DOI:
10.1039/C4MB00478G
(Paper)
Mol. BioSyst., 2015,
11, 126-136
Identification of cancer-related lncRNAs through integrating genome, regulome and transcriptome features†
Received
9th August 2014
, Accepted 22nd October 2014
First published on 22nd October 2014
Abstract
LncRNAs have become rising stars in biology and medicine, due to their versatile functions in a wide range of important biological processes and active roles in various human cancers. Here, we developed a computational method based on the naïve Bayesian classifier method to identify cancer-related lncRNAs by integrating genome, regulome and transcriptome data, and identified 707 potential cancer-related lncRNAs. We demonstrated the performance of the method by ten-fold cross-validation, and found that integration of multi-omic data was necessary to identify cancer-related lncRNAs. We identified 707 potential cancer-related lncRNAs and our results showed that these lncRNAs tend to exhibit significant differential expression and differential DNA methylation in multiple cancer types, and prognosis effects in prostate cancer. We also found that these lncRNAs were more likely to be direct targets of TP53 family members than others. Moreover, based on 147 lncRNA knockdown data in mice, we validated that four of six mouse orthologous lncRNAs were significantly involved in many cancer-related processes, such as cell differentiation and the Wnt signaling pathway. Notably, one lncRNA, lnc-SNURF-1, which was found to be associated with TNF-mediated signaling pathways, was up-regulated in prostate cancer and the protein-coding genes affected by knockdown of the lncRNA were also significantly aberrant in prostate cancer patients, suggesting its probable importance in tumorigenesis. Taken together, our method underlines the power of integrating multi-omic data to uncover cancer-related lncRNAs.
Introduction
Most of the genome is transcribed, less than 2% of which is protein-coding.1 The vast majority of the genome-wide transcriptional repertoire is composed of non-coding RNAs (ncRNAs), which were previously thought to be junk without functions and thus are largely underestimated. Among these ncRNAs, long non-coding RNAs (lncRNAs) longer than 200 nucleotides without a clearly defined open reading frame are currently attracting considerable attention.2
Growing evidence suggests that lncRNAs are involved in diverse biological processes and play important molecular mechanistic roles in fundamental functions.2 They can function as key regulators of transcription through changing chromatin architecture or modulating the activity of transcription factors to activate or repress genes. Specifically, lncRNAs can recruit and guide chromatin-modifying complexes to target genes, and mediate looping to change the 3-dimensional structure of chromatin to regulate transcription. They can also act as signals and decoys to control key transcription factors, and scaffolds to assemble chromatin-modifying complexes for the activation and repression of genes.3,4
Accumulating evidence of dysregulated lncRNAs expression and genetic variants in lncRNAs in many cancers5–7 implies their potential oncogenic or tumor-suppressor roles in the development of cancer. LncRNAs can participate in aberrations of epigenetic status, such as changes in DNA methylation, histone modifications and chromatin remodeling, to silence or activate transcription of tumor-associated genes.1,8–10 LncRNAs have also been shown to recruit and direct chromatin-modifying complexes to specifically target promoter regions of tumor genes, which eventually impact key biological processes such as cell adhesion, cell cycle and apoptosis in cancers. Additionally, lncRNAs can induce aberrant expression of cancer-associated genes during post-transcriptional processing by regulating alternative pre-mRNA splicing, mRNA translation and the stability of mRNA involved in cancer progression.11,12 For instance, HOX antisense intergenic RNA (HOTAIR), a lncRNA located in the HOXC locus, has been reported to be upregulated in several cancer types.13 Upregulation of HOTAIR could be correlated with an advanced pathological stage and lymph-node metastasis, and indicated poor prognosis in non-small cell lung cancer (NSCLC). Downregulation of HOTAIR by RNAi repressed the migration and invasion of cancer cells in vitro and impeded cell metastasis in vivo.14
Recently, there have been numerous efforts applied to systematically identify cancer lncRNAs and explore their functions in tumorigenesis. Cabili et al. identified a mass of lincRNAs and found significant links with disease-associated regions derived from genome-wide association studies, supporting their potential roles in human disease.15 Thus, subsequent studies begin to take risk SNPs into consideration to identify novel cancer lncRNAs.16,17 Genome-wide transcriptional profiling of lncRNAs was also widely used to explore their functions in cancer.1,18,19 By sequencing 102 prostate tissues and cell lines using next generation sequencing technology, Prensner et al. characterized a novel cancer lincRNA named PCAT-1 which regulates cell proliferation and acts as a transcriptional repressor involved in prostate cancer progression.6 Likewise, through profiling the transcriptome of lncRNAs across multiple human cancers, Brunner et al. suggested lncRNAs can be potential biomarkers of cancers.20 Recently, Du et al. identified cancer subtype-specific driver lncRNAs by combining re-annotated lncRNA expression profiles, clinical information and somatic copy-number alterations data.21 However, discovering lncRNAs involved in cancer remains a significant challenge.
In this study, we applied the naive Bayesian classifier method to construct a model by integrating genomic, miRNA targeting and expression features. Through the model, we predicted a set of cancer-related lncRNAs and found that they were significantly enriched in differentially expressed lncRNAs and differential methylation regions (DMRs) in different cancer types, and were prognosis factors in prostate cancer. We further demonstrated the model using lncRNA knockdown data in mice, and also found an lncRNA, lnc-SNURF-1, showing a strong association with prostate cancer by regulating tumor necrosis factor-mediated signaling pathways.
Materials and methods
Positive and negative lncRNA sets
Known cancer-related lncRNAs were collected by manual curation of the published literature. The recently developed lncRNA disease database (lncRNADisease)22 referring to associations of lncRNAs with disease phenotypes was also used to obtain cancer-related lncRNAs. All of these known cancer-related lncRNAs were re-annotated according to lncRNA genomic positions derived from the LNCipedia database.23 Known cancer-related lncRNAs were mapped to LNCipedia lncRNA IDs when they had at least 50% reciprocal overlap. These known cancer-related lncRNAs with LNCipedia IDs were regarded as the positive lncRNA set. The negative lncRNA set was composed of lncRNAs without any mutations or phenotype-related SNPs within the range of 10 kb upstream and downstream of them. The mutation information (including various types of point mutations in the coding and non-coding regions detected in cancer) was downloaded from the COSMIC database.24 The phenotype-related SNPs were obtained from the GWAS Catalog.5
Construction of features
To reflect the differences between positive lncRNAs and negative lncRNAs, we constructed eight features from aspects of the genome, regulome and transcriptome.
Genomic features.
Based on exon structures of lncRNAs extracted from LNCipedia, we determined whether lncRNAs belonged to the antisense group25 (feature I). We counted the numbers of miRNAs (obtained from miRBase, version 20) present in the region of each lncRNA (feature II). Also, for each lncRNA, the total number of repeat elements (including SINE, LINE, LTR, low complexity, satellite and simple repeat derived from the UCSC genome database) located within the lncRNA was calculated (feature III). Next, we identified whether lncRNAs had an AU-rich element (ARE) according to the method described by Clark et al.26 (feature IV). Sequence conservation scores of exons of lncRNAs were calculated using UCSC 44-way vertebrate genome alignment (feature V).
Regulome features.
Mature miRNA sequences and miRNA family data were obtained from miRBase. Cancer-related miRNAs were extracted from HMDD2.27 We predicted miRNA–lncRNA interactions using the method described in miRcode.28 For each lncRNA, the number of cancer-related miRNAs targeting this lncRNA was calculated (feature VI).
Transcriptome features.
FPKM (fragments per kilobase of exon per million fragments mapped) values of 3213 lncRNAs and 18342 protein-coding genes were estimated from RNA-seq data of the Human Body Map using TopHat29 and Cufflinks30 with default parameters. Pearson correlation coefficient (r ≥ 0.8) was used to determine the co-expression relationships between lncRNAs and protein-coding genes. We calculated the number of co-expressed cancer protein-coding genes for each lncRNA based on cancer gene lists from the Genetic Association Database (GAD)31 (feature VII). In addition, using expression profiles of the Human Body Map data referring to 16 normal tissues,15 a tissue specific score S was calculated for each lncRNA as follows (feature VIII):
where n represents the number of tissues, ei represents the expression value of the lncRNA in the ith tissue and emax represents the maximal expression value of the lncRNA across the n tissues.
Construction of a naive Bayesian classifier model
All of the genomic, regulome and transcriptome features described above were used to train a naive Bayesian classifier (R package e1071, Fig. 1), which is well-suited for integration of disparate data types. The aim of the naive Bayesian classifier is to determine the possibility that a given lncRNA is related to cancer, which can be expressed as the probabilistic combination of distinct evidence.
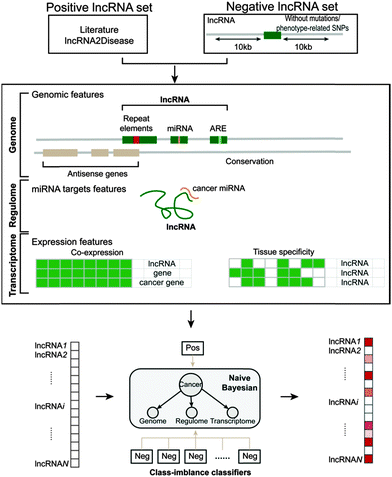 |
| Fig. 1 Schematic overview of the construction of the naive Bayesian classifier model for predicting cancer-related lncRNAs. | |
Due to the size difference between the positive and negative lncRNAs, we randomly constructed 1000 negative sets with the same number of lncRNAs as in the positive set from the negative lncRNAs. On the basis of the features, 1000 naive Bayesian classifiers were built using the positive set and the 1000 negative sets. We combined the outputs of these 1000 classifiers, and computed an average prediction score for a specific lncRNA. The prediction scores were used to identify cancer-related lncRNAs.
Re-annotation of exon array data
We designed a custom pipeline to re-annotate the Affymetrix exon array taking advantage of its huge amount of probes annotated to thousands of long non-coding RNAs.21,32 The probe sequences were downloaded from the manufacturer's website (http://www.affymetrix.com) and were then uniquely mapped to the human genome (hg19) by Bowtie without mismatch.33 Using BEDTools (http://code.google.com/p/bedtools), probes completely falling into exons of lncRNAs but that did not overlap with protein-coding genes were retained. Finally, expression levels of lncRNA genes having at least four probes were computed.
Orthologous lncRNAs
We downloaded a set of expression data referring to 147 lncRNAs knockdown experiments in mice. The “Reciprocal Best Hits BLAST” approach was used to identify orthologous human-mouse lncRNA pairs.34 A given human lncRNA was compared against the mouse lncRNA set using a BLAST e-value cutoff of 10 × 10−10. Then we sorted the BLAST hits in descending order of bit-scores, the first one would be the best hit. A similar BLAST procedure was performed for mouse lncRNAs against the human lncRNA set. Orthologs were determined if two lncRNAs in a human or mouse lncRNA set both found each other as the best hit in the other set.
Function enrichment
DAVID was used to perform gene function enrichment analysis based on GO and KEGG annotation using a set of genes associated with each knockdown of mouse lncRNA.35 Function and pathway terms with P-values < 0.05 were considered to be significantly enriched.
Results
Construction of positive and negative lncRNA sets
Through literature searches and information extraction from the lncRNADisease database, a total of 70 cancer-related lncRNAs were obtained, which served as the positive lncRNA set, and were used for the follow-up prediction of cancer-related lncRNAs (Table S1, ESI†). To select a negative set, we reasoned that lncRNAs not harboring any point mutations and phenotype-related SNPs around their loci are far less likely to be linked with human disease. Using a large number of cancer somatic point mutations derived from the COSMIC database and phenotype-related SNPs derived from the GWAS Catalog, we identified 205 lncRNAs without any mutations and phenotype-related SNPs located within 10 kb upstream and downstream of their loci as the negative lncRNA set (Table S1, ESI†). We found that most of the cis and antisense protein-coding genes of the negative lncRNAs are not known to be known cancer genes relative to the positive lncRNAs (Fig. 2A). Further, the negative lncRNAs had a significantly larger distance to the most proximate cancer-related protein-coding genes than the positive lncRNAs (P = 2.6 × 10−11 for GAD and P = 1.3 × 10−02 for OMIM, Wilcoxon rank-sum test, Fig. 2B), indicating in part that the negative lncRNAs are unlikely to be associated with cancer.
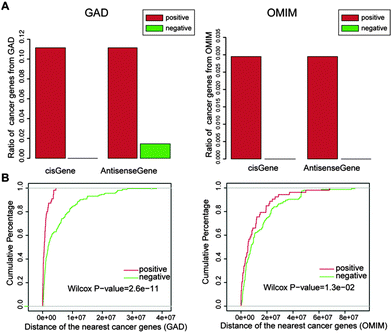 |
| Fig. 2 Proximate cancer-related genes of the positive and negative lncRNAs. Ratios of cancer-related genes in cis and antisense genes of positive (red) and negative (green) lncRNAs, calculated according to GAD and OMIM, respectively (A). Cumulative distributions of distances of the nearest cancer genes from GAD and OMIM for positive and negative lncRNAs (B). | |
Integrative identification of cancer-related lncRNAs
To distinguish positive and negative lncRNAs, a total of eight features that were composed of five genomic features, one regulome feature and two transcriptome features (see the Materials and methods section for details) were considered. Using the eight features, we trained the model including 1000 class-imbalanced classifiers with the positive and negative sets and predicted cancer-related lncRNAs from the rest of the 17125 unknown lncRNAs. The naive Bayesian classifier assumes that the input features are independent of each other, although it is clearly difficult to be satisfied, which may lead to overestimation of the possibilities for some lncRNAs. Therefore, to minimize the effect, a very high threshold of 0.9999 was used to maintain a high specificity of the prediction. Finally, we identified 707 lncRNAs, which were regarded as potential cancer-related lncRNAs (Table S2, ESI†). Among them, there were 449 intergenic lncRNAs, 81 overlapping lncRNAs and 214 antisense lncRNAs.
Evaluation by cross-validation method
We assessed whether the model was capable of predicting cancer lncRNAs using ten-fold cross-validation. As shown in Fig. 3, genomic, miRNA targeting and expression features achieved AUC scores of 0.689, 0.648 and 0.557, respectively. When combining all features, the AUC score reached 0.793, higher than any single type of feature. This suggests that the combination of these features is necessary to improve the performance of the model. In addition, we generated 100 random positive lncRNA sets with the same size as the real one, constructed corresponding models with the features, and re-evaluated them using ten-fold cross-validation. The average AUC score based on randomly selected positive sets was 0.505, suggesting that the real model was sensitive and specific in identification of cancer-related lncRNAs.
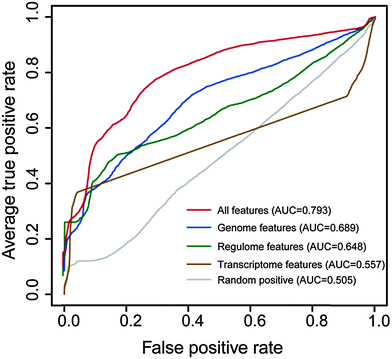 |
| Fig. 3 Results of ten-fold cross-validation. ROC curves corresponding to individual types of features and all features. The values in brackets represent the corresponding AUC scores. | |
Then, we evaluated the effects of the sample size of the positive lncRNA set on the performance of the model. From the known cancer-related lncRNAs, we randomly chose different numbers of lncRNAs as the positive sets and then constructed corresponding models. Using ten-fold cross-validation, the models were evaluated and their AUC scores were calculated. We repeated the procedure ten times and found that the average AUC scores had a slight increase with the increase of sample size (Fig. S1, ESI†), suggesting little influence of the positive sample size on the performance.
Contribution of features to identify cancer-related lncRNAs
By examining these eight features, we found that most of them were significantly distinct between the positive and negative sets. More positive lncRNAs with antisense genes were found relative to negative ones (P = 0.049, chi-square test), highlighting the importance of lncRNAs that have an antisense orientation of known protein-coding genes, which can function through base pairing interactions between the antisense and sense RNAs. RNAs with AREs are more unstable. We observed that the proportion of the positive lncRNAs with AREs was much higher than the negative ones (P = 2.42 × 10−3, chi-square test), consistent with a recent report that several novel lncRNAs involved in cell proliferation were from the group of short-lived ncRNAs.36 Positive lncRNAs showed obviously higher exon conservation than negative lncRNAs, implying that cancer-related lncRNAs may undergo evolutionary pressure for some important functions37 used for maintaining normal cell behavior (Fig. 4A, P = 0.031, Wilcoxon rank-sum test). We observed that more repeat elements were present in the positive set than the negative set, with the Wilcoxon rank-sum test P-value of 1.94 × 10−03 (Fig. 4B). Repeat elements have recently been shown to participate in lncRNA functions. The mouse Uchl1-AS lncRNA could increase Uchl1 protein synthesis through a SINE repeat element at a post-transcriptional level, and Alu repeat-containing lncRNAs are involved in destabilizing target mRNA transcripts for Staufen-mediated decay (SMD).9
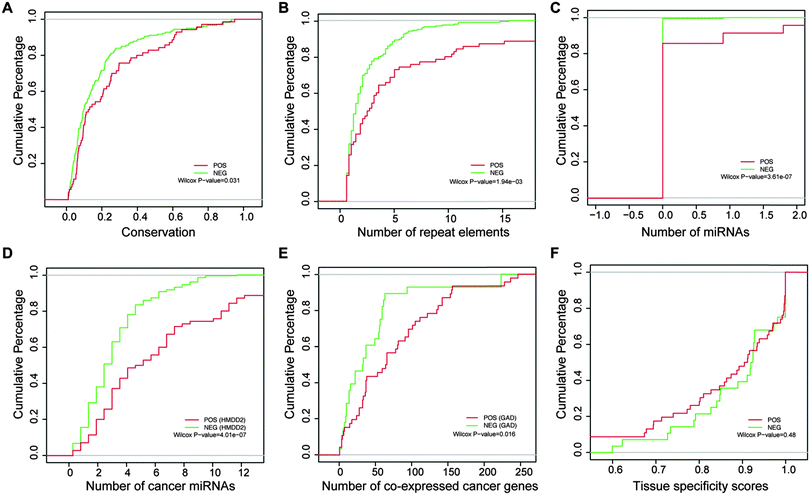 |
| Fig. 4 Comparisons of distinct features between the positive and negative lncRNAs. Cumulative distributions of conservation scores of exons (A), the number of repeat elements (B), the number of miRNAs located within the lncRNAs (C), the number of cancer miRNA targeting sites in the lncRNAs (D), the number of co-expressed cancer protein-coding genes (E), and tissue specific scores (F) for the positive lncRNAs (red) and the negative lncRNAs (green). | |
Also, we found that more miRNAs were located in the positive set compared to the negative set (Fig. 4C, P = 3.61 × 10−07, Wilcoxon rank-sum test), indicating that lncRNAs may be involved in tumorigenesis by controlling the release of some cancer-related miRNAs embedded in the lncRNAs.38,39 With the emergence of the competitive endogenous RNA (ceRNA) hypothesis, endogenous lncRNAs acting as sponges were recently linked to the progression of cancer.40 Consistently, we found that cancer-related miRNAs tended to target the positive lncRNAs relative to the negative ones (Fig. 4D, P = 4.01 × 10−07 for HMDD2, Wilcoxon rank-sum test). In addition, the cumulative distribution curves in Fig. 4E showed that the positive lncRNAs had more co-expressed cancer genes than the negative ones (P = 0.016 for GAD, Wilcoxon rank-sum test). Such a co-expression strategy had been previously used for discovering the function of lncRNAs.41,42 It is thus plausible that lncRNAs co-expressed with more cancer protein-coding genes are more likely to be cancer-related lncRNAs. In addition, the positive lncRNAs showed relatively lower tissue specificity scores than the negative ones, however without significance.
Systematic evaluation of predicted cancer-related lncRNAs
We assumed that these potential cancer-related lncRNAs are likely to be dysregulated in human cancer. To validate the assumption, we developed a custom pipeline to re-annotate eight exon array data of diverse cancer types including prostate cancer (47 patients and 48 controls, GSE29079), colorectal cancer (160 patients and 13 controls, GSE24551), lung cancer (24 patients and 14 controls, GSE40275), multiple myeloma (170 patients and 6 controls, GSE39754), gastric cancer (80 patients and 80 controls, GSE27342), head and neck squamous cell carcinoma (44 patients and 25 controls, GSE33205), glioblastoma (26 patients and 6 controls, GSE9385) and oligodendroglioma (23 patients and 6 controls, GSE9385). For each cancer type, expression profiles of 9043 lncRNAs and 18367 protein-coding genes were generated. Differential expression analyses (FDR < 0.05, t test) of these re-annotated data sets resulted in 3538 (prostate cancer), 885 (colorectal cancer), 3772 (lung cancer), 5834 (multiple myeloma), 345 (gastric cancer), 164 (head and neck squamous cell carcinoma), 304 (glioblastoma) and 398 (oligodendroglioma) differentially expressed lncRNAs (Table S3, ESI†). There were 521 lncRNAs with expression values across these cancer types among the 707 potential cancer-related lncRNAs. We found that the 521 potential cancer-related lncRNAs were significantly associated with differentially expressed lncRNAs in different cancer types (Fig. 5A, P = 0.014 for prostate cancer, P = 0.005 for colorectal cancer, P = 0.089 for lung cancer, P = 4.17 × 10−05 for Multiple Myeloma, P = 0.362 for gastric cancer, P = 7.6 × 10−05 for head and neck squamous cell carcinoma, P = 2.32 × 10−08 for glioblastoma and P = 0.001 for oligodendroglioma, chi-square test).
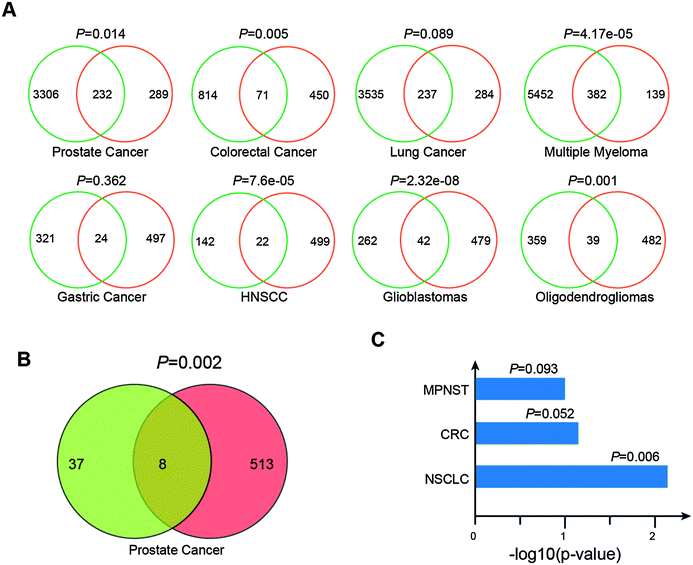 |
| Fig. 5 Overall estimation of predicted cancer-related lncRNAs. The predicted cancer-related lncRNAs show significant overlaps with differentially expressed lncRNAs from re-annotated exon array data of six types of cancers (A) and lncRNAs associated with prognosis in prostate cancer (B), and their promoters tend to have DNA methylation alterations (C). HNSCC, NSCLC, CRC and MPNST represent head and neck squamous cell carcinoma, non-small cell lung carcinomas, colorectal cancer and malignant peripheral nerve sheath tumors, respectively. | |
Moreover, we used multivariate cox proportional hazard regression analyses to evaluate associations between the expression of lncRNAs and progression-free survival (PFS) in the prostate cancer data (GSE29079). Using tumor stage, ethnicity and age as additional covariates, we found approximately 45 lncRNAs whose expressions were markedly associated with PFS (FDR < 0.05) (Table S4, ESI†). These potential cancer-related lncRNAs showed significant enrichment in these PFS relevant lncRNAs (Fig. 5B, P = 0.002, chi-square test). In addition, we collected three sets of genome-wide DMRs from non-small cell lung carcinomas,43 colorectal cancer44 and malignant peripheral nerve sheath tumors,45 and then investigated DNA methylation states of promoters (5 kb upstream and 1 kb downstream of transcription start site) of these 707 lncRNAs in these three DMR sets. We found that these cancer-related lncRNAs are more likely to have DNA methylation alterations at their promoters than other lncRNAs (Fig. 5C, P = 0.006 for non-small cell lung carcinomas, P = 0.052 for colorectal cancer and P = 0.093 for malignant peripheral nerve sheath tumors, chi-square test). Together, these findings supported the involvement of these predicted lncRNAs in cancer and also the power of the classifier model.
TP53 family members play important roles in various functions, such as apoptosis induction and cell cycle arrest, which are inactivated in approximately half of human cancers. Recent studies found several lncRNAs involved in TP53-induced apoptosis.46 Therefore, we assumed that some of these potential cancer-related lncRNAs may be the direct transcriptional targets of TP53 family members. We obtained a total of 11086 TP53 family ChIP-seq peaks whose sequences contain the canonical TP53 motif in human lung cancer cells from ref. 47 and then examined whether the peaks were located in the promoter regions of lncRNAs. Among these cancer-related lncRNAs, 25 overlapped with the peaks. In comparison to all lncRNAs, these cancer-related lncRNAs tend to be the direct targets of TP53 family members (P = 0.003, Fisher exact test). The findings highlight the potential roles of these lncRNAs in cancer.
In order to further evaluate these potential cancer-related lncRNAs, we systematically searched publicly available expression data of lncRNA perturbation from GEO. In the human hepatoma cell line SMMC-7721, a lncRNA with a size of 2446 bp was stably overexpressed and the microarray expression data were generated (GSE54798).48 The lncRNA was located within two potential cancer-related lncRNAs (lnc-POTEM-2 and lnc-POTEM-6). Through functional enrichment analysis of differentially expressed genes, we found that stable expression of the lncRNA lead to the dysfunction of the TGF-beta signaling pathway (P = 0.0003), which has been demonstrated to be associated with tumor development.49 In addition, we obtained 147 lncRNA knockdown data in mice50 and performed orthologous alignments of these 707 lncRNAs with mouse lncRNAs based on the "Reciprocal Best Hits BLAST" approach. We found six human lncRNAs with mouse orthologous lncRNAs (e-value < 10 × 10−10). For each orthologous lncRNA, its affected genes were determined through identification of differentially expressed genes (fold change ≥ 2 and t-test with FDR ≤ 0.05) based on its knockdown expression data. We then performed GO and KEGG pathway enrichment analyses using the affected genes to identify functions of lncRNAs. Of the six lncRNAs, four (including lnc-PDE7B-1, lnc-SUNRF-1, lnc-SUNRF-3 and lnc-TSHR-4) that were found to affect at least 10 protein-coding genes were significantly involved in some important cancer-related biological processes and pathways, such as cell differentiation, the Wnt signaling pathway and the TGF-beta signaling pathway (Fig. 6). Knockdown of the other two (including lnc-NME7-1 and lnc-TMEM178-1) did not affect any genes, probably due to unsuccessful shRNA knockdown. Interestingly, lnc-NME7-1 was recently verified to be associated with apoptosis involved in non-small-cell lung cancer progression.51
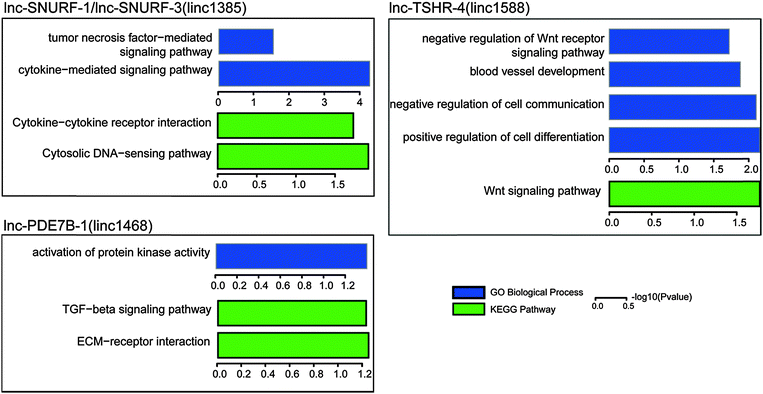 |
| Fig. 6 Gene ontology and KEGG pathway enrichment of differentially expressed genes from lncRNA knockdown data in mice. | |
lnc-SNURF-1, a candidate cancer-related lncRNA of prostate cancer
Using the re-annotated expression data, we found that these six lncRNAs showed significantly differential expression in at least two different cancer types (Fig. 7A). Interestingly, lnc-SNURF-1 showed differential expression across six cancer types. lnc-SNURF-1 is antisense to UBE3A which is an oncogenic protein-coding gene and involved in the development of prostate cancer by regulating cancer cell proliferation.52 Through analyzing RNA-seq data of 16 normal human tissues from the Human Body Map, we identified 178 protein-coding genes showing similar expression patterns with lnc-SNURF-1 (Pearson correlation coefficient ≥ 0.6). We found many known cancer-related genes co-expressed with lnc-SNURF-1, such as CDK11B, E2F7, FOXD4, POU1F1 and ANAPC10. GO enrichment analysis of these co-expressed genes showed that lnc-SNURF-1 was associated with cancer-related biological processes, such as development, cell differentiation and the cell cycle.
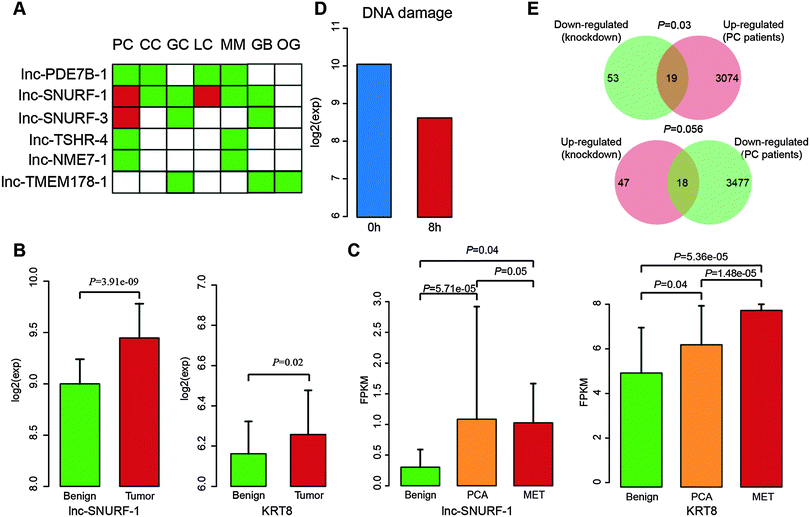 |
| Fig. 7 Up-regulation of lnc-SNURF-1 in prostate cancer. (A) Six orthologous lncRNAs show extensive different expression across multiple cancer types (red for up-regulation and green for down-regulation). Expression levels of lnc-SNURF-1 and KRT8 are significantly increased in an exon array data (B, GSE29079) and additional sequencing data of prostate cancer (C, GSE25183). (D) DNA damage induces the down-regulation of lnc-SNURF-1 (GSE43509). (E) Up-regulated and down-regulated genes influenced by knockdown of lnc-SNURF-1 exhibit converse change patterns in prostate cancer. PC, CC, GC, LC, MM, GB and OG represent prostate cancer, colon cancer, gastric cancer, lung cancer, multiple myeloma, glioblastoma and oligodendroglioma, respectively. | |
Based on the results of lnc-SNURF-1 ortholog knockdown in mice, we found that the lncRNA was associated with the tumor necrosis factor (TNF) -mediated signaling pathway and cytokine–cytokine receptor interaction by down-regulation of KRT8 and KRT18. KRT8, which is sensitive to TNF-dependent epithelial apoptosis,53 has been proved to be an important distinguishing marker in prostate cancer.54 Furthermore, we observed that both lnc-SNURF-1 and KRT8 showed significantly increased expression in the re-annotated exon array (Fig. 7B) and other RNA-seq data (Fig. 7C, including 42 disease samples and 16 controls, GSE25183) of prostate cancer.6 Using published lncRNA transcriptome data (GSE43509),55 we found that expression of lnc-SNURF-1 was reduced when treated with DNA damage (Fig. 7D). Previous studies demonstrated a significant decrease of TNF-α mRNA expression associated with DNA damage and cell loss.56 Another study also showed that TNF treatment could cause apoptosis and DNA damage in HepG2 cells.57 In addition, we found a significant overlap between up-regulated protein-coding genes in prostate cancer and down-regulated genes after lnc-SNURF-1 knockdown (Fig. 7E, P = 0.0267), and a marginal significance between down-regulated protein-coding genes in prostate cancer and up-regulated genes in the knockdown data (Fig. 7E, P = 0.0566). These findings indicate that abnormal expression of lnc-SNURF-1 induces expression change of KRT8, which in turn dysregulates the TNF-mediated signaling pathway and contributes to the development of prostate cancer.
Discussion
Extensive effort has been devoted to the systematic identification of cancer-related protein-coding genes58,59 and miRNAs,60,61 whereas only a few studies have been done to systematically identify cancer-related lncRNAs.13,62 In this study, we proposed a systematic strategy for the integrative identification of cancer-related lncRNAs by a naive Bayesian classifier model, and evaluated the performance of the model using re-annotated publicly available exon array data of multiple cancer types and knockdown data of orthologous lncRNAs in mice.
Using known cancer-related lncRNAs, a classifier model based on genomic, regulome and transcriptome data was constructed. The model showed a good performance by ten-fold cross-validation, and thus allowed us to identify 707 potential cancer-related lncRNAs. These potential cancer-related lncRNAs were found to show obvious expression differences, prognosis associations and DNA methylation alterations, indicative of their probable importance in cancer. We further validated our predictions through lncRNA knockdown data in mice. The majority of the six lncRNAs that were found to have orthologs in mouse knockdown data were demonstrated to be involved in cancer-related biological processes. One of the lncRNAs, called lnc-SNURF-1, showed differential expression in six different cancer types. Knockdown of lnc-SNURF-1 significantly influenced the TNF-mediated signaling pathway, which appears to be mediated by inducing down-regulation of a cancer-related marker KRT8. We found that lnc-SNURF-1 and KRT8 showed consistent up-regulation in both exon array data and additional RNA-seq data of prostate cancer. Significant overlaps between different expressed genes in prostate cancer and lnc-SNURF-1 knockdown data were observed. These results strongly suggest that lnc-SNURF-1 may play critical roles in prostate cancer by influencing the expression of downstream KRT8, and also support that this integrative strategy can be useful for identifying cancer-related lncRNAs.
Notably, two methods including LRLSLDA and RWRlncD were recently proposed to predict disease-related lncRNAs.19,63 Both of the methods were based on an assumption that similar diseases tend to be associated with functionally similar lncRNAs, and thus focus on known disease-related lncRNAs. They predicted novel associations of known disease-related lncRNAs with other diseases based on the assumption. LRLSLDA calculates Gaussian interaction profile kernel similarity for diseases and lncRNAs based on known disease–lncRNA associations and uses the framework of Laplacian Regularized Least Squares to identify potential disease–lncRNA associations. RWRlncD uses the random walk with restart method on a lncRNA functional similarity network that is constructed based on known disease–lncRNA associations to infer potential disease–lncRNA associations. We obtained 112 predicted cancer-related lncRNAs derived from the top 500 disease-lncRNA associations from LRLSLDA and 117 potential prostate cancer-related lncRNAs from RWRlncD for comparison with our method. We found that our results significantly overlapped with the other two results (P = 0.029 for LRLSLDA and P = 0.019 for RWRlncD, chi-square test). In addition, we also found many potential cancer-related lncRNAs not identified by the two methods. Interestingly, these lncRNAs tend to show significantly differential expression in different cancer types (P = 0.013 for prostate cancer, P = 0.014 for colorectal cancer, P = 0.134 for lung cancer, P = 1.55 × 10−05 for Multiple Myeloma, P = 0.366 for gastric cancer, P = 1.5 × 10−04 for head and neck squamous cell carcinoma, P = 5.33 × 10−09 for glioblastoma and P = 0.002 for oligodendroglioma, chi-square test). The results indicated that our method based on integration of multi-omic data can be applied to whole genome-wide screening of cancer-related lncRNAs.
Recently, much of the available information focusing on different aspects of lncRNAs has been generated and used for identification of lncRNAs and their functional exploration, including various epigenetic states,64 DNA binding sites identified by chromatin isolation by RNA purification,65 RNA interactions identified by RIA-seq,11 genome-wide map of lncRNA–miRNA interactions66 and binding proteins identified by ribonucleo-protein immunoprecipitation followed by sequencing,67 transcriptional regulation by transcription factors68 and RNA stability.26 Such diverse information will enhance the performance of the classifier model. Considering a slightly increased performance with the increase of the positive sample size (Fig. S1, ESI†), we believe that, with increasing discovery of cancer-related lncRNAs, the reliability and robustness of the model will be further improved. Moreover, widespread genomic studies have identified a large number of somatic copy number alterations (SCNAs) in a variety of cancer types, which are important for cancer initiation and cancer progression.69 Addition of SCNAs may help to reveal novel lncRNA drivers contributing to tumorigenesis.21
Conclusions
In summary, we offered a systematic, integrated and useful strategy in research for cancer-related lncRNAs, which can further facilitate identification of novel cancer-related lncRNAs and help researchers to understand the roles of lncRNAs in cancer. Also we generated a valuable set of candidate cancer-related lncRNAs, which can be used for further wet laboratory validation.
Contributions
Liming Zhang, Xia Li and Yun Xiao participated in the design and coordination of the study. Tingting Zhao, Jinyuan Xu, Ling Liu and Jing Bai carried out the method and performed the analysis. Chaohan Xu helped to analyze the results. All authors read and approved the final manuscript.
Conflicts of interest
There is no competing financial interest in relation to the work.
Acknowledgements
This work was supported in part by the National High Technology Research and Development Program of China [863 Program, Grant Nos. SS2014AA021102], the National Program on Key Basic Research Project [973 Program, Grant No. 2014CB910504], the National Natural Science Foundation of China [Grant No. 91129710, 61073136, 31200997, 61170154, 81070946 and 81171122], the National Science Foundation of Heilongjiang Province (Grant No. C201207, H0906 and ZD201208), and Key Laboratory of Cardiovascular Medicine Research (Harbin Medical University), Ministry of Education. Wu lien-teh youth science fund project of Harbin medical university [Grant No. WLD-QN1407].
Notes and references
- S. R. Atkinson, S. Marguerat and J. Bahler, Semin. Cell Dev. Biol., 2012, 23, 200–205 CrossRef CAS PubMed.
- C. P. Ponting, P. L. Oliver and W. Reik, Cell, 2009, 136, 629–641 CrossRef CAS PubMed.
- M. Guttman and J. L. Rinn, Nature, 2012, 482, 339–346 CrossRef CAS PubMed.
- J. F. Kugel and J. A. Goodrich, Trends Biochem. Sci., 2012, 37, 144–151 CrossRef CAS PubMed.
- L. A. Hindorff, P. Sethupathy, H. A. Junkins, E. M. Ramos, J. P. Mehta, F. S. Collins and T. A. Manolio, Proc. Natl. Acad. Sci. U. S. A., 2009, 106, 9362–9367 CrossRef CAS PubMed.
- J. R. Prensner, M. K. Iyer, O. A. Balbin, S. M. Dhanasekaran, Q. Cao, J. C. Brenner, B. Laxman, I. A. Asangani, C. S. Grasso, H. D. Kominsky, X. Cao, X. Jing, X. Wang, J. Siddiqui, J. T. Wei, D. Robinson, H. K. Iyer, N. Palanisamy, C. A. Maher and A. M. Chinnaiyan, Nat. Biotechnol., 2011, 29, 742–749 CrossRef CAS PubMed.
- P. M. Guenzl and D. P. Barlow, RNA Biol., 2012, 9, 731–741 CrossRef CAS PubMed.
- Y. Huang, S. Nayak, R. Jankowitz, N. E. Davidson and S. Oesterreich, Breast Cancer Res., 2011, 13, 225 CrossRef CAS PubMed.
- S. Geisler and J. Coller, Nat. Rev. Mol. Cell Biol., 2013, 14, 699–712 CrossRef CAS PubMed.
- S. Guil and M. Esteller, Nat. Struct. Mol. Biol., 2012, 19, 1068–1075 CAS.
- M. Kretz, Z. Siprashvili, C. Chu, D. E. Webster, A. Zehnder, K. Qu, C. S. Lee, R. J. Flockhart, A. F. Groff, J. Chow, D. Johnston, G. E. Kim, R. C. Spitale, R. A. Flynn, G. X. Zheng, S. Aiyer, A. Raj, J. L. Rinn, H. Y. Chang and P. A. Khavari, Nature, 2013, 493, 231–235 CrossRef CAS PubMed.
- X. Shi, M. Sun, H. Liu, Y. Yao and Y. Song, Cancer Lett., 2013, 339, 159–166 CrossRef CAS PubMed.
- P. J. Batista and H. Y. Chang, Cell, 2013, 152, 1298–1307 CrossRef CAS PubMed.
- X. H. Liu, Z. L. Liu, M. Sun, J. Liu, Z. X. Wang and W. De, BMC Cancer, 2013, 13, 464 CrossRef PubMed.
- M. N. Cabili, C. Trapnell, L. Goff, M. Koziol, B. Tazon-Vega, A. Regev and J. L. Rinn, Genes Dev., 2011, 25, 1915–1927 CrossRef CAS PubMed.
- G. Jin, J. Sun, S. D. Isaacs, K. E. Wiley, S. T. Kim, L. W. Chu, Z. Zhang, H. Zhao, S. L. Zheng, W. B. Isaacs and J. Xu, Carcinogenesis, 2011, 32, 1655–1659 CrossRef CAS PubMed.
- Z. Jiang, Y. Zhou, K. Devarajan, C. M. Slater, M. B. Daly and X. Chen, Front. Genet., 2012, 3, 299 CAS.
- X. Zhang, S. Sun, J. K. Pu, A. C. Tsang, D. Lee, V. O. Man, W. M. Lui, S. T. Wong and G. K. Leung, Neurobiol. Dis., 2012, 48, 1–8 CrossRef PubMed.
- X. Chen and G. Y. Yan, Bioinformatics, 2013, 29, 2617–2624 CrossRef CAS PubMed.
- A. L. Brunner, A. H. Beck, B. Edris, R. T. Sweeney, S. X. Zhu, R. Li, K. Montgomery, S. Varma, T. Gilks, X. Guo, J. W. Foley, D. M. Witten, C. P. Giacomini, R. A. Flynn, J. R. Pollack, R. Tibshirani, H. Y. Chang, M. van de Rijn and R. B. West, Genome Biol., 2012, 13, R75 CrossRef PubMed.
- Z. Du, T. Fei, R. G. Verhaak, Z. Su, Y. Zhang, M. Brown, Y. Chen and X. S. Liu, Nat. Struct. Mol. Biol., 2013, 20, 908–913 CAS.
- X. Su, J. Xing, Z. Wang, L. Chen, M. Cui and B. Jiang, Chin. J. Cancer Res., 2013, 25, 235–239 Search PubMed.
- P. J. Volders, K. Helsens, X. Wang, B. Menten, L. Martens, K. Gevaert, J. Vandesompele and P. Mestdagh, Nucleic Acids Res., 2013, 41, D246–D251 CrossRef CAS PubMed.
- S. A. Forbes, N. Bindal, S. Bamford, C. Cole, C. Y. Kok, D. Beare, M. Jia, R. Shepherd, K. Leung, A. Menzies, J. W. Teague, P. J. Campbell, M. R. Stratton and P. A. Futreal, Nucleic Acids Res., 2011, 39, D945–D950 CrossRef CAS PubMed.
- T. Derrien, R. Johnson, G. Bussotti, A. Tanzer, S. Djebali, H. Tilgner, G. Guernec, D. Martin, A. Merkel, D. G. Knowles, J. Lagarde, L. Veeravalli, X. Ruan, Y. Ruan, T. Lassmann, P. Carninci, J. B. Brown, L. Lipovich, J. M. Gonzalez, M. Thomas, C. A. Davis, R. Shiekhattar, T. R. Gingeras, T. J. Hubbard, C. Notredame, J. Harrow and R. Guigo, Genome Res., 2012, 22, 1775–1789 CrossRef CAS PubMed.
- M. B. Clark, R. L. Johnston, M. Inostroza-Ponta, A. H. Fox, E. Fortini, P. Moscato, M. E. Dinger and J. S. Mattick, Genome Res., 2012, 22, 885–898 CrossRef CAS PubMed.
- Y. Li, C. Qiu, J. Tu, B. Geng, J. Yang, T. Jiang and Q. Cui, Nucleic Acids Res., 2013, 42, D1070–D1074 CrossRef PubMed.
- A. Jeggari, D. S. Marks and E. Larsson, Bioinformatics, 2012, 28, 2062–2063 CrossRef CAS PubMed.
- C. Trapnell, L. Pachter and S. L. Salzberg, Bioinformatics, 2009, 25, 1105–1111 CrossRef CAS PubMed.
- C. Trapnell, B. A. Williams, G. Pertea, A. Mortazavi, G. Kwan, M. J. van Baren, S. L. Salzberg, B. J. Wold and L. Pachter, Nat. Biotechnol., 2010, 28, 511–515 CrossRef CAS PubMed.
- K. G. Becker, K. C. Barnes, T. J. Bright and S. A. Wang, Nat. Genet., 2004, 36, 431–432 CrossRef CAS PubMed.
- P. Gellert, Y. Ponomareva, T. Braun and S. Uchida, Nucleic Acids Res., 2013, 41, e20 CrossRef CAS PubMed.
- B. Langmead, C. Trapnell, M. Pop and S. L. Salzberg, Genome Biol., 2009, 10, R25 CrossRef PubMed.
- G. Moreno-Hagelsieb and K. Latimer, Bioinformatics, 2008, 24, 319–324 CrossRef CAS PubMed.
- W. Huang da, B. T. Sherman and R. A. Lempicki, Nat. Protoc., 2009, 4, 44–57 CrossRef PubMed.
- H. Tani, R. Mizutani, K. A. Salam, K. Tano, K. Ijiri, A. Wakamatsu, T. Isogai, Y. Suzuki and N. Akimitsu, Genome Res., 2012, 22, 947–956 CrossRef CAS PubMed.
- A. N. Khachane and P. M. Harrison, PLoS One, 2010, 5, e10316 Search PubMed.
- A. Keniry, D. Oxley, P. Monnier, M. Kyba, L. Dandolo, G. Smits and W. Reik, Nat. Cell Biol., 2012, 14, 659–665 CrossRef CAS PubMed.
- K. Augoff, B. McCue, E. F. Plow and K. Sossey-Alaoui, Mol. Cancer, 2012, 11, 5 CrossRef CAS PubMed.
- L. Salmena, L. Poliseno, Y. Tay, L. Kats and P. P. Pandolfi, Cell, 2011, 146, 353–358 CrossRef CAS PubMed.
- Q. Liao, C. Liu, X. Yuan, S. Kang, R. Miao, H. Xiao, G. Zhao, H. Luo, D. Bu, H. Zhao, G. Skogerbo, Z. Wu and Y. Zhao, Nucleic Acids Res., 2011, 39, 3864–3878 CrossRef CAS PubMed.
- X. Guo, L. Gao, Q. Liao, H. Xiao, X. Ma, X. Yang, H. Luo, G. Zhao, D. Bu, F. Jiao, Q. Shao, R. Chen and Y. Zhao, Nucleic Acids Res., 2013, 41, e35 CrossRef CAS PubMed.
- R. H. Carvalho, V. Haberle, J. Hou, T. van Gent, S. Thongjuea, W. van Ijcken, C. Kockx, R. Brouwer, E. Rijkers, A. Sieuwerts, J. Foekens, M. van Vroonhoven, J. Aerts, F. Grosveld, B. Lenhard and S. Philipsen, Epigenet. Chromatin, 2012, 5, 9 CrossRef PubMed.
- F. Simmer, A. B. Brinkman, Y. Assenov, F. Matarese, A. Kaan, L. Sabatino, A. Villanueva, D. Huertas, M. Esteller, T. Lengauer, C. Bock, V. Colantuoni, L. Altucci and H. G. Stunnenberg, Epigenetics, 2012, 7, 1355–1367 CrossRef CAS PubMed.
- A. Feber, G. A. Wilson, L. Zhang, N. Presneau, B. Idowu, T. A. Down, V. K. Rakyan, L. A. Noon, A. C. Lloyd, E. Stupka, V. Schiza, A. E. Teschendorff, G. P. Schroth, A. Flanagan and S. Beck, Genome Res., 2011, 21, 515–524 CrossRef CAS PubMed.
- M. Huarte, M. Guttman, D. Feldser, M. Garber, M. J. Koziol, D. Kenzelmann-Broz, A. M. Khalil, O. Zuk, I. Amit, M. Rabani, L. D. Attardi, A. Regev, E. S. Lander, T. Jacks and J. L. Rinn, Cell, 2010, 142, 409–419 CrossRef CAS PubMed.
- M. Idogawa, T. Ohashi, Y. Sasaki, R. Maruyama, L. Kashima, H. Suzuki and T. Tokino, Hum. Mol. Genet., 2014, 23, 2847–2857 CrossRef CAS PubMed.
- J. H. Yuan, F. Yang, F. Wang, J. Z. Ma, Y. J. Guo, Q. F. Tao, F. Liu, W. Pan, T. T. Wang, C. C. Zhou, S. B. Wang, Y. Z. Wang, Y. Yang, N. Yang, W. P. Zhou, G. S. Yang and S. H. Sun, Cancer Cell, 2014, 25, 666–681 CrossRef CAS PubMed.
- J. Massague, Cell, 2008, 134, 215–230 CrossRef CAS PubMed.
- M. Guttman, J. Donaghey, B. W. Carey, M. Garber, J. K. Grenier, G. Munson, G. Young, A. B. Lucas, R. Ach, L. Bruhn, X. Yang, I. Amit, A. Meissner, A. Regev, J. L. Rinn, D. E. Root and E. S. Lander, Nature, 2011, 477, 295–300 CrossRef CAS PubMed.
- Y. Yang, H. Li, S. Hou, B. Hu, J. Liu and J. Wang, PLoS One, 2013, 8, e65309 CAS.
- S. Srinivasan and Z. Nawaz, Biochim. Biophys. Acta, 2011, 1809, 119–127 CrossRef CAS PubMed.
- D. Jaquemar, S. Kupriyanov, M. Wankell, J. Avis, K. Benirschke, H. Baribault and R. G. Oshima, J. Cell Biol., 2003, 161, 749–756 CrossRef CAS PubMed.
- M. H. Kuchma, J. H. Kim, M. T. Muller and P. A. Arlen, Protein J., 2012, 31, 195–205 CrossRef CAS PubMed.
- G. Wan, R. Mathur, X. Hu, Y. Liu, X. Zhang, G. Peng and X. Lu, Cell. Signalling, 2013, 25, 1086–1095 CrossRef CAS PubMed.
- M. J. Tuorkey, K. K. Abdul-Aziz and A. A. Zidan, Endocr., Metab. Immune Disord.: Drug Targets, 2013, 13, 269–274 CrossRef CAS.
- K. M. Beggs, A. M. Fullerton, K. Miyakawa, P. E. Ganey and R. A. Roth, Toxicol. Sci., 2013, 88, 1083–1095 Search PubMed.
- Y. Moreau and L. C. Tranchevent, Nat. Rev. Genet., 2012, 13, 523–536 CrossRef CAS PubMed.
- Y. Xiao, C. Xu, Y. Ping, J. Guan, H. Fan, Y. Li and X. Li, Genomics, 2011, 98, 64–71 CAS.
- Y. Xiao, J. Guan, Y. Ping, C. Xu, T. Huang, H. Zhao, H. Fan, Y. Li, Y. Lv, T. Zhao, Y. Dong, H. Ren and X. Li, Nucleic Acids Res., 2012, 40, 7653–7665 CrossRef CAS PubMed.
- Y. Xiao, Y. Ping, H. Fan, C. Xu, J. Guan, H. Zhao, Y. Li, Y. Lv, Y. Jin, L. Wang and X. Li, Neuro-oncology, 2013, 15, 818–828 CrossRef CAS PubMed.
- E. A. Gibb, E. A. Vucic, K. S. Enfield, G. L. Stewart, K. M. Lonergan, J. Y. Kennett, D. D. Becker-Santos, C. E. MacAulay, S. Lam, C. J. Brown and W. L. Lam, PLoS One, 2011, 6, e25915 CAS.
- J. Sun, H. Shi, Z. Wang, C. Zhang, L. Liu, L. Wang, W. He, D. Hao, S. Liu and M. Zhou, Mol. BioSyst., 2014, 10, 2074–2081 RSC.
- S. Sati, S. Ghosh, V. Jain, V. Scaria and S. Sengupta, Nucleic Acids Res., 2012, 40, 10018–10031 CrossRef CAS PubMed.
- C. Chu, K. Qu, F. L. Zhong, S. E. Artandi and H. Y. Chang, Mol. Cell, 2011, 44, 667–678 CrossRef CAS PubMed.
- S. Jalali, D. Bhartiya, M. K. Lalwani, S. Sivasubbu and V. Scaria, PLoS One, 2013, 8, e53823 CAS.
- J. Zhao, T. K. Ohsumi, J. T. Kung, Y. Ogawa, D. J. Grau, K. Sarma, J. J. Song, R. E. Kingston, M. Borowsky and J. T. Lee, Mol. Cell, 2010, 40, 939–953 CrossRef CAS PubMed.
- J. H. Yang, J. H. Li, S. Jiang, H. Zhou and L. H. Qu, Nucleic Acids Res., 2013, 41, D177–D187 CrossRef CAS PubMed.
- R. Beroukhim, C. H. Mermel, D. Porter, G. Wei, S. Raychaudhuri, J. Donovan, J. Barretina, J. S. Boehm, J. Dobson, M. Urashima, K. T. Mc Henry, R. M. Pinchback, A. H. Ligon, Y. J. Cho, L. Haery, H. Greulich, M. Reich, W. Winckler, M. S. Lawrence, B. A. Weir, K. E. Tanaka, D. Y. Chiang, A. J. Bass, A. Loo, C. Hoffman, J. Prensner, T. Liefeld, Q. Gao, D. Yecies, S. Signoretti, E. Maher, F. J. Kaye, H. Sasaki, J. E. Tepper, J. A. Fletcher, J. Tabernero, J. Baselga, M. S. Tsao, F. Demichelis, M. A. Rubin, P. A. Janne, M. J. Daly, C. Nucera, R. L. Levine, B. L. Ebert, S. Gabriel, A. K. Rustgi, C. R. Antonescu, M. Ladanyi, A. Letai, L. A. Garraway, M. Loda, D. G. Beer, L. D. True, A. Okamoto, S. L. Pomeroy, S. Singer, T. R. Golub, E. S. Lander, G. Getz, W. R. Sellers and M. Meyerson, Nature, 2010, 463, 899–905 CrossRef CAS PubMed.
Footnote |
† Electronic supplementary information (ESI) available. See DOI: 10.1039/c4mb00478g |
|
This journal is © The Royal Society of Chemistry 2015 |