DOI:
10.1039/C5IB00083A
(Review Article)
Integr. Biol., 2015,
7, 846-858
Genome scale models of yeast: towards standardized evaluation and consistent omic integration
Received
23rd March 2015
, Accepted 9th June 2015
First published on 9th June 2015
Abstract
Genome scale models (GEMs) have enabled remarkable advances in systems biology, acting as functional databases of metabolism, and as scaffolds for the contextualization of high-throughput data. In the case of Saccharomyces cerevisiae (budding yeast), several GEMs have been published and are currently used for metabolic engineering and elucidating biological interactions. Here we review the history of yeast's GEMs, focusing on recent developments. We study how these models are typically evaluated, using both descriptive and predictive metrics. Additionally, we analyze the different ways in which all levels of omics data (from gene expression to flux) have been integrated in yeast GEMs. Relevant conclusions and current challenges for both GEM evaluation and omic integration are highlighted.
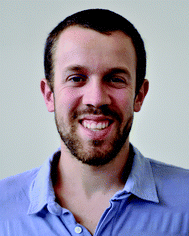
Benjamín J. Sánchez
| Benjamín Sánchez has an MSc degree (2014) in Biotechnology Engineering from the Pontifical Catholic University of Chile. As of 2014 he is a PhD student in the Division of Systems and Synthetic Biology in Chalmers University of Technology, Sweden. For his thesis, he is working on genome scale modeling (GEM) of yeast, developing new tools for integration of GEMs with omic data and for computational prediction of bio-based production. |
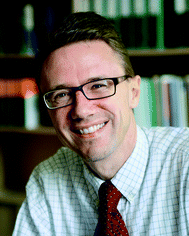
Jens Nielsen
| Jens Nielsen has an MSc degree in Chemical Engineering and a PhD degree (1989) in Biochemical Engineering from the Danish Technical University. In 2008 he was recruited as a full Professor in Chalmers University of Technology, Sweden, where he currently directs the Division of Systems and Synthetic Biology. His group is mainly focused on systems biology of yeast, for bio-based production of compounds of high value, such as proteins, fatty acids and biofuels. He is member of the Royal Danish Academy of Science, the National Academy of Engineering in USA, and the Royal Swedish Academy of Sciences. |
Insight, innovation, integration
A genome scale model (GEM) is a simple mathematical representation of a cell that models the interplay of all metabolites, reactions and genes for a given organism. GEMs have been used in systems biology since the beginning of the 2000s for understanding and predicting metabolic strategies. Using these models as computational scaffolds, different kinds of biological insights can be obtained when integrating one or more types of omics data. Here we review the history of Saccharomyces cerevisiae's GEMs and the current approaches for their evaluation and integration with omics data.
|
1. Introduction
Saccharomyces cerevisiae, also referred to as budding yeast, is undoubtedly the best studied eukaryal unicellular organism. It has been used to understand basic biochemical and biomolecular processes, as well as a model organism for studying human diseases.1 It is also an important cell factory in metabolic engineering, with successful applications in the biofuel,2,3 pharmaceutical4 and food5 industries. Understanding how yeast uses its metabolism for growth and production of compounds of interest is fundamental for its use in metabolic engineering. Considering the very large number of reactions involved in metabolism, a mathematical modeling approach becomes a necessity.
Modeling and predicting cellular metabolism has always been at the center of systems biology. Already in the 1960s, as the biochemical steps of the main metabolic pathways were being elucidated, kinetic models of metabolism were presented for mathematical simulation and analysis.6 Because these models required identifying a considerable amount of unknown rate laws and parameters, constraint based modeling was developed through the 1980s and 1990s, as a way of circumventing the unavailability of kinetic data.7 With the advent of whole-genome sequencing techniques and the exponential increase in computational performance, genome scale modeling was introduced at the beginning of the 2000s and until today is the most widely used modeling approach for studying metabolism.8
Genome scale models of metabolism, referred to as GEMs or sometimes as GSMMs, are usually reconstructed in a semi-automatized manner, and contain 3 main levels of information: metabolites, reactions and genes. The interplay between metabolites and reactions is represented by a stoichiometric matrix, and the interaction between reaction and genes with Boolean relationships. The main advantage of the approach is that it allows quantitative predictions of metabolism, such as the effect of genetic perturbations on the cell's physiology, with almost no parameters. However, as discussed elsewhere,6 the approach has several limitations, such as an assumption of a steady-state of metabolite concentrations and the difficulty of incorporating regulatory constraints.9 For studying yeast metabolism, several GEMs have been developed10,11 and applied extensively with satisfactory results.12–15
Here we review the genome scale modeling technique in yeast. We start by briefly surveying the S. cerevisiae's GEMs that have been published so far. We then focus on how GEMs of yeast typically are evaluated and tested, using both descriptive and predictive metrics, and how they can be integrated with omics data. Finally, challenges in the field are discussed.
2.
S. cerevisiae GEMs: an ongoing story
The history of yeast genome scale modeling, shown schematically in Fig. 1A, has been reviewed in depth previously.10,11 We will therefore review it briefly and focus on recent advances. The first genome scale model of yeast, iFF708, was published 12 years ago and considered three cellular compartments: the cytosol, mitochondria and the extracellular space.16 It was the first eukaryal GEM and also the basis for three subsequent yeast models: iND750, which increased the number of compartments to eight,17 iLL672, which improved the gene essentiality predictions,18 and iIN800, which included a more detailed lipid metabolism.19 iND750 was later updated to iMM904, achieving better gene essentiality predictions and integrating metabolomics data.20
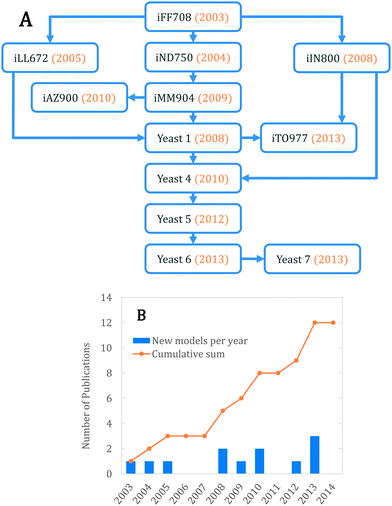 |
| Fig. 1 (A) Schematic history of GEMs in yeast. (B) Increase in the number of published yeast GEMs through the years. | |
Both iMM904 and iLL672 were used to generate Yeast 1, the first consensus genome scale network reconstruction (GENRE), created using a ‘jamboree’ approach where several yeast research groups collaborated.21 The network was expanded using iIN800 to improve lipid metabolism and connectivity, and was also modified to allow constraint based simulations, resulting in Yeast 4 (the first consensus GEM).22 Since then, it has been further improved 3 times, namely Yeast 5 (improved sphingolipid metabolism),23 Yeast 6 (refined coverage and improved anaerobic predictions)24 and Yeast 7 (enhanced fatty acid, glycerolipid and glycerophospholipid metabolism).25
Besides the consensus yeast models, there have been additional model developments from individual groups. In one study, iMM904 was further improved using a procedure to reconcile growth prediction inconsistencies; this resulted in iAZ900.26 Finally, the models iIN800 and Yeast 1 were recently merged to create iTO97727 with different criteria than Yeast 4, using less compartments and more unique reactions. Fig. 1B shows the number of models published by year. We expect that the number of yeast GEMs will continue to increase in the future, as more biological data gets published and more manual/automatic curations are performed.
3. GEM evaluation approaches
Each time a new GEM is published, it is evaluated to display whether it is more complete in coverage and/or has better prediction performance than previous models. These evaluation metrics can be divided into four general groups (Fig. 2): general metrics, connectivity metrics, growth metrics and genetic deletion metrics. The former two are metrics for describing the model (both quantitatively and qualitatively), and the latter two are metrics for predicting biological behavior. In the following we review in depth each category, highlighting which yeast studies have included which of them.
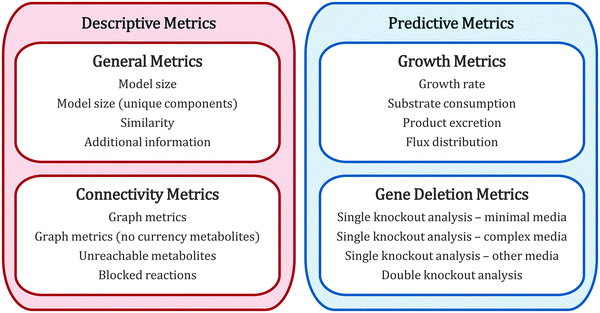 |
| Fig. 2 Main metrics used to evaluate GEMs. | |
3.1. General metrics
The most basic descriptive evaluation of a GEM is to count the total amount of reactions, metabolites, genes and compartments it has. This has been done for all reviewed yeast models;16–27 a summary of all metrics is displayed in Fig. 3. Over the years, the amount of reactions, metabolites and compartments has increased, but the amount of genes remains relatively similar, indicating that even though most of the metabolic genes are included in the model, our understanding of metabolism is still at a developing stage. It has been argued that the same metabolite in different compartments should be counted only once, in order to make a fair assessment of size.27 The amount of unique reactions and unique metabolites are therefore also used as metrics for comparison of GEMs.
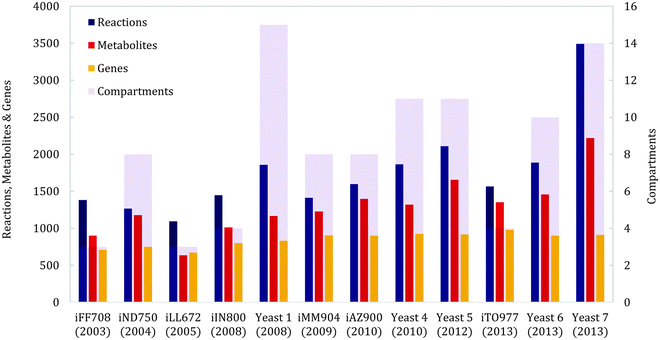 |
| Fig. 3 Number of reactions, metabolites, genes and compartments in all 12 published yeast GEMs. | |
Going further than merely counting, a useful general metric scarcely used so far is comparing the similarity or heterogeneity between models, with respect to metabolites, reactions or genes. For this, a reference set including the union of all elements (metabolites, reactions or genes) must be created. Then, binary vectors can be computed for each model (in which the i-th position will be = 1 if the i-th element is present in the model and = 0 otherwise), and distance scores can be computed between models.28,29 Using this technique, it has been recently shown that yeast models fall into “clusters” depending on their historical development and research group origin.29 However, the lack of standardized metabolite names between models can severely hamper these analyses (an issue further discussed later).
There are additional qualitative general metrics for assessing and comparing models: if additional metabolic pathways are included,19,25 if there is a distinction between GENRE and GEM,23 if standardized identifiers are used,21 or if additional levels of data are included.27
3.2. Connectivity metrics
A central part of any genome scale model is the metabolic network, which can be simplified as an undirected bipartite graph, connecting metabolites with reactions. Here we refer to this as the metabolic graph. In it, a metabolite Mi will have an edge to reaction Rj if Mi participates in reaction Rj, either as a substrate or a product. This is presented for an example network in Fig. 4. The metabolic graph can be split into two smaller graphs: the metabolite graph, in which the nodes are metabolites and there is a connection between two metabolites if they are present in the same reaction, and the reaction graph, in which the nodes are reactions and there is a connection between two reactions if they both share a metabolite (also schematically shown in Fig. 4).30
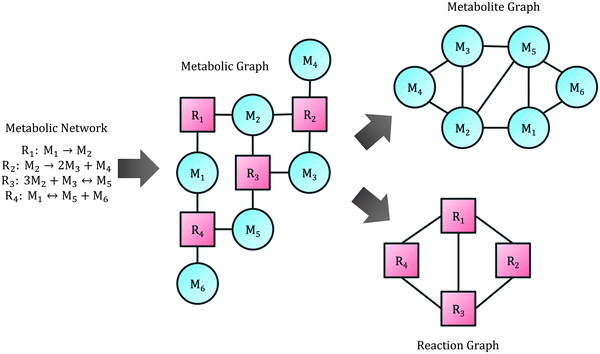 |
| Fig. 4 Metabolic, reaction and metabolite graphs for a fictional metabolic network. | |
Of the three mentioned graphs, the metabolite graph has been the most studied in yeast GEMs.21,31–33 As any undirected graph, several connectivity metrics can be computed, which give insights about the network topological properties. The most common descriptive calculations used are:
• Metrics for any pair of nodes (Mi, Mj):
– Shortest path: the smallest number of intermediate nodes between Mi and Mj, typically calculated with a shortest path algorithm such as Dijkstra's algorithm.
– Shortest path diversity: the amount of paths between Mi and Mj of length equal to the shortest path. Used for measuring robustness in the network.
• Metrics for any node Mi:
– Node degree: the amount of nodes connected to Mi. Used for finding the best connected metabolites.
– Local clustering coefficient: the fraction of connected nodes between the neighbors of Mi, without considering Mi. Used for finding local clusters.
– Betweenness centrality: the average fraction of times Mi is present in the shortest path between any other couple of nodes. Used for finding hubs in the network.
• Global metrics:
– Global clustering coefficient: the fraction of closed triplets among all triplets, where a triplet consists of 3 nodes connected by at least 2 connections, and a closed triplet is a triplet with all 3 connections. Used for measuring the cluster-like behavior of the total network.
– Characteristic path length: the average shortest path.
– Network diameter: the maximum shortest path.
– Averages for other previous metrics (node degree, local clustering coefficient, etc.) can also be computed.
Usually these metrics are computed with and/or without currency metabolites (H2O, H+, CO2, phosphate, diphosphate, ammonium, ATP, ADP, AMP, NAD+, NADH, NADP+ and NADPH).21,30 Software such as Network Analyzer34 or cyto-Hubba35 can calculate most of these metrics. Although this analyses can be extended to the reaction – gene network of GEMs, usually protein–gene networks are studied instead, which are typically attained from other sources.36,37
Two additional descriptive metrics, specific for metabolic networks, have been used in yeast models regularly. One is the percentage of unreachable metabolites from the extracellular space, which is a good metric to detect closed sub-networks within the model, i.e. a set of reactions and metabolites that are disconnected from the rest of the network.22 It is easily calculated using the metabolite graph with no currency metabolites, by searching for the shortest path between each intracellular metabolite and each extracellular metabolite. The other descriptive metric is the percentage of blocked reactions, which is relevant for detecting the part of the network that will always have zero flux due to dead-end metabolites (metabolites that are not consumed).22,23,25 It is usually checked using a flux variability analysis (FVA)38 approach, allowing exchange for all extracellular substrates, and maximizing/minimizing each reaction of the model.
3.3. Growth metrics
One of the main uses of GEMs is for model predictions. The most basic prediction a GEM can give, using the flux balance (FBA) approach,39 is the specific growth rate of an organism, together with the uptake and excretion rates of substrates and products, respectively. Therefore, the validation of the specific growth rate and other exchange fluxes with experimental data has for several yeast models been a standard evaluation procedure.16,19,20,23,27 Chemostat data is regularly used for this, under carbon (usually glucose) or nitrogen (usually ammonium) limitation, aerobic or anaerobic conditions, and varying specific growth rate. As prediction performance improves, it could be of interest to expand the evaluation of yeast GEM predictions to complex media, or other carbon (ethanol, glycerol, galactose, fructose, etc.) or nitrogen (amino acids) sources.
When predicting the growth rate, in order to correctly account for the energy consumption for all cellular processes an ATP requirement value has to be introduced, commonly known as ATP maintenance. This maintenance can be divided in a growth-associated maintenance (GAM; mmol(ATP) per gDW) and a non-growth counterpart (NGAM; mmol(ATP) per gDW per h). Both are usually obtained from experimental measurements, and the former one is typically adjusted to improve the fitting to experimental data.16,40
An additional growth metric is to evaluate flux distributions inside the network. This is rarely done as a model evaluation metric in yeast,19,27 but can give insights into how the carbon and or nitrogen are being consumed, and how the simulated cell is obtaining its energy, both at aerobic and anaerobic conditions. Typical studied paths are glycolysis, pyruvate metabolism, tricarboxylic acid (TCA) cycle, pentose phosphate pathway (PPP) and the fermentative pathway.19
3.4. Gene deletion metrics
Another predictive metric, so far tested in all yeast GEMs,17–20,22–27,29,41 is the ability to reproduce experimental gene deletion data.42 The most common approach for this is to perform a single knockout analysis, with the goal of correctly predicting essential genes, i.e. genes for which no growth is observed when removed from the model. This has been done using experimental data of yeast growing on minimal glucose-limited media,17–20,22–27,29 complex glucose-limited media (usually YPD),17–19,26,27,29,41 and/or non-glucose limited media (such as ethanol, galactose and glycerol).17–20,29 Recently, there have been studies that also include double knockout analysis, with the aim of detecting synthetic-lethal genes, i.e. pairs of genes that are not essential by themselves, but when both of them are deleted, the cell does not grow.27,29
The computational procedure is the same for all of the abovementioned approaches: first the wild type strain is simulated using FBA and maximizing biomass,39 and the specific growth rate is saved as a reference. Then, for each gene (or for each pair of genes in the double knockout analysis), the knockout is computed by fixing the flux through all reactions controlled by the corresponding gene(s) to zero. A second optimization is then performed, by maximizing biomass, minimizing the metabolic adjustment (MOMA)43 or using regulatory on/off minimization (ROOM).44 After repeating the procedure for all genes, a growth threshold is used to define if each knockout is able to grow or not. This is later compared with experimental data, and metrics that account for global performance of the model such as sensitivity (also known as recall; the fraction of correct viable predictions), specificity (the fraction of correct inviable predictions), accuracy (the fraction of correct predictions), Matthew's correlation coefficient (MCC) or geometric mean (GM) can be computed.
For gene essentiality analysis there are three main factors that should be considered: the threshold for considering a knockout viable, the experimental dataset used and the simulated media composition. The growth threshold is the minimum specific growth rate required for a knockout to be considered viable and therefore the gene non-essential. Some studies have used small thresholds, such as 1 × 10−6 1 h−1
23–25,29 or 1% of the wild type growth.26 These thresholds will increase the sensitivity but decrease the specificity, as a higher proportion of knockouts will be categorized as viable. Other studies have used higher thresholds, such as 80% of the wild type growth27 or one standard deviation below the mean of all knockout values.17 In turn, for these cases the sensitivity will decrease and the specificity will increase. Because the choice of threshold is always an arbitrary decision, it is recommended to study how the results vary depending on the choice of threshold.20,27
Choice of the experimental dataset used for model evaluation is also an important issue, especially since results from whole-genome knockout analysis are published regularly. Among all published yeast GEMs there is no consensus on dataset usage, and most of the times two or more datasets are employed. For gene essentiality, the most used approaches so far have been mining and querying various versions of the Saccharomyces Genome Deletion Project45–47 and the Saccharomyces Genome Database (SGD).48,49 For gene synthetic lethality, a study that constructed all possible double knockouts for 1712 genes50 and also the SGD database have been used. It is important to use the same dataset when comparing models,29 otherwise predictive metrics (sensitivity, specificity, MCC, etc.) between models will not be comparable. Ideally, the dataset should have similar experimental conditions; in this sense, studies that have developed their own gene deletion datasets for genome-scale model validation51,52 present important advantages.
Finally, it is important to note that the composition of the simulated medium has a major influence on the results of the deletion analysis.17,29 When using minimal media, the carbon source is typically fixed at a limiting rate, oxygen is supplied at a small amount (but not limiting) and ammonium, sulfate, and phosphate are supplied in unlimited amounts.17,19,20,22–27,29,41 Sometimes, the simulation allows for vitamins20,22,26 and metabolites associated with auxotrophic markers17,20,22,26 consumption as well. In the latter case, the associated marker genes are deleted from the model as well. When using complex media on the other hand, the regular practice is to additionally include a supply of all 20 amino acids and 4 nucleotide bases.17,19,26,27,41 In some simulations trehalose is added as well.17 One key challenge, especially when comparing models, is to use a standardized medium, and to have consistency between the medium and the experimental dataset, something that is not always done correctly. Finally, recent studies suggest that there is no yeast GEM which is better than the others in predicting gene essentiality in all media, all datasets and under all metrics; each one will perform different depending on the conditions employed.29,51
4. Integration of omics data into GEMs
There are many studies on the use of genome scale models as scaffolds for integrative analysis of omics data.8 This is especially beneficial considering that the constraint based approach involves a large degree of freedom in the feasible flux space.39 Consequently, by integrating omics data into GEMs it is possible to get improved predictions of cellular phenotypes and gain new insight into regulation of metabolism in response to environmental or genetic perturbations.11,53 Several approaches exist for doing this, depending on the type of omics data.7,54,55 In the following, we will review yeast GEM studies that have included: gene expression (transcriptomics), gene–gene, protein–gene and protein–protein interactions (interactomics), protein level (proteomics), reaction's physicochemical (thermodynamics) and biochemical (kinetics) parameters, metabolite levels (metabolomics) and reaction fluxes (fluxomics). Fig. 5A shows all 86 cases found in literature for yeast, distinguishing in each case what type of omics data was used. Correspondently, Fig. 5B shows the number of integration studies per year, suggesting an exponential-like increase of studies over time, and Fig. 5C displays a break-down in use of different types of omics data.
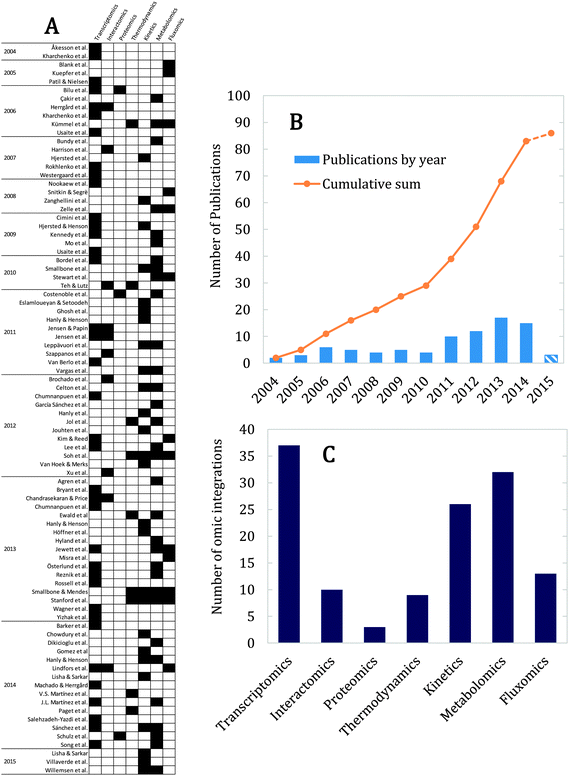 |
| Fig. 5 Integration of omics data in yeast GEMs. (A) Table showing all integrations studies found in literature, indicating which kinds of omics data were used. (B) Integration studies per year. (C) Integration studies classified by type of omics data. If a study used more than one kind of omics data, it is counted more than once as well. | |
4.1. Transcriptomics
Transcriptome data, in form of microarray data or RNAseq data, is the most widely used omics data for integration with GEMs (Fig. 5C),56 most likely due to the relative easiness of generating these data and the fact that they are genome-wide in contrary to most other omics data.57 The basic idea underlying the use of transcriptome data is that there is a relationship between the expression level of a gene and the flux that the corresponding enzyme catalyzes. Transcriptome data are relatively easy to integrate in GEMs thanks to the standardized annotation of genes in the models, using the MIPS codes. Its first use in a yeast GEM was more than 10 years ago and consisted of blocking fluxes of the model that were controlled by genes with no expression under a given threshold.58 Because of the simplicity of this approach for integration with FBA, other studies have use it59,60 and additional methods have been developed from it.61,62
Instead of using an expression threshold for on/off fluxes, other studies have found correlations between flux values and gene expression. For instance, combining the random sampling method63 with transcriptomics has been used to find correlations between flux and mRNA levels,64,65 and to identify the so-called transcriptionally controlled reactions (reactions with high flux and corresponding high gene expression) in yeast.27,66,67 Other correlation studies have used traditional FBA, e.g. with linear programming, instead of random sampling.68
Other studies included different sets of conditions to study the metabolic adjustment by differential expression,69 accounted for up/down constraints depending on expression levels,70,71 studied relative flux changes compared to a reference distribution,72 used the expression data in the objective function (i.e. maximizing consistency between fluxes and expression data),73–75 integrated gene expression and GEMs with regulatory networks,69,76–78 analyzed the shadow prices in FBA79 of metabolites80 and genes,81 transformed the metabolic state via perturbations82 and included enzyme complex abundance.83 Recently, some of these approaches were compared under the same conditions in yeast, showing that none of them outperforms the rest under all conditions.84 Therefore more than one method should always be tested when using gene expression in FBA calculations.
Another popular approach for transcriptome integration has been to use the network topology instead of FBA, inferring reporter metabolites (metabolites that are present in several highly-expressed reactions) from the metabolic graph, and highly correlated subnetworks from the reaction graph (Fig. 4). This was formulated for the first time for yeast,85 and since then it has been extended to the broader concept “reporter feature”86 and used numerous times.19,67,87–92 Other gene-expression approaches using network topology have also been developed, using the metabolic graph,93,94 the reaction graph71,95 and the gene graph (in which the nodes are genes and there is a connection between two genes if they control the same reaction).96,97
A main disadvantage of using transcriptome data is that there are many intermediate biological processes between gene expression and metabolic fluxes, sometimes leading to low correlation between the transcriptome and the biological functions.57 To improve this, additional levels of information have been combined with transcriptome data in a number of studies, such as proteomics,64 metabolomics27,68,73,75,80,94 and fluxomics.66,72,94,95
4.2. Interactomics
Experimental measurements of interactions have been used in genome scale modeling of yeast for understanding, from an omics perspective, the positive or negative relationships between yeast genes and/or proteins. These approaches can be classified into two main groups: regulatory mechanisms (protein–gene or protein–protein) and epistasis (gene–gene). A pioneer study developed an integrated model of metabolism and transcriptional regulation, consisting of a GEM and a transcriptionally regulatory network (TRN).76 The study combined different databases sources, ChIP-chip data and transcription-factor binding site motifs for the regulatory network construction. The model showed good performance in predicting growth under different transcription factor deletions and carbon sources. TRNs have been also used in a thermodynamic analysis of yeast's anaerobic fermentation process,98 and for further refinement in transcriptome integration methods.69,77,78 A different study merged a GEM with a protein–protein and kinase phosphatase interaction network to construct an expanded reaction graph, for correlating fluxes to mRNA levels.95
Genome scale modeling has been used to predict epistasis, i.e. positive or negative correlations between genes, performing double knockout analysis and using the knockout's specific growth rates for computing epistasis scores.99–101 However, initial studies lacked experimental verification, due to the combinatorial problem of generating all double knockouts in vitro. This was first overcome by extracting experimental data from different sources in the literature102 and later by generating and using large datasets of genetic interactions.103,104 More recently, an alternative approach, which optimizes metabolite balances instead of fluxes, was used for predicting genetic interactions.105 These studies revealed a clearer picture of the interactions in the metabolic network and have given insights into yeast metabolic network's plasticity,102 modularity103 and allele-specificity.104
4.3. Proteomics
Proteomics, measured in relative or absolute abundance, is the least included omics data in yeast GEMs (Fig. 5C), which is likely because of the difficulty of acquiring good quality data, but also given that enzymes are the least well annotated component in GEMs; only a fraction of the EC numbers are present, and high-order relationships between enzymes and reactions (such as complexes, isozymes or enzyme promiscuity) are often incomplete or non-existent.
All of the reviewed proteomic studies have compared the protein levels with other types of data to find correlations between different conditions. One study correlated proteomics with transcriptomics and metabolic fluxes obtained from random sampling to explain expression conservation in certain genes.64 A second study related metabolic predictions (constrained by extracellular metabolite measurements) with proteome data for detecting “non-necessary proteins” (i.e. metabolic enzymes that are present under a given condition but no flux is being predicted to go through its corresponding reaction).106 Finally, a third study used phosphoproteomics, metabolomics and the pairwise distance between enzymes and metabolites (using the metabolic graph) to suggest the function of enzymatic phosphorylation levels.107 Clearly, proteomics can answer important questions regarding yeast metabolism, and should be further explored in the future.
4.4. Thermodynamics
Integrating thermodynamics data with GEMs is extremely useful since it reveals which reactions are likely to occur and in which direction. It is also a convenient way of relating metabolomic data with model predicted fluxes. Although all yeast models have included thermodynamics in the model reconstruction to some extent, as it has to be specified for each reaction whether it is reversible or not, there are some studies that have developed methods for further improving the model's thermodynamic consistency. A highly used approach in yeast has been network-embedded thermodynamic (NET) analysis, which reconciles GEM predictions with Gibbs energies of formation, using fluxomic and metabolomic data.108 This approach allows consistency checks on the measured metabolomics data, prediction of metabolite concentrations in the case of missing data, and detection of reactions subject to allosteric or genetic regulation. NET has been published as software109 and it has been used to analyze metabolome differences between yeast knockouts grown under different carbon sources.110 Recently it was expanded to analyze elementary flux modes,111 and to infer reaction directionalities from metabolomic and thermodynamic data.112
Other thermodynamic approaches have also been presented. To determine growth efficiency in anaerobic fermentation, the general thermodynamic balance was analyzed connected to a FBA problem, instead of considering the thermodynamics of each reaction.98 Also, most of the kinetic modeling approaches (reviewed in detail in the following section) use thermodynamic data, such as the equilibrium constant for defining modular rate laws,113,114 or the Gibbs energies of formation for sampling metabolite concentrations.115 Finally, a recent study estimated the Gibbs energy of all GEM reactions at varying temperatures, in order to detect “cold-favoring” reactions for generating a cold-tolerant yeast mutant.116
4.5. Kinetics
Another vastly used approach in yeast genome scale modeling has been to include kinetic parameters (regarding the rate and saturation) of enzymes in the models. This has been done up to now using mainly two techniques: dynamic flux balance analysis59,60,117–132 and kinetic modeling.113–115,133–135
Dynamic flux balance analysis (dFBA) is a simple kinetic approach that uses the assumption that the intracellular kinetics can be disregarded (given that they are much faster than the extracellular ones),136 and therefore iterative FBA problems can be solved for a dynamic simulation of the organism. The main advantage of the approach is that it uses minimal kinetic data (most of the times the consumption and/or production kinetics of extracellular metabolites are sufficient). In yeast, dFBA has been used on GEMs to predict ethanol production,117,118 triacylglycerol metabolism,120 cofactor balance in pentose utilization,119 wine fermentation performance123 and the shift from respirative to fermentative metabolism.125 Some studies have assessed the influence of different parameter values in the overall predictions compared to experimental data.59,60,122 In the last years, numerous studies have used dFBA to simulate microbial co-cultures of S. cerevisiae with other organisms, such as E. coli,121,124,127,130,131S. stipitis126,129 and C. reinhardtii.128 Some of the presented dFBA studies have included metabolomics data, usually from the extracellular media.60,122,123,129 Recently, an extension to dFBA has been developed that accounts for intracellular metabolomics integration as well (METdFBA).132
Differing from the simplified approach of dFBA, kinetic models have emerged that account for all kinetics outside and inside the cell. Although the idea of kinetic modeling of metabolism has been around for some time,137,138 it was just recently that the first genome scale model of yeast was adapted for this purpose,134 using linlog kinetics and metabolic control analysis (MCA).139 This was later expanded to include modular rate laws113 as well as thermodynamic and fluxomic data.114 An alternative method has been presented,115 that uses uncertainty and risk analysis methods (ORACLE)140 for missing metabolomics or kinetic data. Another kinetic yeast model was developed with the objective of allowing easier and systematic comparison between parameter estimation methods.135 The main advantage of kinetic modeling is that it gives additional insights to the metabolic network, such as bottle-necks and controlling reactions. However, the scarcity of data, and in particular on kinetic parameters,6 represents a significant drawback. Additionally, most of the studies simplify the GEM to a fraction of the complete metabolic network, and include measures for only a small part of the total number of metabolites, thereby assigning estimates for most of them. To overcome these problems, a new method has been proposed that instead of estimating missing kinetic or metabolomic data, operates with the available data only for further constraining the FBA simulation (k-OptForce).133
Two additional approaches that also include kinetics have been tried out in yeast. FBA with molecular crowding (FBAwMC),141 which limits the amount of protein available to perform metabolic activities, has been performed using a yeast GEM.142 It used random values of enzymatic turnover rates derived from a typical distribution to better understand the overflow metabolism of yeast at high specific growth rates (known as the Crabtree effect). Finally, an alternative approach to dFBA was recently developed, namely dynamic mass balance analysis (dMBA), which computes a distribution of metabolite masses instead of fluxes, simplifying integration of metabolomics data.143
4.6. Metabolomics
There are many studies on integrating metabolite concentration measurements with yeast GEMs. The most typical approach is to use extracellular measurements (generally under chemostat conditions) to infer exchange fluxes used for FBA simulation, either as sole data20,144,145 or in combination with other data types.27,66–68,106,146 Intracellular metabolite concentration, on the other hand, have been used far less. They are difficult to integrate with FBA as this simulation approach does not consider the actual metabolite levels. However, intracellular metabolite data have been used together with the metabolic graph (Fig. 4) for identification of reporter reactions, which are reactions that are likely to carry flux changes in response to environmental or genetic perturbations.147 Intracellular metabolomics data has been used together with flux coupling analysis148 as well, to classify and understand metabolite profiles of mutant strains.149 Finally, metabolomics data has been used as well as a calibration/validation metric for both static73,75,150–152 and dynamic60,122,123,129 models, and as input in kinetic modeling113–115,133,134 and other of the already reviewed methods.80,94,107,108,110,111,132,143
4.7. Fluxomics
Fluxomics is recurrently used in genome scale modeling as it is easy to integrate with FBA modeling. Intracellular fluxes can be quantified using 13C-based flux analysis, a very widespread technique, albeit experimentally challenging, reviewed in depth elsewhere.153 This approach uses information about the labeling distribution in intracellular metabolites to quantify flux ratios at key branch points in the metabolism, and combined with a simple (or complete GEM) it is possible to quantify the absolute fluxes through the different parts of the metabolic network. Typically the data allows for estimation of an average flux value, with corresponding standard deviations, for a limited amount of reactions inside the model, usually the central carbon metabolism and amino acid biosynthesis pathways, in units of mmol (gDW h)−1. These estimated fluxes can be then used to constrain the FBA simulations. This approach improves considerably the model predictions, given that it reduces the otherwise large degrees of freedom in the system, and has been used in several yeast studies constraining fluxes to experimental values.18,95,145,146,154,155 Alternatively, the flux measurements can be used as part of the objective function (minimizing the absolute differences between the predicted and measures fluxes)72 or for later validation of the model predictions.94,156 Finally, several yeast studies already mentioned have used fluxomic measurements as general inputs for their procedures.108,113–115
5. Current challenges
12 years of genome scale modeling of yeast have contributed significantly to our understanding of yeast's cellular processes and life functions, and have improved strain performance through model guided metabolic engineering.6 As new model expansions (improving coverage and accuracy) and new approaches for omics data integration (improving specificity and applicability) appear, our understanding will grow even further. However, important challenges remain in the field, both in the area of model development/evaluation and data integration.
One challenge in yeast GEMs is the need of standardized evaluation and comparison. E. coli and S. cerevisiae are the only two unicellular organisms for which several iterated versions of GEMs exist,53 and therefore a crucial step when modeling these organisms is the right choice of GEM. It has been frequently discussed that there is a trade-off in GEMs between completeness and prediction accuracy;22,23 usually models that contain the most coverage (metabolites, reactions) have lower performance under prediction metrics. No model is suited for all purposes; larger models should be used for studying particular pathways that are not present in other models, whereas smaller models may be better for predicting growth phenotypes of knockout strains. Consequently, there is need of benchmark tests that can evaluate the performance of new models and compare results among existing models, in order to identify the best model for a particular purpose. Recent studies that evaluate yeast models under the same gene deletion metrics are the first examples of this.29,52
Another critical aspect for GEM evaluation is the standardization of the chemical species involved, to avoid recurrent annotation problems with dissimilar nomenclature.157,158 For achieving this, several standard identifiers for enzymes (EC numbers, PMID and KEGG36 codes), metabolites (SMILES,159 ChEBI160 and InChI161 identifiers) and genes (MIPS162 and SGD49 codes) have been used, albeit scarcely. An important challenge is to maintain a continuous update and curation of the models using these unified nomenclatures in the yeast GEMs. This will decrease the difficulty of model development, and increase the quality of model comparisons.29
We have detected areas for improvement for omic integration. Fig. 5C pinpoints omics data types that have not been sufficiently studied in context of yeast GEMs, such as regulation, thermodynamics and especially proteomics. Nonetheless, the biggest challenge is not to focus on only one level of data but to use the GEM as a scaffold for integration of more than one or two levels, which has only been tried in a handful of studies, and using subsets or simplifications of the full GEM (Fig. 5A). Further considerations must be taken into account when performing this type of studies, especially regarding the data; all datasets should ideally come from the same source (similar media, strain, condition, etc.). In this sense, experimental studies that measure several layers of data are especially useful.163 However, even more important is the constant crosstalk between experimental and computational biologists, in order to on one side generate useful data in statistically significant experimental setups, and in the other one answer relevant biological questions.
Appropriately addressing the mentioned issues in model evaluation and data integration will enable researchers to construct a full picture of yeast metabolism, with all the “key players” correctly considered, and ultimately enhance predictions for applications in systems biology and metabolic engineering.
Acknowledgements
The authors would like to thank Avlant Nilsson, Petri-Jaan Lahtvee and the anonymous reviewers for providing valuable feedback on the final manuscript. This work has been financially supported by the Novo Nordisk Foundation and the Knut and Alice Wallenberg Foundation. B.J.S. gratefully acknowledges financial support from CONICYT.
References
- D. Botstein, S. A. Chervitz and J. M. Cherry, Science, 1997, 277, 1259–1260 CrossRef CAS.
- E. J. Steen, R. Chan, N. Prasad, S. Myers, C. J. Petzold, A. Redding, M. Ouellet and J. D. Keasling, Microb. Cell Fact., 2008, 7, 36 CrossRef PubMed.
- J. Zaldivar, J. Nielsen and L. Olsson, Appl. Microbiol. Biotechnol., 2001, 56, 17–34 CrossRef CAS.
- D. K. Ro, E. M. Paradise, M. Ouellet, K. J. Fisher, K. L. Newman, J. M. Ndungu, K. A. Ho, R. A. Eachus, T. S. Ham, J. Kirby, M. C. Y. Chang, S. T. Withers, Y. Shiba, R. Sarpong and J. D. Keasling, Nature, 2006, 440, 940–943 CrossRef CAS PubMed.
- S. Yamano, T. Ishii, M. Nakagawa, H. Ikenaga and N. Misawa, Biosci., Biotechnol., Biochem., 1994, 58, 1112–1114 CrossRef CAS.
- E. J. Kerkhoven, P. J. Lahtvee and J. Nielsen, FEMS Yeast Res., 2014, 15, 1–13 Search PubMed.
- A. Bordbar, J. M. Monk, Z. A. King and B. Ø. Palsson, Nat. Rev. Genet., 2014, 15, 107–120 CrossRef CAS PubMed.
- M. A. Oberhardt, B. Ø. Palsson and J. A. Papin, Mol. Syst. Biol., 2009, 5, 320 CrossRef PubMed.
- G. Liu, A. Marras and J. Nielsen, Quant. Biol., 2014, 2, 30–46 CrossRef CAS PubMed.
- I. Nookaew, R. Olivares-Hernández, S. Bhumiratana and J. Nielsen, Methods Mol. Biol., 2011, 759, 445–463 CAS.
- T. Österlund, I. Nookaew and J. Nielsen, Biotechnol. Adv., 2012, 30, 979–988 CrossRef PubMed.
- C. Bro, B. Regenberg, J. Förster and J. Nielsen, Metab. Eng., 2006, 8, 102–111 CrossRef CAS PubMed.
- D. Byrne, A. Dumitriu and D. Segrè, BMC Syst. Biol., 2012, 6, 127 CrossRef CAS PubMed.
- B. K. S. Chung, M. Lakshmanan, M. Klement, B. Mohanty and D. Y. Lee, Chem. Eng. Sci., 2013, 103, 100–108 CrossRef CAS PubMed.
- C. Y. Ng, M.-Y. Jung, J. Lee and M.-K. Oh, Microb. Cell Fact., 2012, 11, 68 CrossRef CAS PubMed.
- J. Förster, I. Famili, B. Ø. Palsson and J. Nielsen, Genome Res., 2003, 244–253 CrossRef PubMed.
- N. C. Duarte, M. J. Herrgård and B. Ø. Palsson, Genome Res., 2004, 14, 1298–1309 CrossRef CAS PubMed.
- L. Kuepfer, U. Sauer and L. M. Blank, Genome Res., 2005, 15, 1421–1430 CrossRef CAS PubMed.
- I. Nookaew, M. C. Jewett, A. Meechai, C. Thammarongtham, K. Laoteng, S. Cheevadhanarak, J. Nielsen and S. Bhumiratana, BMC Syst. Biol., 2008, 2, 71 CrossRef PubMed.
- M. L. Mo, B. Ø. Palsson and M. J. Herrgård, BMC Syst. Biol., 2009, 3, 37 CrossRef PubMed.
- M. J. Herrgård, N. Swainston, P. D. Dobson, W. B. Dunn, K. Y. Arga, M. Arvas, N. Blüthgen, S. Borger, R. Costenoble, M. Heinemann, M. Hucka, N. Le Novère, P. Li, W. Liebermeister, M. L. Mo, A. P. Oliveira, D. Petranovic, S. Pettifer, E. Simeonidis, K. Smallbone, I. Spasić, D. Weichart, R. Brent, D. S. Broomhead, H. V Westerhoff, B. Kirdar, M. Penttilä, E. Klipp, B. Ø. Palsson, U. Sauer, S. G. Oliver, P. Mendes, J. Nielsen and D. B. Kell, Nat. Biotechnol., 2008, 26, 1155–1160 CrossRef PubMed.
- P. D. Dobson, K. Smallbone, D. Jameson, E. Simeonidis, K. Lanthaler, P. Pir, C. Lu, N. Swainston, W. B. Dunn, P. Fisher, D. Hull, M. Brown, O. Oshota, N. J. Stanford, D. B. Kell, R. D. King, S. G. Oliver, R. D. Stevens and P. Mendes, BMC Syst. Biol., 2010, 4, 145 CrossRef PubMed.
- B. D. Heavner, K. Smallbone, B. Barker, P. Mendes and L. P. Walker, BMC Syst. Biol., 2012, 6, 1 CrossRef PubMed.
- B. D. Heavner, K. Smallbone, N. D. Price and L. P. Walker, Database, 2013, 2013, bat059 CrossRef PubMed.
- H. W. Aung, S. A. Henry and L. P. Walker, Ind. Biotechnol., 2013, 9, 215–228 CrossRef CAS PubMed.
- A. R. Zomorrodi and C. D. Maranas, BMC Syst. Biol., 2010, 4, 178 CrossRef PubMed.
- T. Österlund, I. Nookaew, S. Bordel and J. Nielsen, BMC Syst. Biol., 2013, 7, 36 CrossRef PubMed.
- P. Ghaffari, A. Mardinoglu, A. Asplund, S. Shoaie, C. Kampf, M. Uhlen and J. Nielsen, Sci. Rep., 2015, 5, 8183 CrossRef CAS PubMed.
-
B. D. Heavner and N. D. Price, in preparation.
- A. Wagner and D. A. Fell, Proc. Biol. Sci., 2001, 268, 1803–1810 CrossRef CAS PubMed.
- G. Del Rio, D. Koschützki and G. Coello, BMC Syst. Biol., 2009, 3, 102 CrossRef PubMed.
-
T. Rubina, M. Mednis and E. Stalidzans, CINTI 2013 - 14th IEEE Int. Symp. Comput. Intell. Informatics, Proc., 2013, 411–418.
- D. Vitkup, P. Kharchenko and A. Wagner, Genome Biol., 2006, 7, R39 CrossRef PubMed.
- Y. Assenov, F. Ramírez, S. E. Schelhorn, T. Lengauer and M. Albrecht, Bioinformatics, 2008, 24, 282–284 CrossRef CAS PubMed.
- C. H. Chin, S. H. Chen, H. H. Wu, C. W. Ho, M. T. Ko and C. Y. Lin, BMC Syst. Biol., 2014, 8, S11 CrossRef PubMed.
- M. Kanehisa, S. Goto, Y. Sato, M. Kawashima, M. Furumichi and M. Tanabe, Nucleic Acids Res., 2014, 42, 199–205 CrossRef PubMed.
- R. Caspi, T. Altman, R. Billington, K. Dreher, H. Foerster, C. A. Fulcher, T. A. Holland, I. M. Keseler, A. Kothari, A. Kubo, M. Krummenacker, M. Latendresse, L. A. Mueller, Q. Ong, S. Paley, P. Subhraveti, D. S. Weaver, D. Weerasinghe, P. Zhang and P. D. Karp, Nucleic Acids Res., 2014, 42, 459–471 CrossRef PubMed.
- R. Mahadevan and C. H. Schilling, Metab. Eng., 2003, 5, 264–276 CrossRef CAS PubMed.
- J. D. Orth, I. Thiele and B. Ø. Palsson, Nat. Biotechnol., 2010, 28, 245–248 CrossRef CAS PubMed.
- A. Varma and B. Ø. Palsson, Appl. Environ. Microbiol., 1994, 60, 3724–3731 CAS.
- J. Förster, I. Famili, B. Ø. Palsson and J. Nielsen, OMICS, 2003, 7, 193–202 CrossRef.
- G. Basler, Methods Mol. Biol., 2015, 1279, 183–204 Search PubMed.
- D. Segrè, D. Vitkup and G. M. Church, Proc. Natl. Acad. Sci. U. S. A., 2002, 99, 15112–15117 CrossRef PubMed.
- T. Shlomi, O. Berkman and E. Ruppin, Proc. Natl. Acad. Sci. U. S. A., 2005, 102, 7695–7700 CrossRef CAS PubMed.
- G. Giaever, A. M. Chu, L. Ni, C. Connelly, L. Riles, S. Véronneau, S. W. Dow, A. Lucau-Danila, K. Anderson, B. André, A. P. Arkin, A. Astromoff, M. El Bakkoury, R. Bangham, R. Benito, S. Brachat, S. Campanaro, M. Curtiss, K. Davis, A. M. Deutschbauer, K.-D. Entian, P. Flaherty, F. Foury, D. J. Garfinkel, M. Gerstein, D. Gotte, U. Güldener, J. H. Hegemann, S. Hempel, Z. S. Herman, D. F. Jaramillo, D. E. Kelly, S. L. Kelly, P. Kötter, D. LaBonte, D. C. Lamb, N. Lan, H. Liang, H. Liao, L. Liu, C. Luo, M. Lussier, R. Mao, P. Menard, S. L. Ooi, J. L. Revuelta, C. J. Roberts, M. Rose, P. Ross-Macdonald, B. Scherens, G. Schimmack, B. Shafer, D. D. Shoemaker, S. Sookhai-Mahadeo, R. K. Storms, J. N. Strathern, G. Valle, M. Voet, G. Volckaert, C. Wang, T. R. Ward, J. Wilhelmy, E. A. Winzeler, Y. Yang, G. S. Yen, E. Youngman, K. Yu, H. Bussey, J. D. Boeke, M. Snyder, P. Philippsen, R. W. Davis and M. Johnston, Nature, 2002, 418, 387–391 CrossRef CAS PubMed.
- L. M. Steinmetz, C. Scharfe, A. M. Deutschbauer, D. Mokranjac, Z. S. Herman, T. Jones, A. M. Chu, G. Giaever, H. Prokisch, P. J. Oefner and R. W. Davis, Nat. Genet., 2002, 31, 400–404 CAS.
- E. A. Winzeler, D. D. Shoemaker, A. Astromoff, H. Liang, K. Anderson, B. Andre, R. Bangham, R. Benito, J. D. Boeke, H. Bussey, A. M. Chu, C. Connelly, K. Davis, F. Dietrich, S. W. Dow, M. El Bakkoury, F. Foury, S. H. Friend, E. Gentalen, G. Giaever, J. H. Hegemann, T. Jones, M. Laub, H. Liao, N. Liebundguth, D. J. Lockhart, A. Lucau-Danila, M. Lussier, N. M'Rabet, P. Menard, M. Mittmann, C. Pai, C. Rebischung, J. L. Revuelta, L. Riles, C. J. Roberts, P. Ross-MacDonald, B. Scherens, M. Snyder, S. Sookhai-Mahadeo, R. K. Storms, S. Véronneau, M. Voet, G. Volckaert, T. R. Ward, R. Wysocki, G. S. Yen, K. Yu, K. Zimmermann, P. Philippsen, M. Johnston and R. W. Davis, Science, 1999, 285, 901–906 CrossRef CAS.
- S. R. Engel, R. Balakrishnan, G. Binkley, K. R. Christie, M. C. Costanzo, S. S. Dwight, D. G. Fisk, J. E. Hirschman, B. C. Hitz, E. L. Hong, C. J. Krieger, M. S. Livstone, S. R. Miyasato, R. S. Nash, R. Oughtred, J. Park, M. S. Skrzypek, S. Weng, E. D. Wong, K. Dolinski, D. Botstein and J. M. Cherry, Nucleic Acids Res., 2009, 38, 1–4 Search PubMed.
- J. M. Cherry, E. L. Hong, C. Amundsen, R. Balakrishnan, G. Binkley, E. T. Chan, K. R. Christie, M. C. Costanzo, S. S. Dwight, S. R. Engel, D. G. Fisk, J. E. Hirschman, B. C. Hitz, K. Karra, C. J. Krieger, S. R. Miyasato, R. S. Nash, J. Park, M. S. Skrzypek, M. Simison, S. Weng and E. D. Wong, Nucleic Acids Res., 2012, 40, 1–6 CrossRef PubMed.
- M. Costanzo, A. Baryshnikova, J. Bellay, Y. Kim, E. D. Spear, C. S. Sevier, H. Ding, J. L. Y. Koh, K. Toufighi, S. Mostafavi, J. Prinz, R. P. St Onge, B. VanderSluis, T. Makhnevych, F. J. Vizeacoumar, S. Alizadeh, S. Bahr, R. L. Brost, Y. Chen, M. Cokol, R. Deshpande, Z. Li, Z. Y. Lin, W. Liang, M. Marback, J. Paw, B. J. San Luis, E. Shuteriqi, A. H. Y. Tong, N. Van Dyk, I. M. Wallace, J. A. Whitney, M. T. Weirauch, G. Zhong, H. Zhu, W. A. Houry, M. Brudno, S. Ragibizadeh, B. Papp, C. Pál, F. P. Roth, G. Giaever, C. Nislow, O. G. Troyanskaya, H. Bussey, G. D. Bader, A. C. Gingras, Q. D. Morris, P. M. Kim, C. A. Kaiser, C. L. Myers, B. J. Andrews and C. Boone, Science, 2010, 327, 425–431 CrossRef CAS PubMed.
- E. S. Snitkin, A. M. Dudley, D. M. Janse, K. Wong, G. M. Church and D. Segrè, Genome Biol., 2008, 9, R140 CrossRef PubMed.
- B. Vandersluis, D. C. Hess, C. Pesyna, E. W. Krumholz, T. Syed, B. Szappanos, C. Nislow, B. Papp, O. G. Troyanskaya, C. L. Myers and A. A. Caudy, Genome Biol., 2014, 15, R64 CrossRef PubMed.
- D. McCloskey, B. Ø. Palsson and A. M. Feist, Mol. Syst. Biol., 2013, 9, 661 CrossRef CAS PubMed.
- E. Gonçalves, J. Bucher, A. Ryll, J. Niklas, K. Mauch, S. Klamt, M. Rocha and J. Saez-Rodriguez, Mol. BioSyst., 2013, 9, 1576–1583 RSC.
- R. Saha, A. Chowdhury and C. D. Maranas, Curr. Opin. Biotechnol., 2014, 29, 39–45 CrossRef CAS PubMed.
- A. S. Blazier and J. A. Papin, Front. Physiol., 2012, 3, 299 Search PubMed.
- A. Hoppe, Metabolites, 2012, 2, 614–631 CrossRef CAS PubMed.
- M. Åkesson, J. Förster and J. Nielsen, Metab. Eng., 2004, 6, 285–293 CrossRef PubMed.
- J. L. Hjersted and M. A. Henson, IET Syst. Biol., 2009, 3, 167–179 CrossRef CAS PubMed.
- B. J. Sánchez, J. R. Pérez-Correa and E. Agosin, Metab. Eng., 2014, 25, 159–173 CrossRef PubMed.
- S. A. Becker and B. Ø. Palsson, PLoS Comput. Biol., 2008, 4, e1000082 Search PubMed.
- H. Zur, E. Ruppin and T. Shlomi, Bioinformatics, 2010, 26, 3140–3142 CrossRef CAS PubMed.
- E. Almaas, B. Kovács, T. Vicsek, Z. N. Oltvai and A.-L. Barabási, Nature, 2004, 427, 839–843 CrossRef CAS PubMed.
- Y. Bilu, T. Shlomi, N. Barkai and E. Ruppin, PLoS Comput. Biol., 2006, 2, 0932–0938 CAS.
- O. Rokhlenko, T. Shlomi, R. Sharan, E. Ruppin and R. Y. Pinter, Bioinformatics, 2007, 23, 2139–2146 CrossRef CAS PubMed.
- S. Bordel, R. Agren and J. Nielsen, PLoS Comput. Biol., 2010, 6, 16 Search PubMed.
- J. L. Martínez, S. Bordel, K. K. Hong and J. Nielsen, FEMS Yeast Res., 2014, 14, 654–662 CrossRef PubMed.
- C. J. Kennedy, P. M. Boyle, Z. Waks and P. A. Silver, Genetics, 2009, 183, 385–397 CrossRef CAS PubMed.
- P. A. Jensen and J. A. Papin, Bioinformatics, 2011, 27, 541–547 CrossRef CAS PubMed.
- R. J. P. Van Berlo, D. de Ridder, J. M. Daran, P. A. S. Daran-Lapujade, B. Teusink and M. J. T. Reinders, IEEE/ACM Trans. Comput. Biol. Bioinf., 2011, 8, 206–216 CrossRef PubMed.
- A. Salehzadeh-Yazdi, Y. Asgari, A. A. Saboury and A. Masoudi-Nejad, PLoS One, 2014, 9, e111686 Search PubMed.
- J. Kim and J. L. Reed, Genome Biol., 2012, 13, R78 CrossRef PubMed.
- D. Lee, K. Smallbone, W. B. Dunn, E. Murabito, C. L. Winder, D. B. Kell, P. Mendes and N. Swainston, BMC Syst. Biol., 2012, 6, 1 CrossRef PubMed.
- S. Rossell, M. A. Huynen and R. A. Notebaart, PLoS Comput. Biol., 2013, 9, e1002988 CAS.
- H. S. Song, J. Reifman and A. Wallqvist, PLoS One, 2014, 9, e112524 Search PubMed.
- M. J. Herrgård, B. S. Lee, V. Portnoy and B. Ø. Palsson, Genome Res., 2006, 16, 627–635 CrossRef PubMed.
- P. A. Jensen, K. A. Lutz and J. A. Papin, BMC Syst. Biol., 2011, 5, 147 CrossRef CAS PubMed.
- S. Chandrasekaran and N. D. Price, PLoS Comput. Biol., 2013, 9, e1003370 Search PubMed.
- J. M. Savinell and B. Ø. Palsson, J. Theor. Biol., 1992, 154, 421–454 CrossRef CAS.
- E. Reznik, P. Mehta and D. Segrè, PLoS Comput. Biol., 2013, 9, e1003195 CAS.
- A. Wagner, R. Zarecki, L. Reshef, C. Gochev, R. Sorek, U. Gophna and E. Ruppin, Proc. Natl. Acad. Sci. U. S. A., 2013, 110, 19166–19171 CrossRef CAS PubMed.
- K. Yizhak, O. Gabay, H. Cohen and E. Ruppin, Nat. Commun., 2013, 4, 2632 Search PubMed.
- B. Barker, N. Sadagopan, Y. Wang, K. Smallbone, M. R. Christopher, H. Xi, J. W. Locasale and Z. Gu, Mol. Networks, 2014 Search PubMed , arXiv:1404.4755.
- D. Machado and M. J. Herrgård, PLoS Comput. Biol., 2014, 10, e1003580 Search PubMed.
- K. R. Patil and J. Nielsen, Proc. Natl. Acad. Sci. U. S. A., 2005, 102, 2685–2689 CrossRef CAS PubMed.
- A. P. Oliveira, K. R. Patil and J. Nielsen, BMC Syst. Biol., 2008, 2, 17 CrossRef PubMed.
- R. Usaite, K. R. Patil, T. Grotkjær, J. Nielsen and B. Regenberg, Appl. Environ. Microbiol., 2006, 72, 6194–6203 CrossRef CAS PubMed.
- S. L. Westergaard, A. P. Oliveira, C. Bro, L. Olsson and J. Nielsen, Biotechnol. Bioeng., 2007, 96, 134–145 CrossRef CAS PubMed.
- D. Cimini, K. R. Patil, C. Schiraldi and J. Nielsen, BMC Syst. Biol., 2009, 3, 17 CrossRef PubMed.
- R. Usaite, M. C. Jewett, A. P. Oliveira, J. R. Yates, L. Olsson and J. Nielsen, Mol. Syst. Biol., 2009, 5, 319 CrossRef PubMed.
- P. Chumnanpuen, J. Zhang, I. Nookaew and J. Nielsen, Mol. Genet. Genomics, 2012, 287, 541–554 CrossRef CAS PubMed.
- P. Chumnanpuen, I. Nookaew and J. Nielsen, BMC Syst. Biol., 2013, 7, S7 CrossRef PubMed.
- W. A. Bryant, M. J. E. Sternberg and J. W. Pinney, BMC Syst. Biol., 2013, 7, 26 CrossRef PubMed.
- M. C. Jewett, C. T. Workman, I. Nookaew, F. A. Pizarro, E. Agosin, L. I. Hellgren and J. Nielsen, G3, 2013, 3, 1979–1995 CrossRef PubMed.
- E. Lindfors, P. Jouhten, M. Oja, E. Rintala, M. Orešič and M. Penttilä, BMC Syst. Biol., 2014, 8, 16 CrossRef PubMed.
- P. Kharchenko, D. Vitkup and G. M. Church, Bioinformatics, 2004, 20, 178–185 CrossRef PubMed.
- P. Kharchenko, L. Chen, Y. Freund, D. Vitkup and G. M. Church, BMC Bioinf., 2006, 7, 177 CrossRef PubMed.
- K. Y. Teh and A. E. Lutz, J. Biotechnol., 2010, 147, 80–87 CrossRef CAS PubMed.
- D. Segrè, A. Deluna, G. M. Church and R. Kishony, Nat. Genet., 2005, 37, 77–83 Search PubMed.
- X. He, W. Qian, Z. Wang, Y. Li and J. Zhang, Nat. Genet., 2010, 42, 272–276 CrossRef CAS PubMed.
- E. S. Snitkin and D. Segrè, PLoS Genet., 2011, 7, e1001294 CAS.
- R. Harrison, B. Papp, C. Pál, S. G. Oliver and D. Delneri, Proc. Natl. Acad. Sci. U. S. A., 2007, 104, 2307–2312 CrossRef CAS PubMed.
- B. Szappanos, K. Kovács, B. Szamecz, F. Honti, M. Costanzo, A. Baryshnikova, G. Gelius-Dietrich, M. J. Lercher, M. Jelasity, C. L. Myers, B. J. Andrews, C. Boone, S. G. Oliver, C. Pál and B. Papp, Nat. Genet., 2011, 43, 656–662 CrossRef CAS PubMed.
- L. Xu, B. Barker and Z. Gu, Proc. Natl. Acad. Sci. U. S. A., 2012, 109, 10420–10425 CrossRef CAS PubMed.
- A. R. Brochado, S. Andrejev, C. D. Maranas and K. R. Patil, PLoS Comput. Biol., 2012, 8, e1002758 CAS.
- R. Costenoble, P. Picotti, L. Reiter, R. Stallmach, M. Heinemann, U. Sauer and R. Aebersold, Mol. Syst. Biol., 2011, 7, 464 CrossRef PubMed.
- J. C. Schulz, M. Zampieri, S. Wanka, C. Von Mering and U. Sauer, Sci. Signaling, 2014, 7, rs6 CrossRef PubMed.
- A. Kümmel, S. Panke and M. Heinemann, Mol. Syst. Biol., 2006, 2, 2006.0034 CrossRef PubMed.
- N. Zamboni, A. Kümmel and M. Heinemann, BMC Bioinf., 2008, 9, 199 CrossRef PubMed.
- J. C. Ewald, T. Matt and N. Zamboni, Mol. BioSyst., 2013, 9, 440–446 RSC.
- S. J. Jol, A. Kümmel, M. Terzer, J. Stelling and M. Heinemann, PLoS Comput. Biol., 2012, 8, 1–9 Search PubMed.
- V. S. Martínez, L. E. Quek and L. K. Nielsen, Biophys. J., 2014, 107, 493–503 CrossRef PubMed.
- K. Smallbone and P. Mendes, Ind. Biotechnol., 2013, 9, 179–184 CrossRef CAS.
- N. J. Stanford, T. Lubitz, K. Smallbone, E. Klipp, P. Mendes and W. Liebermeister, PLoS One, 2013, 8, e79195 Search PubMed.
- K. C. Soh, L. Miskovic and V. Hatzimanikatis, FEMS Yeast Res., 2012, 12, 129–143 CrossRef CAS PubMed.
- C. M. Paget, J. M. Schwartz and D. Delneri, Mol. Ecol., 2014, 23, 5241–5257 CrossRef CAS PubMed.
- J. L. Hjersted, M. A. Henson and R. Mahadevan, Biotechnol. Bioeng., 2007, 97, 1190–1204 CrossRef CAS PubMed.
- R. Eslamloueyan and P. Setoodeh, Chem. Eng. Commun., 2011, 198, 1309–1338 CrossRef CAS PubMed.
- A. Ghosh, H. Zhao and N. D. Price, PLoS One, 2011, 6, e27316 CAS.
- J. Zanghellini, K. Natter, C. Jungreuthmayer, A. Thalhammer, C. F. Kurat, G. Gogg-Fassolter, S. D. Kohlwein and H. H. Von Grünberg, FEBS J., 2008, 275, 5552–5563 CrossRef CAS PubMed.
- T. J. Hanly and M. A. Henson, Biotechnol. Bioeng., 2011, 108, 376–385 CrossRef CAS PubMed.
- J. T. Leppävuori, M. M. Domach and L. T. Biegler, Ind. Eng. Chem. Res., 2011, 50, 12080–12091 CrossRef.
- F. A. Vargas, F. A. Pizarro, J. R. Pérez-Correa and E. Agosin, BMC Syst. Biol., 2011, 5, 75 CrossRef CAS PubMed.
- T. J. Hanly, M. Urello and M. A. Henson, Appl. Microbiol. Biotechnol., 2012, 93, 2529–2541 CrossRef CAS PubMed.
- P. Jouhten, M. Wiebe and M. Penttilä, FEBS J., 2012, 279, 3338–3354 CrossRef CAS PubMed.
- T. J. Hanly and M. A. Henson, Biotechnol. Biofuels, 2013, 6, 44 CrossRef CAS PubMed.
- K. Höffner, S. M. Harwood and P. I. Barton, Biotechnol. Bioeng., 2013, 110, 792–802 CrossRef PubMed.
- J. A. Gomez, K. Höffner and P. I. Barton, BMC Bioinf., 2014, 15, 409 CrossRef PubMed.
- T. J. Hanly and M. A. Henson, Biotechnol. Bioeng., 2014, 111, 272–284 CrossRef CAS PubMed.
- P. K. Lisha and D. Sarkar, Bioprocess Biosyst. Eng., 2014, 37, 617–627 CrossRef PubMed.
- P. K. Lisha and D. Sarkar, Biotechnol. Res. Int., 2015, 2015, 238082 Search PubMed.
- A. M. Willemsen, D. M. Hendrickx, H. C. J. Hoefsloot, M. M. W. B. Hendriks, S. A. Wahl, B. Teusink, A. K. Smilde and A. H. C. Van Kampen, Mol. BioSyst., 2015, 11, 137–145 RSC.
- A. Chowdhury, A. R. Zomorrodi and C. D. Maranas, PLoS Comput. Biol., 2014, 10, e1003487 Search PubMed.
- K. Smallbone, E. Simeonidis, N. Swainston and P. Mendes, BMC Syst. Biol., 2010, 4, 6 CrossRef PubMed.
- A. F. Villaverde, D. Henriques, K. Smallbone, S. Bongard, J. Schmid, D. Cicin-Sain, A. Crombach, J. Saez-Rodriguez, K. Mauch, E. Balsa-Canto, P. Mendes, J. Jaeger and J. R. Banga, BMC Syst. Biol., 2015, 9, 8 CrossRef PubMed.
- R. Mahadevan, J. S. Edwards and F. J. Doyle III, Biophys. J., 2002, 83, 1331–1340 CrossRef CAS.
- B. Teusink, J. Passarge, C. A. Reijenga, E. Esgalhado, C. C. Van Der Weijden, M. Schepper, M. C. Walsh, B. M. Bakker, K. Van Dam, H. V Westerhoff and J. L. Snoep, Eur. J. Biochem., 2000, 267, 5313–5329 CrossRef CAS.
- E. Klipp, Yeast, 2007, 24, 943–959 CrossRef CAS PubMed.
- C. Reder, J. Theor. Biol., 1988, 135, 175–201 CrossRef CAS.
- L. Miskovic and V. Hatzimanikatis, Trends Biotechnol., 2010, 28, 391–397 CrossRef CAS PubMed.
- Q. K. Beg, A. Vazquez, J. Ernst, M. A. de Menezes, Z. Bar-Joseph, A. L. Barabási and Z. N. Oltvai, Proc. Natl. Acad. Sci. U. S. A., 2007, 104, 12663–12668 CrossRef CAS PubMed.
- M. J. Van Hoek and R. M. Merks, BMC Syst. Biol., 2012, 6, 22 CrossRef PubMed.
- M. Celton, A. Goelzer, C. Camarasa, V. Fromion and S. Dequin, Metab. Eng., 2012, 14, 366–379 CrossRef CAS PubMed.
- D. Dikicioglu, S. Oc, B. M. Rash, W. B. Dunn, P. Pir, D. B. Kell, B. Kirdar and S. G. Oliver, Mol. BioSyst., 2014, 10, 93–102 RSC.
- B. J. Stewart, A. Navid, K. W. Turteltaub and G. Bench, Anal. Chem., 2010, 82, 9812–9817 CrossRef CAS PubMed.
- R. M. Zelle, E. De Hulster, W. A. Van Winden, P. De Waard, C. Dijkema, A. A. Winkler, J. M. A. Geertman, J. P. Van Dijken, J. T. Pronk and A. J. A. Van Maris, Appl. Environ. Microbiol., 2008, 74, 2766–2777 CrossRef CAS PubMed.
- T. Çakir, K. R. Patil, Z. iIsen Onsan, K. O. Ulgen, B. Kirdar and J. Nielsen, Mol. Syst. Biol., 2006, 2, 50 CrossRef PubMed.
- A. P. Burgard, E. V Nikolaev, C. H. Schilling and C. D. Maranas, Genome Res., 2004, 14, 301–312 CrossRef CAS PubMed.
- J. G. Bundy, B. Papp, R. Harmston, R. A. Browne, E. M. Clayson, N. Burton, R. J. Reece, S. G. Oliver and K. M. Brindle, Genome Res., 2007, 17, 510–519 CrossRef CAS PubMed.
- C. E. García Sánchez, C. A. Vargas García and R. G. Torres Sáez, PLoS One, 2012, 7, e43006 Search PubMed.
- R. Agren, J. M. Otero and J. Nielsen, J. Ind. Microbiol. Biotechnol., 2013, 40, 735–747 CrossRef CAS PubMed.
- P. B. Hyland, S. L. S. Mun and R. Mahadevan, Ind. Biotechnol., 2013, 9, 229–235 CrossRef CAS.
- U. Sauer, Mol. Syst. Biol., 2006, 2, 62 CrossRef PubMed.
- L. M. Blank, L. Kuepfer and U. Sauer, Genome Biol., 2005, 6, R49 CrossRef PubMed.
- A. Misra, M. F. Conway, J. Johnnie, T. M. Qureshi, B. Lige, A. M. Derrick, E. C. Agbo and G. Sriram, Front. Microbiol., 2013, 4, 1–15 Search PubMed.
- E. S. Snitkin and D. Segrè, Int. Conf. Genome Inf., 2008, 20, 123–134 CAS.
- A. Dräger and B. Ø. Palsson, Front. Bioeng. Biotechnol., 2014, 2, 1–20 Search PubMed.
- A. Kumar, P. F. Suthers and C. D. Maranas, BMC Bioinf., 2012, 13, 6 CrossRef PubMed.
- D. Weininger, J. Chem. Inf. Comput. Sci., 1988, 28, 31–36 CrossRef CAS.
- C. Brooksbank, G. Cameron and J. Thornton, Nucleic Acids Res., 2005, 33, 46–53 CrossRef PubMed.
- S. J. Coles, N. E. Day, P. Murray-Rust, H. S. Rzepa and Y. Zhang, Org. Biomol. Chem., 2005, 3, 1832–1834 CAS.
- H. W. Mewes, A. Ruepp, F. Theis, T. Rattei, M. Walter, D. Frishman, K. Suhre, M. Spannagl, K. F. X. Mayer, V. Stümpflen and A. Antonov, Nucleic Acids Res., 2011, 39, 220–224 CrossRef PubMed.
- H. W. Wisselink, C. Cipollina, B. Oud, B. Crimi, J. J. Heijnen, J. T. Pronk and A. J. A. van Maris, Metab. Eng., 2010, 12, 537–551 CrossRef CAS PubMed.
|
This journal is © The Royal Society of Chemistry 2015 |