DOI:
10.1039/C4MB00404C
(Review Article)
Mol. BioSyst., 2015,
11, 980-993
Systems biology approach to understanding post-traumatic stress disorder
Received
10th July 2014
, Accepted 13th January 2015
First published on 14th January 2015
Abstract
Post-traumatic stress disorder (PTSD) is a psychological disorder affecting individuals that have experienced life-changing traumatic events. The symptoms of PTSD experienced by these subjects—including acute anxiety, flashbacks, and hyper-arousal—disrupt their normal functioning. Although PTSD is still categorized as a psychological disorder, recent years have witnessed a multi-directional research effort attempting to understand the biomolecular origins of the disorder. This review begins by providing a brief overview of the known biological underpinnings of the disorder resulting from studies using structural and functional neuroimaging, endocrinology, and genetic and epigenetic assays. Next, we discuss the systems biology approach, which is often used to gain mechanistic insights from the wealth of available high-throughput experimental data. Finally, we provide an overview of the current computational tools used to decipher the heterogeneous types of molecular data collected in the study of PTSD.
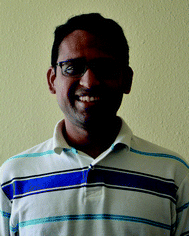
Gunjan S. Thakur
| Gunjan Singh Thakur is a postdoctoral scholar in the Institute for Collaborative Biotechnologies at University of California Santa Barbara. He received his Bachelor of Technology from Indian Institute of Technology, Guwahati in Mechanical Engineering in 2000, Masters of Science in Engineering Science and Mechanics from Virginia Polytechnic Institute and State University, in 2004 and PhD in Mechanical Engineering form UCSB in 2011. His research interests include dynamical systems, system biology, controls, machine learning and mathematical modeling of biological systems. |
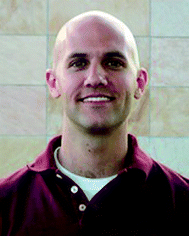
Bernie J. Daigle Jr
| Bernie Daigle, Jr is Assistant Researcher in the Institute for Collaborative Biotechnologies at the University of California, Santa Barbara. Prior to his current appointment, he was a postdoctoral researcher in the Department of Computer Science at UCSB. He received his BS in Biology, summa cum laude, from Cornell University in 2002. In 2010, he received his PhD in Genetics from Stanford University School of Medicine, where he was the recipient of Geraldine Jackson Fuhrman and Howard Hughes Medical Institute Predoctoral Fellowships. His research interests include computational modeling and analysis of stochastic biochemical systems, and integration of genome-scale datasets for disease biomarker identification. |
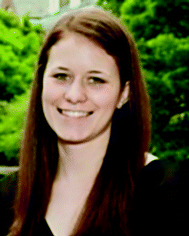
Kelsey R. Dean
| Kelsey R. Dean is a third year chemical engineering graduate student at the University of California Santa Barbara, where she was awarded the Heslin Fellowship in 2012. She received her BS in Chemical and Biomolecular Engineering from Johns Hopkins University in 2012. Her research interests include unsupervised learning techniques for disease subtype identification, and clinical and biological data integration for disease biomarker identification. |
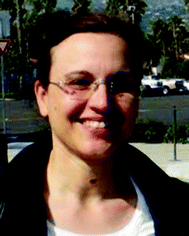
Rasha Hammamieh
| Dr Hammamieh is the Director of Integrative Systems Biology at US Army Center for Environmental Health Research. Her work focuses on the development of diagnostic biomarkers and therapeutic strategies for diseases of military relevance, including PTSD, exposure to pathogens, battlefield-like stress, coagulopathy and pain. Dr Hammamieh has authored and co-authored over 40 publications on responses to stressors including pathogens, trauma, PTSD, battlefield-like stress and nutrigenomics. |
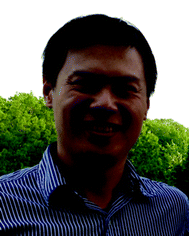
Ruoting Yang
| Ruoting Yang is Bioinformatics Supervisor in the Frederick National Laboratory for Cancer Research. Prior to his current appointment, he was a postdoctoral researcher in the Institute for Collaborative Biotechnologies, University of California Santa Barbara. He received his BS in Automation, from Xiamen University in 2000, and MS in Control Theory in Academy of Mathematics and Systems Science, Chinese Academy of Sciences. In 2008, he received his PhD in Systems Science and Mathematics and MS in Electrical Engineering from Washington University in Saint Louis. His research interests include systems biology, computational biology, mathematical modeling, and biomarker identification. |
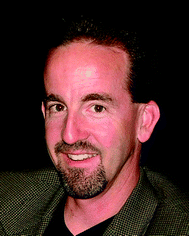
Francis J. Doyle III
| Dr Doyle is the Chair of the Department of Chemical Engineering at UCSB, and is Director of the Army Institute for Collaborative Biotechnologies. He holds the Duncan and Suzanne Mellichamp Chair in Process Control in the Department of Chemical Engineering, as well as appointments in the Electrical Engineering Department, and the Biomolecular Science and Engineering Program. He received his BSE from Princeton (1985), C.P.G.S. from Cambridge (1986), and PhD from Caltech (1991), all in Chemical Engineering. He is the recipient of numerous research awards (including the NSF National Young Investigator, ONR Young Investigator, Humboldt Research Fellowship) as well as teaching awards (including the Purdue Potter Award, the ASEE Ray Fahien Award, and the ASEE Chemstations Lectureship Award). He is a Fellow of multiple professional societies including IEEE, IFAC, AIMBE, and AAAS. His research interests are in systems biology, network science, modeling and analysis of circadian rhythms, drug delivery for diabetes, and model-based control. |
1 Introduction
Post-traumatic stress disorder (PTSD) is the fifth most common psychiatric disorder with an occurrence rate of about 8% in the United States.1 Unlike other psychiatric disorders, PTSD may develop in individuals only after they have experienced a terrifying traumatic event such as torture, rape, kidnapping, or natural disasters such as floods or earthquakes. The incidence of occurrence of PTSD in war veterans is relatively high. Approximately 22% of veterans returning from Iraq and Afghanistan are diagnosed with the disorder.2 Before 1980, when it was officially recognized as a medical disorder, PTSD was referred to by different names. During the American Civil War it was referred to as “Soldier's Heart”, during World War I as “Combat Fatigue”, during World War II as “Gross Stress Reaction”, and during the Vietnam War as “Post-Vietnam Syndrome”. Other names for PTSD include “Battle Fatigue” and “Shell Shock”.
Currently, survey-based assays are used to diagnose PTSD, including the clinician-administered PTSD scale (CAPS)3 from the “Diagnostic and Statistical Manual of Mental Disorder” (considered to be the gold standard) and self assessment scales such as the “Posttraumatic Diagnostic Scale”.4 These tests help diagnose whether or not a subject has PTSD with fairly good accuracy. However, these tests are not designed to diagnose stages of the disorder, from an early manifestation to later advanced stages. Thus there is a need to identify reliable biomarkers for PTSD that can be used for the accurate diagnosis, prognosis, and evaluation of therapeutics for the disorder. Although many candidate biomarkers have been identified for PTSD, reliable markers for specific clinical applications are still lacking.
In a number of disease models, system biology approaches have shed light on the complex underlying biophysical networks.5–8 Systems biology aims to understand the complexity of a biological system by integrating experimental data at different scales with mathematical models (e.g., differential equations, stochastic models and data-driven approaches such as machine learning) to obtain useful information about the system at the scale of interest.9 These scales range from the single-cell and single-molecule level up to the genome-wide, organismal level (see Fig. 1). Depending on the scale of investigation, informative experimental data may consist of “omics” measurements (e.g., transcriptomics and proteomics), quantification of individual metabolites, or brain images. The systems approach enables us to make testable predictions (e.g., identification of diagnostic/prognostic biomarkers or drug targets) and gain mechanistic insights into the system in question.
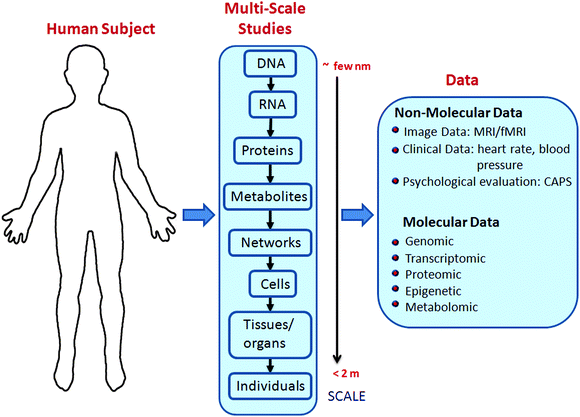 |
| Fig. 1 Systems biology approach for the study of PTSD: a multitude of data types can be experimentally collected by probing biological systems at different scales. This wealth of data is then further processed by employing mathematical and computational techniques (e.g., statistics, machine learning, computational modeling) to gain insight into the system under study. | |
In the remainder of this review, we first provide a brief overview of the known biological underpinnings of PTSD, noting that many excellent reviews are available reflecting the vast, but often conflicting, amount of information available on the subject.10–14 Next, we focus on the range of systems biology approaches that have been used to understand the disorder. Finally, we present model studies which have employed these tools to obtain new clinical and biological insights.
2 Biology of PTSD
A large body of literature has documented symptoms of PTSD in human subjects. These symptoms include memories/avoidance of traumatic events, emotional numbing, hyper-arousal (e.g. exaggerated startle and lack of sleep), alteration in fear conditioning, and impaired extinction learning.15–17 Recently, many neurobiological studies have been performed, effectively broadening the view that PTSD is purely a psychological disorder and helping to obtain a deeper insight into the disease mechanism. Psychophysiological observables such as heart rate,18 skin conductance (measurement of sweat activity), eye blinking (measurement of startle state), facial electromyogram (EMG, used to assess the neuronal signaling to muscles) and cortical electroencephalographic event related potentials (ERPs, measurement of brain activity)19,20 have been used to quantify PTSD symptoms in a systematic manner. Most psychophysiological studies conduct cross-sectional comparisons between the treatment (individuals with PTSD) and control (individuals without PTSD) groups. These studies generally find autonomic reactivity, such as heart rate and skin conductance, and startle response to trauma-related stimuli to be elevated in the treatment group. Specifically, a strong correlation has been observed between the level of reactivity to trauma-related stimuli and the severity of PTSD.21,22 Increases in heart rate, recurrent recall of trauma memories, and exaggerated startling are typically all acquired in individuals with the disorder, but these behaviors may not be unique to PTSD.
Neuroimaging
To gain a better understanding of the disorder, many studies have focused on macroscopic features of the human brain by employing techniques such as structural (sMRI) and functional magnetic resonance imaging (fMRI). The brain regions related to symptoms of the disorder include the hippocampus, amygdala, ventromedial prefrontal cortex (vmPFC), dorsal anterior cingulate cortex (dACC) and insular cortex (see Table 1). sMRI studies have shown a decrease in the volume of hippocampus,23,24 vmPFC,25 dACC25,26 and the insular cortex27,28 for patients with PTSD. In the case of changes to the hippocampal volume, it is not clear whether this decrease is caused by the extreme trauma or simply represents a risk factor; however, some studies point to the latter.29 We note that exceptions to the above have been reported in children with PTSD,30 possibly due to lack of neuronal maturation, and in cases where symptom severity was not very strong.31 Studies performed with combat twins using voxel-based morphometry suggest that the reduction in ACC volume may be an acquired feature due to extreme trauma.25
Table 1 Summary of neuroimaging findings in subjects with PTSD. Abbreviations used: vmPFC denotes ventromedial prefrontal cortex and dACC denotes dorsal anterior cingulate cortex
Brain regions |
Function imaging |
Structural imaging |
Normal function |
Activity in PTSD subjects |
Correlation with PTSD severity |
Volume change |
Amygdala |
Detection of threat, processing/learning of fear stimulation47,48 |
Increased32,33 |
Positively44–46 |
Not clear |
vmPFC |
Goal-directed decisions making49 |
Decreased36,44,50 |
Negatively44,51 |
Decrease25 |
dACC |
Regulates cognitive control, error detection, fear appraisal/expression54,55 |
Increased51–53 |
Positively34,38 |
Decrease25,26 |
Hippocampus |
Short and long term memory56,57 |
Mixed58,59 |
Negatively29 |
Decrease23,24 |
Insular cortex |
Monitoring internal body states60 |
Increased35,61 |
Positively35 |
Decrease35 |
Functional MRI studies have shown increased activity in the amygdala,32,33 dACC34 and insular cortex,35 and decreased activity in the vmPFC36 for PTSD patients subjected to trauma-related stimuli. Positron emission tomography, an fMRI technique quantifying local changes in cerebral blood flow, has been performed on identical twins to study resting dACC glucose metabolism and dACC activation during non-emotional inference tasks. These studies demonstrated that combat-exposed veterans with PTSD and their co-twins have greater metabolism37 and greater activation38 compared to veterans without PTSD and their co-twins. This suggests that increased activation of dACC is a PTSD risk factor rather than a symptom. Finally, activation in the amygdala39 and insular cortex40 does not appear to be unique to PTSD, as these responses are also seen in other anxiety disorders. Functional MRI techniques are also used to measure resting state brain activity and functional connectivity (i.e., temporal correlations between brain regions with common functional properties). A recent paper by Yan et al.41 found increased activity in the amygdala and the anterior insula and decreased activity in the thalamus to be common features in both resting state brain activity and task-based fMRI studies (as reported by other authors, see citations included in the paper), in male combat veterans with PTSD. Their results also highlight decreased activity in the precuneus region of the brain, which is responsible for integrating information from the past and future. Furthermore, Rabinak et al.42 found enhanced connectivity between the amygdala and insula in combat-related PTSD patients. However, as many studies of anxiety-related disorders have also reported fMRI-based amygdala and insula activation,43 these responses may not be unique to PTSD.
Neuroendocrinological studies
The major constituents of the neuroendocrine response to physical and emotional threat and stress are the sympathetic nervous system (SNS) and the hypothalamic-pituitary adrenal (HPA) axis.62 The immediate SNS response mobilizes the acute fight-or-flight response and is followed by a response from the HPA axis that reinstates homeostasis. PTSD patients showed low cortisol levels, which is surprising for a disorder precipitated by extreme stress, and differs from that observed in studies of acute and chronic stress and major depressive disorder (which have been associated with increased cortisol levels).63 Moreover, PTSD patients exhibited increased levels of norepinephrine, corticotropin-releasing hormone (CRH) and proinflammatory cytokines, reflecting reduced glucocorticoid signaling. This profile of alterations has been associated with PTSD pathophysiology. However, emerging research indicates that these alterations may instead reflect pre-traumatic vulnerabilities to the later development of PTSD.62
Gene expression
Previous gene expression studies of PTSD have focused on two types of biomarkers: predictive and diagnostic signatures. Predictive biomarkers indicate risk of PTSD development after trauma exposure and require longitudinal studies. Multiple longitudinal studies have presented panels of potential risk biomarkers.64,65 These biomarkers include up-regulated genes involved in type-1 interferon signaling (IFI27, OAS1, OAS2, OAS3, XAF1, and USP18)64 and differentially expressed genes which associate with glucocorticoid receptors (GR), including low FKBP5 and high GILZ expression.65 Additionally, many studies suggest potential mRNA diagnostic biomarkers, including the GR-associated genes FKBP5 and STAT5B66 among others.67,68 Early markers for PTSD development have been reported which may aid in developing early detection diagnostic tools. Gene expression data collected in emergency rooms immediately following trauma led to the discovery of 574 differentially expressed genes distinguishing those who would later develop PTSD from those who would recover.69 These transcripts were enriched in genes involved in immune activation, cell cycle, and signal transduction, among other biological processes. While these and other initial results are promising, no validation studies have shown that these biomarkers are valid in independent data sets. Additionally, the biomarkers were identified strictly by differential expression statistics or panels derived from classification algorithms (e.g., support vector machines) and do not incorporate known or inferred biological network information. This limits the ability of these gene panels to give biological insight into the development and progression of PTSD and does not provide a clear path for identification of therapeutics.
Epigenetics
Research on epigenetic mechanisms has revealed that environmental experiences such as early life stress and fear conditioning can modify the expression of genes without altering the corresponding DNA sequence.11,70–73 Most of the studies investigating the role of epigenetics in PTSD have focused on DNA methylation, typically comparing peripheral blood methylation levels between PTSD patients and controls.74–78 In particular, studies have found that the number of uniquely methylated genes was significantly higher in PTSD-affected patients.79 Furthermore, genes found to be hyper-methylated in PTSD patients include: COMT,80 H19, IL18,81 LINE-1,82 PAC1 (female),83 SLC6A3,84 MAN2C1,85 CLEC9A,86 ACP5,86 and TLR8.86 Conversely, genes such as FKBP5,87 Alu,82 SLC6A4,74 TPR, and ANXA286 were found to be hypo-methylated in PTSD. Studies have also found that for subjects with low socioeconomic status, the relationship between DNA methylation and risk of developing PTSD may be modified in genes predominantly related to nervous system function.88
Subtypes of PTSD
The heterogeneous nature of the symptoms and development of PTSD create challenges in diagnosing and treating the disease. While multiple studies have emphasized clear divisions into PTSD subgroups,89,90 very little work has focused on identifying unique biological signatures for these subtypes. Instead, recent work has focused on using symptoms and symptom trajectories to identify these distinct subgroups, or on correlating specific biological measures to these symptom classes. For example, longitudinal data from the Jerusalem Trauma Outreach and Prevention Study identified three trajectories of PTSD symptoms which they called rapid-remitting, slow-remitting and non-remitting.89 Interestingly, they note that only subjects classified as slow-remitting showed symptom improvements when given antidepressants. Similar responses to antidepressants were seen in another study90 where promoter methylation of FKBP5 and GR showed differences between responders and non-responders to treatment. These examples further emphasize the existence of subgroups within PTSD, but overall provide little biological insight into the key differences between these groups. In order to progress in the identification and characterization of these subtypes in a manner useful for diagnostic and therapeutic development, specific biological signatures must be extracted from large-scale data. In particular, the biological features extracted from this type of analysis would provide a more comprehensive picture of subtype biology than these single gene correlations. We note that the identification of subgroups from DNA microarray data has previously been applied to the study of diseases such as cancer and fibromyalgia.91–93
3 Systems biology approach
The molecular inputs used for understanding a given biological system from a systems perspective include genomic, transcriptomic, epigenomic, metabolomic, and proteomic data. These data types measure the genome-wide amounts of and changes to DNA sequence, mRNA expression, DNA methylation/histone modification, metabolite production, and protein expression, respectively. Commonly used experimental techniques for collecting this data include high-throughput sequencing methods, array-based hybridization assays (e.g. microarrays), liquid and gas chromatography, and mass spectrometry. In addition to high-throughput molecular measurements, neuroimaging assays, such as functional and structural MRI, provide an organism-wide measure of brain activity and morphology, respectively. When studying disease mechanisms, each of the above assays can be utilized in either a cross-sectional or longitudinal manner. In the former case, different subjects with and without the disease phenotype are measured simultaneously, with the goal of identifying time point-specific differences between the groups. In the latter case, measurements are repeatedly collected from the same subjects over time, which helps to reduce inter-individual variability and can provide temporal information regarding disease progression. Fig. 2 shows the analysis pipeline typically applied to “omics” data to uncover the underlying biology of PTSD. In the remainder of this section, we review some commonly used methods in this pipeline.
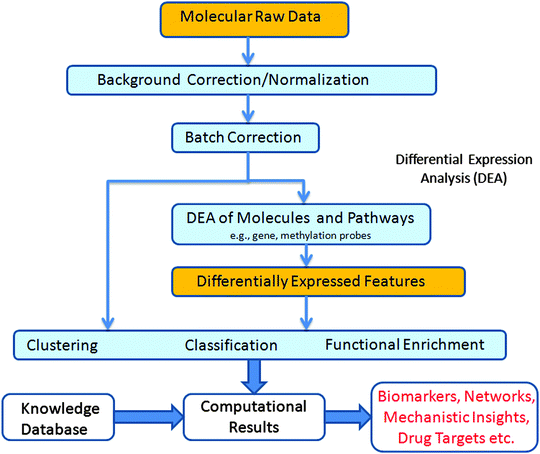 |
| Fig. 2 Analysis pipeline for heterogeneous molecular data generated in PTSD studies. The raw data is first corrected for systematic errors and batch effects, both of which may be introduced during the experimental process. Provided the biological signal is significant, correcting these errors improves the signal-to-noise ratio and ensures more reliable downstream analyses. Following this step, statistical and machine learning tools are used at appropriate stages (shown in the blue boxes) to obtain useful system information (shown in red). | |
Raw data from all of the above experimental modalities are corrupted with noise. It is essential to understand the sources of this noise in order to control for them in downstream analyses, as the final conclusions of the study depends on it. Noise sources can be broadly categorized into two groups: one deriving from the stochastic nature of molecular interactions (referred to as biological noise), and the second coming from the measurement techniques and other experimental strategies used (measurement noise). As an example of the first group, gene expression levels measured in genetically identical cells show substantial cell-to-cell variations.94,95 These unavoidable variations are attributed to random molecular interactions and are known to influence phenotypic properties,96 environmental response,97 decision making at different stages of cell development,98 and information processing.99 In contrast, the measurement noise introduced into the data must be minimized, otherwise it can lead to incorrect downstream analyses.100
As the first step of data analysis, the raw data is corrected for measurement noise. Methods for performing this task with high-throughput data include background correction, normalization and batch correction. The first technique is typically used in fluorescence-based assays for reducing the effects of non-specific background fluorescence. One part of this procedure often involves removing those measurements that are indistinguishable from background noise. Although this introduces missing values into the dataset, such values are preferable to measurements entirely lacking in biological signal. The second technique, normalization, is used in most high-throughput assays to remove systematic biases in the data due to experimental artifacts rather than biological signal. Examples of such biases include differing amounts of biological starting material across assays (between-assay normalization) and unequal fluorescence labeling efficiencies in dual-color fluorescence-based assays (within-assay normalization). Normalization methods often make assumptions about the biological signal present in the data, including that the overall signal range is the same across all samples and that the majority of assayed measurements do not differ significantly between samples.101
Variability in high-throughput data is often associated with location,102 time,103 individuals conducting the experiment and other technical factors.104,105 In addition to measurement noise, this gives rise to what is known as a batch effect—where the structure of measurement error, generally thought to be fixed within each batch, varies across batches. This effect must be taken into account (see the second step in Fig. 2) to allow the integration of data from different batches, thus obtaining greater statistical power for further analyses.106 Methods such as surrogate variable analysis107 (SVA), independent surrogate variable analysis108 (ISVA), and ComBat106 have been used to perform batch correction. ComBat is an empirical Bayes method which is robust to outliers and can also correct for small batch size. When the sources of experimental batches are simple100 (e.g., processing time), ComBat outperforms many other methods.109 However, certain confounding factors are more difficult to ascertain. For these cases, SVA and ISVA provide methods to correct for noise due to unmodelled, unmeasured, or unknown factors. However, we note that these methods do not address the situation where the phenotype of interest is highly correlated with the experimental batches.
Once noise correction has been performed on the available high-throughput data, a range of analysis methods can be applied to characterize the biological signal present. One of the most common methods used to study disease mechanism is differential expression (DE) analysis. The goal of DE analysis in this context is to identify genes/metabolites/proteins that are differentially expressed between disease and control samples. Use of proper statistical testing is essential for this task, as the huge numbers of candidate biomarkers in genome wide studies (e.g., roughly 20
000 genes on a typical microarray) can lead to large numbers of false positive and false negative predictions. Statistical methods like the classical t-test, moderated t-test110 and SAM statistic111 have all been successfully used to generate DE p-values (i.e., probabilities that candidate biomarkers' DE scores are due to random chance rather than biological signal). Given a list of p-values, multiple hypothesis testing correction methods that control the false discovery rate (FDR) or family wise error rate (FWER) can then be used to reduce numbers of false positives and negatives.112 After identifying disease-specific DE candidates, a natural next step is to perform gene set enrichment analysis (GSEA).113 Using a Kolmogorov–Smirnov (K–S) statistic, GSEA identifies statistically enriched biological functions or pathways within a list of candidate biomarkers. GSEA can be very useful for ascribing biological meaning to output from DE analyses.
An alternative to DE analysis for identifying disease biomarkers is to employ machine learning tools. In general, there are two distinct algorithmic approaches, namely (1) supervised and (2) unsupervised learning. The nature of the available data and the objective of the learning task dictates the selection of the proper approach. Unsupervised learning is used when observation labels (e.g., class or category) are not available. Algorithms of this type are widely used for clustering, dimensional reduction, principal component analysis, and probability density estimation of a population. In contrast, supervised learning refers to algorithms that use available observation labels in the learning process. These algorithms are mostly used for classification (i.e., assigning membership labels to a given observation) and regression (i.e., estimating relationships between variables). Supervised classification generally consists of two phases: training and testing. During the training phase, features from samples of known status (e.g., disease or control) are used to build a predictor that is then evaluated during the testing phase on samples of unknown status. Many algorithms are available for classification, including: decision trees, random forests, k-nearest neighbors, Bayesian methods, neural networks, support vector machine (SVM), nearest shrunken centroids (NSC),114 pathway-based classifiers like the condition-responsive genes (CORGs) method,115 and core module biomarker identification with network exploration (COMBINER).116 However, only a limited number of these algorithms have been previously used for PTSD research (e.g., SVM,64 Logistic regression,65 COMBINER116). Currently used methods for PTSD identify biomarker panels by considering combinations of single, unrelated genes or gene sets associated with known pathways. However, none of these methods utilize known molecular interaction networks, which can provide useful insight into network perturbations associated with PTSD, and has shown improvement in classification performance and stability for diseases such as cancer.117 Generally, classification performance can be quantified in several ways; some of the most common include: prediction error rate, sensitivity, precision, F-score and area under the receiver operating characteristic curve (AUC). The choice of which specific classification algorithm to use is critical, as an incorrectly chosen classifier often leads to poor performance. In this case, one must try to select a more appropriate algorithm given factors such as size of the training set, preselection of features given as input to the algorithm, the dimensionality of the dataset, and a potential imbalance in class sample sizes. In addition, algorithm parameters can often be more optimally tuned to improve classification performance. Further information addressing the above can be found in published reviews.118–121
In order to derive mechanistic insights for a given condition, the systems biology approach also attempts to predict interaction networks between different chemical species. To do this, the expression levels of genes, proteins, and metabolites are first measured using high-throughput technologies. Depending on the nature of the species under study, different types of interaction networks can be inferred. For example, network analysis of gene expression data gives rise to gene regulatory networks, while a similar analysis of protein expression data generates signal transduction networks. The inferred relationships between species range from physical interactions (e.g., between transcription factors and their target genes) to functional interactions (e.g., correlated expression between groups of genes). Statistical methods such as regression and correlation analysis,122 mutual information-based approaches,123 Bayesian networks,124 and probabilistic graphical models125 have all been used to predict interactions between species. Detailed reviews of this topic can be found in the following ref. 122, 124, 126–129.
An important challenge in the systems biology approach is the fusion of information from multiple “omics” sources.130 Zhao et al. have used machine learning techniques to perform unsupervised gene biomarker selection in cancer by integrating both mRNA and miRNA data.131,132 Ye et al. have identified Alzheimer's disease biomarkers by combining MRI data, demographic information, and genetic information.133 Finally, Xiang et al. have identified Alzheimer's biomarkers by combining proteomics, PET, MRI and CSF datasets.134 However, to our knowledge there are no studies performing heterogeneous data integration for the analysis of PTSD.
4 Model studies
In this section we review representative studies employing systems tools to investigate PTSD. The first two studies use ordinary differential equation (ODE) models to obtain a mathematical description of the disorder, while those remaining use statistical and machine learning techniques.
Subjects with PTSD are known to have altered fear conditioning responses. As a first case study we highlight the work done by Li et al.,136 where they performed systems modeling of neurons in the lateral amygdala (LA) region (a key site of synaptic events that contribute to fear learning). Using experimental data from the literature, the authors built a computational network model consisting of ten neurons found in the amygdala—eight pyramidal cells and two GABAergic interneurons. Their twin-compartment model represents a soma (diameter of 15 μm; length of 15 μm) and the dominant apical dendrite. The authors derived ODEs for somatic and dendritic membrane potentials by balancing different current sources (e.g. intrinsic, synaptic currents and electrode current). They then used their model to demonstrate the characteristic abilities of the LA in both fear conditioning and extinction. Their results suggest that fear expression is determined by a balance between pyramidal cell and interneuron excitations. Furthermore, the authors show that fear memory is stored in the pyramidal cells, whereas extinction memory is stored in both interneurons and pyramidal cells. Finally, results from the model suggests that fear memory is not erased fully by extinction; rather, it is inhibited by interneurons that undergo synaptic plasticity during extinction training. As emphasized by the authors, their model for the plasticity of inhibitory synapses in the LA improves understanding of the fear mechanism.
Mathematical models of varying complexity have been proposed to describe the circadian dynamics of the HPA axis (see Vinther et al.137 and references therein). An ordinary differential equation model for cortisol dynamics in the HPA axis has been developed by Sriram et al.135 (see Fig. 3), where they seek to understand the mechanism leading to hypocortisolemia in PTSD and hypercortisolemia in depressed patients. The developed model and its estimated parameters support Yehuda's hypothesis that hypocortisolemia in PTSD is due to the strong negative feedback loop operating in the neuroendocrine axis under severe stress.138 The model was used to simulate in silico patients whose behavior suggested that, due to disrupted negative feedback loops, cortisol levels are different in normal, PTSD and depressed subjects during the night. Moreover, the model predicted transitions from normal to diseased states due to changes in the strength of the negative feedback loop and the stress intensity in the neuro-endocrine axis.
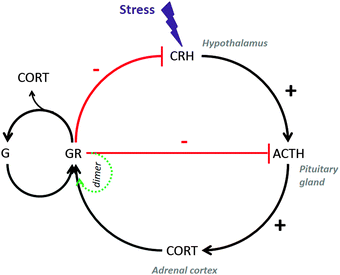 |
| Fig. 3 Regulatory network of cortisol in the HPA axis. Stress induces the secretion of corticotropin release hormone (CRH) in the hypothalamus that in turn activates the release of adreno-corticotropin hormone (ACTH) in the anterior pituitary gland. ACTH moves to the adrenal cortex and stimulates cortisol (CORT). The secreted cortisol binds to glucocorticoid receptors (G) to form a complex GR followed by dimerization. Cortisol complex GR binds to both CRH and ACTH to down regulate the production of cortisol. This closed loop gives rise to a negative feedback in the circuit that is vital in maintaining the homeostasis of the system during stress.135 | |
Animal models have been used to investigate the effects of trauma and draw parallels with PTSD and other neurodegenerative disorders in humans. Next we consider a recent study by Hammamieh et al. where they developed a “social-defeat” model by exposing male C57BL/6J mice to aggressor mice for six hours daily for five or 10 days in total, using a “cage-within-cage resident-intruder” protocol.140 The mice showed significant behavioral, physiological, and histological changes associated with the trauma stressor. Furthermore, seven brain regions, including the hippocampus, amygdala, and medial prefrontal cortex, as well as whole blood were harvested and the corresponding whole-cell mRNA populations were extracted. In order to understand the underlying molecular principles, Yang et al.139 used a computational tool entitled core module biomarker identification with network exploration (COMBINER). This approach using machine learning and statistical tools, was first developed by Yang et al.116 COMBINER provides a novel method for the identification of disease biomarkers. Designed to take multiple data cohorts as input, COMBINER identifies gene module biomarkers that are consistent across the population of samples and constructs their associated regulatory networks. The social defeat study identified common differentially expressed genes in multiple brain regions as well as between the brain and blood. These DEGs were used to obtain the core disease module (see Fig. 4). Many of the resulting modular gene networks were found to be previously associated with PTSD, depression, and other neurodegenerative diseases.
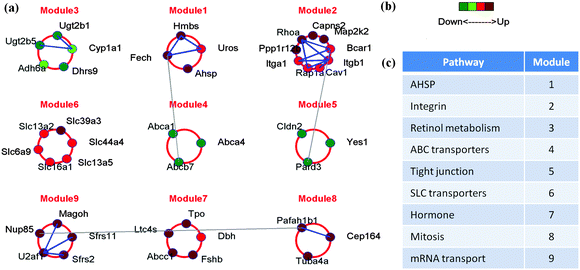 |
| Fig. 4 Blood-brain gene network for a “social defeat” mouse model. (a) Nine core gene expression modules identified by COMBINER. The core modules consist of only those genes that are expressed in both blood and multiple brain regions (which include the amygdala, hippocampus, hemibrain, medial PFC, septal region, stria terminalis and ventral striatum). Known protein–protein interactions (PPIs) are marked by lines connecting genes—blue lines denote within-module interactions, while gray lines denote between-module interactions. (b) The color scale for the blood expression level of each gene in the nine modules, as indicated by the colored circles in (a). (c) Putative biological functions of the expression modules as inferred using KEGG annotation. The above figure has been adapted from Yang et al.139 | |
Studies141,142 have indicated that subjects with PTSD also have a higher risk of cardiovascular conditions, including increased basal heart rate and blood pressure, higher risk for hypertension and stroke. A recent study by Cho et al.143 used a systems approach to study the effect of traumatic experiences on heart function using the animal model developed by Hammamieh et al.140 In particular, they found that myocarditis was a frequently detected pathology in stressed subject mice, suggesting a stress-associated heart injury. The authors studied the temporal expression of mRNA and miRNA in heart samples obtained from stressed and control animals, providing a characterization of the heart tissue repair process in response to stress-induced tissue injury. Inflammatory-related genes were found to be significantly regulated after as little as one day of exposure to stress, and most of the changes in gene expression returned to normal levels after a recovery period. Additionally, key molecular processes involved in classical wound healing in the heart tissues of subject mice were identified, including the chemokine signaling pathway, extracellular remodeling and epithelial to mesenchymal transition (EMT). Taken together, the results of Cho et al.143 suggest a molecular basis for the observed higher risk of cardiovascular disorders in PTSD patients, further illustrating the increased likelihood of cardiac dysfunction induced by long-term stress exposure.
Blood samples are one of the most common sources for obtaining biological data. A gene expression study of the peripheral blood mononuclear cells (PBMC) has been performed by Segman et al.69 In this study, blood samples were collected for subjects visiting the emergency room following trauma as well as after a four month follow-up visit. The aim of the study was to identify the PTSD gene expression signature by applying differential expression analysis, classification, and functional enrichment of the blood samples collected. Differential expression analysis identified DEGs as genes which were significantly (p < 0.05) expressed using three methods: t-test, threshold number of misclassifications (TNoM), and the Info144 method. The number of DEGs identified by the intersection of these three methods is 408, 574, and 656 genes for the emergency room, four month follow-up, and combined times points, respectively. Next, a Naive Bayesian Classifier was trained and tested using leave-one-out cross validation (LOOCV) on the samples from the emergency room (ER) (6 PTSD, 5 control) and the four month follow-up (5 PTSD, 4 control). The ER and follow-up classifiers had misclassification error rates of 18% and 11%, respectively with all misclassifications being false positives. Additionally, gene ontology enrichment analysis was used to understand the biological pathways related to the identified PTSD signature. Terms significantly enriched in DEGs from the differential expression analysis were determined using the hypergeometric p-value and a false discovery rate (FDR) cutoff of 0.1. Terms related to RNA processing, metabolism, and binding as well as nucleic acid metabolism and binding were found to be significant. Overall, this exploratory analysis presented both gene and pathway-level analysis of PTSD mRNA signatures from blood, the result of which require further validation in larger studies to understand their usefulness as molecular markers of PTSD.
In addition to gene expression signatures in blood, there has been increasing interest in epigenetic markers of PTSD, specifically DNA methylation. The changes in DNA methylation can occur during development, but also later in life in response to an individual's environment and experience. These epigenetic changes can create long-term changes in gene expression, resulting in disease phenotypes involving perturbed biological function. The first published epigenetic study of PTSD79 identified genes which were uniquely methylated or unmethylated in those who developed PTSD, compared to control subjects who had experienced similar levels of trauma. This exploratory study of methylation in 14
000 genes over 23 PTSD and 77 control samples identified approximately 400 genes which were uniquely methylated or unmethylated in PTSD subjects using the Wilcoxon test (p < 0.01). To understand the related biology, the authors used functional annotation clustering (FAC) analysis to identify Gene Ontology terms that were enriched in the set of uniquely methylated and unmethylated genes. Terms related to both the innate and adaptive immune system were identified as the most significantly enriched. This finding was further strengthened by an independent measure of immune function: the amount of antibodies to a common herpesvirus, CMV. Antibody levels were significantly different in PTSD vs. control subjects (p = 0.016). Finally, the authors used ANOVA to assess the contributions of comorbid diseases and found that only a small number of genes showed differential methylation across groups divided by comorbidity, indicating that the PTSD methylation findings are specific to PTSD and not affected by the increased rates of major depressive disorder or generalized anxiety disorder in PTSD populations.
5 Conclusions and future challenges
As stated in the Introduction, post-traumatic stress disorder is the only psychological disorder for which the onset of the pathological condition is fairly precise, i.e., occurring after an extreme traumatic event. A wealth of often contradictory physiological findings point to the underlying complexity of the disorder. Although considered a psychological disorder, PTSD also affects the functioning of numerous non-neurological systems in the body, including the immune, endocrine and cardiovascular systems. In order to understand the relationship between the progression of PTSD and these other systemic responses, specific details about the molecular mechanism must be unraveled. In particular, details analogous to the increased negative feedback identified in the HPA axis135 must be identified to understand the cardiovascular and immunological changes that frequently occur in PTSD. However, identifying the relevant components in these systems is further complicated by the high rates of comorbid diseases (biological noise) and experimental artifacts from high-throughput experiments (experimental noise). Addressing these challenges will require careful consideration and likely novel adaptations to current machine learning strategies, including the use (or development) of data integration techniques that can accommodate data from multiple biological length scales.
The complexity of the disorder has posed a great challenge to the identification of biomarkers for diagnostic, prognostic and therapeutic applications. The time dependent profile, different for each subject, further complicates the diagnosis. A recent clinical study89 has shown three different trajectories of symptom progression: rapid-remitting (fast decrease of symptoms in one to five months), slow-remitting (symptoms decrease after 15 months) and non-remitting (persistent symptoms). In order to understand the three clinical trajectories from a biological perspective and to explore the prognostic and therapeutic opportunities, it is increasingly evident that integration of both the biological and the clinical manifestations of PTSD is essential. Additionally, if these symptom trajectories or other distinct subtypes of PTSD exist, the choice of how and where these subgroups should be integrated or separated for biomarker identification analysis must be systematically explored. Specifically, the biological mechanism of PTSD development must be extensively studied (using longitudinal data) in all potential subtypes of PTSD to determine if overlapping signals exist. In the absence of overlapping biological mechanisms, multi-class classification strategies may be required. Since PTSD originates in the brain, it is extremely difficult to collect samples for scientific study. Alternatively, animal models are used to correlate with clinical findings, thus enabling one to draw inferences that may lead to diagnostic and therapeutic solutions.
Many animal models that exhibit one or more “PTSD-specific” symptoms have been proposed, including predator-exposure,145–147 exposure to single prolonged stress,148 and exposure to foot shock149,150 with additional stress. However none to date have been widely accepted as an ideal model for PTSD. These models approximately mimic physiological and tissue-level responses in human PTSD subjects and have helped in identifying biological processes, such as the HPA axis, neurotransmitter receptor system, and others (see review by Pitman et al.12), that may be involved in PTSD. In the model studies section of this review, we presented a study by Cho et al.143 based on the social defeat mouse model140 that identifies key short-term physiological and histological changes that occur in PTSD-like mice. These changes, including cardiac histopathology differences as well as increased weight gain, show similarities to established comorbid PTSD phenotypes of obesity and cardiovascular disease. In addition to gaining insight into development and progression of PTSD, animal models may also provide an avenue for pharmacological studies. For example, the social defeat mouse model responds to chronic (but not acute) exposure to antidepressant medication, which agrees with human PTSD responses. In general, these proposed animal models of PTSD have shown agreement with previously identified pharmacological, organismal and tissue-level responses in humans. However, to the best of our knowledge, no study to date has compared long-term animal model response with human PTSD subjects. These long-term responses, as well as detailed cellular and molecular changes across all time scales must be carefully addressed in future studies to more conclusively identify how well animal model findings can be translated to understand PTSD in humans.
In light of the complexity of the PTSD disease mechanism, a systems approach integrating both the clinical findings and animal validation studies is necessary to understand the underlying mechanisms driving PTSD and its variants. Such an approach establishes a multidimensional research direction combining patients, published animal models and mathematical analysis tools. The goals of such an approach is to (1) identify the differentially expressed genomic, proteomic and metabolic drivers of PTSD; (2) identify approaches to diagnose different clinically observed PTSD trajectories; (3) identify possible biological risk factors, prognostic indicators, and therapeutic strategies; (4) identify the underlying molecular principles to facilitate detailed studies of relevant molecular networks. In order to achieve these goals there is a need to integrate heterogeneous experimental data (e.g., molecular and neuroimaging data) from different studies by employing new or existing mathematical tools to uncover useful information from large noisy datasets (on the order of a million data points per subject). In this paper we outline the preliminary studies that pave the way for a multidisciplinary integrated systems biology approach, and highlight some current challenges to identifying and understanding the molecular underpinnings of post-traumatic stress disorder.
Acknowledgements
This work was supported in part by the institute for Collaborative Biotechnologies through grant from the US Army research office (W911NF-10-2-0111) and the grant from USAMRMC (09284002).
References
-
T. M. Keane, B. P. Marx and D. M. Sloan, Post-Traumatic Stress Disorder, Springer, 2009, pp. 1–19 Search PubMed.
- K. H. Seal, T. J. Metzler, K. S. Gima, D. Bertenthal, S. Maguen and C. R. Marmar, Am. J. Public Health, 2009, 99, 1651–1658 CrossRef PubMed.
-
D. Blake, F. Weathers, L. Nagy, D. Kaloupek, G. Klauminzer, D. Charney and T. Keane, Clinician-Administered PTSD Scale (CAPS), National Center for Post-Traumatic Stress Disorder, Behavioral Science Division, Boston, MA, 1990 Search PubMed.
- E. B. Foa, L. Cashman, L. Jaycox and K. Perry, Psychol. Assess., 1997, 9, 445 CrossRef.
- L. Hood, J. R. Heath, M. E. Phelps and B. Lin, Science, 2004, 306, 640–643 CrossRef CAS PubMed.
- S. Draghici, P. Khatri, A. L. Tarca, K. Amin, A. Done, C. Voichita, C. Georgescu and R. Romero, Genome Res., 2007, 17, 1537–1545 CrossRef CAS PubMed.
- H.-Y. Chuang, M. Hofree and T. Ideker, Annu. Rev. Cell Dev. Biol., 2010, 26, 721 CrossRef CAS PubMed.
- A.-L. Barabási, N. Gulbahce and J. Loscalzo, Nat. Rev. Genet., 2011, 12, 56–68 CrossRef PubMed.
- H. Kitano, Science, 2002, 295, 1662–1664 CrossRef CAS PubMed.
- R. Patel, R. N. Spreng, L. M. Shin and T. A. Girard, Neurosci. Biobehav. Rev., 2012, 36, 2130–2142 CrossRef PubMed.
- P. R. Zoladz and D. M. Diamond, Neurosci. Biobehav. Rev., 2013, 1 CAS.
- R. K. Pitman, A. M. Rasmusson, K. C. Koenen, L. M. Shin, S. P. Orr, M. W. Gilbertson, M. R. Milad and I. Liberzon, Nat. Rev. Neurosci., 2012, 13, 769–787 CrossRef CAS PubMed.
- K. Skelton, K. J. Ressler, S. D. Norrholm, T. Jovanovic and B. Bradley-Davino, Neuropharmacology, 2012, 62, 628–637 CrossRef CAS PubMed.
- D. G. Baker, C. M. Nievergelt and D. T. O'Connor, Neuropharmacology, 2012, 62, 663–673 CrossRef CAS PubMed.
- E. B. Foa, D. S. Riggs and B. S. Gershuny, Am. J. Psychiatry, 1995, 1 Search PubMed.
-
A. Prins, D. G. Kaloupek and T. M. Keane, Psychophysiological evidencefor autonomic arousal and startle in traumatized adult populations., Lippincott Williams & Wilkins Publishers, 1995, pp. 291–314 Search PubMed.
- N. Pole, Psychol. Bull., 2007, 133, 725 CrossRef PubMed.
- A. Y. Shalev, T. Sahar, S. Freedman, T. Peri, N. Glick, D. Brandes, S. P. Orrand and R. K. Pitman, Arch. Gen. Psychiatry, 1998, 55, 553 CrossRef CAS.
-
J. J. P. Wilson and T. M. Keane, in Assessing psychological trauma and PTSD, ed. J. P. Wilson and T. M. Keane, Guilford Press, 2004 Search PubMed.
-
J. J. Vasterling and C. R. Brewin, Neuropsychology of PTSD: Biological, cognitive, and clinical perspectives, Guilford Press, 2005 Search PubMed.
- E. B. Blanchard, E. J. Hickling, T. C. Buckley, A. E. Taylor, A. Vollmer and W. R. Loos, J. Consult. Clin. Psychol., 1996, 64, 742 CrossRef CAS.
- B. Kleim, F. H. Wilhelm, E. Glucksman and A. Ehlers, Psychosom. Med., 2010, 72, 917–924 CrossRef PubMed.
- N. Kitayama, V. Vaccarino, M. Kutner, P. Weiss and J. D. Bremner, J. Affective Disord., 2005, 88, 79–86 CrossRef PubMed.
- Z. Wang, T. C. Neylan, S. G. Mueller, M. Lenoci, D. Truran, C. R. Marmar, M. W. Weiner and N. Schuff, Arch. Gen. Psychiatry, 2010, 67, 296–303 CrossRef PubMed.
- K. Kasai, H. Yamasue, M. W. Gilbertson, M. E. Shenton, S. L. Rauch and R. K. Pitman, Biol. Psychiatry, 2008, 63, 550–556 CrossRef PubMed.
- N. Kitayama, S. Quinn and J. D. Bremner, J. Affective Disord., 2006, 90, 171–174 CrossRef PubMed.
- S. Chen, W. Xia, L. Li, J. Liu, Z. He, Z. Zhang, L. Yan, J. Zhang and D. Hu, Psychiatry Res., Neuroimaging, 2006, 146, 65–72 CrossRef PubMed.
- S. Kühn and J. Gallinat, Biol. Psychiatry, 2013, 73, 70–74 CrossRef PubMed.
- M. W. Gilbertson, M. E. Shenton, A. Ciszewski, K. Kasai, N. B. Lasko, S. P. Orrand and R. K. Pitman, Nat. Neurosci., 2002, 5, 1242–1247 CrossRef CAS PubMed.
- M. D. De Bellis, J. Hall, A. M. Boring, K. Frustaci and G. Moritz, Biol. Psychiatry, 2001, 50, 305–309 CrossRef CAS.
- O. Bonne, D. Brandes, A. Gilboa, J. M. Gomori, M. E. Shenton, R. K. Pitman and A. Y. Shalev, Am. J. Psychiatry, 2001, 158, 1248–1251 CrossRef CAS PubMed.
- I. Liberzon, S. F. Taylor, R. Amdur, T. D. Jung, K. R. Chamberlain, S. Minoshima, R. A. Koeppe and L. M. Fig, Biol. Psychiatry, 1999, 45, 817–826 CrossRef CAS.
- A. Etkin and T. D. Wager, Am. J. Psychiatry, 2007, 164, 1476 CrossRef PubMed.
- G. A. Fonzo, A. N. Simmons, S. R. Thorp, S. B. Norman, M. P. Paulus and M. B. Stein, Biol. Psychiatry, 2010, 68, 433–441 CrossRef PubMed.
- A. N. Simmons, M. P. Paulus, S. R. Thorp, S. C. Matthews, S. B. Norman and M. B. Stein, Biol. Psychiatry, 2008, 64, 681–690 CrossRef PubMed.
- A. Gold, L. Shin, S. Orr, M. Carson, S. Rauch, M. Macklin, N. Lasko, L. Metzger, D. Dougherty and N. Alpert,
et al.
, Psychol. Med., 2011, 41, 1–10 CrossRef PubMed.
- L. M. Shin, N. B. Lasko, M. L. Macklin, R. D. Karpf, M. R. Milad, S. P. Orr, J. M. Goetz, A. J. Fischman, S. L. Rauch and R. K. Pitman, Arch. Gen. Psychiatry, 2009, 66, 1099–1107 CrossRef PubMed.
- L. M. Shin, G. Bush, M. R. Milad, N. B. Lasko, K. H. Brohawn, K. C. Hughes, M. L. Macklin, A. L. Gold, R. D. Karpf and S. P. Orr,
et al.
, Am. J. Psychiatry, 2011, 168, 979–985 CrossRef PubMed.
- M. Stein, A. Simmons, J. Feinstein and M. Paulus, Am. J. Psychiatry, 2007, 164, 318–327 CrossRef PubMed.
- M. P. Paulus and M. B. Stein, Biol. Psychiatry, 2006, 60, 383–387 CrossRef PubMed.
- X. Yan, A. D. Brown, M. Lazar, V. L. Cressman, C. Henn-Haase, T. C. Neylan, A. Shalev, O. M. Wolkowitz, S. P. Hamilton and R. Yehuda,
et al.
, Neurosci. Lett., 2013, 547, 1–5 CrossRef CAS PubMed.
- C. A. Rabinak, M. Angstadt, R. C. Welsh, A. E. Kenndy, M. Lyubkin, B. Martis and K. L. Phan, Front. Psychiatry, 2011, 2, 241–249 Search PubMed.
- C. Damsa, M. Kosel and J. Moussally, Curr. Opin. Psychiatry, 2009, 22, 96–110 CrossRef PubMed.
- L. M. Shin, S. P. Orr, M. A. Carson, S. L. Rauch, M. L. Macklin, N. B. Lasko, P. M. Peters, L. J. Metzger, D. D. Dougherty and P. A. Cannistraro,
et al.
, Arch. Gen. Psychiatry, 2004, 61, 168 CrossRef PubMed.
- L. M. Shin, C. I. Wright, P. A. Cannistraro, M. M. Wedig, K. McMullin, B. Martis, M. L. Macklin, N. B. Lasko, S. R. Cavanagh and T. S. Krangel,
et al.
, Arch. Gen. Psychiatry, 2005, 62, 273 CrossRef PubMed.
- L. M. Williams, A. H. Kemp, K. Felmingham, M. Barton, G. Olivieri, A. Peduto, E. Gordon and R. A. Bryant, NeuroImage, 2006, 29, 347–357 CrossRef PubMed.
- M. Davis and P. J. Whalen,
et al.
, Mol. Psychiatry, 2001, 6, 13–34 CrossRef CAS.
- J. S. Morris, A. Öhman and R. J. Dolan, Nature, 1998, 393, 467–470 CrossRef CAS PubMed.
- T. A. Hare, C. F. Camerer and A. Rangel, Science, 2009, 324, 646–648 CrossRef CAS PubMed.
- K. Felmingham, L. M. Williams, A. H. Kemp, B. Liddell, E. Falconer, A. Peduto and R. Bryant, J. Abnorm. Psychol., 2010, 119, 241 CrossRef PubMed.
- M. R. Milad, R. K. Pitman, C. B. Ellis, A. L. Gold, L. M. Shin, N. B. Lasko, M. A. Zeidan, K. Handwerger, S. P. Orr and S. L. Rauch, Biol. Psychiatry, 2009, 66, 1075–1082 CrossRef PubMed.
- A. Rougemont-Bücking, C. Linnman, T. A. Zeffiro, M. A. Zeidan, K. Lebron-Milad, J. Rodriguez-Romaguera, S. L. Rauch, R. K. Pitman and M. R. Milad, CNS Neurosci. Ther., 2011, 17, 227–236 CrossRef PubMed.
- J. Pannu Hayes, K. S. LaBar, C. M. Petty, G. McCarthy and R. A. Morey, Psychiatry Res., Neuroimaging, 2009, 172, 7–15 CrossRef PubMed.
- G. Bush, P. Luu and M. I. Posner, Trends Cognit. Sci., 2000, 4, 215–222 CrossRef.
- A. Etkin, T. Egner and R. Kalisch, Trends Cognit. Sci., 2011, 15, 85–93 CrossRef PubMed.
- L. R. Squire and S. Zola-Morgan, Science, 1991, 253, 1380–1386 CrossRef CAS.
-
H. Eichenbaum and N. J. Cohen, From conditioning to consciousrecollection: Memory systems of the brain., Oxford University Press, 2001 Search PubMed.
- L. M. Shin and I. Liberzon, Neuropsychopharmacology, 2009, 35, 169–191 CrossRef PubMed.
- J. D. Bremner, M. Vythilingam, E. Vermetten, S. M. Southwick, T. McGlashan, A. Nazeer, S. Khan, L. V. Vaccarino, R. Soufer and P. K. Garg,
et al.
, Am. J. Psychiatry, 2003, 160, 924–932 CrossRef PubMed.
- H. D. Critchley, S. Wiens, P. Rotshtein, A. Öhman and R. J. Dolan, Nat. Neurosci., 2004, 7, 189–195 CrossRef CAS PubMed.
- I. A. Strigo, A. N. Simmons, S. C. Matthews, E. M. Grimes, C. B. Allard, L. E. Reinhardt, M. P. Paulus and M. B. Stein, Biol. Psychiatry, 2010, 68, 442–450 CrossRef PubMed.
- N. P. Daskalakis, A. Lehrner and R. Yehuda, Endocrinol. Metab. Clin. North Am., 2013, 42, 503–513 Search PubMed.
- R. Yehuda, Ann. N. Y. Acad. Sci., 2006, 1071, 137–166 CrossRef CAS PubMed.
- S. J. Glatt, D. S. Tylee, S. D. Chandler, J. Pazol, C. M. Nievergelt, C. H. Woelk, D. G. Baker, J. B. Lohr, W. S. Kremen and B. T. Litz,
et al.
, Am. J. Med. Genet., Part B, 2013, 1 Search PubMed.
- M. van Zuiden, E. Geuze, H. L. Willemen, E. Vermetten, M. Maas, K. Amarouchi, A. Kavelaars and C. J. Heijnen, Biol. Psychiatry, 2012, 71, 309–316 CrossRef CAS PubMed.
- R. Yehuda, G. Cai, J. A. Golier, C. Sarapas, S. Galea, M. Ising, T. Rein, J. Schmeidler, B. Muller-Myhsok, F. Holsboer and J. D. Buxbaum, Biol. Psychiatry, 2009, 66, 708–711 CrossRef CAS PubMed.
- J. Zieker, D. Zieker, A. Jatzko, J. Dietzsch, K. Nieselt, A. Schmitt, T. Bertsch, K. Fassbender, R. Spanagel, H. Northoff and P. Beicke-Haerter, Mol. Psychiatry, 2007, 12, 116–118 CrossRef CAS PubMed.
- T. Neylan, B. Sun, H. Rempel, J. Ross, M. Lenoci, A. O'Donovan and L. Pulliam, Brain, Behav., Immun., 2011, 25, 524–531 CrossRef CAS PubMed.
- R. Segman, N. Shefi, T. Goltser-Dubner, N. Friedman, N. Kaminski and Y. Shalev, Mol. Psychiatry, 2005, 10, 500–513 CrossRef CAS PubMed.
- M. Heinzelmann and J. Gill, Nurs. Res. Pract., 2013, 417010 Search PubMed.
- I. B. Zovkic and J. D. Sweatt, Neuropsychopharmacology, 2012, 38, 77–93 CrossRef PubMed.
- E. B. Binder, R. G. Bradley, W. Liu, M. P. Epstein, T. C. Deveau, K. B. Mercer, Y. Tang, C. F. Gillespie, C. M. Heim and C. B. Nemeroff,
et al.
, JAMA, J. Am. Med. Assoc., 2008, 299, 1291–1305 CrossRef CAS PubMed.
- C. L. Hirsch and K. Bonham, FEBS Lett., 2004, 570, 37–40 CrossRef CAS PubMed.
- K. C. Koenen, M. Uddin, S.-C. Chang, A. E. Aiello, D. E. Wildman, E. Goldmann and S. Galea, Depression Anxiety, 2011, 28, 639–647 CrossRef CAS PubMed.
- T. Klengel, D. Mehta, C. Anacker, M. Rex-Haffner, J. C. Pruessner, C. M. Pariante, T. W. Pace, K. B. Mercer, H. S. Mayberg and B. Bradley,
et al.
, Nat. Neurosci., 2012, 16, 33–41 CrossRef PubMed.
- J. Feng, Y. Zhou, S. L. Campbell, T. Le, E. Li, J. D. Sweatt, A. J. Silva and G. Fan, Nat. Neurosci., 2010, 13, 423–430 CrossRef CAS PubMed.
- J. U. Guo, D. K. Ma, H. Mo, M. P. Ball, M.-H. Jang, M. A. Bonaguidi, J. A. Balazer, H. L. Eaves, B. Xie and E. Ford,
et al.
, Nat. Neurosci., 2011, 14, 1345–1351 CrossRef CAS PubMed.
- F. D. Lubin, T. L. Roth and J. D. Sweatt, J. Neurosci., 2008, 28, 10576–10586 CrossRef CAS PubMed.
- M. Uddin, A. E. Aiello, D. E. Wildman, K. C. Koenen, G. Pawelec, R. de Los Santos, E. Goldmann and S. Galea, Proc. Natl. Acad. Sci. U. S. A., 2010, 107, 9470–9475 CrossRef CAS PubMed.
- S. D. Norrholm, T. Jovanovic, A. K. Smith, E. Binder, T. Klengel, K. Conneely, K. B. Mercer, J. S. Davis, K. Kerley and J. Winkler,
et al.
, Front. Behav. Neurosci., 2013, 7, 524–531 Search PubMed.
- J. A. Rusiecki, C. Byrne, Z. Galdzicki, V. Srikantan, L. Chen, M. Poulin, L. Yan and A. Baccarelli, Front. Psychiatry, 2013, 4, 29–40 Search PubMed.
- J. A. Rusiecki, L. Chen, V. Srikantan, L. Zhang, L. Yan, M. L. Polin and A. Baccarelli, Epigenomics, 2012, 4, 29–40 CrossRef CAS PubMed.
- K. J. Ressler, K. B. Mercer, B. Bradley, T. Jovanovic, A. Mahan, K. Kerley, S. D. Norrholm, V. Kilaru, A. K. Smith and A. J. Myers,
et al.
, Nature, 2011, 470, 492–497 CrossRef CAS PubMed.
- S.-C. Chang, K. C. Koenen, S. Galea, A. E. Aiello, R. Soliven, D. E. Wildman and M. Uddin, PLoS One, 2012, 7, e39184 CAS.
- M. Uddin, S. Galea, S.-C. Chang, A. E. Aiello, D. E. Wildman, R. de los Santos and K. C. Koenen, Dis. Markers, 2011, 30, 111–121 CrossRef CAS PubMed.
- A. K. Smith, K. N. Conneely, V. Kilaru, K. B. Mercer, T. E. Weiss, B. Bradley, Y. Tang, C. F. Gillespie, J. F. Cubells and K. J. Ressler, Am. J. Med. Genet., Part B, 2011, 156, 700–708 CrossRef CAS PubMed.
- T. Klengel, D. Mehta, C. Anacker, M. Rex-Haffner, J. C. Pruessner, C. M. Pariante, T. W. Pace, K. B. Mercer, H. S. Mayberg and B. Bradley,
et al.
, Nat. Neurosci., 2013, 16, 33–41 CrossRef CAS PubMed.
- M. Uddin, S. Galea, S. C. Chang, K. C. Koenen, E. Goldmann, D. E. Wildman and A. E. Aiello, Biodemography Soc. Biol., 2013, 59, 68–84 CrossRef PubMed.
- I. R. Galatzer-Levy, Y. Ankri, S. Freedman, Y. Israeli-Shalev, P. Roitman, M. Gilad and A. Y. Shalev, PLoS One, 2013, 8, e70084 CAS.
- R. Yehuda, N. P. Daskalakis, F. Desarnaud, I. Makotkine, A. L. Lehrner, E. Koch, J. D. Flory, J. D. Buxbaum, M. J. Meaney and L. M. Bierer, Front. Psychiatry, 2013, 4, 1 CrossRef PubMed.
- Y. Drier, M. Sheffer and E. Domany, Proc. Natl. Acad. Sci. U. S. A., 2013, 2013, 6388–6393 CrossRef PubMed.
- Y. Liu, Q. Gu, J. P. Hou and J. Ma, BMC Bioinf., 2014, 15, 37 CrossRef PubMed.
- E. Docampo, A. Collado, G. Escarams, J. Carbonell, J. Rivera, J. Vidal, J. Alegre, R. Rabionet and X. Estivill, PLoS One, 2013, 8, e74873 CAS.
- M. Krn, T. C. Elston, W. J. Blake and J. J. Collins, Nat. Rev. Genet., 2005, 6, 451–464 CrossRef PubMed.
-
Y. Pilpel, Yeast Systems Biology, Springer, 2011, pp. 407–425 Search PubMed.
- D. Fraser and M. Krn, Mol. Microbiol., 2009, 71, 1333–1340 CrossRef CAS PubMed.
- A. Singh, B. S. Razooky, R. D. Dar and L. S. Weinberger, Mol. Syst. Biol., 2012, 8, 383–387 CrossRef PubMed.
- T. Enver, C. M. Heyworth and T. M. Dexter, Blood, 1998, 92, 348–351 CAS.
- I. Lestas, G. Vinnicombe and J. Paulsson, Nature, 2010, 467, 174–178 CrossRef CAS PubMed.
- J. T. Leek, R. B. Scharpf, H. C. Bravo, D. Simcha, B. Langmead, W. E. Johnson, D. Geman, K. Baggerly and R. A. Irizarry, Nat. Rev. Genet., 2010, 11, 733–739 CrossRef CAS PubMed.
- J. Quackenbush, Nat. Genet., 2002, 32, 496–501 CrossRef CAS PubMed.
- A. P. Gasch, P. T. Spellman, C. M. Kao, O. Carmel-Harel, M. B. Eisen, G. Storz, D. Botstein and P. O. Brown, Mol. Biol. Cell, 2000, 11, 4241–4257 CrossRef CAS.
- J. D. Storey, W. Xiao, J. T. Leek, R. G. Tompkins and R. W. Davis, Proc. Natl. Acad. Sci. U. S. A., 2005, 102, 12837–12842 CrossRef CAS PubMed.
- M. K. Kerr and G. A. Churchill, Biostatistics, 2001, 2, 183–201 CrossRef PubMed.
- M. K. Kerr, M. Martin and G. A. Churchill, J. Comput. Biol., 2000, 7, 819–837 CrossRef CAS PubMed.
- W. E. Johnson, C. Li and A. Rabinovic, Biostatistics, 2007, 8, 118–127 CrossRef PubMed.
- J. T. Leek and J. D. Storey, PLoS Genet., 2007, 3, e161 Search PubMed.
- A. E. Teschendorff, J. Zhuang and M. Widschwendter, Bioinformatics, 2011, 27, 1496–1505 CrossRef CAS PubMed.
- C. Chen, K. Grennan, J. Badner, D. Zhang, E. Gershon, L. Jin and C. Liu, PLoS One, 2011, 6, e17238 CAS.
- G. K. Smyth,
et al.
, Stat. Appl. Genet. Mol. Biol., 2004, 3, 3 Search PubMed.
- V. G. Tusher, R. Tibshirani and G. Chu, Proc. Natl. Acad. Sci. U. S. A., 2001, 98, 5116–5121 CrossRef CAS PubMed.
- S. Dudoit, J. P. Shaffer and J. C. Boldrick, Stat. Sci., 2003, 71–103 CrossRef.
- A. Subramanian, P. Tamayo, V. K. Mootha, S. Mukherjee, B. L. Ebert, M. A. Gillette, A. Paulovich, S. L. Pomeroy, T. R. Golub and E. S. Lander,
et al.
, Proc. Natl. Acad. Sci. U. S. A., 2005, 102, 15545–15550 CrossRef CAS PubMed.
- T. Srlie, R. Tibshirani, J. Parker, T. Hastie, J. Marron, A. Nobel, S. Deng, H. Johnsen, R. Pesich and S. Geisler,
et al.
, Proc. Natl. Acad. Sci. U. S. A., 2003, 100, 8418–8423 CrossRef PubMed.
- E. Lee, H.-Y. Chuang, J.-W. Kim, T. Ideker and D. Lee, PLoS Comput. Biol., 2008, 4, e1000217 Search PubMed.
- R. Yang, B. J. Daigle, L. R. Petzold and F. J. Doyle, BMC Bioinf., 2012, 13, 12 CrossRef PubMed.
- H.-Y. Chuang, E. Lee, Y.-T. Liu, D. Lee and T. Ideker, Mol. Syst. Biol., 2007, 3, 140 CrossRef PubMed.
- C. Ma, H. H. Zhang and X. Wang, Trends Plant Sci., 2014, 19, 798–808 CrossRef CAS PubMed.
- S. B. Kotsiantis, Informatica, 2007, 31, 249–268 Search PubMed.
- A. L. Swan, A. Mobasheri, D. Allaway, S. Liddell and J. Bacardit, OMICS: J. Integr. Biol., 2013, 17, 595–610 CrossRef CAS PubMed.
-
C. M. Bishop, et al., Pattern recognition and machine learning, Springer, New York, 2006, vol. 1 Search PubMed.
- D. Marbach, J. C. Costello, R. Küffner, N. M. Vega, R. J. Prill, D. M. Camacho, K. R. Allison, M. Kellis, J. J. Collins and G. Stolovitzky,
et al.
, Nat. Methods, 2012, 9, 796–804 CrossRef CAS PubMed.
- X. Zhang, X.-M. Zhao, K. He, L. Lu, Y. Cao, J. Liu, J.-K. Hao, Z.-P. Liu and L. Chen, Bioinformatics, 2012, 28, 98–104 CrossRef CAS PubMed.
-
H. Guo and W. Hsu, AAAI/KDD/UAI02 Joint Workshop on Real-Time Decision Support and Diagnosis Systems, 2002.
- N. Friedman, Science, 2004, 303, 799–805 CrossRef CAS PubMed.
- M. Bansal, V. Belcastro, A. Ambesi-Impiombato and D. Di Bernardo, Mol. Syst. Biol., 2007, 3, 1–10 CrossRef.
- P. Dhaeseleer, S. Liang and R. Somogyi, Bioinformatics, 2000, 16, 707–726 CrossRef CAS PubMed.
- D. Marbach, R. J. Prill, T. Schaffter, C. Mattiussi, D. Floreano and G. Stolovitzky, Proc. Natl. Acad. Sci. U. S. A., 2010, 107, 6286–6291 CrossRef CAS PubMed.
-
J. D. Gibbons and S. Chakraborti, Nonparametric statistical inference, Springer, 2011 Search PubMed.
- G. Genovese, A. Ergun, S. A. Shukla, B. Campos, J. Hanna, P. Ghosh, S. N. Quayle, K. Rai, S. Colla and H. Ying,
et al.
, Cancer Discovery, 2012, 2, 736–749 CrossRef CAS PubMed.
- Z. Zhao and H. Liu, J. Mach. Learn Res., 2008, 4, 36–47 Search PubMed.
-
Z. Zhao, J. Wang, H. Liu, J. Ye and Y. Chang, Proceedings of the 14th ACMSIGKDD international conference on Knowledge discovery and data mining, 2008, pp. 839–847.
-
J. Ye, K. Chen, T. Wu, J. Li, Z. Zhao, R. Patel, M. Bae, R. Janardan, H. Liu and G. Alexander, et al., Proceedings
of the 14th ACM SIGKDD international conference on Knowledge discovery and data mining, 2008, pp. 1025–1033.
-
S. Xiang, L. Yuan, W. Fan, Y. Wang, P. M. Thompson and J. Ye, Proceedings of the 19th ACM SIGKDD international conference on Knowledge discovery and data mining, 2013, pp. 185–193.
- K. Sriram, M. Rodriguez-Fernandez and F. J. Doyle III, PLoS Comput. Biol., 2012, 8, e1002379 CAS.
- G. Li, S. S. Nair and G. J. Quirk, J. Neurophysiol., 2009, 101, 1629 CrossRef PubMed.
- F. Vinther, M. Andersen and J. T. Ottesen, J. Math. Biol., 2011, 63, 663–690 CrossRef PubMed.
- R. Yehuda, S. M. Southwick, J. H. Krystal, D. Bremner, D. S. Charney and J. W. Mason, Am. J. Psychiatry, 1993, 150, 83 CrossRef CAS PubMed.
- R. Yang, B. J. Daigle Jr, S. Y. Muhie, R. Hammamieh, M. Jett, L. Petzold and F. J. Doyle, BMC Syst. Biol., 2013, 7, 1 CrossRef PubMed.
- R. Hammamieh, N. Chakraborty, T. Lima, J. Meyerhoff, A. Gautam, S. Muhie, P. D'Arpa, L. Lumley, E. Carroll and M. Jett, Behav. Brain Res., 2012, 1 Search PubMed.
- J. L. Kibler, J. Trauma Dissociation, 2009, 10, 135–150 CrossRef PubMed.
- R. von Känel, R. Hari, J. P. Schmid, L. Wiedemar, E. Guler, J. Barth, H. Saner, U. Schnyder and S. Begré, J. Cardiol., 2011, 58, 61–68 CrossRef PubMed.
- J.-H. Cho, I. Lee, R. Hammamieh, K. Wang, D. Baxter, K. Scherler, A. Etheridge, A. Kulchenko, A. Gautam and S. Muhie,
et al.
, Proc. Natl. Acad. Sci. U. S. A., 2014, 111, 3188–3193 CrossRef CAS PubMed.
- A. Ben-Dor, N. Friedman and Z. Yakhini, Technical Reports of the Leibniz Center, 2002, 1–26 Search PubMed.
- H. Cohen, N. Kozlovsky, C. Alona, M. A. Matar and Z. Joseph, Neuropharmacology, 2012, 62, 715–724 CrossRef CAS PubMed.
- D. C. Blanchard, G. Griebel and R. J. Blanchard, Prog. Neuro-Psychopharmacol. Biol. Psychiatry, 2003, 27, 1177–1185 CrossRef PubMed.
- R. Adamec, P. Kent, H. Anisman, T. Shallow and Z. Merali, Neurosci. Biobehav. Rev., 1998, 23, 301–318 CrossRef CAS.
- S. Yamamoto, S. Morinobu, S. Takei, M. Fuchikami, A. Matsuki, S. Yamawaki and I. Liberzon, Depression Anxiety, 2009, 26, 1110–1117 CrossRef PubMed.
- J. A. King, S. Abend and E. Edwards, Biol. Psychiatry, 2001, 50, 231–237 CrossRef CAS.
- A. M. Rasmusson, L. Shi and R. Duman, Neuropsychopharmacology, 2002, 27, 133–142 CrossRef CAS.
|
This journal is © The Royal Society of Chemistry 2015 |
Click here to see how this site uses Cookies. View our privacy policy here.