DOI:
10.1039/C6LC00118A
(Paper)
Lab Chip, 2016,
16, 1909-1916
On-chip analysis, indexing and screening for chemical producing bacteria in a microfluidic static droplet array†
Received
26th January 2016
, Accepted 8th April 2016
First published on 8th April 2016
Abstract
Economic production of chemicals from microbes necessitates development of high-producing strains and an efficient screening technology is crucial to maximize the effect of the most popular strain improvement method, the combinatorial approach. However, high-throughput screening has been limited for assessment of diverse intracellular metabolites at the single-cell level. Herein, we established a screening platform that couples a microfluidic static droplet array (SDA) and an artificial riboswitch to analyse intracellular metabolite concentration from single microbial cells. Using this system, we entrapped single Escherichia coli cells in SDA to measure intracellular L-tryptophan concentrations. It was validated that intracellular L-tryptophan concentration can be evaluated by the fluorescence from the riboswitch. Moreover, high-producing strains were successfully screened from a mutagenized library, exhibiting up to 145% productivity compared to its parental strain. This platform will be widely applicable to strain improvement for diverse metabolites by developing new artificial riboswitches.
Introduction
Chemical production from renewable resources such as biomass is known to be the most promising way to avoid petroleum consumption.1 Efficient microbial strains are essential to accomplish this economic process for the production of chemicals from biomass, but naturally occurring strains do not generally perform economical production in terms of the productivity and yield.2,3 Therefore, strain improvement is necessary to develop an efficient bioprocess. Due to the complexity of biological systems,4 microbial strains are not easily redesigned by rational approaches. Accordingly, the combinatorial approach, which consists of random mutagenesis and screening, is currently known as the most popular strain improvement method.5–7 The success of combinatorial engineering, however, still depends on the screening method because it determines the phenotype and performance of the screened variants.8,9 Technology for the screening of metabolite-producing microbial cells has requirements such as high-throughput and wide applicability to diverse molecules.8,10,11 In addition, it would be preferable to evaluate the innate cellular performance in regards to metabolite production with responses to the culture conditions.
Cellular performance for the production of metabolites has been conventionally measured by the accumulation of the target metabolites in the culture medium after several hours of cultivation. However, metabolite accumulation outside the cell does not properly represent the cellular physiology for metabolite production at the single-cell level of analysis because the metabolite concentration outside the cell varies depending on the medium volume, and the reactions visualizing the metabolites are not generally robust enough to evaluate cell physiology for metabolite production. Therefore, in order to evaluate the cellular performance for the production of metabolites at the single-cell level, intracellular metabolite measurement is the more reliable way.
For this reason, dozens of sensors based on biomolecules have been developed for the detection and measurement of intracellular metabolites.12–15 Metabolite sensors are generally composed of biomolecules such as riboswitches and transcription factors that specifically bind to the target metabolite and regulate gene expression upon the binding event. These metabolite biosensors were successfully applied to diverse applications from elucidation of the regulation mechanism of metabolic pathways to the evolution of high metabolite producing strains.16–18 Considering that the creation of a novel riboswitch for the new target molecule is rather straightforward, biosensor-associated measurement of intracellular metabolite should be regarded as a promising tool for screening with wide applicability.19–21
Signals that reflect intracellular metabolite concentration could be monitored by fluorescence-activated cell sorting (FACS). This method is a well-established technology for analysing intracellular fluorescence from a large number of cells and separating them at the single-cell level. Nonetheless, the viability of the sorted cells can be negatively affected by numerous factors of FACS instruments, such as mechanical and physiological stresses during sorting, making recovery of the desired cells difficult.23
To overcome the aforementioned obstacles, microfluidic fluorescence-activated droplet sorting (FADS) systems have been developed because they provide rather mild conditions for the cells in comparison with FACS.24–26 FADS is able to generate and monitor picoliter-sized droplets with encapsulated cells to analyse consumption or production of specific molecules by detection of fluorescence. In this platform, however, it is difficult to measure the intracellular fluorescence signals directly from single cells because the size of a microbial cell is very small to be observed in short period of time in which a droplet passes by. Moreover, the types of metabolites that can be detected using this system are restricted to the molecules that can be linked to fluorescent assay reactions, and consequently, applications for this system are limited. Recently, we have reported several microfluidic static droplet arrays (SDA) as a universal analytical platform by combining hydrodynamics and microvalve technology for generation, manipulation, analysis, and retrieval of multiple microdroplets in parallel.27–30 This SDA is able to analyse at the single-cell level in real time since it deals with static droplets with fixed positions. In addition, selective release and retrieval of desired droplets encapsulating cells and reusability of the system are also attractive features as a screening method.31,32 We expect that the SDA will solve the weak points of previously developed FADS.
In this study, we combined the selectively releasable SDA platform with an artificial riboswitch to set up an efficient high-throughput screening system that enables quantification of intracellular metabolite and retrieval of single-cell encapsulated droplets. The SDA device is able to generate the droplet array with single Escherichia coli cells by individual manipulation of droplets. Furthermore, the droplets containing the desired E. coli cells were selectively extracted after on-chip analysis. The artificial riboswitch that responds to L-tryptophan allows visualization of the intracellular metabolite and also differentiation of the metabolite concentration on the SDA chip. By integrating the two technologies, we could perform an efficient screening to obtain E. coli strains that have an improved L-tryptophan productivity from a mutant library with diversified metabolic flux.
Experimental section
Chemicals and reagents
Phusion DNA polymerase and restriction endonucleases were purchased from New England Biolabs (Beverly, MA, USA). Oligonucleotides were synthesized by Macrogen (Seoul, Korea) and are listed in Table S1.† Plasmids were prepared using AccuPrep Nano-Plus Plasmid Mini Extraction Kit (Bioneer, Daejeon, Korea). PCR-amplified DNA fragments were purified using the Expin Gel SV kit (GeneAll Biotechnology, Seoul, Korea). Counter-Selection BAC Modification Kit for generation of a genomic mutant library was acquired from Gene Bridges GmbH (Heidelberg, Germany). Ingredients of cell culture media for plasmid cloning and chromosomal manipulation were obtained from BD Biosciences (Sparks, MD, USA). Other reagents were purchased from Sigma (St. Louis, MO, USA) unless otherwise indicated. The bacterial strains and plasmids used in this study are listed in Table S2.†
Bacterial strains and plasmids
pMD20-FKF(f2161) was constructed by subcloning of the FRT(f2161)-KanR-FRT(f2161) fragment that was amplified by Phusion polymerase with the f2161_F/R into pMD20 T-vector. pTrpEfbr was constructed by subcloning of the trpE fragment amplified from the genomic DNA of Salmonella typhimurium with styp_trpE_F/R primers into pGFKF2. Point mutation that confers feedback-resistance was generated by PCR-based site-directed mutagenesis using mut_trpE_F/R primers. To construct pRpsLneo, the rpsL-neo cassette provided from the Counter-Selection BAC Modification Kit was amplified with rneo_F/R and subcloned to the pMD20 T-vector.
All chromosomal work was done by a Red recombination system using pKD46 and pCP20. To construct the base strain (SJT0) for L-tryptophan production, rpsL A128G mutation was generated by direct transformation and recombination of rpsL A128G oligonucleotide to E. coli W3110. The parental strain (SJT1) was constructed by, first, deletion of trpR, tnaA, trpE, and aroG from SJT0 by insertion of FRT-KanR-FRT fragments amplified with del_trpR_F/R, del_tnaA_F/R, del_trpE_F/R, del_aroG_F/R, respectively. Three different versions of FRT sequences were employed to avoid unwanted interactions between FRT sequences on the chromosome. The wild type FRT sequence was applied for deletion of aroG, the F72 mutant for trpR and trpE, and the F2161 mutant for tnaA, respectively. Then, the native aroG was replaced by the PCR fragment of pAroGfbr amplified with ins_aroG_F/R and the native trpE by the PCR fragment of pTrpEfbr amplified with del_trpE_F and ins_trpE_R. Finally, the native promoter of tktA was replaced by PBBa_J23100 and a redesigned 5′-untranslated region (UTR) using the FRT-KanR-FRT (WT) fragment amplified with ins_tktA_F/R.
The random UTR sequence library for chromosomal ppsA was generated utilizing the Counter-Selection BAC Modification Kit. The rpsL-neo cassette was amplified from pRpsLneo with pr_ppsA_F/R and recombined to the promoter-UTR region of ppsA of the SJT1 strain. The successful recombinant was named SJT10 and was resistant to kanamycin while sensitive to streptomycin due to the coexistence of mutant and wild-type rpsL. Then the oligonucleotide ppsA_UTR Library was directly transformed and recombined to the SJT10 to replace the rpsL-neo cassette. The recombinants were grown on streptomycin-supplemented agar plates since the wild type rpsL was removed by the recombination, and the resistance to streptomycin was conferred on the cell by the mutant rpsL in successful recombinants.33 Then, the pTrpRibo plasmid was transformed to the resulting recombinants for construction of the library to be screened on a chip.
Fabrication of the SDA device
The SDA device was fabricated by a multi-step soft lithography process to integrate microvalves using polydimethylsiloxane (PDMS) (Sylgard 184, Dow Corning, MI, USA). Three different master molds were fabricated by photolithography to generate the fluidic, control, and block layers. The fluidic layer master was created on a silicon wafer by spinning an SU-8 3025 negative photoresist (Microchem Corp, Newton, MA) at a thickness of 20 μm and patterned by photolithography. The control layer and block layer master were patterned in the same way and the thickness was 20 μm. The mixture of PDMS prepolymer and its curing agent (10
:
1 ratio) were poured onto the master mold for the fluidic layer, cured in a 65 °C oven for 60 min, and peeled off from the master mold; injection holes were then punched into the fluidic layer. The bottom pneumatic microvalve layer was formed on the master mold by spin-coating the PDMS mixture (20
:
1 ratio of the prepolymer and curing agent) at 3000 rpm for 1 min and curing at 65 °C for 45 min. Then, the fluidic layer was aligned over the microvalve layer and cured in a 65 °C oven for 50 min. After that, the two-layered PDMS slab was attached onto the master mold for the block layer and cured in a 65 °C oven overnight. The PDMS chip with the fluidic, control, and block layers were peeled off from the master mold, and some holes were generated to connect the inlet ports to the microvalve channels using biopsy punch. Finally, the PDMS chip was bonded to a glass slide by plasma treatment for 1 min and then kept in a 65 °C oven overnight to improve the bonding strength.
Operation of the SDA device
The microfluidic device was operated by solenoid valve manifolds and a controller (Stanford Microfluidics Foundry, USA). A USB controller-mounted computer controlled the switching of the 3-way solenoid valves of the manifolds. A custom-built LabVIEW program was used to switch the individual on-chip microvalves. Nitrogen gas was used to inject the reagents into the microchannels and microvalves. Before operating the device, we filled some distilled water in the microvalve channels under 0.30 MPa. FC-40 oil with 30% 1H,1H,2H,2H-perfluorooctanol as the continuous phase and aqueous solutions for the dispersed phase were injected into the microchannels. First, we optimized the droplet generating conditions with all control valves turned off. The experimental pressure was 0.10 MPa and 0.12 MPa for oil and aqueous phase injections, respectively. For trap-and-release of droplets, 0.2 MPa and 0.3 MPa were applied to the control and block valves. To handle a 3 × 8 array, we used eight and three inlet ports to control and block the valves. Control valves were expanded to entrap the generated droplet in array chambers and the three block valves barricaded each array row to freeze the control valves on demand so that a desired droplet can selectively be extracted from the droplet array without any interference from other droplets (Fig. 1A).
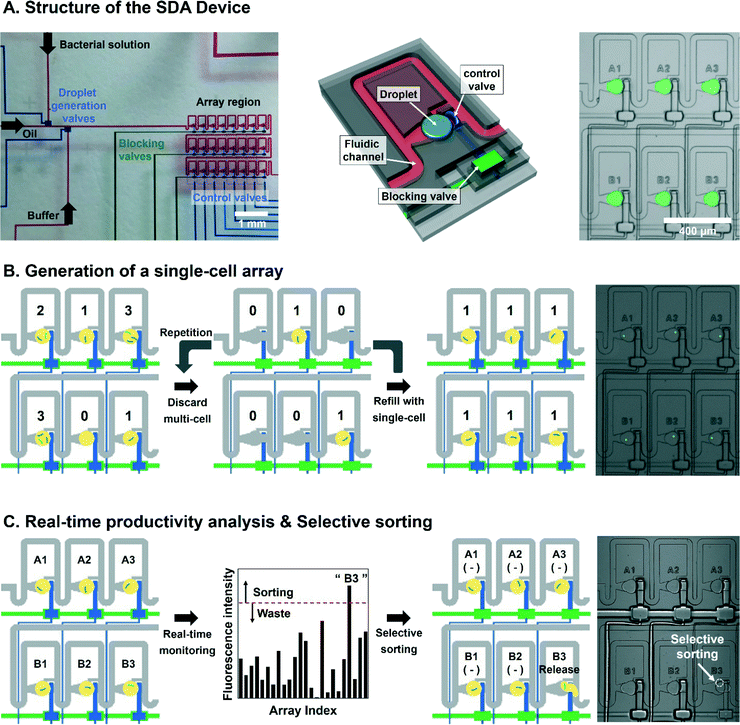 |
| Fig. 1 Illustration of a SDA device for single cell sorting. (A) Macro-structure of the SDA device and 3D rendering image with three valve types; droplet generation valve, control valve, and block valve. (B) Sequential scheme of repetitive manipulation for generating a single-cell array: droplet filling, wasting droplet with multi-cells, and droplet re-filling. (C) Procedure for the sorting of high chemical-producing cells by selective releasing of droplets based on area-normalized fluorescence intensity. | |
Screening of the bacteria library
The bacterial solution was diluted to an appropriate optical density (O.D.600) and introduced into the sample inlet on the fluidic channel, and the other sample channel was filled with PBS buffer. Single-cell encapsulated droplets with a diameter of 100 μm were trapped sequentially in array chambers and imaged to analyse the intensity of sGFP fluorescence from bacteria-occupied areas using a fluorescence microscope (Eclipse Ti-E, Nikon, Japan) equipped with a 488 nm argon-ion laser and a PMT with GFP filter and NIS Elements software (Nikon, Japan). Then, we calculated sGFP fluorescence intensity per unit area to determine the intracellular L-tryptophan concentration of each single-cell. The cells with the highest ANFI values were retrieved from the SDA and grown on LB-agar plates (Cm). Promoter and 5′-UTRs of the cells were amplified by PCR with chk_ppsA_F/R and sequenced using the same primer set.
Tryptophan production and detection of metabolites
The base strain (SJT0), the parental strain (SJT1), and the three chip-screened strains (SJT11, SJT12, SJT13) were grown for 24 hours in M9 minimal medium supplemented with 4 g L−1 glucose and 34 μg ml−1 chloramphenicol (Cm). The cultures were diluted to an O.D.600 of 0.05 in the same medium until reaching an O.D.600 of 0.8 to prepare fresh seeds. The seed cultures were inoculated 30 mL of the same medium in 300 ml flasks to a final O.D.600 of 0.05 and incubated at 37 °C with shaking (250 rpm).
Concentrations of accumulated L-tryptophan and consumed glucose in culture broth were analysed using an Ultimate 3000 analytical high-performance liquid chromatography (HPLC) system (Dionex, Sunnyvale, CA, USA) equipped with appropriate columns. The concentration of L-tryptophan was determined by a pre-column derivatization method using o-phthalaldehyde.34 The derivatized samples were eluted with a gradient of acetonitrile, methanol, and water (v/v% 45
:
45
:
10) and 50 mM sodium acetate buffer (pH 6.5) at a flow rate of 1.5 ml min−1 through an Acclaim 120 C18 reverse-phase column (Dionex) maintained at 40 °C, and monitored by an ultraviolet-visible diode array detector at 338 nm. The concentration of glucose was measured with an Aminex HPX-87H column (Bio-Rad Laboratories, Richmond, CA, USA) maintained at 65 °C. The samples were eluted with 5 mM H2SO4 at a flow rate of 0.6 ml min−1 and monitored by a Shodex RI-101 detector (Shodex, Klokkerfaldet, Denmark).
Results and discussion
Generation of the static droplet array with entrapped single cells for screening
Screening of metabolite-producing microbial strains from a library comprises measurement of the target metabolite from each constituent cell and selective retrieval of the desired cells. It is especially preferable to analyse the intracellular metabolite concentration at the single-cell level to properly evaluate the cellular performance for the production of target metabolite. In this study, we screened an E. coli library for L-tryptophan production using an SDA device consisting of three layers: fluidic layer for generating and guiding of droplets, control layer for trapping and releasing of the droplets, and a blocking layer for freezing the control valves (Fig. 1A). The three layers were assembled to construct a 3 × 8 array chamber within 1.8 mm × 2.6 mm to trap droplets on a chip.
The first step for screening of bacterial strains with high metabolite production was to spatially separate library cells before analysing the metabolite concentration. Therefore, we demonstrated the generation of the single-cell droplet array by selective droplet manipulation that was previously reported. We adequately diluted the pre-cultured bacteria to maximize the number of single-cell encapsulated droplets according to Poisson's law22 and injected the bacterial solution into the SDA device. 100-picoliter mono-disperse droplets containing cells were generated and moved to the array region while control valves were on. The cells encapsulated in each droplet were visualized by sGFP encoded by the plasmid in the cells. Since the number of cells stored in droplets follows Poisson's law, the chambers with multiple cells should be replaced with single-cell droplets by repeating the selective releasing and refilling manipulations while other single-cell droplets remain intact. (Fig. 1B). In general, based on our procedure, the generating time of the single-cell array consisted of three steps: formation of droplet array containing 24 droplets (5 seconds), analysis and selective release of undesired droplets (2 seconds), and droplet re-filling into array (1 second). Consequently, it takes an average of 15 seconds to generate the single cell array after an average number of repetitions (n = 5), including selective removing of undesired droplets (2 s) and refilling cell-encapsulating droplets (1 s). Therefore, the droplet array system is able to analyze 4320 cells per hour because the analysis of 24 droplets consumes 20 seconds.
Fig. 1C shows our screening procedure in the SDA based on fluorescence signal intensity. As a selection parameter, we analysed the fluorescent signal intensity normalized by the area occupied by a single bacterial cell (ANFI, area-normalized fluorescence intensity) in the generated droplet array. After that, the ANFI values were indexed by the location of array chamber in the 3 × 8 array to recognize the desired single cells. Single cells whose ANFI was higher than a certain pre-defined threshold were selectively released into the collection chamber to be harvested. Otherwise, the encapsulated cells with lower values were wasted (Fig. 1C and S1†).
Fluorescence-based measurement of intracellular metabolite concentration
Intracellular concentration of the target metabolite should be easily measured to screen a large library efficiently. In that regard, the optical detection method using fluorescent protein is one of the best approaches for high-throughput screening. Therefore, we applied an artificial riboswitch, which is able to activate the expression level of sGFP in response to L-tryptophan,13 for on-chip visualization and quantification of L-tryptophan in this study. To utilize the riboswitch for quantification of L-tryptophan, functionality and dose–response curves of the riboswitch were validated using the SDA device.
E. coli W3110 was transformed with the plasmid that encodes the riboswitch and cultured in M9 (Cm) supplemented with 0, 0.01, 0.05, 0.1, 0.5, and 1 g L−1 of L-tryptophan for 4 hours to adjust and stabilize the expression level of sGFP for each L-tryptophan concentration. Extracellular L-tryptophan can be readily transported across the cell membrane through a set of transporters35 and activate the riboswitch inside the cell. Next, the cells were injected into the SDA device to create the single-cell SDA as described in the materials and methods section. Then, we measured ANFI from about fifty single cells in the droplets for each L-tryptophan concentration and the measured ANFI values were averaged. As anticipated, the ANFI increased as concentration of the supplemented L-tryptophan increased, displaying a sigmoidal dose–response curve (Fig. 2). Accordingly, the riboswitch enabled distinction of different intracellular L-tryptophan concentrations based on the ANFI, and the dose–response curve was used as a criterion for screening of the E. coli library.
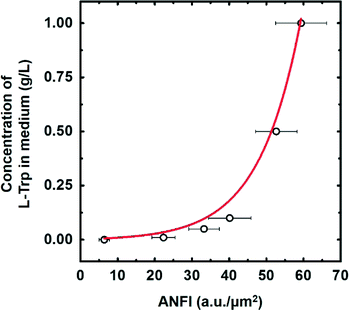 |
| Fig. 2 Dose–response curve between L-tryptophan concentration and ANFI of single cells generated by the riboswitch. L-Tryptophan was added to the medium at concentrations of 0, 0.01, 0.05, 0.1, 0.5 and 1 g L−1. About fifty single cells were analysed for each L-tryptophan concentration. | |
Analysis and screening of a bacteria library
We combined the single-cell SDA and the riboswitch for screening of an E. coli library whose L-tryptophan productivity was diversified. Specifically, the 5′-UTR of chromosomal ppsA of SJT1 (see section 2.2) was randomized to modulate its expression levels; as a result, metabolic flux towards L-tryptophan biosynthesis was diversified in this library (Fig. 3A). Next, the library transformed with the riboswitch plasmid was cultured overnight, and sub-cultured for 5 hours, to prepare fresh cells with a distinctive fluorescence intensity distribution as a consequence of the diversified L-tryptophan productivity. Then, we analysed 4231 total cells to investigate a population distribution of diversified ANFIs on the SDA device. The cells were collected in the “collection chamber”, which is shown in Fig. S1,† after droplet generation with cells. The top 10% of the population, which is roughly 423 cells, showed ANFIs of 51–65 a.u. μm−2, and only 83 bacteria corresponding to the top 2% exhibited values of above 60 a.u. μm−2 (Fig. 3B). We selectively retrieved 51 droplets harbouring single cells into the chamber whose ANFI was above 50 a.u. μm−2 from observation of over 4000 single-cell droplets utilizing the plot shown in Fig. 2 and 3B.
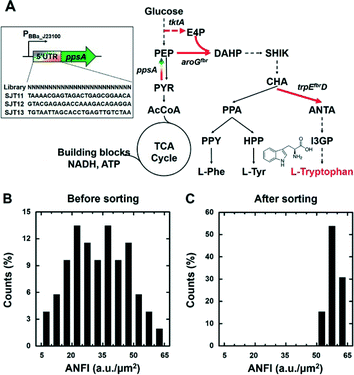 |
| Fig. 3 (A) Metabolic pathway towards L-tryptophan in E. coli (right): phosphoenolpyruvate (PEP), pyruvate (PYR), acetyl-CoA (AcCoA), D-erythrose 4-phosphate (E4P), 3-deoxy-D-arabino-heptulosonate-7-phosphate (DAHP), shikimate (SHIK), chorismate (CHA), prephenate (PPA), 2-oxo-3-phenylpropanoate (PPY), L-phenylalanine (L-Phe), 4-hydroxyphenylpyruvate (HPP), L-tyrosine (L-Tyr), anthranilate (ANTA), and (1S,2R)-1-C-(indol-3-yl)glycerol 3-phosphate (I3GP). Thick, red arrows indicate target genes whose expression were amplified by the synthetic promoter and redesigned 5′-UTRs: phosphoenolpyruvate synthase (ppsA), transketolase (tktA), 3-deoxy-D-arabino-heptulosonate-7-phosphate synthase, feedback-resistant (aroGfbr), anthranilate synthase, and feedback-resistant (trpEfbrD). Expression level of ppsA was diversified by randomizing its 5′-UTR sequence. Sequences of ppsA 5′-UTRs from the screened variants are shown (left). (B) Cell population distribution of ANFI before sorting and (C) after sorting. The results (B) were obtained from the analysis of 4231 total cells in 1 hour to investigate a population distribution showing different ANFIs on the SDA device. | |
The fluorescence intensity distribution of the selected cells was analysed to corroborate screening efficiency of our system. We obtained a population where 100% of the cells of the population displayed an ANFI above 50 a.u. μm−2 and 31% of cells showed above 60 a.u. μm−2. This result indicates that we successfully enriched the library towards high fluorescence intensity, reaching an increase in over 833% of the proportion of cells whose ANFI is over 50 a.u. μm−2, and 1500% increase whose value is larger than 60 a.u. μm−2 compared to the starting population. Collectively, the single-cell SDA and the riboswitch were successfully coupled to analyse the fluorescence intensity of the E. coli population and to enrich the population towards a specific direction, namely, high L-tryptophan production.
Chemical production from the screened bacteria
We acquired three screened cells whose ANFI were 58.6, 61.4, and 63.2. It was confirmed that the sequences of promoter and 5′-UTRs of the isolated cells were consistent with the design of the library (Fig. 3A). The cells were cultured to confirm that the L-tryptophan productivity of the enriched cells was improved in comparison with the wild type and the parental strain. The SJT0, SJT1, and the three screened strains (SJT11, SJT12, and SJT13) were cultured to validate the L-tryptophan production. Each strain was inoculated in 30 mL of M9 (Cm) and cultured at 37 °C with shaking at 250 rpm. At first, L-tryptophan was not detected from the culture broth of SJT0, whereas SJT1 produced 0.045 ± 0.0034 g L−1 in 24 hours (Fig. 4A, Table S3†). Moreover, all the three screened strains showed improved L-tryptophan production, reaching up to 0.065 ± 0.0084 g L−1, which corresponds to a 45.4% increase compared to SJT1 (Fig. 4A, Table S3†). Moreover, the screened strains were enhanced in terms of the specific productivity that quantifies the production rate normalized by the cell mass. Interestingly, the measured ANFI of each strain could be used to estimate the specific productivity since both values were normalized by the number of cells (Fig. 4B). In the meantime, the specific growth rate of SJT1 was decreased compared to SJT0, and the rates of SJT11, SJT12, and SJT13 were decreased even further (Table S3†). The cell growth can be negatively affected by the production of L-tryptophan because this pathway consumes PEP, one of the intermediates of the central carbon metabolism that has a pivotal role in the generation of building blocks and energy materials for cell growth (Fig. 3A).36,37 This result emphasizes again that high-throughput screening is necessary to find an optimum where L-tryptophan production and cell growth are well-balanced. Taken altogether, the SDA device, combined with the artificial riboswitch, was able to efficiently screen improved L-tryptophan producer strains based on its ability to manipulate single cells and to visualize intracellular metabolites.
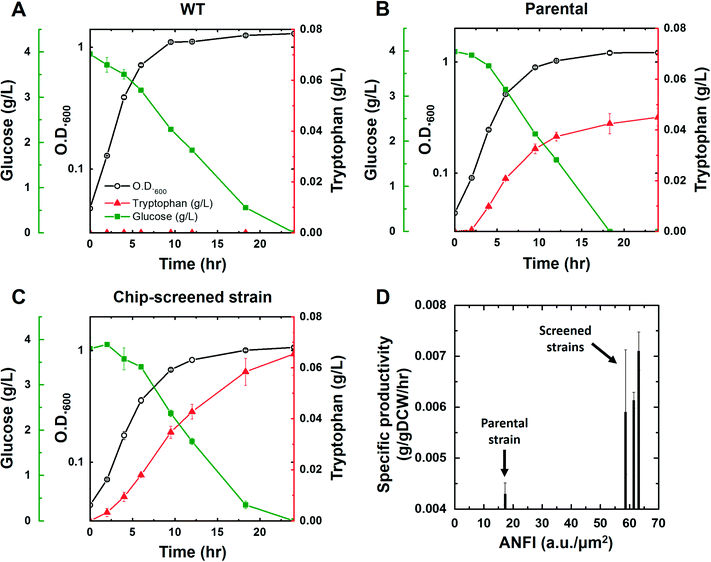 |
| Fig. 4 (A)–(C) HPLC measurements from the flask culture of E. coli strains. Tryptophan production, O.D.600, and consumption of glucose of the E. coli (A) wild type, (B) parental strain, and (C) chip-screened strains were represented by red triangles, black open circles, and green squares, respectively. Briefly, each strain was inoculated to 30 mL of M9 (Cm) medium in a 300 mL flask. Metabolites in the culture broth were detected using HPLC. Detailed procedure for the flask culture and metabolite measurements are explained in the experimental section. (D) L-Tryptophan specific productivity of the E. coli strains whose ANFI were 58.6, 61.4 and 63.1. | |
Conclusions
Herein, we established a screening method that could enrich bacterial strains with high metabolite production based on optical analysis at the single-cell level. The SDA device and the artificial riboswitch were joined together for quantitative visualization of intracellular L-tryptophan, on-chip analysis, indexing, and selective retrieval of single cells. We were able to readily investigate a dose–response curve of the riboswitch and fluorescence population of the mutagenized E. coli library using this method. Bacterial cells with high concentrations of intracellular L-tryptophan were screened employing the dose–response curve and the fluorescence population as selection criteria. Efficiency of the enrichment reached up to 1500%, and most of the screened cells belonged to the highest quartile of the population. The titer of L-tryptophan from the screened variants was increased to 145% in comparison with the parental strain; and the specific productivity was also improved.
Our method provides several advantages over conventional screening technologies. Unnecessary use of large amount of culture medium and consumables could be avoided thanks to the capability of analysing metabolites at the single cell level. Selective manipulation and indexing guarantees only the desired cells are collected for further study. Unwanted growth impairment can be prevented as well since the SDA device offers mild conditions for the cells. Furthermore, this method may be extended to a wide variety of chemicals considering that riboswitch-based metabolite sensors could be easily engineered through a variety of synthetic biology techniques.
Acknowledgements
This work was supported by the Advanced Biomass R&D Center (ABC) of Global Frontier Project funded by the Ministry of Science, ICT and Future Planning (ABC-2015M3A6A2066119), the Marine Biomaterials Research Center of the Marine Biotechnology Program funded by the Ministry of Oceans and Fisheries, Basic Core Technology Development Program (NRF-2015M1A5A1037054), Basic Science Research Program (NRF-2011-0017322, NRF-2015R1A2A1A10056126), and Global Research Laboratory (NRF-2015K1A1A2033054) of the National Research Foundation of Korea (NRF) grant funded by the Korea government (Ministry of Science, ICT & Future Planning).
References
- T. Willke and K. D. Vorlop, Appl. Microbiol. Biotechnol., 2004, 66, 131–142 CrossRef CAS PubMed
.
- J. D. Keasling, Science, 2010, 330, 1355–1358 CrossRef CAS PubMed
.
- B. M. Woolston, S. Edgar and G. Stephanopoulos, Annu. Rev. Chem. Biomol. Eng., 2013, 4, 259–288 CrossRef CAS PubMed
.
- J. Mampel, J. M. Buescher, G. Meurer and J. Eck, Trends Biotechnol., 2013, 31, 52–60 CrossRef CAS PubMed
.
- J. Abatemarco, A. Hill and H. S. Alper, Biotechnol. J., 2013, 8, 1397–1410 CrossRef CAS PubMed
.
- R. E. Cobb, N. Sun and H. Zhao, Methods, 2013, 60, 81–90 CrossRef CAS PubMed
.
- S. Gregoire, J. Irwin and I. Kwon, Korean J. Chem. Eng., 2012, 29, 693–702 CrossRef CAS PubMed
.
- J. A. Dietrich, A. E. McKee and J. D. Keasling, Annu. Rev. Biochem., 2010, 79, 563–590 CrossRef CAS PubMed
.
- W. Liu and R. Jiang, Appl. Microbiol. Biotechnol., 2015, 99, 2093–2104 CrossRef CAS PubMed
.
- H. H. Jeong, Y. G. Kim, S. C. Jang, H. Yi and C. S. Lee, Lab Chip, 2012, 12, 3290–3295 RSC
.
- M. Olsen, B. Iverson and G. Georgiou, Curr. Opin. Biotechnol., 2000, 11, 331–337 CrossRef CAS PubMed
.
- J. Zhang, M. K. Jensen and J. D. Keasling, Curr. Opin. Chem. Biol., 2015, 28, 1–8 CrossRef PubMed
.
- Z. Kang, C. Zhang, J. Zhang, P. Jin, G. Du and J. Chen, Appl. Microbiol. Biotechnol., 2014, 98, 3413–3424 CrossRef CAS PubMed
.
- R. Mahr and J. Frunzke, Appl. Microbiol. Biotechnol., 2016, 100, 79–90 CrossRef CAS PubMed
.
- H. H. Jeong, S. H. Lee, J. M. Kim, H. E. Kim, Y. G. Kim, J. Y. Yoo, W. S. Chang and C. S. Lee, Biosens. Bioelectron., 2010, 26, 351–356 CrossRef CAS PubMed
.
- J. Yang, S. W. Seo, S. Jang, S. I. Shin, C. H. Lim, T. Y. Roh and G. Y. Jung, Nat. Commun., 2013, 4, 1413 CrossRef PubMed
.
- J. K. Michener and C. D. Smolke, Metab. Eng., 2012, 14, 306–316 CrossRef CAS PubMed
.
- C. C. Fowler, E. D. Brown and Y. Li, Chem. Biol., 2010, 17, 756–765 CrossRef CAS PubMed
.
- S. Jang, J. Yang, S. W. Seo and G. Y. Jung, Methods Enzymol., 2015, 550, 341–362 Search PubMed
.
- C. Berens and B. Suess, Curr. Opin. Biotechnol., 2015, 31, 10–15 CrossRef CAS PubMed
.
- S. Topp and J. P. Gallivan, ACS Chem. Biol., 2010, 5, 139–148 CrossRef CAS PubMed
.
- G. Yang and S. G. Withers, ChemBioChem, 2009, 10, 2704–2715 CrossRef CAS PubMed
.
-
L. W. Arnold and J. Lannigan, in Curr. Protocol. Cytom., John Wiley & Sons, Inc., 2010, vol. 51, pp. 1.24.1–1.24.30 Search PubMed
.
- S. L. Sjostrom, Y. Bai, M. Huang, Z. Liu, J. Nielsen, H. N. Joensson and H. Andersson Svahn, Lab Chip, 2014, 14, 806–813 RSC
.
- B. L. Wang, A. Ghaderi, H. Zhou, J. Agresti, D. A. Weitz, G. R. Fink and G. Stephanopoulos, Nat. Biotechnol., 2014, 32, 473–478 CrossRef CAS PubMed
.
- M. Huang, Y. Bai, S. L. Sjostrom, B. M. Hallstrom, Z. Liu, D. Petranovic, M. Uhlen, H. N. Joensson, H. Andersson-Svahn and J. Nielsen, Proc. Natl. Acad. Sci. U. S. A., 2015, 112, E4689–4696 CrossRef CAS PubMed
.
- D. J. Im, Korean J. Chem. Eng., 2015, 32, 1001–1008 CrossRef CAS
.
- R. S. Boogar, R. Gheshlaghi and M. A. Mahdavi, Korean J. Chem. Eng., 2013, 30, 45–49 CrossRef CAS
.
- Y.-R. Lee, J. Kim and W.-S. Ahn, Korean J. Chem. Eng., 2013, 30, 1667–1680 CrossRef CAS
.
- J. W. Hwang, J.-H. Choi, B. Choi, G. Lee, S. W. Lee, Y.-M. Koo and W.-J. Chang, Korean J. Chem. Eng., 2015, 33, 57–62 CrossRef
.
- H. H. Jeong, S. H. Jin, B. J. Lee, T. Kim and C. S. Lee, Lab Chip, 2015, 15, 889–899 RSC
.
- S. H. Jin, H. H. Jeong, B. Lee, S. S. Lee and C. S. Lee, Lab Chip, 2015, 15, 3677–3686 RSC
.
- R. Heermann, T. Zeppenfeld and K. Jung, Microb. Cell Fact., 2008, 7, 14 CrossRef PubMed
.
- B. N. Jones and J. P. Gilligan, J. Chromatogr., 1983, 266, 471–482 CrossRef CAS PubMed
.
-
K. Marin and R. Krämer, in Amino Acid Biosynthesis ~ Pathways, Regulation and Metabolic Engineering, ed. V. Wendisch, Springer Berlin Heidelberg, 2007, ch. 69, vol. 5, pp. 289–325 Search PubMed
.
- T. Shen, Q. Liu, X. Xie, Q. Xu and N. Chen, J. Biomed. Biotechnol., 2012, 2012, 605219 Search PubMed
.
- R. Patnaik and J. C. Liao, Appl. Environ. Microbiol., 1994, 60, 3903–3908 CAS
.
Footnotes |
† Electronic supplementary information (ESI) available. See DOI: 10.1039/c6lc00118a |
‡ The authors contributed equally to this work. |
|
This journal is © The Royal Society of Chemistry 2016 |
Click here to see how this site uses Cookies. View our privacy policy here.