponsored project “IRAN”. COH would like to acknowledge the Center for the Environmental Implications of NanoTechnology (CEINT) funding from National Science Foundation (NSF) and the Environmental Protection Agency (EPA) under NSF Cooperative Agreement DBI-1266252 and EF-0830093. SLH would like to acknowledge support provided by the National Institute of Health (grant # ES017552). TP acknowledges the financial support of the Foundation for Polish Science (FOCUS Programme). Christoph Steinbach (DECHEMA) and the caNanoLab team are thanked for acting as additional liaisons for the DaNa2.0 Project (funded by the BMBF, Grant No. 03X0131) and caNanoLab (funded in whole or in part with Federal funds from the National Cancer Institute, National Institutes of Health, under Contract No. HHSN261200800001E) databases respectively. Mervi Heiskanen (NIH/NCI) is thanked for organising discussions between the authors of this paper and relevant stakeholders. Mark A. Musen (Stanford University), PI of CEDAR Project, is thanked for useful discussions and for commenting on part of the draft manuscript. Egon Willighagen (Maastricht University, eNanoMapper project), Nina Jeliazkova (IdeaConsult Ltd, eNanoMapper project), Sharon Gaheen (Leidos Biomedical Research Inc.), Sharon Ku (Drexel University), Martin J. Fritts (Nanotechnology Characterization Laboratory, SAIC-Fredrick, Inc., NCI at Fredrick), the U.S. National Cancer Institute (NCI) National Cancer Informatics Program (NCIP) Nanotechnology Working Group and the E.U. NanoSafety Cluster Databases Working Group are thanked for useful discussions. The findings and conclusions in this work are those of the authors and do not necessarily represent the views of their respective organisations or funding bodies. The information and opinions provided in this publication by members of the caNanoLab team are solely attributable to the caNanoLab team and none of the content of this publication necessarily reflects the views or policies of the National Cancer Institute (NCI), National Institutes of Health (NIH), Department of Health and Human Services, nor does mention of trade names, commercial products, or organizations imply endorsement by the U.S. Government.
References
-
G. Lövestam, H. Rauscher, G. Roebben, B. Sokull Klüttgen, N. Gibson, J.-P. Putaud and H. Stamm, Considerations on a Definition of Nanomaterial for Regulatory Purposes, European Commission Joint Research Centre, 2010 Search PubMed.
- D. G. Thomas, R. V. Pappu and N. A. Baker, NanoParticle Ontology for cancer nanotechnology research, J. Biomed. Inf., 2011, 44, 59–74 CrossRef PubMed.
- A. B. Stefaniak, V. A. Hackley, G. Roebben, K. Ehara, S. Hankin, M. T. Postek, I. Lynch, W.-E. Fu, T. P. J. Linsinger and A. F. Thünemann, Nanoscale reference materials for environmental, health and safety measurements: needs, gaps and opportunities, Nanotoxicology, 2013, 7, 1325–1337 CrossRef PubMed.
-
I. Lynch, Compendium of Projects in the European NanoSafety Cluster: 2014 Edition, 2014 Search PubMed.
- Y. Xia, Editorial: Are We Entering the Nano Era?, Angew. Chem., Int. Ed., 2014, 53, 12268–12271 CAS.
- N. Golbamaki, B. Rasulev, A. Cassano, R. L. M. Robinson, E. Benfenati, J. Leszczynski and M. T. D. Cronin, Genotoxicity of metal oxide nanomaterials: review of recent data and discussion of possible mechanisms, Nanoscale, 2015, 7, 2154–2198 RSC.
- European Commission, Commission Recommendation of 18 October 2011 on the definition of nanomaterial, Off. J. Eur. Union, 2011, 275, 38–40 Search PubMed.
- H. Rauscher, B. Sokull-Klüttgen and H. Stamm, The European Commission's recommendation on the definition of nanomaterial makes an impact, Nanotoxicology, 2013, 7, 1195–1197 CrossRef PubMed.
- C. O. Hendren, C. M. Powers, M. D. Hoover and S. L. Harper, The Nanomaterial Data Curation Initiative: A collaborative approach to assessing, evaluating, and advancing the state of the field, Beilstein J. Nanotechnol., 2015, 6, 1752–1762 CrossRef CAS PubMed.
-
ISO Technical Committee (ISO/TC) 229 - Nanotechnologies, ISO/TS 80004-1:2015 - Nanotechnologies – Vocabulary – Part 1: Core terms, International Standards Organisation, 2015 Search PubMed.
-
ISO Technical Committee (ISO/TC) 229 - Nanotechnologies, ISO/TS 80004-2:2015 - Nanotechnologies – Vocabulary – Part 2: Nano-objects, International Standards Organisation, 2015 Search PubMed.
- C. O. Hendren, X. Mesnard, J. Dröge and M. R. Wiesner, Estimating Production Data for Five Engineered Nanomaterials As a Basis for Exposure Assessment, Environ. Sci. Technol., 2011, 45, 2562–2569 CrossRef CAS PubMed.
- A. Gajewicz, B. Rasulev, T. C. Dinadayalane, P. Urbaszek, T. Puzyn, D. Leszczynska and J. Leszczynski, Advancing risk assessment of engineered nanomaterials: Application of computational approaches, Adv. Drug Delivery Rev., 2012, 64, 1663–1693 CrossRef CAS PubMed.
- A. D. Maynard, Is novelty overrated?, Nat. Nanotechnol., 2014, 9, 409–410 CrossRef CAS PubMed.
- R. S. Kookana, A. B. A. Boxall, P. T. Reeves, R. Ashauer, S. Beulke, Q. Chaudhry, G. Cornelis, T. F. Fernandes, J. Gan, M. Kah, I. Lynch, J. Ranville, C. Sinclair, D. Spurgeon, K. Tiede and P. J. Van den Brink, Nanopesticides: Guiding Principles for Regulatory Evaluation of Environmental Risks, J. Agric. Food Chem., 2014, 62, 4227–4240 CrossRef CAS PubMed.
- G. Oberdörster, E. Oberdörster and J. Oberdörster, Nanotoxicology: An Emerging Discipline Evolving from Studies of Ultrafine Particles, Environ. Health Perspect., 2005, 113, 823–839 CrossRef.
- C. M. Powers, K. A. Mills, S. A. Morris, F. Klaessig, S. Gaheen, N. Lewinski and C. O. Hendren, Nanocuration workflows: Establishing best practices for identifying, inputting, and sharing data to inform decisions on nanomaterials, Beilstein J. Nanotechnol., 2015, 6, 1860–1871 CrossRef CAS PubMed.
-
N. Jeliazkova, P. Doganis, B. Fadeel, R. Grafstrom, J. Hastings, V. Jeliazkov, P. Kohonen, C. R. Munteanu, H. Sarimveis, B. Smeets, G. Tsiliki, D. Vorgrimmler and E. Willighagen, The first eNanoMapper prototype: A substance database to support safe-by-design, in 2014 IEEE International Conference on Bioinformatics and Biomedicine (BIBM), 2014, pp. 1–9 Search PubMed.
-
A. P. Mustad, B. Smeets, N. Jeliazkova, V. Jeliazkov and E. Willighagen, Summary of the Spring 2014 NSC Database Survey, 2014 Search PubMed.
- C. Batini, C. Cappiello, C. Francalanci and A. Maurino, Methodologies for Data Quality Assessment and Improvement, ACM Comput. Surv., 2009, 41, 16 CrossRef.
- X. Fu, A. Wojak, D. Neagu, M. Ridley and K. Travis, Data governance in predictive toxicology: A review, J. Cheminf., 2011, 3, 24 Search PubMed.
- H.-J. Klimisch, M. Andreae and U. Tillmann, A Systematic Approach for Evaluating the Quality of Experimental Toxicological and Ecotoxicological Data, Regul. Toxicol. Pharmacol., 1997, 25, 1–5 CrossRef CAS PubMed.
-
A. Auvinen, J. Bridges, K. Dawson, W. De Jong, P. Hartemann, P. Hoet, T. Jung, M.-O. Mattsson, H. Norppa, J.-M. Pagès, A. Proykova, E. Rodríguez-Farré, K. Schulze-Osthoff, J. Schüz, M. Thomsen and T. Vermeire, Memorandum on the use of the scientific literature for human health risk assessment purposes – weighing of evidence and expression of uncertainty, SCENIHR (Scientific Committee on Emerging and Newly Identified Health Risks), 2012 Search PubMed.
-
Guidance on information requirements and chemical safety assessment. Chapter R.4: Evaluation of available information, European Chemicals Agency, 2011 Search PubMed.
-
ISO/TMBG (Technical Management Board - groups), ISO/IEC Guide 98-3:2008 - Uncertainty of measurement – Part 3: Guide to the expression of uncertainty in measurement (GUM:1995), International Standards Organisation, 2008 Search PubMed.
- R. D. Chirico, M. Frenkel, J. W. Magee, V. Diky, C. D. Muzny, A. F. Kazakov, K. Kroenlein, I. Abdulagatov, G. R. Hardin, W. E. Acree, J. F. Brenneke, P. L. Brown, P. T. Cummings, T. W. de Loos, D. G. Friend, A. R. H. Goodwin, L. D. Hansen, W. M. Haynes, N. Koga, A. Mandelis, K. N. Marsh, P. M. Mathias, C. McCabe, J. P. O'Connell, A. Pádua, V. Rives, C. Schick, J. P. M. Trusler, S. Vyazovkin, R. D. Weir and J. Wu, Improvement of Quality in Publication of Experimental Thermophysical Property Data: Challenges, Assessment Tools, Global Implementation, and Online Support, J. Chem. Eng. Data, 2013, 58, 2699–2716 CrossRef CAS.
- X. Fu, A. Wojak, D. Neagu, M. Ridley and K. Travis, Data governance in predictive toxicology: A review, J. Cheminf., 2011, 3, 24 Search PubMed.
- C. F. Taylor, D. Field, S.-A. Sansone, J. Aerts, R. Apweiler, M. Ashburner, C. A. Ball, P.-A. Binz, M. Bogue, T. Booth, A. Brazma, R. R. Brinkman, A. Michael Clark, E. W. Deutsch, O. Fiehn, J. Fostel, P. Ghazal, F. Gibson, T. Gray, G. Grimes, J. M. Hancock, N. W. Hardy, H. Hermjakob, R. K. Julian, M. Kane, C. Kettner, C. Kinsinger, E. Kolker, M. Kuiper, N. L. Novère, J. Leebens-Mack, S. E. Lewis, P. Lord, A.-M. Mallon, N. Marthandan, H. Masuya, R. McNally, A. Mehrle, N. Morrison, S. Orchard, J. Quackenbush, J. M. Reecy, D. G. Robertson, P. Rocca-Serra, H. Rodriguez, H. Rosenfelder, J. Santoyo-Lopez, R. H. Scheuermann, D. Schober, B. Smith, J. Snape, C. J. Stoeckert, K. Tipton, P. Sterk, A. Untergasser, J. Vandesompele and S. Wiemann, Promoting coherent minimum reporting guidelines for biological and biomedical investigations: the MIBBI project, Nat. Biotechnol., 2008, 26, 889–896 CrossRef CAS PubMed.
- D. G. Thomas, F. Klaessig, S. L. Harper, M. Fritts, M. D. Hoover, S. Gaheen, T. H. Stokes, R. Reznik-Zellen, E. T. Freund, J. D. Klemm, D. S. Paik and N. A. Baker, Informatics and standards for nanomedicine technology, Wiley Interdiscip. Rev.: Nanomed. Nanobiotechnol., 2011, 3, 511–532 CAS.
-
R. Van Noorden, Sluggish data sharing hampers reproducibility effort (Nature News & Comment) DOI:10.1038/nature.2015.17694 (accessed Nov 25, 2015).
- Challenges in irreproducible research (Nature News & Comment articles collection) http://www.nature.com/news/reproducibility-1.17552 (accessed Nov 25, 2015).
-
B. N. Taylor and C. E. Kuyatt, Guidelines for Evaluating and Expressing the Uncertainty of NIST Measurement Results, National Institute of Standards and Technology, 1994 Search PubMed.
-
L. P. van Reeuwijk and V. J. G. Houba, Guidelines for Quality Management in Soil and Plant Laboratories. (FAO Soils Bulletin - 74), Food and Agriculture Organization of the United Nations, 1998 Search PubMed.
- IUPAC. Compendium of Chemical Terminology, 2nd ed. (the ‘Gold Book’). Compiled by A. D. McNaught and A. Wilkinson. Blackwell Scientific Publications, Oxford (1997). XML on-line corrected version: http://goldbook.iupac.org (2006-) created by M. Nic, J. Jirat, B. Kosata; updates compiled by A. Jenkins. ISBN 0-9678550-9-8. DOI: DOI:10.1351/goldbook.
-
J. C. Madden, Chapter 5: Sources of Chemical Information, Toxicity Data and Assessment of Their Quality, in Chemical Toxicity Prediction: Category Formation and Read-Across, Royal Society of Chemistry, 2013 Search PubMed.
- K. W. Powers, S. C. Brown, V. B. Krishna, S. C. Wasdo, B. M. Moudgil and S. M. Roberts, Research Strategies for Safety Evaluation of Nanomaterials. Part VI. Characterization of Nanoscale Particles for Toxicological Evaluation, Toxicol. Sci., 2006, 90, 296–303 CrossRef CAS PubMed.
- R. C. Murdock, L. Braydich-Stolle, A. M. Schrand, J. J. Schlager and S. M. Hussain, Characterization of Nanomaterial Dispersion in Solution Prior to In Vitro Exposure Using Dynamic Light Scattering Technique, Toxicol. Sci., 2008, 101, 239–253 CrossRef CAS PubMed.
- R. M. Crist, J. H. Grossman, A. K. Patri, S. T. Stern, M. A. Dobrovolskaia, P. P. Adiseshaiah, J. D. Clogston and S. E. McNeil, Common pitfalls in nanotechnology: lessons learned from NCI's Nanotechnology Characterization Laboratory, Integr. Biol., 2013, 5, 66–73 RSC.
- K. C. Mills, D. Murry, K. A. Guzan and M. L. Ostraat, Nanomaterial registry: database that captures the minimal information about nanomaterial physico-chemical characteristics, J. Nanopart. Res., 2014, 16, 2219 CrossRef.
- E. Izak-Nau, M. Voetz, S. Eiden, A. Duschl and V. F. Puntes, Altered characteristics of silica nanoparticles in bovine and human serum: the importance of nanomaterial characterization prior to its toxicological evaluation, Part. Fibre Toxicol., 2013, 10, 1 CrossRef PubMed.
- J. Rumble and S. Freiman, Describing Nanomaterials: Meeting the Needs of Diverse Data Communities, Data Sci. J., 2012, 11, ASMD1–ASMD6 CrossRef.
- T. Puzyn, B. Rasulev, A. Gajewicz, X. Hu, T. P. Dasari, A. Michalkova, H.-M. Hwang, A. Toropov, D. Leszczynska and J. Leszczynski, Using nano-QSAR to predict the cytotoxicity of metal oxide nanoparticles, Nat. Nanotechnol., 2011, 6, 175–178 CrossRef CAS PubMed.
- R. Liu, R. Rallo, S. George, Z. Ji, S. Nair, A. E. Nel and Y. Cohen, Classification NanoSAR Development for Cytotoxicity of Metal Oxide Nanoparticles, Small, 2011, 7, 1118–1126 CrossRef CAS PubMed.
- I. Lynch, C. Weiss and E. Valsami-Jones, A strategy for grouping of nanomaterials based on key physico-chemical descriptors as a basis for safer-by-design NMs, Nano Today, 2014, 9, 266–270 CrossRef CAS.
-
Guidance on Grouping of Chemicals (ENV/JM/MONO(2014)4), Organisation for Economic Co-operation and Development, 2nd edn, 2014 Search PubMed.
- J. C. Dearden, M. T. D. Cronin and K. L. E. Kaiser, How not to develop a quantitative structure–activity or structure–property relationship (QSAR/QSPR), SAR QSAR Environ. Res., 2009, 20, 241–266 CrossRef CAS PubMed.
- L. Lubinski, P. Urbaszek, A. Gajewicz, M. T. D. Cronin, S. J. Enoch, J. C. Madden, D. Leszczynska, J. Leszczynski and T. Puzyn, Evaluation criteria for the quality of published experimental data on nanomaterials and their usefulness for QSAR modelling, SAR QSAR Environ. Res., 2013, 24, 995–1008 CrossRef CAS PubMed.
- H.-J. Klimisch, M. Andreae and U. Tillmann, A Systematic Approach for Evaluating the Quality of Experimental Toxicological and Ecotoxicological Data, Regul. Toxicol. Pharmacol., 1997, 25, 1–5 CrossRef CAS PubMed.
- K. R. Przybylak, J. C. Madden, M. T. D. Cronin and M. Hewitt, Assessing toxicological data quality: basic principles, existing schemes and current limitations, SAR QSAR Environ. Res., 2012, 23, 435–459 CrossRef CAS PubMed.
- R. M. Kaplan, D. A. Chambers and R. E. Glasgow, Big Data and Large Sample Size: A Cautionary Note on the Potential for Bias, Clin. Transl. Sci., 2014, 7, 342–346 CrossRef PubMed.
-
NSI: Nanotechnology Knowledge Infrastructure (NKI) Data Readiness Levels discussion draft, Nanotechnology Signature Initiative Nanotechnology Knowledge Infrastructure (NSI NKI), 2013 Search PubMed.
-
ISO/TMBG (Technical Management Board - groups), ISO/IEC Guide 98-1:2009 - Uncertainty of measurement – Part 1: Introduction to the expression of uncertainty in measurement, International Standards Organisation, 2009 Search PubMed.
- R. D. Chirico, M. Frenkel, J. W. Magee, V. Diky, C. D. Muzny, A. F. Kazakov, K. Kroenlein, I. Abdulagatov, G. R. Hardin, W. E. Acree, J. F. Brenneke, P. L. Brown, P. T. Cummings, T. W. de Loos, D. G. Friend, A. R. H. Goodwin, L. D. Hansen, W. M. Haynes, N. Koga, A. Mandelis, K. N. Marsh, P. M. Mathias, C. McCabe, J. P. O'Connell, A. Pádua, V. Rives, C. Schick, J. P. M. Trusler, S. Vyazovkin, R. D. Weir and J. Wu, Improvement of Quality in Publication of Experimental Thermophysical Property Data: Challenges, Assessment Tools, Global Implementation, and Online Support, J. Chem. Eng. Data, 2013, 58, 2699–2716 CrossRef CAS.
-
ISO Technical Committee (ISO/TC) 146/SC 4 - General aspects, ISO 20988:2007 - Air quality – Guidelines for estimating measurement uncertainty, International Standards Organisation, 2007 Search PubMed.
-
ISO Technical Committee (ISO/TC) 69/SC 6 - Measurement methods and results, ISO/TR 22971:2005 - Accuracy (trueness and precision) of measurement methods and results – Practical guidance for the use of ISO 5725-2:1994 in designing, implementing and statistically analysing interlaboratory repeatability and reproducibility results, International Standards Organisation, 2005 Search PubMed.
- V. Ruusmann and U. Maran, From data point timelines to a well curated data set, data mining of experimental data and chemical structure data from scientific articles, problems and possible solutions, J. Comput. Aided Mol. Des., 2013, 27, 583–603 CrossRef CAS PubMed.
-
B. Saha and D. Srivastava, Data quality: The other face of Big Data, in 2014 IEEE 30th International Conference on Data Engineering (ICDE), 2014, pp. 1294–1297 Search PubMed.
- XML Tutorial http://www.w3schools.com/xml/default.asp (accessed Nov 25, 2015).
- XML Schema Tutorial http://www.w3schools.com/xml/schema_intro.asp (accessed Nov 25, 2015).
- The Minimum Information for Nanomaterial Characterization (MINChar) Initiative Parameters List https://characterizationmatters.wordpress.com/parameters/ (accessed Mar 28, 2015).
-
M. D. Hoover, A. L. Miller, N. T. Lowe, A. B. Stefaniak, G. A. Day and K. D. Linch, Information Management for Nanotechnology Safety and Health, in Report
of Presentations at Plenary and Workshop Sessions and Summary of Conclusions, First International Symposium on Nanotechnology and Occupational Health, Palace Hotel, Buxton, Derbyshire, UK, 2004 Search PubMed.
- A. L. Miller, M. D. Hoover, D. M. Mitchell and B. P. Stapleton, The Nanoparticle Information Library (NIL): a prototype for linking and sharing emerging data, J. Occup. Environ. Hyg., 2007, 4, D131–D134 CrossRef CAS PubMed.
- Nanoparticle Information Library Homepage http://www.nanoparticlelibrary.net/ (accessed Dec 7, 2015).
- A. G. Oomen, P. M. J. Bos, T. F. Fernandes, K. Hund-Rinke, D. Boraschi, H. J. Byrne, K. Aschberger, S. Gottardo, F. von der Kammer, D. Kühnel, D. Hristozov, A. Marcomini, L. Migliore, J. Scott-Fordsmand, P. Wick and R. Landsiedel, Concern-driven integrated approaches to nanomaterial testing and assessment – report of the NanoSafety Cluster Working Group 10, Nanotoxicology, 2014, 8, 334–348 CrossRef PubMed.
- CODATA-VAMAS Working Group On the Description of nanomaterials, Uniform Description System for Materials on the Nanoscale v1.0, 2015.
-
C. Aberg, NanoSafety Cluster Databases Working Group. Overview and recommendation of data quality: Working draft http://www.nanosafetycluster.eu/working-groups/4-database-wg/tasks-2/2013-2.html (accessed Mar 20, 2015).
- J. H. E. Arts, M. Hadi, M.-A. Irfan, A. M. Keene, R. Kreiling, D. Lyon, M. Maier, K. Michel, T. Petry, U. G. Sauer, D. Warheit, K. Wiench, W. Wohlleben and R. Landsiedel, A decision-making framework for the grouping and testing of nanomaterials (DF4nanoGrouping), Regul. Toxicol. Pharmacol., 2015, 71, S1–S27 CrossRef CAS PubMed.
-
S. K. D. Nme, M. M. J. M. B. Eaj, S. Dthm and van B. Fa, Grouping nanomaterials : A strategy towards grouping and read-across, Rijksinstituut voor Volksgezondheid en Milieu RIVM, 2015 Search PubMed.
- A. G. Oomen, E. A. J. Bleeker, P. M. J. Bos, F. van Broekhuizen, S. Gottardo, M. Groenewold, D. Hristozov, K. Hund-Rinke, M.-A. Irfan, A. Marcomini, W. J. G. M. Peijnenburg, K. Rasmussen, A. S. Jiménez, J. J. Scott-Fordsmand, M. van Tongeren, K. Wiench, W. Wohlleben and R. Landsiedel, Grouping and Read-Across Approaches for Risk Assessment of Nanomaterials, Int. J. Environ. Res. Public Health, 2015, 12, 13415–13434 CrossRef PubMed.
- C. Hirsch, M. Roesslein, H. F. Krug and P. Wick, Nanomaterial cell interactions: are current in vitro tests reliable?, Nanomedicine, 2011, 6, 837–847 CrossRef CAS PubMed.
- J. A. Kim, C. Åberg, A. Salvati and K. A. Dawson, Role of cell cycle on the cellular uptake and dilution of nanoparticles in a cell population, Nat. Nanotechnol., 2012, 7, 62–68 CrossRef CAS PubMed.
- M. J. Ware, B. Godin, N. Singh, R. Majithia, S. Shamsudeen, R. E. Serda, K. E. Meissner, P. Rees and H. D. Summers, Analysis of the Influence of Cell Heterogeneity on Nanoparticle Dose Response, ACS Nano, 2014, 8, 6693–6700 CrossRef CAS PubMed.
- G. Maiorano, S. Sabella, B. Sorce, V. Brunetti, M. A. Malvindi, R. Cingolani and P. P. Pompa, Effects of Cell Culture Media on the Dynamic Formation of Protein−Nanoparticle Complexes and Influence on the Cellular Response, ACS Nano, 2010, 4, 7481–7491 CrossRef CAS PubMed.
- J. A. Kim, A. Salvati, C. Åberg and K. A. Dawson, Suppression of nanoparticle cytotoxicity approaching in vivo serum concentrations: limitations of in vitro testing for nanosafety, Nanoscale, 2014, 6, 14180–14184 RSC.
- DaNa Literature Criteria Checklist http://nanopartikel.info/files/methodik/DaNa-Literature-Criteria-Checklist_PDF-document.pdf (accessed Mar 20, 2015).
- DaNa Literature Criteria Checklist (revised) http://www.nanopartikel.info/files/methodik/DaNa_criteria_checklist_2015_form.pdf (accessed Nov 25, 2015).
- A. Huk, E. Izak-Nau, B. Reidy, M. Boyles, A. Duschl, I. Lynch and M. Dušinska, Is the toxic potential of nanosilver dependent on its size?, Part. Fibre Toxicol., 2014, 11, 65 CrossRef PubMed.
- S. A. Morris, S. Gaheen, M. Lijowski, M. Heiskanen and J. Klemm, Experiences in supporting the structured collection of cancer nanotechnology data using caNanoLab, Beilstein J. Nanotechnol., 2015, 6, 1580–1593 CrossRef CAS PubMed.
- Organisation for Economic Co-operation and Development, Publications in the Series on the Safety of Manufactured Nanomaterials - OECD http://www.oecd.org/science/nanosafety/publications-series-on-safety-of-manufactured-nanomaterials.htm (accessed Nov 25, 2015).
-
Guidance Manual for the Testing of Manufactured Nanomaterials: OECD Sponsorship Programme: First Revision (ENV/JM/MONO(2009)20/REV), Organisation for Economic Co-operation and Development, 2010 Search PubMed.
-
Guidance on Sample Preparation and Dosimetry for the Safety Testing of Manufactured Nanomaterials (ENV/JM/MONO(2012)40), Organisation for Economic Co-operation and Development, 2012 Search PubMed.
- International Standards Organisation, ISO Standards catalogue - ISO/TC 229 - Nanotechnologies http://www.iso.org/iso/home/store/catalogue_tc/catalogue_tc_browse.htm?commid=381983&published=on&includesc=true (accessed Nov 25, 2015).
-
ISO Technical Committee (ISO/TC) 229 - Nanotechnologies, ISO/TS 12805:2011 - Nanotechnologies—Materials specifications—Guidance on specifying nano-objects, International Standards Organisation, 2011 Search PubMed.
-
ISO Technical Committee (ISO/TC) 229 - Nanotechnologies, ISO/TR 13014:2012 - Nanotechnologies—Guidance on physico-chemical characterization of engineered nanoscale materials for toxicologic assessment, International Standards Organisation, 2012 Search PubMed.
-
ISO Technical Committee (ISO/TC) 229 - Nanotechnologies, ISO/TR 13329:2012 - Nanomaterials—Preparation of material safety data sheet (MSDS), International Standards Organisation, 2012 Search PubMed.
- M. L. Ostraat, K. Mills, K. Guzan and D. Murry, The Nanomaterial Registry: facilitating the sharing and analysis of data in the diverse nanomaterial community, Int. J. Nanomed., 2013, 8, 7 CAS.
- NanomaterialRegistry Team, RTI International, Data Compliance in a Minimal Information about Nanomaterials (Oral Presentation) https://wiki.nci.nih.gov/download/attachments/138281847/RegistryCL_nanoWG_2013-09-19_508_compliant.pdf?version=1&modificationDate=1383334268000&api=v2 (accessed Nov 25, 2015).
- B. Fadeel, A. Fornara, M. S. Toprak and K. Bhattacharya, Keeping it real: The importance of material characterization in nanotoxicology, Biochem. Biophys. Res. Commun., 2015, 468, 498–503 CrossRef PubMed.
- A. E. Nel, W. J. Parak, W. C. W. Chan, T. Xia, M. C. Hersam, C. J. Brinker, J. I. Zink, K. E. Pinkerton, D. R. Baer and P. S. Weiss, Where Are We Heading in Nanotechnology Environmental Health and Safety and Materials Characterization?, ACS Nano, 2015, 9, 5627–5630 CrossRef CAS PubMed.
-
C. O. Hendren, Y. Tian, S. Karcher, J. VanBriessen, G. V. Lowry and M. R. Wiesner, The CEINT Informatics Knowledge Commons (Poster Presentation) http://www.nseresearch.org/2014/posters/Christine_Hendren_Yuan_Tian_Sandra_Karcher_Jeanne_VanBriessen_Gregory_Lowry_Mark_Wiesner~NSF_Grantees_2014.pdf (accessed Nov 25, 2015).
-
S. Karcher and Y. Tian, Design and Applications of the CEINT Database (Oral Presentation) https://nciphub.org/resources/501 (accessed Nov 25, 2015).
- CEINT Data Integration Team Homepage http://ceint.vm.duke.edu/ (accessed Nov 25, 2015).
- D. R. Hristozov, S. Gottardo, A. Critto and A. Marcomini, Risk assessment of engineered nanomaterials: a review of available data and approaches from a regulatory perspective, Nanotoxicology, 2012, 6, 880–898 CrossRef CAS PubMed.
- DaNa2.0 Project Homepage http://www.nanopartikel.info/en/ (accessed Nov 25, 2015).
- D. Kühnel, C. Marquardt, K. Nau, H. F. Krug, B. Mathes and C. Steinbach, Environmental impacts of nanomaterials: providing comprehensive information on exposure, transport and ecotoxicity - the project DaNa2.0, Environ. Sci. Eur., 2014, 26, 21 CrossRef.
- C. Marquardt, D. Kühnel, V. Richter, H. F. Krug, B. Mathes, C. Steinbach and K. Nau, Latest research results on the effects of nanomaterials
on humans and the environment: DaNa – Knowledge Base Nanomaterials, J. Phys.: Conf. Ser., 2013, 429, 012060 CrossRef.
-
OECD, OECD Principles on Good Laboratory Practice, Organisation for Economic Co-operation and Development, Paris, 1998 Search PubMed.
- J. M. Wörle-Knirsch, K. Pulskamp and H. F. Krug, Oops They Did It Again! Carbon Nanotubes Hoax Scientists in Viability Assays, Nano Lett., 2006, 6, 1261–1268 CrossRef PubMed.
- A. Kroll, M. H. Pillukat, D. Hahn and J. Schnekenburger, Current in vitro methods in nanoparticle risk assessment: Limitations and challenges, Eur. J. Pharm. Biopharm., 2009, 72, 370–377 CrossRef CAS PubMed.
- A. Kroll, M. H. Pillukat, D. Hahn and J. Schnekenburger, Interference of engineered nanoparticles with in vitro toxicity assays, Arch. Toxicol., 2012, 86, 1123–1136 CrossRef CAS PubMed.
- R. D. Handy, N. van den Brink, M. Chappell, M. Mühling, R. Behra, M. Dušinská, P. Simpson, J. Ahtiainen, A. N. Jha, J. Seiter, A. Bednar, A. Kennedy, T. F. Fernandes and M. Riediker, Practical considerations for conducting ecotoxicity test methods with manufactured nanomaterials: what have we learnt so far?, Ecotoxicology, 2012, 21, 933–972 CrossRef CAS PubMed.
-
J. Domey, L. Haslauer, I. Grau, C. Strobel, M. Kettering and I. Hilger, Probing the Cytotoxicity of Nanoparticles: Experimental Pitfalls and Artifacts, Springer, Berlin Heidelberg, 2014, pp. 1–14 Search PubMed.
- H. F. Krug, Nanosafety Research—Are We on the Right Track?, Angew. Chem., Int. Ed., 2014, 53, 12304–12319 CAS.
- E. J. Petersen, T. B. Henry, J. Zhao, R. I. MacCuspie, T. L. Kirschling, M. A. Dobrovolskaia, V. Hackley, B. Xing and J. C. White, Identification and Avoidance of Potential Artifacts and Misinterpretations in Nanomaterial Ecotoxicity Measurements, Environ. Sci. Technol., 2014, 48, 4226–4246 CrossRef CAS PubMed.
- M. Rösslein, J. T. Elliott, M. Salit, E. J. Petersen, C. Hirsch, H. F. Krug and P. Wick, Use of Cause-and-Effect Analysis to Design a High-Quality Nanocytotoxicology Assay, Chem. Res. Toxicol., 2015, 28, 21–30 CrossRef PubMed.
- R. Guadagnini, B. H. Kenzaoui, L. Walker, G. Pojana, Z. Magdolenova, D. Bilanicova, M. Saunders, L. Juillerat-Jeanneret, A. Marcomini, A. Huk, M. Dusinska, L. M. Fjellsbø, F. Marano and S. Boland, Toxicity screenings of nanomaterials: challenges due to interference with assay processes and components of classic in vitro tests, Nanotoxicology, 2015, 9, 13–24 CrossRef CAS PubMed.
- H. L. Karlsson, S. Di Bucchianico, A. R. Collins and M. Dusinska, Can the comet assay be used reliably to detect nanoparticle-induced genotoxicity?, Environ. Mol. Mutagen., 2015, 56, 82–96 CrossRef CAS PubMed.
- M. Simkó, S. Tischler and M.-O. Mattsson, Pooling and Analysis of Published in Vitro Data: A Proof of Concept Study for the Grouping of Nanoparticles, Int. J. Mol. Sci., 2015, 16, 26211–26236 CrossRef PubMed.
- K. A. Guzan, K. C. Mills, V. Gupta, D. Murry, C. N. Scheier, D. A. Willis and M. L. Ostraat, Integration of data: the Nanomaterial Registry project and data curation, Comput. Sci. Discovery, 2013, 6, 014007 CrossRef.
- Nanomaterial Registry https://www.nanomaterialregistry.org/ (accessed Nov 25, 2015).
- MODERN Project Web Applications http://modern-fp7.biocenit.cat/tools.html (accessed Mar 28, 2015).
- MODERN Project Homepage http://modern-fp7.biocenit.cat/index.html (accessed Mar 28, 2015).
- D. G. Thomas, S. Gaheen, S. L. Harper, M. Fritts, F. Klaessig, E. Hahn-Dantona, D. Paik, S. Pan, G. A. Stafford, E. T. Freund, J. D. Klemm and N. A. Baker, ISA-TAB-Nano: A Specification for Sharing Nanomaterial Research Data in Spreadsheet-based Format, BMC Biotechnol., 2013, 13, 2 CrossRef PubMed.
- ISA-TAB-Nano Wiki https://wiki.nci.nih.gov/display/ICR/ISA-TAB-Nano (accessed Mar 27, 2015).
- R. L. Marchese Robinson, M. T. D. Cronin, A.-N. Richarz and R. Rallo, An ISA-TAB-Nano based data collection framework to support data-driven modelling of nanotoxicology, Beilstein J. Nanotechnol., 2015, 6, 1978–1999 CrossRef PubMed.
- A. Brazma, Minimum Information About a Microarray Experiment (MIAME) – Successes, Failures, Challenges, Sci. World J., 2009, 9, 420–423 CrossRef CAS PubMed.
-
Journals unite for reproducibility, Nature, 2014, 515, 7 Search PubMed.
- GigaScience | Instructions for Authors http://www.gigasciencejournal.com/authors/instructions/ (accessed Nov 25, 2015).
- A. Brazma, P. Hingamp, J. Quackenbush, G. Sherlock, P. Spellman, C. Stoeckert, J. Aach, W. Ansorge, C. A. Ball, H. C. Causton, T. Gaasterland, P. Glenisson, F. C. P. Holstege, I. F. Kim, V. Markowitz, J. C. Matese, H. Parkinson, A. Robinson, U. Sarkans, S. Schulze-Kremer, J. Stewart, R. Taylor, J. Vilo and M. Vingron, Minimum information about a microarray experiment (MIAME)—toward standards for microarray data, Nat. Genet., 2001, 29, 365–371 CrossRef CAS PubMed.
-
S. Martínez-Bartolomé, P.-A. Binz and J. Albar, The Minimal Information About a Proteomics Experiment (MIAPE) from the Proteomics Standards Initiative, in Plant Proteomics, ed. J. V. Jorrin-Novo, S. Komatsu, W. Weckwerth and S. Wienkoop, Humana Press, 2014, pp. 765–780 Search PubMed.
- J. M. Fostel, L. Burgoon, C. Zwickl, P. Lord, J. C. Corton, P. R. Bushel, M. Cunningham, L. Fan, S. W. Edwards, S. Hester, J. Stevens, W. Tong, M. Waters, C. Yang and R. Tennant, Toward a Checklist for Exchange and Interpretation of Data from a Toxicology Study, Toxicol. Sci., 2007, 99, 26–34 CrossRef CAS PubMed.
- S. Orchard, B. Al-Lazikani, S. Bryant, D. Clark, E. Calder, I. Dix, O. Engkvist, M. Forster, A. Gaulton, M. Gilson, R. Glen, M. Grigorov, K. Hammond-Kosack, L. Harland, A. Hopkins, C. Larminie, N. Lynch, R. K. Mann, P. Murray-Rust, E. Lo Piparo, C. Southan, C. Steinbeck, D. Wishart, H. Hermjakob, J. Overington and J. Thornton, Minimum information about a bioactive entity (MIABE), Nat. Rev. Drug Discovery, 2011, 10, 661–669 CrossRef CAS PubMed.
- BioSharing https://biosharing.org/ (accessed Nov 25, 2015).
- C. Kettner, D. Field, S.-A. Sansone, C. Taylor, J. Aerts, N. Binns, A. Blake, C. M. Britten, A. de Marco, J. Fostel, P. Gaudet, A. González-Beltrán, N. Hardy, J. Hellemans, H. Hermjakob, N. Juty, J. Leebens-Mack, E. Maguire, S. Neumann, S. Orchard, H. Parkinson, W. Piel, S. Ranganathan, P. Rocca-Serra, A. Santarsiero, D. Shotton, P. Sterk, A. Untergasser and P. L. Whetzel, Meeting Report from the Second ‘Minimum Information for Biological and Biomedical Investigations’
(MIBBI) workshop, Stand. Genomic Sci., 2010, 3, 259–266 CrossRef PubMed.
- D. Field, S. Sansone, E. F. DeLong, P. Sterk, I. Friedberg, P. Gaudet, S. Lewis, R. Kottmann, L. Hirschman, G. Garrity, G. Cochrane, J. Wooley, F. Meyer, S. Hunter, O. White, B. Bramlett, S. Gregurick, H. Lapp, S. Orchard, P. Rocca-Serra, A. Ruttenberg, N. Shah, C. Taylor and A. Thessen, Meeting Report: BioSharing at ISMB 2010, Stand. Genomic Sci., 2010, 3, 254–258 CrossRef PubMed.
- D. Field, S.-A. Sansone, A. Collis, T. Booth, P. Dukes, S. K. Gregurick, K. Kennedy, P. Kolar, E. Kolker, M. Maxon, S. Millard, A.-M. Mugabushaka, N. Perrin, J. E. Remacle, K. Remington, P. Rocca-Serra, C. F. Taylor, M. Thorley, B. Tiwari and J. Wilbanks, Omics Data Sharing, Science, 2009, 326, 234–236 CrossRef CAS PubMed.
- NIST Standard Reference Data Program http://www.nist.gov/srd/ (accessed Nov 30, 2015).
- D. A. Hobbs, M. S. J. Warne, S. J. Markich and D. A. Hobbs, Evaluation of criteria used to assess the quality of aquatic toxicity data, Integr. Environ. Assess. Manage., 2005, 1, 174–180 CrossRef.
-
Determining the adequacy of existing data, guideline for the HPV challenge program, U.S. Environmental Protection Agency (EPA), 1999 Search PubMed.
- J. L. Durda and D. V. Preziosi, Data Quality Evaluation of Toxicological Studies Used to Derive Ecotoxicological Benchmarks, Hum. Ecol. Risk Assess. Int. J., 2000, 6, 747–765 CrossRef CAS.
- K. Schneider, M. Schwarz, I. Burkholder, A. Kopp-Schneider, L. Edler, A. Kinsner-Ovaskainen, T. Hartung and S. Hoffmann, ‘ToxRTool’, a new tool to assess the reliability of toxicological data, Toxicol. Lett., 2009, 189, 138–144 CrossRef CAS PubMed.
- A. Beronius, L. Molander, C. Rudén and A. Hanberg, Facilitating the use of non-standard in vivo studies in health risk assessment of chemicals: a proposal to improve evaluation criteria and reporting, J. Appl. Toxicol., 2014, 34, 607–617 CrossRef CAS PubMed.
- M. Ågerstrand, M. Breitholtz and C. Rudén, Comparison of four different methods for reliability evaluation of ecotoxicity data: a case study of non-standard test data used in environmental risk assessments of pharmaceutical substances, Environ. Sci. Eur., 2011, 23, 17 CrossRef.
- N. Jeliazkova, C. Chomenidis, P. Doganis, B. Fadeel, R. Grafström, B. Hardy, J. Hastings, M. Hegi, V. Jeliazkov, N. Kochev, P. Kohonen, C. R. Munteanu, H. Sarimveis, B. Smeets, P. Sopasakis, G. Tsiliki, D. Vorgrimmler and E. Willighagen, The eNanoMapper database for nanomaterial safety information, Beilstein J. Nanotechnol., 2015, 6, 1609–1634 CrossRef CAS PubMed.
- ToxRTool - Toxicological data Reliability Assessment Tool—EURL ECVAM https://eurl-ecvam.jrc.ec.europa.eu/about-ecvam/archive-publications/toxrtool (accessed Nov 26, 2015).
- L. Yang, D. Neagu, M. T. D. Cronin, M. Hewitt, S. J. Enoch, J. C. Madden and K. Przybylak, Towards a Fuzzy Expert System on Toxicological Data Quality Assessment, Mol. Inf., 2013, 32, 65–78 CrossRef CAS.
- J. P. Myers, F. S. vom Saal, B. T. Akingbemi, K. Arizono, S. Belcher, T. Colborn, I. Chahoud, D. A. Crain, F. Farabollini, L. J. Guillette, T. Hassold, S. Ho, P. A. Hunt, T. Iguchi, S. Jobling, J. Kanno, H. Laufer, M. Marcus, J. A. McLachlan, A. Nadal, J. Oehlmann, N. Olea, P. Palanza, S. Parmigiani, B. S. Rubin, G. Schoenfelder, C. Sonnenschein, A. M. Soto, C. E. Talsness, J. A. Taylor, L. N. Vandenberg, J. G. Vandenbergh, S. Vogel, C. S. Watson, W. V. Welshons and R. T. Zoeller, Why public health agencies cannot depend on good laboratory practices as a criterion for selecting data: the case of bisphenol A, Environ. Health Perspect., 2009, 117, 309–315 CrossRef CAS PubMed.
- Clinical and Biospecimen XML Validation - TCGA - National Cancer Institute - Confluence Wiki https://wiki.nci.nih.gov/display/TCGA/Clinical+and+Biospecimen+XML+Validation (accessed Nov 26, 2015).
- P. Rocca-Serra, M. Brandizi, E. Maguire, N. Sklyar, C. Taylor, K. Begley, D. Field, S. Harris, W. Hide, O. Hofmann, S. Neumann, P. Sterk, W. Tong and S.-A. Sansone, ISA software suite: supporting standards-compliant experimental annotation and enabling curation at the community level, Bioinformatics, 2010, 26, 2354–2356 CrossRef CAS PubMed.
- ISA-Tools Software http://www.isa-tools.org/software-suite/ (accessed Jul 21, 2015).
- M. Watson, Quality assessment and control of high-throughput sequencing data, Front. Genet., 2014, 5, 235 Search PubMed.
- R. M. Leggett, R. H. Ramirez-Gonzalez, B. Clavijo, D. Waite and R. P. Davey, Sequencing quality assessment tools to enable data-driven informatics for high throughput genomics, Front. Genet., 2013, 4, 288 Search PubMed.
- K. H. Paszkiewicz, A. Farbos, P. O'Neill and K. Moore, Quality control on the frontier, Front. Genet., 2014, 5, 157 Search PubMed.
- M. Lohse, A. M. Bolger, A. Nagel, A. R. Fernie, J. E. Lunn, M. Stitt and B. Usadel, RobiNA: a user-friendly, integrated software solution for RNA-Seq-based transcriptomics, Nucleic Acids Res., 2012, 40, W622–W627 CrossRef CAS PubMed.
- H. Li, B. Handsaker, A. Wysoker, T. Fennell, J. Ruan, N. Homer, G. Marth, G. Abecasis, R. Durbin and 1000 Genome Project Data Processing Subgroup, The Sequence Alignment/Map format and SAMtools, Bioinformatics, 2009, 25, 2078–2079 CrossRef CAS PubMed.
- (IUCr) IUCr Journals - details of checkCIF/PLATON tests for IUCr Journals http://journals.iucr.org/services/cif/checkcif.html (accessed Nov 26, 2015).
- A. L. Spek, Structure validation in chemical crystallography, Acta Crystallogr., Sect. D: Biol. Crystallogr., 2009, 65, 148–155 CrossRef CAS PubMed.
- S. R. Hall, F. H. Allen and I. D. Brown, The crystallographic information file (CIF): a new standard archive file for crystallography, Acta Crystallogr., Sect. A: Fundam. Crystallogr., 1991, 47, 655–685 CrossRef.
- R. Shankar, H. Parkinson, T. Burdett, E. Hastings, J. Liu, M. Miller, R. Srinivasa, J. White, A. Brazma, G. Sherlock, C. J. Stoeckert and C. A. Ball, Annotare—a tool for annotating high-throughput biomedical investigations and resulting data, Bioinformatics, 2010, 26, 2470–2471 CrossRef CAS PubMed.
- About TCGA - TCGA - National Cancer Institute - Confluence Wiki https://wiki.nci.nih.gov/display/TCGA/About+TCGA (accessed Nov 26, 2015).
- S.-A. Sansone, P. Rocca-Serra, M. Brandizi, A. Brazma, D. Field, J. Fostel, A. G. Garrow, J. Gilbert, F. Goodsaid, N. Hardy, P. Jones, A. Lister, M. Miller, N. Morrison, T. Rayner, N. Sklyar, C. Taylor, W. Tong, G. Warner and S. Wiemann, The First RSBI (ISA-TAB) Workshop: ‘Can a Simple Format Work for Complex Studies?’, OMICS: J. Integr. Biol., 2008, 12, 143–149 CrossRef CAS PubMed.
-
P. Rocca-Serra, S.-A. Sansone, M. Brandizi, D. Hancock, S. Harris, A. Lister, M. Miller, K. O'Neill, C. Taylor and W. Tong, Specification documentation: release candidate 1, ISA-TAB 1.0 http://isatab.sourceforge.net/docs/ISA-TAB_release-candidate-1_v1.0_24nov08.pdf (accessed Jul 21, 2015).
- S.-A. Sansone, P. Rocca-Serra, D. Field, E. Maguire, C. Taylor, O. Hofmann, H. Fang, S. Neumann, W. Tong, L. Amaral-Zettler, K. Begley, T. Booth, L. Bougueleret, G. Burns, B. Chapman, T. Clark, L.-A. Coleman, J. Copeland, S. Das, A. de Daruvar, P. de Matos, I. Dix, S. Edmunds, C. T. Evelo, M. J. Forster, P. Gaudet, J. Gilbert, C. Goble, J. L. Griffin, D. Jacob, J. Kleinjans, L. Harland, K. Haug, H. Hermjakob, S. J. H. Sui, A. Laederach, S. Liang, S. Marshall, A. McGrath, E. Merrill, D. Reilly, M. Roux, C. E. Shamu, C. A. Shang, C. Steinbeck, A. Trefethen, B. Williams-Jones, K. Wolstencroft, I. Xenarios and W. Hide, Toward interoperable bioscience data, Nat. Genet., 2012, 44, 121–126 CrossRef CAS PubMed.
- A. Mikolajczyk, A. Gajewicz, B. Rasulev, N. Schaeublin, E. Maurer-Gardner, S. Hussain, J. Leszczynski and T. Puzyn, Zeta Potential for Metal Oxide Nanoparticles: A Predictive Model Developed by a Nano-Quantitative Structure–Property Relationship Approach, Chem. Mater., 2015, 27, 2400–2407 CrossRef CAS.
- A. Gajewicz, N. Schaeublin, B. Rasulev, S. Hussain, D. Leszczynska, T. Puzyn and J. Leszczynski, Towards understanding mechanisms governing cytotoxicity of metal oxides nanoparticles: Hints from nano-QSAR studies, Nanotoxicology, 2015, 9, 313–325 CrossRef CAS PubMed.
- P. Hole, K. Sillence, C. Hannell, C. M. Maguire, M. Roesslein, G. Suarez, S. Capracotta, Z. Magdolenova, L. Horev-Azaria, A. Dybowska, L. Cooke, A. Haase, S. Contal, S. Manø, A. Vennemann, J.-J. Sauvain, K. C. Staunton, S. Anguissola, A. Luch, M. Dusinska, R. Korenstein, A. C. Gutleb, M. Wiemann, A. Prina-Mello, M. Riediker and P. Wick, Interlaboratory comparison of size measurements on nanoparticles using nanoparticle tracking analysis (NTA), J. Nanopart. Res., 2013, 15, 1–12 CrossRef PubMed.
- W.-S. Cho, R. Duffin, F. Thielbeer, M. Bradley, I. L. Megson, W. MacNee, C. A. Poland, C. L. Tran and K. Donaldson, Zeta Potential and Solubility to Toxic Ions as Mechanisms of Lung Inflammation Caused by Metal/Metal Oxide Nanoparticles, Toxicol. Sci., 2012, 126, 469–477 CrossRef CAS PubMed.
- I. Lynch and K. A. Dawson, Protein-nanoparticle interactions, Nano Today, 2008, 3, 40–47 CrossRef CAS.
- S. Milani, F. Baldelli Bombelli, A. S. Pitek, K. A. Dawson and J. Rädler, Reversible versus Irreversible Binding of Transferrin to Polystyrene Nanoparticles: Soft and Hard Corona, ACS Nano, 2012, 6, 2532–2541 CrossRef CAS PubMed.
- M. P. Monopoli, C. Åberg, A. Salvati and K. A. Dawson, Biomolecular coronas provide the biological identity of nanosized materials, Nat. Nanotechnol., 2012, 7, 779–786 CrossRef CAS PubMed.
- E. Casals, T. Pfaller, A. Duschl, G. J. Oostingh and V. Puntes, Time Evolution of the Nanoparticle Protein Corona, ACS Nano, 2010, 4, 3623–3632 CrossRef CAS PubMed.
- R. F. Domingos, C. Franco and J. P. Pinheiro, The role of charged polymer coatings of nanoparticles on the speciation and fate of metal ions in the environment, Environ. Sci. Pollut. Res., 2014, 22, 2900–2906 CrossRef PubMed.
- Y. Ma and V. Chechik, Aging of Gold Nanoparticles: Ligand Exchange with Disulfides, Langmuir, 2011, 27, 14432–14437 CrossRef CAS PubMed.
- C. E. Nanayakkara, J. Pettibone and V. H. Grassian, Sulfur dioxide adsorption and photooxidation on isotopically-labeled titanium dioxide nanoparticle surfaces: roles of surface hydroxyl groups and adsorbed water in the formation and stability of adsorbed sulfite and sulfate, Phys. Chem. Chem. Phys., 2012, 14, 6957–6966 RSC.
- D. M. Mitrano, S. Motellier, S. Clavaguera and B. Nowack, Review of nanomaterial aging and transformations through the life cycle of nano-enhanced products, Environ. Int., 2015, 77, 132–147 CrossRef CAS PubMed.
- E. Izak-Nau, A. Huk, B. Reidy, H. Uggerud, M. Vadset, S. Eiden, M. Voetz, M. Himly, A. Duschl, M. Dusinska and I. Lynch, Impact of storage conditions and storage time on silver nanoparticles’ physicochemical properties and implications for their biological effects, RSC Adv., 2015, 5, 84172–84185 RSC.
- K. Donaldson and C. A. Poland, Nanotoxicity: challenging the myth of nano-specific toxicity, Curr. Opin. Biotechnol., 2013, 24, 724–734 CrossRef CAS PubMed.
- J. M. Cohen, J. G. Teeguarden and P. Demokritou, An integrated approach for the in vitro dosimetry of engineered nanomaterials, Part. Fibre Toxicol., 2014, 11, 20 CrossRef PubMed.
- E. J. Petersen, S. A. Diamond, A. J. Kennedy, G. G. Goss, K. Ho, J. Lead, S. K. Hanna, N. B. Hartmann, K. Hund-Rinke, B. Mader, N. Manier, P. Pandard, E. R. Salinas and P. Sayre, Adapting OECD Aquatic Toxicity Tests for Use with Manufactured Nanomaterials: Key Issues and Consensus Recommendations, Environ. Sci. Technol., 2015, 49, 9532–9547 CrossRef CAS PubMed.
- E. C. Cho, Q. Zhang and Y. Xia, The effect of sedimentation and diffusion on cellular uptake of gold nanoparticles, Nat. Nanotechnol., 2011, 6, 385–391 CrossRef CAS PubMed.
- C. D. Walkey, J. B. Olsen, F. Song, R. Liu, H. Guo, D. W. H. Olsen, Y. Cohen, A. Emili and W. C. W. Chan, Protein Corona Fingerprinting Predicts the Cellular Interaction of Gold and Silver Nanoparticles, ACS Nano, 2014, 8, 2439–2455 CrossRef CAS PubMed.
- L. M. Gilbertson, J. B. Zimmerman, D. L. Plata, J. E. Hutchison and P. T. Anastas, Designing nanomaterials to maximize performance and minimize undesirable implications guided by the Principles of Green Chemistry, Chem. Soc. Rev., 2015, 44, 5758–5777 RSC.
- Sample Processing and Separation Techniques Ontology (SEP): Definition of Batch Identifier http://bioportal.bioontology.org/ontologies/SEP?p=classes&conceptid=http%3A%2F%2Fpurl.obolibrary.org%2Fobo%2Fsep_00015 (accessed Nov 26, 2015).
- ISA-TAB-Nano Wiki: Material File Documentation https://wiki.nci.nih.gov/display/ICR/Material (accessed Mar 28, 2015).
- H. Zhang, D. R. Dunphy, X. Jiang, H. Meng, B. Sun, D. Tarn, M. Xue, X. Wang, S. Lin, Z. Ji, R. Li, F. L. Garcia, J. Yang, M. L. Kirk, T. Xia, J. I. Zink, A. Nel and C. J. Brinker, Processing Pathway Dependence of Amorphous Silica Nanoparticle Toxicity: Colloidal vs Pyrolytic, J. Am. Chem. Soc., 2012, 134, 15790–15804 CrossRef CAS PubMed.
- S. H. Doak, B. Manshian, G. J. S. Jenkins and N. Singh, In vitro genotoxicity testing strategy for nanomaterials and the adaptation of current OECD guidelines, Mutat. Res., Genet. Toxicol. Environ. Mutagen., 2012, 745, 104–111 CrossRef CAS PubMed.
- M. Fenech, Cytokinesis-block micronucleus cytome assay, Nat. Protocols, 2007, 2, 1084–1104 CAS.
-
V. A. Hackley and C. F. Ferraris, NIST Recommended Practice Guide: The Use of Nomenclature in Dispersion Science and Technology, National Institute of Standards and Technology, 2001 Search PubMed.
-
Report of the OECD Expert Meeting on the Physical Chemical Properties of Manufactured Nanomaterials and Test Guidelines, Organisation for Economic Co-operation and Development, 2014.
-
Dynamic light scattering - common terms defined (Version 1 Whitepaper), Malvern Instruments Ltd., 2014 Search PubMed.
- M. Baalousha and J. R. Lead, Rationalizing Nanomaterial Sizes Measured by Atomic Force Microscopy, Flow Field-Flow Fractionation, and Dynamic Light Scattering: Sample Preparation, Polydispersity, and Particle Structure, Environ. Sci. Technol., 2012, 46, 6134–6142 CrossRef CAS PubMed.
-
D. L. Kaiser and R. L. Watters, National Institute of Standards and Technology (NIST) Certificate of Analysis for Standard Reference Material® 1898 Titanium Dioxide Nanomaterial, National Institute of Standards and Technology (NIST), 2012 Search PubMed.
- A. Dhawan and V. Sharma, Toxicity assessment of nanomaterials: methods and challenges, Anal. Bioanal. Chem., 2010, 398, 589–605 CrossRef CAS PubMed.
- A. M. Horst, R. Vukanti, J. H. Priester and P. A. Holden, An Assessment of Fluorescence- and Absorbance-Based Assays to Study Metal-Oxide Nanoparticle ROS Production and Effects on Bacterial Membranes, Small, 2013, 9, 1753–1764 CrossRef CAS PubMed.
- K. J. Ong, T. J. MacCormack, R. J. Clark, J. D. Ede, V. A. Ortega, L. C. Felix, M. K. M. Dang, G. Ma, H. Fenniri, J. G. C. Veinot and G. G. Goss, Widespread Nanoparticle-Assay Interference: Implications for Nanotoxicity Testing, PLoS One, 2014, 9, e90650 Search PubMed.
- I. V. Vrček, I. Pavičić, T. Crnković, D. Jurašin, M. Babič, D. Horák, M. Lovrić, L. Ferhatović, M. Ćurlin and S. Gajović, Does surface coating of metallic nanoparticles modulate their interference with in vitro assays?, RSC Adv., 2015, 5, 70787–70807 RSC.
-
OECD, Test No. 203: Fish, Acute Toxicity Test, Organisation for Economic Co-operation and Development, OECD Guidelines for the Testing of Chemicals, Section 2, OECD Publishing, Paris, 1992 Search PubMed.
-
Preliminary Review of OECD Test Guidelines for their Applicability to Manufactured Nanomaterials (ENV/JM/MONO(2009)21), Organisation for Economic Co-operation and Development, 2009 Search PubMed.
- D. Kühnel and C. Nickel, The OECD expert meeting on ecotoxicology and environmental fate—Towards the development of improved OECD guidelines for the testing of nanomaterials, Sci. Total Environ., 2014, 472, 347–353 CrossRef PubMed.
-
Ecotoxicology and Environmental Fate of Manufactured Nanomaterials: Test Guidelines (ENV/JM/MONO(2014)1), Organisation for Economic Co-operation and Development, 2014 Search PubMed.
-
Genotoxicity of Manufactured Nanomaterials: Report of the OECD Expert Meeting, Organisation for Economic Co-operation and Development, 2014.
- H. Bouwmeester, I. Lynch, H. J. P. Marvin, K. A. Dawson, M. Berges, D. Braguer, H. J. Byrne, A. Casey, G. Chambers, M. J. D. Clift, G. Elia, T. F. Fernandes, L. B. Fjellsbø, P. Hatto, L. Juillerat, C. Klein, W. G. Kreyling, C. Nickel, M. Riediker and V. Stone, Minimal analytical characterization of engineered nanomaterials needed for hazard assessment in biological matrices, Nanotoxicology, 2011, 5, 1–11 CrossRef CAS PubMed.
- D. B. Warheit, S. C. Brown and E. M. Donner, Acute and subchronic oral toxicity studies in rats with nanoscale and pigment grade titanium dioxide particles, Food Chem. Toxicol., 2015, 84, 208–224 CrossRef CAS PubMed.
- D. B. Warheit and E. M. Donner, How meaningful are risk determinations in the absence of a complete dataset? Making the case for publishing standardized test guideline and ‘no effect’ studies for evaluating the safety of nanoparticulates versus spurious ‘high effect’ results from single investigative studies, Sci. Technol. Adv. Mater., 2015, 16, 034603 CrossRef.
- I. L. Gunsolus and C. L. Haynes, Analytical Aspects of Nanotoxicology, Anal. Chem., 2016, 88, 451–479 CrossRef PubMed.
- T. Gebel, H. Foth, G. Damm, A. Freyberger, P.-J. Kramer, W. Lilienblum, C. Röhl, T. Schupp, C. Weiss, K.-M. Wollin and J. G. Hengstler, Manufactured nanomaterials: categorization and approaches to hazard assessment, Arch. Toxicol., 2014, 88, 2191–2211 CrossRef CAS PubMed.
- M. J. D. Clift, P. Gehr and B. Rothen-Rutishauser, Nanotoxicology: a perspective and discussion of whether or not in vitro testing is a valid alternative, Arch. Toxicol., 2011, 85, 723–731 CrossRef CAS PubMed.
- A. Gallud and B. Fadeel, Keeping it small: towards a molecular definition of nanotoxicology, Eur. J. Nanomed., 2015, 7, 143–151 CAS.
- I. Lynch, I. L. Feitshans and M. Kendall, Bio-nano interactions: new tools, insights and impacts’: summary of the Royal Society discussion meeting, Philos. Trans. R. Soc. London, Ser. B, 2015, 370, 20140162 CrossRef PubMed.
- O. Bondarenko, K. Juganson, A. Ivask, K. Kasemets, M. Mortimer and A. Kahru, Toxicity of Ag, CuO and ZnO nanoparticles to selected environmentally relevant test organisms and mammalian cells in vitro: a critical review, Arch. Toxicol., 2013, 87, 1181–1200 CrossRef CAS PubMed.
- P. A. Holden, J. P. Schimel and H. A. Godwin, Five reasons to use bacteria when assessing manufactured nanomaterial environmental hazards and fates, Curr. Opin. Biotechnol., 2014, 27, 73–78 CrossRef CAS PubMed.
- F. Laborda, E. Bolea and J. Jiménez-Lamana, Single particle inductively coupled plasma mass spectrometry for the analysis of inorganic engineered nanoparticles in environmental samples, Trends Environ. Anal. Chem., 2016, 9, 15–23 CrossRef.
- B. Nowack, M. Baalousha, N. Bornhöft, Q. Chaudhry, G. Cornelis, J. Cotterill, A. Gondikas, M. Hassellöv, J. Lead, D. M. Mitrano, F. von der Kammer and T. Wontner-Smith, Progress towards the validation of modeled environmental concentrations of engineered nanomaterials by analytical measurements, Environ. Sci.: Nano, 2015, 2, 421–428 RSC.
- A. R. Badireddy, M. R. Wiesner and J. Liu, Detection, Characterization, and Abundance of Engineered Nanoparticles in Complex Waters by Hyperspectral Imagery with Enhanced Darkfield Microscopy, Environ. Sci. Technol., 2012, 46, 10081–10088 CrossRef CAS PubMed.
- S. Lee, X. Bi, R. B. Reed, J. F. Ranville, P. Herckes and P. Westerhoff, Nanoparticle Size Detection Limits by Single Particle ICP-MS for 40 Elements, Environ. Sci. Technol., 2014, 48, 10291–10300 CrossRef CAS PubMed.
- H. Bouwmeester, P. Brandhoff, H. J. P. Marvin, S. Weigel and R. J. B. Peters, State of the safety assessment and current use of nanomaterials in food and food production, Trends Food Sci. Technol., 2014, 40, 200–210 CrossRef CAS.
- K. D. Grieger, J. Harrington and N. Mortensen, Prioritizing research needs for analytical techniques suited for engineered nanomaterials in food, Trends Food Sci. Technol., 2016, 50, 219–229 CrossRef CAS.
Footnote |
† Electronic supplementary information (ESI) available: (1) Detailed information regarding issues raised in the main text; (2) original survey responses. See DOI: 10.1039/c5nr08944a |
|
This journal is © The Royal Society of Chemistry 2016 |
Click here to see how this site uses Cookies. View our privacy policy here.