DOI:
10.1039/C5MB00132C
(Paper)
Mol. BioSyst., 2015,
11, 1378-1388
The distribution pattern of genetic variation in the transcript isoforms of the alternatively spliced protein-coding genes in the human genome†
Received
11th February 2015
, Accepted 22nd March 2015
First published on 23rd March 2015
Abstract
By enabling the transcription of multiple isoforms from the same gene locus, alternative-splicing mechanisms greatly expand the diversity of the human transcriptome and proteome. Currently, the alternatively spliced transcripts from each protein-coding gene locus in the human genome can be classified as either principal or non-principal isoforms, providing that they differ with respect to cross-species conservation or biological features. By mapping the variants from the 1000 Genomes Project onto the coding region of each isoform, an interesting pattern of the genetic variation distributions of the coding regions for these two types of transcript isoforms was revealed on a whole-genome scale: compared with the principal isoform-specific coding regions, the non-principal isoform-specific coding regions are significantly enriched in amino acid-changing variants, particularly those that have a strong impact on protein function and have higher derived allele frequencies, suggesting that non-principal isoform-specific substitutions are less likely to be related to phenotype changes or disease. The results herein can help us better understand the potential consequences of alternatively spliced products from a population perspective.
Introduction
Alternative splicing is considered to be one of the major evolutionary mechanisms for expanding the diversity of the transcriptomes and proteomes of many, if not all, eukaryotic genomes.1 For example, it is estimated that over two-thirds of the multi-exon protein-coding genes in the human genome undergo alternative splicing,2,3 with each gene producing at least two alternatively spliced transcript isoforms that may have related, altered or even contrasting biochemical functions.4,5 Interestingly, however, in the past decade, an in-depth study of the human transcriptome using next-generation RNA sequencing (RNA-seq) has raised the question of whether all of these alternatively spliced products actually encode functional proteins.6–9
To analyse functional genomic data and reduce computational complexity, each of the multi-exon protein-coding genes annotated in a given reference genome is generally assigned one of its alternatively spliced transcripts as its representative gene product. For the sake of simplicity, the longest transcript isoform of each alternatively spliced gene is typically used for analysis, although this safe selection is not always the best strategy.10 By integrating eight annotation modules, the recent APPRIS system provides a reliable classification scheme for the transcript isoforms of the alternatively spliced genes in the human genome.11 Meanwhile, APPRIS provides a ranking method that, for each protein-coding genes with alternative splicing, assigns one transcript to be the representative of the gene and denotes the transcript as the principal isoform of the gene. Overall, the principal isoform is the one with the main cellular function, which may manifest the characteristics of its 3D structural integrity, cross-species evolutionary conservation and the main cellular function. In addition, it largely overlaps with the dominant transcript in the expression profile.12 Nonetheless, as we know, not all of the predicted genes with alternative splicing currently possess such diverse additional information mentioned above, for these genes (∼15% in this study), it is plausible to select the longest isoforms as their principal isoforms. Due to its reliable annotation, APPRIS has also been utilised by many large-scale genome databases such as Ensembl13 and GENCODE.14
In addition, due to the implementation of the 1000 Genomes Project (1KG),15 a significant amount of variation in the human population has been identified at the whole-genome level. The 1000 Genomes Project is a valuable resource for evaluating the functional importance of biological elements and estimating the selective constraints on genomic regions.15,16 In our research, which is based on structural models of the alternatively spliced protein-coding genes using GENCODE (version 15) annotation and the APPRIS assignments for each protein-coding gene, we simply classify each of the gene's transcripts into one of the two categories: principal or non-principal isoforms. Our aim is to explore the genome-wide pattern of the genetic variation distributions between these two types of transcript isoforms, namely, the principal isoforms (PIs) and their counterparts—the non-principal isoforms (NPIs). We hope that our integrative analysis using an extended variation dataset, the more highly accurate gene models by GENCODE, and the reliable assignments of principle isoforms will enable us to better understand the genome-wide, rather than anecdotic, consequences of alternative splicing from a population perspective.
Results and discussion
Identification of coding variation and derived allele frequencies
All the coding variations, including single-nucleotide polymorphisms (SNPs) and frameshift small insertions and deletions (indels), in the 1000 Genomes Project Phase I15 were extracted in accordance with GENCODE14 annotation. In total, 206
221 synonymous, 295
997 non-synonymous, and 5820 stop-gain/loss distinct SNPs and 941 distinct frameshift indels passed this filtering step. The stop-gain/loss SNPs were further divided into stop-gain and stop-loss SNPs relative to their ancestral alleles. A stop-gain SNP can be defined as a stop-gain SNP if a derived polymorphism results in a premature stop codon and leads to a truncated protein relative to its ancestral genotype. Similarly, a stop-loss SNP can be defined as a SNP that leads to protein elongation via a loss of normal termination compared with its ancestral state. Stop-gain SNPs accounted for the vast majority (approximately 99.4%) of the total number of stop-gain/loss SNPs, and only 34 SNPs were found to be stop-loss SNPs relative to their genotypes of ancestry. The median derived allele frequency of the stop-loss SNPs (median frequency 0.83) was much higher than the median derived allele frequency of the stop-gain SNPs (median frequency 5 × 10−04) (Fig. S1, ESI†). The vast majority (89.9%) of the stop-gain SNP frequencies were less than 0.5%. A full list of stop-gain/loss SNPs can be found in Supplementary file 1 (ESI†).
Stop-gain SNPs and frameshift indels are defined to be loss-of-function (LoF) variations that have the potential to severely disrupt the function of protein-coding genes.17 LoF variants are widely found in the genomes of healthy individuals.17 They are largely found at low frequencies, possibly due to the effects of purifying selection.18 Many recent studies have focused on this type of variation.19,20 In our dataset, the LoF variants were also found at extremely low population frequencies.
Density comparison of the variation in various frequencies in three isoform-specific categories
The coding regions of the multi-transcript protein coding genes were split into three distinct categories using APPRIS11 and GENCODE annotation (Fig. 1): PI-specific regions (principal isoform-specific coding regions), NPI-specific regions (non-principal isoform-specific coding regions), and overlapping regions (coding sequences that were shared by the principal and non-principal isoforms). (The coordinates of each isoform-specific coding region are provided in Supplementary file 2, ESI†). Overlapping regions accounted for the majority (approximately 73.6%) of the total protein coding regions in the multi-transcript protein coding genes, whereas NPI-specific regions accounted for only approximately 4.9% of the total protein coding regions.
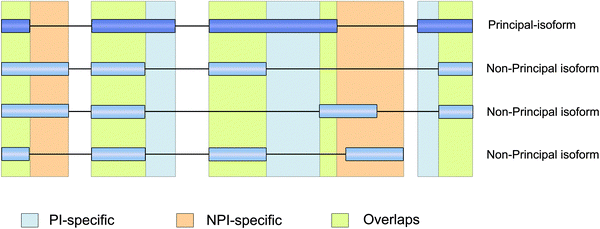 |
| Fig. 1 Schematic model for the classification of PI-specific, NPI-specific and overlapping regions. The diagram shows the classification schema for a principal isoform (coding regions are shown in dark blue) and three non-principal isoforms (coding regions are shown in Cambridge blue), including different types of alternative splicing. The blue background represents the coding region used solely by the principal isoform, the orange background represents the coding region used solely by the non-principal isoforms and the yellow background represents the coding region used by both the principal and non-principal isoforms. | |
Next, we mapped the coding variations onto the three isoform categories. We found that although the NPI-specific regions accounted for a small fraction of the total coding regions in the multi-transcript protein coding genes, over 11.3% of the stop-gain/loss SNPs and 37.5% of the frameshift indels were located in NPI-specific regions. The ratio of the number of stop-gain/loss SNPs in NPI-specific regions to the total number of stop-gain/loss (over 11.3%) was significantly different from the ratio of the NPI-specific lengths vs. total genome length in the multi-transcript genes (Pearson's chi-square test, P = 5.0 × 10−4 for the stop-gain/loss SNPs). The ratio of NPI-specific non-synonymous SNPs to the total number of non-synonymous SNPs (approximately 5.3%) was higher than the ratio of the NPI-specific lengths vs. total genome length as well (Fig. 2). In summary, on the whole genome scale, missense (non-synonymous and stop-gain) SNPs and small frameshift insertions and deletions (indels) are more highly enriched in the NPI-specific coding regions relative to the PI-specific coding and overlapping regions, particularly for stop-gain SNPs.
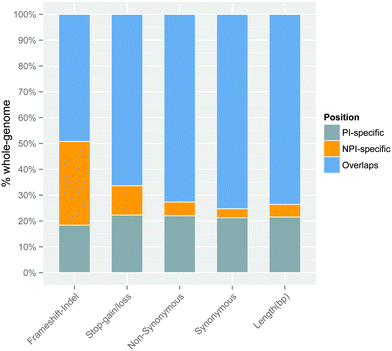 |
| Fig. 2 The proportion of PI-specific, NPI-specific, and overlapping variations associated with different types of substitutions in multi-transcript genes. The ‘Length (bp)’ bar represents the relative percentage of the genomic sequence length of each isoform category on a whole-genome scale. The other bars represent the relative number of variations in different isoform categories. The percentage ratios have been normalised to one hundred percent. The exact number of variations can be found in Table S3 (ESI†). | |
To test the robustness of our results, we sampled a subset (5%) of the protein-coding genes with NPI-specific regions, and then randomised the genomic locations of the SNPs in the coding region of each gene. This process was repeated 1000 times for the stop-gain and non-synonymous datasets. Next, we compared the randomised ratio of the NPI-specific vs. the total SNPs to the observed ratio in the gene set. Out of 1000 randomised experiments, there were only two cases where the random ratio was greater than the observed ratio. This indicates that stop-gain SNPs tend to accumulate in NPI-specific regions. Although the difference was not as pronounced in the non-synonymous dataset compared with the stop-gain dataset, the observed ratios were higher than the randomised values in more than 70% of the random experiments. In addition, as the sampled gene number increased, the number of observed ratios that were higher than the randomised ratio was also increased in the non-synonymous dataset. This indicates that although the pattern was not quite significant in the non-synonymous datasets of a few genes, at the whole-genome level, stop-gain and non-synonymous SNPs tended to be harboured in NPI-specific regions. Furthermore, non-synonymous variations have less impact on protein function than stop-gain SNPs. The effects of non-synonymous substitutions vary and include deleterious, benign, and adaptive effects.21 This may explain the decrease of significance of the non-synonymous dataset.
In addition, we subdivided the SNPs into rare (<0.5%), low (0.5–5%), and common (>5%) groups for each substitution dataset according to their derived allele frequencies (DAFs). By comparing the SNP numbers and their allele frequencies in these three distinct coding regions, we found that high-frequency stop-gain SNPs were more inclined to harbour in the NPI-specific regions than in the PI-specific and overlapping regions, i.e., approximately 31% of the low (0.5–5%) and 40% of the common (>5%) stop-gain SNPs were located in NPI-specific regions (Fig. 3). Similarly the proportion of non-synonymous SNPs was significantly higher in NPI-specific regions accompanied by an increase in allele frequency bins.
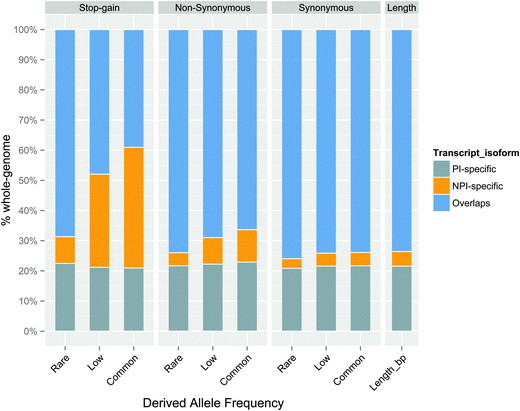 |
| Fig. 3 The proportion of PI-specific, NPI-specific, and overlapping SNPs associated with different types of substitutions at different derived allele frequencies. The percentage ratios have been normalised to one hundred percent. The exact number of variations can be found in Table S4 (ESI†). | |
Coding consequence is one of the determinate factors of the strength of purifying selection against variation.15 Stop-gain variants are expected to undergo stronger purifying selection than non-synonymous variants.20,22 Our results showed that on a whole-genome scale, amino acid changing mutations were preferentially located in NPI-specific regions over PI-specific regions or overlapping regions, particularly for high frequency SNPs. Previous studies have indicated that human alternatively spliced exons are subjected to relaxed selective pressure or positive selection.23,24 Furthermore, the minor alternatively spliced exons, which have low inclusion levels, showed an increased Ka/Ks ratio compared with the major alternatively spliced exons (with higher inclusion levels) and constitutive exons (where the exon is included in every EST).23,24 Similar to constitutive exons, overlapping regions are more sensitive to protein-changing variants. Taking the enrichment of stop-gain SNPs in NPI-specific regions into consideration, our result seems to support the hypothesis that AS relaxes the selective pressure on NPI-specific regions over the hypothesis that AS has an effect on positive selection.
The derived allele frequencies of the NPI-specific SNPs were significantly higher than those of the PI-specific and overlapping SNPs
The 1000 genome data included a considerable proportion of low frequency variations. Low-frequency variations reflect not only recent explosive population growth but also include a considerable number of deleterious mutations that can be suppressed by negative selection.25,26 Previous studies have suggested that an enrichment of rare variations is correlated with the level of purifying selection.15 If a specific genomic feature has a higher proportion of rare non-synonymous SNPs, it is likely to have more selective constraints on it.15,16
To estimate the selective constraints in different coding categories, we delineated the derived allele-frequency spectrum into rare, low, and common bins for each isoform category for all the substitution datasets (Fig. 4). As Fig. 4 shows, the overlapping and PI-specific regions have a much higher fraction of rare stop-gain and non-synonymous SNPs than the NPI-specific regions. Overall, the derived allele frequencies of the SNPs in the NPI-specific regions were remarkably higher than those in the overlapping or PI-specific regions (Mann–Whitney U-test, P < 2.2 × 10−16) in all three substitution datasets. These results showed that the PI-specific and overlapping regions were more sensitive to mutations than the NPI-specific regions. In addition, we further compared the proportion of the rare non-synonymous SNPs in the protein coding genes that produce only one protein-coding transcript (one-to-one coding genes) to the isoform-specific non-synonymous SNPs. The enrichment of rare non-synonymous SNPs may reflect purifying selection on genomic features.15,16 The proportion of rare SNPs in the total non-synonymous SNPs of the one-to-one genes was between the proportions of the PI-specific and NPI-specific SNPs (S2, ESI†).
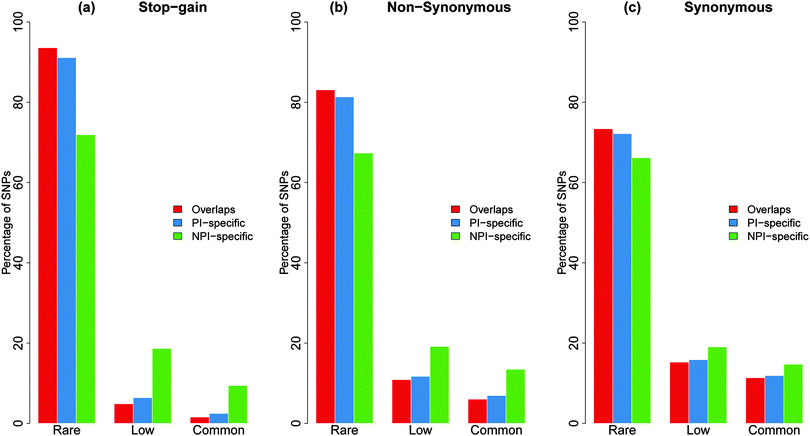 |
| Fig. 4 Derived allele frequency spectrum of the PI-specific, NPI-specific and overlapping SNPs. (a) The derived allele frequency spectrum of the stop-gain SNPs in three frequency bins (rare, low, and common), where the ‘rare’ group represents the proportion of rare SNPs to the total SNPs in the given substitution and isoform categories. The stop-gain SNPs in overlapping regions have the highest proportion of rare SNPs. (b) The derived allele frequency spectrum of the non-synonymous SNPs. (c) The derived allele frequency spectrum of the synonymous SNPs. | |
Intuitively, functional requirements may point to another possible explanation for the increased frequencies of NPI-specific stop-gain SNPs. Alternative splicing has been proposed as a mechanism for regulating expression levels.27,28 A transcript that contains a stop-gain SNP may trigger the nonsense-mediated mRNA decay (NMD-decay) pathway, an important surveillance mechanism to prevent the potential toxicity of a truncated protein by degrading the aberrant protein, by introducing a premature termination codon (PTC).29 Previous studies have suggested that alternative splicing has the potential to couple the NMD pathway to expression levels by including or excluding PTC-containing exons.27,28
To assess the ability of the stop-gain SNPs in the NPI-specific regions to trigger NMD pathways, we compared the NMD-targeting actions of the PI-specific and NPI-specific stop-gain SNPs. If NPI-specific stop-gain SNPs are targeted for NMD, they would be more likely to trigger the NMD pathway than the PI-specific stop-gain SNPs. According to the 50–55-nucleotide rule, a stop-gain SNP has the potential to trigger NMD if the position of the mutation is greater than 50–55 bp beyond the last exon–intron boundary.30 However, in our dataset, there was no evidence for this NMD requirement for the NPI-specific SNPs (Table S1, ESI†). In contrast, the PI-specific stop-gain SNPs had a greater proportion of NMD positive SNPs than the NPI-specific stop-gain SNPs (Table S1, ESI†). Taken together, these results suggest that higher population frequencies of stop-gain SNPs in NPI-specific regions are most likely due to weaker purifying selection. A study showed a reduced negative selection pressure against PTCs that potentially targeted NMD by AS.31 However, nonsense-mediated decay transcripts were filtered out of our dataset during SNP mapping, and only protein-coding transcripts were retained. However, non-principal transcripts, even if they were annotated as full-length protein coding transcripts, still showed an increased tolerance for stop-gain SNPs compared with principal isoforms. The significant increase in the population frequency of NPI-specific protein-changing SNPs suggests that NPI-specific regions might undergo weaker purifying selection than PI-specific and overlapping regions.
Fewer phenotypic associations related with NPI-specific SNPs
To further validate the functional consequences of the variations in the NPI-specific, PI-specific and overlapping regions, we intersected the coding SNPs using the ClinVar database (Table 1), an evidence-based, easily accessible resource of human variations that contribute to disease or phenotypic changes. After comparing the occurrence ratios of the SNPs in the different coding regions, we found that SNPs in NPI-specific regions were less likely to have phenotypic consequences or cause observed health status changes than those in the overlapping and PI-specific regions. Among the distinct 468 stop-gain SNPs in the NPI-specific regions, only 4 SNPs had phenotypic association records in the ClinVar database (rs76826147 in ENST00000400191, rs142934950 in ENST00000389169, rs104894715 in ENST00000324001, and rs142516141 in ENST00000450719 and ENST00000561003). This indicates, on average, that 1/117 stop-gain SNPs were found to be related to a disease or a phenotype in the NPI-specific SNPs, compared with a ratio of 1/41.7 in the PI-specific SNPs (Fisher's exact test, P-value = 0.058). The difference was also very significant in the non-synonymous dataset, which had 1/311.5 NPI-specific SNPs recorded in the ClinVar database; however, this ratio was 1/100.9 in the PI-specific SNPs (Fisher's exact test, P-value < 2.2 × 10−16). In addition, none of the 34 distinct stop-loss SNPs had phenotypic records in the ClinVar database. We further checked the genomic locations of all the single nucleotide variation records in the ClinVar32 database using an identical approach (Table 2), and the ClinVar mutations were also more frequently present in the overlapping and PI-specific regions. We must note that, in certain cases, the NPI-specific SNPs may still contribute to the phenotypic changes. For example, for the pathogenic variant rs104894715 as listed above, it is inside the transcript ENST00000324001 that encodes the larger protein isoform (L-periaxin) of the periaxin (PRX) gene. Interestingly, in APPRIS, this protein isoform is annotated as non-principal and the other isoform is principal which encodes S-periaxin. Many studies show that mutations in this gene causes Charcot–Marie–Tooth neuoropathy or Dejerine–Sottas neuropathy.33 Both periaxins are thought to be essential for the formation and maintenance of peripheral nerve myelin and are differentially targeted in Schwann cells. In addition, L-periaxin is localized to the plasma membrane and S-periaxin is expressed in the cytoplasm.34
Table 1 The number of SNPs in different isoform categories and their representation in a clinical database
|
One-to-one |
PI-specific |
Overlaps |
NPI-specific |
Number of SNPs: the number of distinct SNPs in the 1000 Genomes Project at the corresponding isoform-specific coding regions.
ClinVar records: the number of 1KG SNPs that intersect with the ClinVar database in the corresponding isoform-specific coding regions. One-to-one: protein-coding genes that have only one protein-coding transcript. |
Stop-gain/loss |
Number of SNPsa |
1648 |
917 |
2744 |
468 |
ClinVar recordsb |
20 |
22 |
49 |
4 |
|
Non-synonymous |
Number of SNPsa |
75 220 |
48 223 |
159 554 |
11 526 |
ClinVar recordsb |
339 |
478 |
1353 |
37 |
|
Synonymous |
Number of SNPsa |
49 293 |
33 098 |
117 565 |
5353 |
ClinVar recordsb |
125 |
142 |
542 |
13 |
Table 2 The number of nucleotide base pairs in different isoform categories and their presence in a clinical database
|
One-to-one |
PI-specific |
Overlaps |
NPI-specific |
Region-length: the number of total base pairs in the corresponding coding region.
ClinVar records: the number of ClinVar records in the corresponding category.
|
Stop-gain/loss |
Region-lengtha |
4 097 316 |
3 165 334 |
11 367 942 |
668 004 |
ClinVar recordsb |
767 |
702 |
2734 |
36 |
|
Non-synonymous |
Region-lengtha |
4 097 316 |
3 165 334 |
11 367 942 |
668 004 |
ClinVar recordsb |
6278 |
5205 |
19 665 |
379 |
|
Synonymous |
Region-lengtha |
4 097 316 |
3 165 334 |
11 367 942 |
668 004 |
ClinVar recordsb |
1525 |
999 |
4043 |
126 |
Our results show that although NPI-specific regions were enriched in stop-gain and non-synonymous SNPs, the mutations in NPIs were less likely to be related to phenotypic changes. A recent study demonstrated that cancer-associated mutations are more likely to be located in structured regions than in disordered segments.35 This result is supported by our analysis at the isoform level: disease-associated variation showed a preference for PI-specific and overlapping regions.
Discussion
In this study, we analysed the distribution of human variation in different frequency classes among multiple coding transcripts in the same gene on a genome-wide scale. Our results showed that genetic variations are distributed unevenly across different coding isoforms. Amino-acid changing SNPs and frameshift indels are enriched in NPI-specific regions, particularly in high frequency regions.
Stop-gain SNPs and frameshift indels are some of the largest groups of LoF variants.17 Although studies have shown that a few stop-gain mutations might be beneficial for local adaptations or environmental changes,36,37 a large number of LoF variants are regarded to be deleterious and may reduce fitness.22 Approximately one-third of inherited genetic disorders as well as certain cancers result from premature termination codons.38 However, the human genome shows robustness with respect to these mutations. It has been estimated that a healthy individual's genome carries approximately 100 LoF variants.17,39 In addition, the human population has an abundance of non-synonymous SNPs, with the majority of them having not observed a functional consequence.15,21 After isolating the stop-gain SNPs from the missense dataset, the population variations were found to clearly display a non-uniform distribution of the isoforms across the allele frequency bins and substitution types. Using a specific example, a study showed that a premature termination codon mutation in the ZSCAN9 gene affected just one isoform of eight transcripts, and the main isoform was not affected by the PTC mutation.9 Our dataset demonstrates that this phenomenon is common in the human genome. In addition, we performed a Gene Ontology (GO)40 enrichment analysis on two types of genes: (i) stop-gain SNPs that were solely present in principal isoforms and (ii) stop-gain SNPs that were solely present in non-principal isoforms. The GO analysis revealed that the gene set of the stop-gain SNPs solely present in the PIs was functionally enriched for the sensory perception of chemical stimuli and olfactory receptor activity genes (Fig. 5a), which is in agreement with a previous study of LoF-containing genes.41,42 However, the gene set of the stop-gain SNPs solely present in the NPIs was enriched for purine nucleoside diphosphate metabolic processes and oxidoreductase activity on NAD(P)H (Fig. 5b).
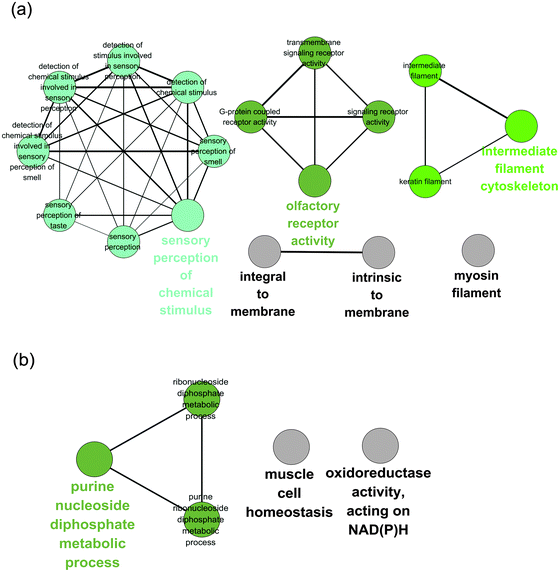 |
| Fig. 5 GO enrichment of two gene sets. (a) Stop-gain SNPs that were solely present in PI-specific regions. (b) Stop-gain SNPs that were solely present in PI-specific regions. The gene set of figure (a) enriched for the sensory perception and olfactory receptor activity genes as previously reported, while the intermediated filament genes were appeared in the enrichment result. The gene set of figure (b) enriched in genes with different functions. | |
Besides, we also performed the GO enrichment analysis on the genes that contain non-synonymous SNPs in the PI-specific regions and the NPI-specific regions, separately (Fig. S3, ESI†). The enrichment results of the non-synonymous SNPs are similar to those of the stop-gain variations in the PI-specific gene set (Fig. 5a and Fig. S3a, ESI†). Interestingly, except for the olfactory receptors, which are well-known for their high tolerance of loss function mutations (Fig. 5a), our results also suggest that the genes that encode intermediate filaments have similar effects from the stop-gain and non-synonymous SNPs (Fig. 5a and Fig. S3a, ESI†). We speculated that this might have occurred due to the functional redundancy of the intermediate filament genes, because there are more than 70 members within this gene family in the human genome.43,44 Different transcript isoforms may have different tolerances for stop-gain mutations. This suggests that when analysing genes containing mutations, an affected isoform should be considered more carefully than a protein-coding variant that resides in a minor coding transcript, which might have only a limited impact on the phenotype.
Furthermore, because the 1000 Genomes Project used an exome sequencing strategy, we considered the possibility of a detection bias towards overlapping and PI-specific regions. First, the target region of whole exome sequencing in the 1000 Genome Project includes the Consensus Coding Sequence (CCDS)45 regions and an extension of 50 bp on both sides; our analysis contains only full-length protein-coding transcripts, thus our analysed coding regions were highly overlapped with exome target regions. Second, the SNPs sampling was completed independent of substitution types, whereas the variation pattern represents significant changes among substitution types. Therefore, we believe that the observed pattern is unlikely to be affected by sampling bias.
In summary, our analyses delineate an uneven distribution of genetic variation in human coding genes. Previous results showed that human alternatively spliced exons might be subjected to a relaxed selective pressure or positive selection.23,24 In this study, by isolating stop-gain SNPs via missense substitution, we obtained results further supporting the relaxation of negative selective pressure on alternative coding regions by AS. We also found an increased frequency and the number of NPI-specific stop-gain SNPs, suggesting that the number of functional alternatively spliced proteins may be smaller than that thought previously. Finally, genetic variation, as a rapid-growing data source, has the potential to supply independent evidence for evaluating the functional probabilities of splicing isoforms.
Material and methods
Data sources of population variation and gene annotation
The human variation data were downloaded from the 1000 Genomes Project web site http://ftp.1000genomes.ebi.ac.uk/vol1/ftp/release/20110521 (Phase I, April 30th, 2012 Version 3 amendments release). Both low-coverage and exome data from autosomes and the X-chromosome were included in the dataset. The derived allele frequencies were determined by comparing ancestral states to reference and alternative alleles. For the 1KG project, the frequency of an allele is defined as the frequency of the alternative allele against the reference genome. Thus, if the ancestral allele is in concert with the reference one, the derived allele frequency (DAF) will then be the same as the alternative one; otherwise, it is equal to one minus the alternative frequency. The ancestral allele states were retrieved from VCF files that were originally identified via a 6-way EPO alignment.46
The gene and transcript annotations were based on GENCODE release 15 (Ensembl70).14 We retained protein-coding genes with at least one full-length protein-coding transcript for analyses. The term ‘full-length protein-coding transcript’ indicates that the start and the end of the coding region were both confirmed. Our multi-transcript gene set comprises 13
625 protein-coding genes, and our one-to-one gene set comprises 6500 protein-coding genes. The variation and genome annotation data sets were based on NCBI's human reference genome build 37 (GRCh37).
Identification of coding variation
Due to the large number of variation records, we built a local database to easily store and query the variation data, and developed a Perl over DBI pipeline to link variation records and genome annotations using genomic coordinates. After this step, the variations were mapped to coding transcripts and gene parents. Only full-length protein-coding transcripts were kept for mapping the coding variations. All the coding SNPs were classified into non-synonymous, synonymous and stop-gain/loss types according to the amino acid changes introduced by alternative alleles. In total, 206
221 synonymous and 295
997 non-synonymous SNPs were obtained, and 199
417 synonymous and 285
142 non-synonymous SNPs were associated with their ancestral states to infer their derived allele frequencies. The stop-gain/loss SNPs were divided into stop-gain and stop-loss SNPs by examining their ancestral states. In addition, 941 frameshift indels were identified using the same pipeline via the addition of information regarding indel length and exon boundaries.
Classification of the PI-specific, NPI-specific, and overlapping regions and variation
The annotations of the principal isoforms were downloaded from the APPRIS web site, Gencode15 release (http://appris.bioinfo.cnio.es). The principal isoforms were assigned in accordance with APPRIS annotations. For those genes without a clear principal isoform prediction, we used their longest candidates according to APPRIS. In addition, we kept only full-length protein-coding transcripts as NPI candidates.
The CDS region of each gene was classified into PI-specific, NPI-specific and overlapping regions. For each gene, the non-synonymous, synonymous, stop-gain SNPs and indels were mapped to these three coding categories.
Randomised experiments
We sampled a subset of the protein-coding genes with NPI-specific regions for each iteration. We then randomly relocated the genomic coordinate of the SNPs in the coding regions of each gene in the stop-gain and non-synonymous datasets separately. For each amino acid substitution category, the ratio of the number of NPI-specific stop-gain SNPs to the total number of stop-gain SNPs in the sampled gene set helped us to determine the extent to which the SNPs were found to be concentrated in the NPI-specific regions for each amino acid substitution category. This process was performed 1000 times for each gene subset. We found that for sampled gene numbers ranging from 5% to 30% of the total gene pool using a 5% step size, as the number of sampled genes increased, the number of random ratios smaller than the observed ratios increased as well (Table S2, ESI†).
Statistical analysis
The significant differences in the SNP numbers of different coding regions were calculated using a chi-square test for given probabilities with simulated p-values (based on 2000 replicates), the given probabilities assigned to be the percentage of each isoform category length (e.g., PI-specific region length vs. total coding length) for multi-transcript genes. We used Fisher's exact test for comparing the clinical records of the coding region SNPs. The derived allele-frequencies of the PI-specific and NPI-specific SNPs were compared with the Mann–Whitney U-test. All of the statistical tests were implemented in R (http://www.r-project.org/).
The clinically relevant variation (ClinVar) resource
To link genotypes to phenotypes, we used ClinVar (http://ftp://ftp.ncbi.nih.gov/pub/clinvar/; released 20131230),32 a public and easily accessible resource of the relationships between human genetic variation and phenotypic changes based on supporting evidence. These variations are assumed to be functionally relevant. ClinVar currently contains 46
094 reports collected from OMIM, GeneReviews, dbSNP, etc. (unreviewed GWAS data were not included). Using ClinVar's VCF format, we were able to easily compare the 1KG SNPs to ClinVar reports based on genomic locations and dbSNPs47 IDs.
GO analyses
The GO analyses were performed using ClueGO (v1.7.1),48 which is a Cytoscape (2.8.3)49 plug-in for gene ontology annotation. ClueGO can be used to easily combine several functionally related GO terms into groups and visualise them. The groups are represented by the terms with the highest significance. We used an adjusted P-value of 0.01 (Benjamini and Hochberg correction50) as a threshold to identify significant GO terms.
Acknowledgements
We thank the two anonymous reviewers for their valuable suggestions and comments. We are grateful to Zhen Li, Erli Pang, Tao Zhu, Jia Song and Professor Deng-Ke Niu for their helpful discussions and suggestions. We could not have finished this study without your help. This research was supported by NSFC Grant No. 31171235.
References
- B. R. Graveley, Trends Genet., 2001, 17, 100–107 CrossRef CAS.
- J. M. Johnson, J. Castle, P. Garrett-Engele, Z. Kan, P. M. Loerch, C. D. Armour, R. Santos, E. E. Schadt, R. Stoughton and D. D. Shoemaker, Science, 2003, 302, 2141–2144 CrossRef CAS PubMed.
- Q. Pan, O. Shai, L. J. Lee, B. J. Frey and B. J. Blencowe, Nat. Genet., 2008, 40, 1413–1415 CrossRef CAS PubMed.
- F. Birzele, G. Csaba and R. Zimmer, Nucleic Acids Res., 2008, 36, 550–558 CrossRef CAS PubMed.
- T. W. Nilsen and B. R. Graveley, Nature, 2010, 463, 457–463 CrossRef CAS PubMed.
- M. L. Tress, P. L. Martelli, A. Frankish, G. A. Reeves, J. J. Wesselink, C. Yeats, P. Ísólfur Ólason, M. Albrecht, H. Hegyi and A. Giorgetti, Proc. Natl. Acad. Sci. U. S. A., 2007, 104, 5495–5500 CrossRef CAS PubMed.
- R. Sorek, R. Shamir and G. Ast, Trends Genet., 2004, 20, 68–71 CrossRef CAS PubMed.
- E. Melamud and J. Moult, Nucleic Acids Res., 2009, 37, 4873–4886 CrossRef CAS PubMed.
- J. M. Mudge, A. Frankish and J. Harrow, Genome Res., 2013, 23, 1961–1973 CrossRef CAS PubMed.
- M. L. Tress, J.-J. Wesselink, A. Frankish, G. López, N. Goldman, A. Löytynoja, T. Massingham, F. Pardi, S. Whelan and J. Harrow, Bioinformatics, 2008, 24, 11–17 CrossRef CAS PubMed.
- J. M. Rodriguez, P. Maietta, I. Ezkurdia, A. Pietrelli, J.-J. Wesselink, G. Lopez, A. Valencia and M. L. Tress, Nucleic Acids Res., 2013, 41, D110–D117 CrossRef CAS PubMed.
- M. Gonzàlez-Porta, A. Frankish, J. Rung, J. Harrow and A. Brazma, Genome Biol., 2013, 14, R70 CrossRef PubMed.
- P. Flicek, M. R. Amode, D. Barrell, K. Beal, S. Brent, D. Carvalho-Silva, P. Clapham, G. Coates, S. Fairley and S. Fitzgerald, Nucleic Acids Res., 2012, 40, D84–D90 CrossRef CAS PubMed.
- J. Harrow, A. Frankish, J. M. Gonzalez, E. Tapanari, M. Diekhans, F. Kokocinski, B. L. Aken, D. Barrell, A. Zadissa and S. Searle, Genome Res., 2012, 22, 1760–1774 CrossRef CAS PubMed.
- G. A. McVean, D. M. Altshuler Co-Chair, R. M. Durbin Co-Chair, G. R. Abecasis, D. R. Bentley, A. Chakravarti, A. G. Clark, P. Donnelly, E. E. Eichler, P. Flicek, S. B. Gabriel, R. A. Gibbs, E. D. Green, M. E. Hurles, B. M. Knoppers, J. O. Korbel, E. S. Lander, C. Lee, H. Lehrach, E. R. Mardis, G. T. Marth, G. A. McVean, D. A. Nickerson, J. P. Schmidt, S. T. Sherry, J. Wang, R. K. Wilson, R. A. Gibbs Principal Investigator, H. Dinh, C. Kovar, S. Lee, L. Lewis, D. Muzny, J. Reid, M. Wang, J. Wang Principal Investigator, X. Fang, X. Guo, M. Jian, H. Jiang, X. Jin, G. Li, J. Li, Y. Li, Z. Li, X. Liu, Y. Lu, X. Ma, Z. Su, S. Tai, M. Tang, B. Wang, G. Wang, H. Wu, R. Wu, Y. Yin, W. Zhang, J. Zhao, M. Zhao, X. Zheng, Y. Zhou, E. S. Lander Principal Investigator, D. M. Altshuler, S. B. Gabriel Co-Chair, N. Gupta, P. Flicek Principal Investigator, L. Clarke, R. Leinonen, R. E. Smith, X. Zheng-Bradley, D. R. Bentley Principal Investigator, R. Grocock, S. Humphray, T. James, Z. Kingsbury, H. Lehrach Principal Investigator, R. Sudbrak Project Leader, M. W. Albrecht, V. S. Amstislavskiy, T. A. Borodina, M. Lienhard, F. Mertes, M. Sultan, B. Timmermann, M.-L. Yaspo, S. T. Sherry Principal Investigator, G. A. McVean Principal Investigator, E. R. Mardis Co-Principal Investigator Co-Chair, R. K. Wilson Co-Principal Investigator, L. Fulton, R. Fulton, G. M. Weinstock, R. M. Durbin Principal Investigator, S. Balasubramaniam, J. Burton, P. Danecek, T. M. Keane, A. Kolb-Kokocinski, S. McCarthy, J. Stalker, M. Quail, J. P. Schmidt Principal Investigator, C. J. Davies, J. Gollub, T. Webster, B. Wong, Y. Zhan, A. Auton Principal Investigator, R. A. Gibbs Principal Investigator, F. Yu Project Leader, M. Bainbridge, D. Challis, U. S. Evani, J. Lu, D. Muzny, U. Nagaswamy, J. Reid, A. Sabo, Y. Wang, J. Yu, J. Wang Principal Investigator, L. J. M. Coin, L. Fang, X. Guo, X. Jin, G. Li, Q. Li, Y. Li, Z. Li, H. Lin, B. Liu, R. Luo, N. Qin, H. Shao, B. Wang, Y. Xie, C. Ye, C. Yu, F. Zhang, H. Zheng, H. Zhu, G. T. Marth Principal Investigator, E. P. Garrison, D. Kural, W.-P. Lee, W. Fung Leong, A. N. Ward, J. Wu, M. Zhang, C. Lee Principal Investigator, L. Griffin, C.-H. Hsieh, R. E. Mills, X. Shi, M. von Grotthuss, C. Zhang, M. J. Daly Principal Investigator, M. A. DePristo Project Leader, D. M. Altshuler, E. Banks, G. Bhatia, M. O. Carneiro, G. del Angel, S. B. Gabriel, G. Genovese, N. Gupta, R. E. Handsaker, C. Hartl, E. S. Lander, S. A. McCarroll, J. C. Nemesh, R. E. Poplin, S. F. Schaffner, K. Shakir, S. C. Yoon Principal Investigator, J. Lihm, V. Makarov, H. Jin Principal Investigator, W. Kim, K. Cheol Kim, J. O. Korbel Principal Investigator, T. Rausch, P. Flicek Principal Investigator, K. Beal, L. Clarke, F. Cunningham, J. Herrero, W. M. McLaren, G. R. S. Ritchie, R. E. Smith, X. Zheng-Bradley, A. G. Clark Principal Investigator, S. Gottipati, A. Keinan, J. L. Rodriguez-Flores, P. C. Sabeti Principal Investigator, S. R. Grossman, S. Tabrizi, R. Tariyal, D. N. Cooper Principal Investigator, E. V. Ball, P. D. Stenson, D. R. Bentley Principal Investigator, B. Barnes, M. Bauer, R. Keira Cheetham, T. Cox, M. Eberle, S. Humphray, S. Kahn, L. Murray, J. Peden, R. Shaw, K. Ye Principal Investigator, M. A. Batzer Principal Investigator, M. K. Konkel, J. A. Walker, D. G. MacArthur Principal Investigator, M. Lek, S. P. Leader, V. S. Amstislavskiy, R. Herwig, M. D. Shriver Principal Investigator, C. D. Bustamante Principal Investigator, J. K. Byrnes, F. M. De La Vega, S. Gravel, E. E. Kenny, J. M. Kidd, P. Lacroute, B. K. Maples, A. Moreno-Estrada, F. Zakharia, E. Halperin Principal Investigator, Y. Baran, D. W. Craig Principal Investigator, A. Christoforides, N. Homer, T. Izatt, A. A. Kurdoglu, S. A. Sinari, K. Squire, S. T. Sherry Principal Investigator, C. Xiao, J. Sebat Principal Investigator, V. Bafna, K. Ye, E. G. Burchard Principal Investigator, R. D. Hernandez Principal Investigator, C. R. Gignoux, D. Haussler Principal Investigator, S. J. Katzman, W. James Kent, B. Howie, A. Ruiz-Linares Principal Investigator, E. T. Dermitzakis Principal Investigator, T. Lappalainen, S. E. Devine Principal Investigator, X. Liu, A. Maroo, L. J. Tallon, J. A. Rosenfeld Principal Investigator, L. P. Michelson, G. R. Abecasis Principal Investigator Co-Chair, H. Min Kang Project Leader, P. Anderson, A. Angius, A. Bigham, T. Blackwell, F. Busonero, F. Cucca, C. Fuchsberger, C. Jones, G. Jun, Y. Li, R. Lyons, A. Maschio, E. Porcu, F. Reinier, S. Sanna, D. Schlessinger, C. Sidore, A. Tan, M. Kate Trost, P. Awadalla Principal Investigator, A. Hodgkinson, G. Lunter Principal Investigator, G. A. McVean Principal Investigator Co-Chair, J. L. Marchini Principal Investigator, S. Myers Principal Investigator, C. Churchhouse, O. Delaneau, A. Gupta-Hinch, Z. Iqbal, I. Mathieson, A. Rimmer, D. K. Xifara, T. K. Oleksyk Principal Investigator, Y. Fu Principal Investigator, X. Liu, M. Xiong, L. Jorde Principal Investigator, D. Witherspoon, J. Xing, E. E. Eichler Principal Investigator, B. L. Browning Principal Investigator, C. Alkan, I. Hajirasouliha, F. Hormozdiari, A. Ko, P. H. Sudmant, E. R. Mardis Co-Principal Investigator, K. Chen, A. Chinwalla, L. Ding, D. Dooling, D. C. Koboldt, M. D. McLellan, J. W. Wallis, M. C. Wendl, Q. Zhang, R. M. Durbin Principal Investigator, M. E. Hurles Principal Investigator, C. A. Albers, Q. Ayub, S. Balasubramaniam, Y. Chen, A. J. Coffey, V. Colonna, P. Danecek, N. Huang, L. Jostins, T. M. Keane, H. Li, S. McCarthy, A. Scally, J. Stalker, K. Walter, Y. Xue, Y. Zhang, M. B. Gerstein Principal Investigator, A. Abyzov, S. Balasubramanian, J. Chen, D. Clarke, Y. Fu, L. Habegger, A. O. Harmanci, M. Jin, E. Khurana, X. Jasmine Mu, C. Sisu, Y. Li, R. Luo, H. Zhu, C. Lee Principal Investigator Co-Chair, L. Griffin, C.-H. Hsieh, R. E. Mills, X. Shi, M. von Grotthuss, C. Zhang, G. T. Marth Principal Investigator, E. P. Garrison, D. Kural, W.-P. Lee, A. N. Ward, J. Wu, M. Zhang, S. A. McCarroll Project Leader, D. M. Altshuler, E. Banks, G. del Angel, G. Genovese, R. E. Handsaker, C. Hartl, J. C. Nemesh, K. Shakir, S. C. Yoon Principal Investigator, J. Lihm, V. Makarov, J. Degenhardt, P. Flicek Principal Investigator, L. Clarke, R. E. Smith, X. Zheng-Bradley, J. O. Korbel Principal Investigator Co-Chair, T. Rausch, A. M. Stütz, D. R. Bentley Principal Investigator, B. Barnes, R. Keira Cheetham, M. Eberle, S. Humphray, S. Kahn, L. Murray, R. Shaw, K. Ye Principal Investigator, M. A. Batzer Principal Investigator, M. K. Konkel, J. A. Walker, P. Lacroute, D. W. Craig Principal Investigator, N. Homer, D. Church, C. Xiao, J. Sebat Principal Investigator, V. Bafna, J. J. Michaelson, K. Ye, S. E. Devine Principal Investigator, X. Liu, A. Maroo, L. J. Tallon, G. Lunter Principal Investigator, G. A. McVean Principal Investigator, Z. Iqbal, D. Witherspoon, J. Xing, E. E. Eichler Principal Investigator Co-Chair, C. Alkan, I. Hajirasouliha, F. Hormozdiari, A. Ko, P. H. Sudmant, K. Chen, A. Chinwalla, L. Ding, M. D. McLellan, J. W. Wallis, M. E. Hurles Principal Investigator Co-Chair, B. Blackburne, H. Li, S. J. Lindsay, Z. Ning, A. Scally, K. Walter, Y. Zhang, M. B. Gerstein Principal Investigator, A. Abyzov, J. Chen, D. Clarke, E. Khurana, X. Jasmine Mu, C. Sisu, R. A. Gibbs Principal Investigator Co-Chair, F. Yu Project Leader, M. Bainbridge, D. Challis, U. S. Evani, C. Kovar, L. Lewis, J. Lu, D. Muzny, U. Nagaswamy, J. Reid, A. Sabo, J. Yu, X. Guo, Y. Li, R. Wu, G. T. Marth Principal Investigator Co-Chair, E. P. Garrison, W. Fung Leong, A. N. Ward, G. del Angel, M. A. DePristo, S. B. Gabriel, N. Gupta, C. Hartl, R. E. Poplin, A. G. Clark Principal Investigator, J. L. Rodriguez-Flores, P. Flicek Principal Investigator, L. Clarke, R. E. Smith, X. Zheng-Bradley, D. G. MacArthur Principal Investigator, C. D. Bustamante Principal Investigator, S. Gravel, D. W. Craig Principal Investigator, A. Christoforides, N. Homer, T. Izatt, S. T. Sherry Principal Investigator, C. Xiao, E.
T. Dermitzakis Principal Investigator, G. R. Abecasis Principal Investigator, H. Min Kang, G. A. McVean Principal Investigator, E. R. Mardis Principal Investigator, D. Dooling, L. Fulton, R. Fulton, D. C. Koboldt, R. M. Durbin Principal Investigator, S. Balasubramaniam, T. M. Keane, S. McCarthy, J. Stalker, M. B. Gerstein Principal Investigator, S. Balasubramanian, L. Habegger, E. P. Garrison, R. A. Gibbs Principal Investigator, M. Bainbridge, D. Muzny, F. Yu, J. Yu, G. del Angel, R. E. Handsaker, V. Makarov, J. L. Rodriguez-Flores, H. Jin Principal Investigator, W. Kim, K. Cheol Kim, P. Flicek Principal Investigator, K. Beal, L. Clarke, F. Cunningham, J. Herrero, W. M. McLaren, G. R. S. Ritchie, X. Zheng-Bradley, S. Tabrizi, D. G. MacArthur Principal Investigator, M. Lek, C. D. Bustamante Principal Investigator, F. M. De La Vega, D. W. Craig Principal Investigator, A. A. Kurdoglu, T. Lappalainen, J. A. Rosenfeld Principal Investigator, L. P. Michelson, P. Awadalla Principal Investigator, A. Hodgkinson, G. A. McVean Principal Investigator, K. Chen, Y. Chen, V. Colonna, A. Frankish, J. Harrow, Y. Xue, M. B. Gerstein Principal Investigator Co-Chair, A. Abyzov, S. Balasubramanian, J. Chen, D. Clarke, Y. Fu, A. O. Harmanci, M. Jin, E. Khurana, X. Jasmine Mu, C. Sisu, R. A. Gibbs Principal Investigator, G. Fowler, W. Hale, D. Kalra, C. Kovar, D. Muzny, J. Reid, J. Wang Principal Investigator, X. Guo, G. Li, Y. Li, X. Zheng, D. M. Altshuler, P. Flicek Principal Investigator Co-Chair, L. Clarke Project Leader, J. Barker, G. Kelman, E. Kulesha, R. Leinonen, W. M. McLaren, R. Radhakrishnan, A. Roa, D. Smirnov, R. E. Smith, I. Streeter, I. Toneva, B. Vaughan, X. Zheng-Bradley, D. R. Bentley Principal Investigator, T. Cox, S. Humphray, S. Kahn, R. Sudbrak Project Leader, M. W. Albrecht, M. Lienhard, D. W. Craig Principal Investigator, T. Izatt, A. A. Kurdoglu, S. T. Sherry Principal Investigator Co-Chair, V. Ananiev, Z. Belaia, D. Beloslyudtsev, N. Bouk, C. Chen, D. Church, R. Cohen, C. Cook, J. Garner, T. Hefferon, M. Kimelman, C. Liu, J. Lopez, P. Meric, C. O'Sullivan, Y. Ostapchuk, L. Phan, S. Ponomarov, V. Schneider, E. Shekhtman, K. Sirotkin, D. Slotta, C. Xiao, H. Zhang, D. Haussler Principal Investigator, G. R. Abecasis Principal Investigator, G. A. McVean Principal Investigator, C. Alkan, A. Ko, D. Dooling, R. M. Durbin Principal Investigator, S. Balasubramaniam, T. M. Keane, S. McCarthy, J. Stalker, A. Chakravarti Co-Chair, B. M. Knoppers Co-Chair, G. R. Abecasis, K. C. Barnes, C. Beiswanger, E. G. Burchard, C. D. Bustamante, H. Cai, H. Cao, R. M. Durbin, N. Gharani, R. A. Gibbs, C. R. Gignoux, S. Gravel, B. Henn, D. Jones, L. Jorde, J. S. Kaye, A. Keinan, A. Kent, A. Kerasidou, Y. Li, R. Mathias, G. A. McVean, A. Moreno-Estrada, P. N. Ossorio, M. Parker, D. Reich, C. N. Rotimi, C. D. Royal, K. Sandoval, Y. Su, R. Sudbrak, Z. Tian, B. Timmermann, S. Tishkoff, L. H. Toji, C. Tyler Smith, M. Via, Y. Wang, H. Yang, L. Yang, J. Zhu, W. Bodmer, G. Bedoya, A. Ruiz-Linares, C. Zhi Ming, G. Yang, C. Jia You, L. Peltonen, A. Garcia-Montero, A. Orfao, J. Dutil, J. C. Martinez-Cruzado, T. K. Oleksyk, L. D. Brooks, A. L. Felsenfeld, J. E. McEwen, N. C. Clemm, A. Duncanson, M. Dunn, E. D. Green, M. S. Guyer, J. L. Peterson, G. R. Abecasis, A. Auton, L. D. Brooks, M. A. DePristo, R. M. Durbin, R. E. Handsaker, H. Min Kang, G. T. Marth and G. A. McVean, Nature, 2012, 491, 56–65 CrossRef CAS PubMed.
- E. Khurana, Y. Fu, V. Colonna, X. J. Mu, H. M. Kang, T. Lappalainen, A. Sboner, L. Lochovsky, J. Chen and A. Harmanci, Science, 2013, 342, 1235587 CrossRef PubMed.
- D. G. MacArthur, S. Balasubramanian, A. Frankish, N. Huang, J. Morris, K. Walter, L. Jostins, L. Habegger, J. K. Pickrell, S. B. Montgomery, C. A. Albers, Z. D. Zhang, D. F. Conrad, G. Lunter, H. Zheng, Q. Ayub, M. A. Depristo, E. Banks, M. Hu, R. E. Handsaker, J. A. Rosenfeld, M. Fromer, M. Jin, X. J. Mu, E. Khurana, K. Ye, M. Kay, G. I. Saunders, M. M. Suner, T. Hunt, I. H. A. Barnes, C. Amid, D. R. Carvalho-Silva, A. H. Bignell, C. Snow, B. Yngvadottir, S. Bumpstead, D. N. Cooper, Y. Xue, I. G. Romero, G. P. Consortium, J. Wang, Y. Li, R. A. Gibbs, S. A. McCarroll, E. T. Dermitzakis, J. K. Pritchard, J. C. Barrett, J. Harrow, M. E. Hurles, M. B. Gerstein and C. Tyler-Smith, Science, 2012, 335, 823–828 CrossRef CAS PubMed.
-
Y. Xue, Y. Chen, Q. Ayub, N. Huang, E. V. Ball, M. Mort, A. D. Phillips, K. Shaw, P. D. Stenson, D. N. Cooper, C. Tyler Smith and T. G. P. Consortium, The American Journal of Human Genetics, The American Society of Human Genetics, 2012, vol. 91, pp. 1022–1032 Search PubMed.
- S. Savas, S. Tuzmen and H. Ozcelik, Hum. Genomics, 2006, 2, 274–286 CrossRef CAS PubMed.
- B. Yngvadottir, Y. Xue, S. Searle, S. Hunt, M. Delgado, J. Morrison, P. Whittaker, P. Deloukas and C. Tyler-Smith, Am. J. Hum. Genet., 2009, 84, 224–234 CrossRef CAS PubMed.
- P. C. Ng and S. Henikoff, Annu. Rev. Genomics Hum. Genet., 2006, 7, 61–80 CrossRef CAS PubMed.
- I. P. Gorlov, M. Kimmel and C. I. Amos, Hum. Mol. Genet., 2006, 15, 1143–1150 CrossRef CAS PubMed.
- Y. Xing and C. Lee, Proc. Natl. Acad. Sci. U. S. A., 2005, 102, 13526–13531 CrossRef CAS PubMed.
- V. E. Ramensky, R. N. Nurtdinov, A. D. Neverov, A. A. Mironov and M. S. Gelfand, Am. J. Hum. Genet., 2008, 83, 94–98 CrossRef CAS PubMed.
- A. Keinan and A. G. Clark, Science, 2012, 336, 740–743 CrossRef CAS PubMed.
- A. R. Boyko, S. H. Williamson, A. R. Indap, J. D. Degenhardt, R. D. Hernandez, K. E. Lohmueller, M. D. Adams, S. Schmidt, J. J. Sninsky, S. R. Sunyaev, T. J. White, R. Nielsen, A. G. Clark and C. D. Bustamante, PLoS Genet., 2008, 4, e1000083 Search PubMed.
- B. P. Lewis, R. E. Green and S. E. Brenner, Proc. Natl. Acad. Sci. U. S. A., 2003, 100, 189–192 CrossRef CAS PubMed.
- J. Rehwinkel, I. Letunic, J. Raes, P. Bork and E. Izaurralde, RNA, 2005, 11, 1530–1544 CrossRef CAS PubMed.
- L. E. Maquat, Nat. Rev. Mol. Cell Biol., 2004, 5, 89–99 CrossRef CAS PubMed.
- E. Nagy and L. E. Maquat, Trends Biochem. Sci., 1998, 23, 198–199 CrossRef CAS.
- Y. Xing and C. J. Lee, Trends Genet., 2004, 20, 472–475 CrossRef CAS PubMed.
- M. J. Landrum, J. M. Lee, G. R. Riley, W. Jang, W. S. Rubinstein, D. M. Church and D. R. Maglott, Nucleic Acids Res., 2014, 42, D980–D985 CrossRef CAS PubMed.
- A. Guilbot, A. Williams, N. Ravise, C. Verny, A. Brice, D. L. Sherman, P. J. Brophy, E. LeGuern, V. Delague, C. Bareil, A. Megarbane and M. Claustres, Hum. Mol. Genet., 2001, 10, 415–421 CrossRef CAS PubMed.
- L. Dytrych, D. L. Sherman, C. S. Gillespie and P. J. Brophy, J. Biol. Chem., 1998, 273, 5794–5800 CrossRef CAS PubMed.
- M. Pajkos, B. Meszaros, I. Simon and Z. Dosztanyi, Mol. BioSyst., 2012, 8, 296–307 RSC.
- M. V. Olson, Am. J. Hum. Genet., 1999, 64, 18 CrossRef CAS PubMed.
- K. R. Veeramah, M. G. Thomas, M. E. Weale, D. Zeitlyn, A. Tarekegn, E. Bekele, N. R. Mendell, E. A. Shephard, N. Bradman and I. R. Phillips, Pharmacogenet. Genomics, 2008, 18, 877 CrossRef CAS PubMed.
- P. A. Frischmeyer and H. C. Dietz, Hum. Mol. Genet., 1999, 8, 1893–1900 CrossRef CAS PubMed.
- D. G. MacArthur and C. Tyler-Smith, Hum. Mol. Genet., 2010, 19, R125–R130 CrossRef CAS PubMed.
- M. Ashburner, C. A. Ball, J. A. Blake, D. Botstein, H. Butler, J. M. Cherry, A. P. Davis, K. Dolinski, S. S. Dwight, J. T. Eppig, M. A. Harris, D. P. Hill, L. Issel-Tarver, A. Kasarskis, S. Lewis, J. C. Matese, J. E. Richardson, M. Ringwald, G. M. Rubin, G. Sherlock and C. Gene Ontology, Nat. Genet., 2000, 25, 25–29 CrossRef CAS PubMed.
- X. Wang, S. D. Thomas and J. Zhang, Hum. Mol. Genet., 2004, 13, 2671–2678 CrossRef CAS PubMed.
- Y. Gilad, O. Man, S. Pääbo and D. Lancet, Proc. Natl. Acad. Sci. U. S. A., 2003, 100, 3324–3327 CrossRef CAS PubMed.
- M. Hesse, T. M. Magin and K. Weber, J. Cell Sci., 2001, 114, 2569–2575 CAS.
- I. Szeverenyi, A. J. Cassidy, C. W. Chung, B. T. K. Lee, J. E. A. Common, S. C. Ogg, H. Chen, S. Y. Sim, W. L. P. Goh and K. W. Ng, Hum. Mutat., 2008, 29, 351–360 CrossRef CAS PubMed.
- K. D. Pruitt, J. Harrow, R. A. Harte, C. Wallin, M. Diekhans, D. R. Maglott, S. Searle, C. M. Farrell, J. E. Loveland, B. J. Ruef, E. Hart, M.-M. Suner, M. J. Landrum, B. Aken, S. Ayling, R. Baertsch, J. Fernandez-Banet, J. L. Cherry, V. Curwen, M. DiCuccio, M. Kellis, J. Lee, M. F. Lin, M. Schuster, A. Shkeda, C. Amid, G. Brown, O. Dukhanina, A. Frankish, J. Hart, B. L. Maidak, J. Mudge, M. R. Murphy, T. Murphy, J. Rajan, B. Rajput, L. D. Riddick, C. Snow, C. Steward, D. Webb, J. A. Weber, L. Wilming, W. Wu, E. Birney, D. Haussler, T. Hubbard, J. Ostell, R. Durbin and D. Lipman, Genome Res., 2009, 19, 1316–1323 CrossRef CAS PubMed.
- B. Paten, J. Herrero, K. Beal, S. Fitzgerald and E. Birney, Genome Res., 2008, 18, 1814–1828 CrossRef CAS PubMed.
- S. T. Sherry, M.-H. Ward, M. Kholodov, J. Baker, L. Phan, E. M. Smigielski and K. Sirotkin, Nucleic Acids Res., 2001, 29, 308–311 CrossRef CAS PubMed.
- G. Bindea, B. Mlecnik, H. Hackl, P. Charoentong, M. Tosolini, A. Kirilovsky, W.-H. Fridman, F. Pages, Z. Trajanoski and J. Galon, Bioinformatics, 2009, 25, 1091–1093 CrossRef CAS PubMed.
- P. Shannon, A. Markiel, O. Ozier, N. S. Baliga, J. T. Wang, D. Ramage, N. Amin, B. Schwikowski and T. Ideker, Genome Res., 2003, 13, 2498–2504 CrossRef CAS PubMed.
- Y. Benjamini and Y. Hochberg, Journal of the Royal Statistical Society. Series B (Methodological), 1995, 289–300 Search PubMed.
Footnote |
† Electronic supplementary information (ESI) available: Tables S1–S4; Fig. S1–S3. Supplementary file 1: a full list of stop-gain/loss SNPs. Supplementary file 2: the coordinates of each isoform-specific coding region. See DOI: 10.1039/c5mb00132c |
|
This journal is © The Royal Society of Chemistry 2015 |
Click here to see how this site uses Cookies. View our privacy policy here.