DOI:
10.1039/C5MB00003C
(Paper)
Mol. BioSyst., 2015,
11, 1041-1051
Understanding the binding of inhibitors of matrix metalloproteinases by molecular docking, quantum mechanical calculations, molecular dynamics simulations, and a MMGBSA/MMBappl study†
Received
3rd January 2015
, Accepted 14th January 2015
First published on 14th January 2015
Abstract
Matrix metalloproteinases (MMPs) consist of a class of proteins required for normal tissue function. Their over expression is associated with many disease states and hence the interest in MMPs as drug targets. Almost all MMP inhibitors have been reported to fail in clinical trials due to lack of specificity. Zinc in the binding site of metalloproteinases performs essential biological functions and contributes to the binding affinity of inhibitors. The multiple possibilities for coordination geometry and the consequent charge on the zinc atom indicate that parameters developed are not directly transferable across different families of zinc metalloproteinases with different zinc coordination geometries, active sites and ligand architectures which makes it difficult to evaluate metal–ligand interactions. In order to assist in drug design endeavors for MMP targets, a computationally tractable pathway is presented, comprising docking of small molecule inhibitors against the target MMPs, derivation of quantum mechanical charges on the zinc ion in the active site and the amino acids coordinating with zinc including the inhibitor molecule, molecular dynamics simulations on the docked ligand–MMP complexes and evaluation of binding affinities of the ligand–MMP complexes via an accurate scoring function for zinc containing metalloprotein–ligand complexes. The above pathway was applied to study the interaction of inhibitor Batimastat with MMPs, which resulted in a high correlation between the predicted binding free energies and experiment, suggesting the potential applicability of the pathway. We then proceeded to formulate a few design principles which identify the key protein residues for generating molecules with high affinity and specificity against each of the MMPs.
Introduction
MMPs as drug targets
Matrix metalloproteinases constitute a family of Ca2+ containing and Zn2+ dependent proteins. The family consists of more than 26 proteinases in mammals. Based on their substrate specificity, these are classified into collagenases (MMP-1, 8, 13 and 18), gelatinases (MMP-2, and 9), stromelysins (MMP-3, 10, 11, and 27), matrilysins (MMP-7 and 26) and membrane-type (MT-MMP) and other enzymes.1 The members contain a pre-domain involved in enzyme secretion and a catalytic domain for the activity of the enzyme. However, a C terminal domain in MMP-7, MMP-27 and MMP-23 is involved in substrate recognition. MMPs synthesized as inactive zymogens are activated through the cleavage of their pro-domains by other enzymes.1 Their enzymatic activity is regulated by their natural inhibitors viz. tissue inhibitors of metalloproteinases (TIMPs).2,3 Expression of these enzymes is responsible for several biological processes such as embryonic development, signal regulation, wound healing, angiogenesis, ovulation, uterine involution, bone resorption and nerve growth.4–8 However a disturbance of this leads to over-expression of MMPs followed by accelerated matrix degradation mediating a number of pathological processes by changing the structures of a large number of substrates. The imbalance leads to several pathologies including cancer, loss of cartilage in osteoarthritis, rheumatoid arthritis, cardiovascular diseases, acute lung injury, peridontitis and many others.9–15 The over expression of MMP-1, MMP-2, MMP-3, MMP-8, MMP-9, MMP-13 has been implicated in various diseases like breast cancer,16 gastric cancer,17 peripheral nerve injury,18–23 neuropathic pain,23 spinal cord injury,24–26 brain injury,27–29 colorectal cancer,30 pathologic bone resorption,31 chronic wound,32 inflammation of the skin,33 inflammation of the pulmonary tract,34 hypersensitivity,35 Alzheimer's disease,36,37 Crohn's disease,37 peridontitis.38 As a result, MMPs have become drug targets of prime interest for finding effective inhibitors. The number of available high resolution X-ray crystal structures of MMP–inhibitor complexes has increased dramatically in recent years helping in the design of potential inhibitors at an early lead generation stage.39 Molecules exhibiting high affinity toward Zn2+ effectively would prevent the binding of the polypeptide to MMPs and thus are considered to act as MMP inhibitors.40 Several Zn2+-binding groups (ZBGs) have been reported: the hydroxamates, reverse hydroxamates, carboxylates, hydroxyureas, hydrazides, phosphinates, sulfones, and sulfonylhydrazides of which the hydroxamates appear to be the most potent ones among them.1 Many broad spectrum ZBG-containing small molecule inhibitors from different pharmaceutical companies have also entered clinical trials for cancer, rheumatoid arthritis, and osteoarthritis.41,42 These broad-spectrum MMP inhibitors include hydroxamate-based Marimastat, Batimastat, Ilomastat, Prinomastat, Solimastat, Tanomastat, MMI-270, Trocade, Periomastat and Metamastat. Nearly all of these MMP inhibitors have failed in clinical trials due to lack of specificity against a given class of MMPs1 posing a challenge to rational drug design of specific MMP inhibitors. Zinc is commonly found to be four-coordinated with a tetrahedral geometry; five and six coordinated geometries are also observed in zinc metalloproteinases, playing an important role in metal–ligand binding.41 The geometries of zinc in different protein–ligand complexes have been illustrated previously.43 Computational docking and prediction of binding affinities of metalloproteinase inhibitors to MMPs remains a challenge due to the multiple coordination geometries of zinc and lack of appropriate force field parameters to model the metal–ligand interactions.44 Addressing these challenges, we combine in the current study, molecular docking, quantum mechanical charge derivation of the Zn atom in the binding site followed by molecular dynamics simulations on MMP–inhibitor complexes, and a post facto analysis of the MD trajectories to evaluate the binding free energies associated with MMP–inhibitor complexation. The ability to effectively predict the binding modes and affinities of small molecule inhibitors45 of these zinc containing enzymes computationally can be of utmost importance in designing very selective clinically relevant inhibitors. Our study has resulted in the identification of key residues in different MMPs and formulation of a few design principles and further a set of new molecules with high affinity and specificity for each of the MMPs. These are described below.
Methodology
Batimastat is a broad spectrum MMP inhibitor reported to have IC50 values (in nM) of 10, 4, 20, 10, 1 and 3 against MMP-1, MMP-2, MMP-3, MMP-8, MMP-9, and MMP-13 respectively.1 A docking and scoring study of Batimastat against these classes of MMPs was performed. A flowchart describing the computational pathway followed for a systematic analysis of the binding of MMP inhibitors to the target protein in the current study is shown in Fig. 1.
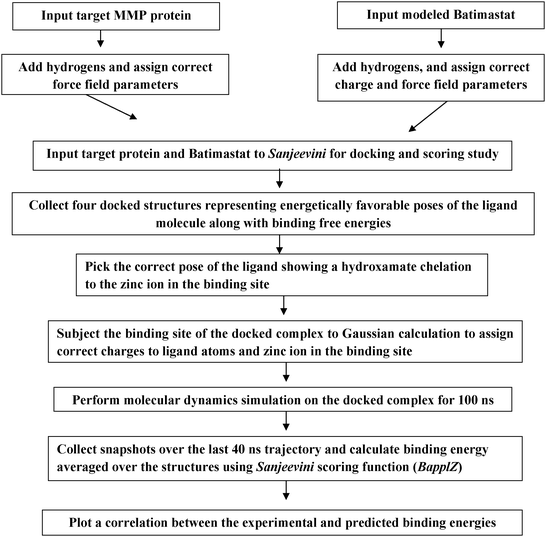 |
| Fig. 1 A flowchart showing the steps followed in the docking and scoring study of Batimastat binding to different MMPs. | |
Docking the inhibitor in the target protein
The three-dimensional structures of MMPs were adapted from RCSB with PDB ids 2TCL, 1HOV, 1BIW, 1MNC, 1GKC, 456C corresponding to MMP-1, MMP-2, MMP-3, MMP-8, MMP-9 and MMP-13 respectively.2 The zinc ion occurs in 4 or 5 coordinate geometry with the protein and the ligand atoms which are lying within a distance of 1.8 Å to 2.7 Å. A vast majority of MMP inhibitors chelate the catalytic zinc ion either in monodentate or bidentate fashion. These inhibitors replace the water molecule and coordinately bind to the zinc. Thus, the crystallographic water was removed from the protein42,46–48 before performing the docking studies. Hydrogens were added49 and the correct force field parameters were assigned to the protein.43 The protonation states of the histidine residue were adjusted according to the hydrogen bond network.50 The basic amino acids were protonated and the carboxylic groups were kept deprotonated. The coordinates of the Batimastat–MMP complexes are not reported. The inhibitor molecule Batimastat was modeled1 maintaining the ionization states mentioned in the literature. The overall formal charge42 on the Batimastat was −1. The molecule was then geometry optimized through the AM1 procedure followed by calculation of partial charges of the ligand by the AM1-BCC procedure.51 GAFF force field parameters52 were used to assign atom types, bond angles, dihedral and van der Waals parameters53 to the inhibitor molecule. The target protein–ligand complex and the inhibitor molecule were given as input to Sanjeevini54 software suite for docking and scoring studies. Sanjeevini is a freely accessible web server for protein and DNA targeted lead molecule discovery. The web server builds in several features including detection of the binding site in the target protein, scanning against a library of million compounds to identify hit molecules against a target protein, all atom based docking and scoring module and various other utilities to design molecules with desired affinity and specificity. The docking and scoring module of Sanjeevini has been previously validated on a large dataset of 335 protein–DNA drug targets for inhibitors with known crystal structures and known experimental binding free energies.54 The protein targets consist of zinc containing metallo proteinases as well. The predicted binding free energy of the top ranked docked structures given as an output when compared with the experimental binding free energies gave a correlation coefficient r of 0.83. The root mean square deviation of the top ranked docked structure against the crystal structure was within 2 Å with 90% accuracy. The docking and scoring module of Sanjeevini was validated on matrix metalloproteinases with known inhibitors. A correlation coefficient r of 0.82 and R2 = 0.68 was obtained between experimental and predicted binding free energies of the top ranked docked structure as shown in Fig. 2. The predicted binding free energies along with the RMSDs of the top ranked docked structure against the crystal structure are given in Table S1 (ESI†).
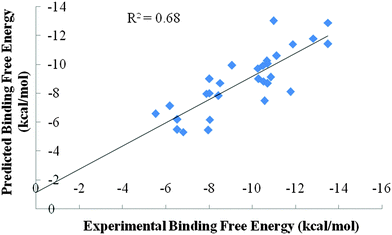 |
| Fig. 2 Correlation between experimental binding free energies and predicted binding free energies (in kcal mol−1) for the binding of known MMP inhibitors. | |
The docking module of Sanjeevini55,56 docks the ligand molecule in the binding site and generates several (∼103) configurations via a six-dimensional rigid body Monte Carlo methodology resulting in many ligand configurations which are scored based on the scoring function in-built in Sanjeevini.43,57–59
Derivation of partial atomic charges on the docked ligand and the zinc ion, and the protein atoms
In the zinc containing metalloproteinases the ligand molecule is bonded with one or two co-ordinate bonds with the zinc ion due to which the total formal charge on the zinc is always less than +2 due to the charge transfer occurring between the amino acids and the ligand molecule coordinately bonded to the zinc ion. An accurate modelling of a large biomolecular system by the quantum mechanical method is computationally very expensive. However, quantum mechanical calculations on a small part of the system (active site of a protein) are affordable which could lead to insufficient descriptions. The semiempirical methods have successfully handled thousands of atoms but are not accurate in modelling systems with transition metals. The hybrid QM/MM method pioneered by Warshel and Levitt60 is successful in providing solutions for the complex systems. The ONIOM (Own N layer Integrated molecular Orbital molecular mechanics) method is successfully applied to metalloenzymes. In a similar approach, where for the smaller region that is on the zinc binding moiety comprising the protein residues within the coordinate bond distance (≤2.7 Å) of the zinc ion, the ligand and the zinc ion were subjected to HF/6-31G* ab initio calculations through Gaussian software61 suite followed by RESP fitting on the resultant electrostatic potentials to obtain partial atomic charges on the ligand and the zinc ion. For the calculations, the protein residues within the coordinate bond distance were deprotonated and the net charge on the zinc binding motif was calculated which was a sum of the formal charge on each amino acid residue, formal charge on the ligand and +2 charge on the zinc ion.43 van der Waals parameters for the zinc ion were adapted from the work of Stote and Karplus62 [σ = 1.95 Å and ε = 0.25 kcal] together with GAFF force field parameters for the ligand molecule.51 The AntechAMBER49 module of AMBER was used to assign the bonded and the nonbonded parameters to the ligand atoms. The protein atoms were assigned RESP derived partial atomic charges, van der Waals and bonded parameters using the AMBER force field.43 The charges on the docked-MMP complexes are available at the following link http://scfbio-iitd.res.in/sanjeevini/MMP.jsp.
Accounting for protein and ligand flexibility through molecular dynamics simulations
The protein–inhibitor complexes were subjected to molecular dynamics simulations to factor in the flexibility/dynamics of the ligand and the active site residues of the target along with explicit solvent and salt effects.63 Simulations were carried out with periodic boundary conditions using AMBER suite of programs.49 11 Na+ ions were added to MMP-2 and MMP-3 inhibitor complexes, 12 Na+ ions to MMP-1, MMP-8, and MMP-9 inhibitor complexes and 7 Na+ ions to the MMP-13 inhibitor complex to ensure electroneutrality of the protein–ligand complex being simulated. The complex was then solvated with a layer of water 8 Å thick. Water was modeled using the TIP4PEW parameters.64 To maintain the orientation of the zinc-chelating histidine residues and to prevent the escape of the zinc atoms into the solvent, a restraint was applied on the distances defined by zinc and the nitrogen atoms of the histidine residue. After the initial preparation of the docked complexes the solvent was subjected to an initial minimization followed by the minimization of the solute–solvent system. A slow heating to 300 K was performed at constant volume over a period of 100 ps using harmonic restraints of 25 kcal mol−1 Å−2 on the solute atoms. These restraints were then slowly relaxed from 5 to 1 kcal mol−1 Å−2 in five segments of 1000 steps of energy minimization and 50 ps of equilibration with a constant temperature of 300 K and a pressure of 1 bar via the Berendsen algorithm65 with a coupling constant of 0.2 ps for both parameters. Finally a 50 ps equilibration with a restraint of 0.5 kcal mol−1 Å−2 and a 50 ps of unrestrained equilibration was performed. Molecular dynamics simulation was then carried out for 100 ns at constant temperature and pressure conditions using Berendsen algorithm with a coupling constant of 5 ps. In all, six molecular dynamics simulation66 runs were performed corresponding to the binding of Batimastat with MMP-1, MMP-2, MMP-3, MMP-8, MMP-9, and MMP-13. RMSD versus time plots were monitored for all the simulation runs to ensure the stability of the docked complexes.
Post molecular dynamics simulation preparation of the docked complexes
At the end of molecular dynamics simulations, 100 structures were obtained from each of the six trajectories at equal intervals from the last 40 ns trajectory file and prepared for binding free energy estimates of the protein–ligand complexes using Bappl-Z43 scoring function. The approach is parameterized within the additivity approximation where the net free energy change is treated as a sum of a comprehensive set of individual energy-component contributions. The components are estimated in a force field compatible manner. The equation for the estimation of free energy change upon binding is: |  | (1) |
This methodology overcomes the limitations inherent in single point calculations (studies on energy minimized structures) by subjecting the systems to configurational averaging via molecular dynamic simulations. The above BapplZ scoring function validated thoroughly the earlier43 captures of the theoretical rigor of MMGBSA/MMPBSA67–71 methodology as well as rapidity of empirical/knowledge based methods.43,72,73 Each component in eqn (1) is described below.
E
el electrostatic term includes interactions between protein with the ligand atoms and the zinc ion with the rest of the complex. The interactions are calculated from Coulomb's law with a sigmoidal dielectric function for solvent screening to incorporate the desolvation electrostatics implicitly.43,57,72 A non-bonded model from the work of Stote and Karplus62 has been adopted to model the electrostatics interactions of zinc with the rest of the complex.
E
vdW van der Waals term includes direct van der Waals between protein with ligand atoms and the zinc ion with the rest of the complex. The interactions were modeled using a (12,6) Lennard-Jones potential.43 Again the work of Stote and Karplus was adopted to model the van der Waals interactions for the zinc ion.
σ
A
ΔALSA hydrophobic component captures mainly the non electrostatic component of desolvation using the modified version of the Eisenberg–Mclachlan model where the atom types in the AMBER force field for proteins and small molecules have been combined into a set of 22 atom types43 to ensure transferability of parameters to other biomolecular systems. ΔALSA in the above eqn (1) represents the net loss in surface area for an atom type.
The ΔSCR term represents the loss in conformational entropy of protein side chains upon the binding of ligand to the protein.43
Computational details of the above work have been explained earlier.43 The ΔG° from the above equation is calculated for the single point system representing each snapshot of the structures obtained over the last 40 ns trajectory file and a block averaging is done to obtain the average binding free energy values.
Results and discussion
In the zinc containing metalloprotein–ligand complexes, the ligand is coordinately bonded to the zinc ion with one or two coordinate bonds. Sanjeevini gives as output four docked structures representing energetically favorable poses of the ligand molecule in the binding site, out of their several configurations based on energy ranking defined by the scoring function. From the four poses, the pose of the ligand molecule in which the hydroxamate group showed a chelation to the zinc ion was collected. The RMSD vs. time plots shown in Fig. S1 to S6 (ESI†) attest to the stability of the docked complexes. Further, structures obtained from each of the molecular dynamics trajectories were processed through BapplZ scoring function and the average binding energies were calculated for Batimastat binding to different MMPs. Fig. S7 to S12 (ESI†) show the convergence of the binding energy values. We further plotted a correlation between the experimental and predicted binding free energies for Batimastat binding to different MMPs (Fig. 3). An efficient docking algorithm, a correct charge assignment protocol, a rigorous molecular dynamics simulation study and an accurate scoring function contribute to structural, dynamic and thermodynamic rationalization of experimental inhibition data, as evident from a high correlation coefficient between the experimental and predicted binding free energies shown in Fig. 3, thus demonstrating the efficiency of the computational pathway followed. The molecular dynamics simulation studies incorporated the structural and dynamic effects of the ligand which cannot be disclosed by docking studies alone.
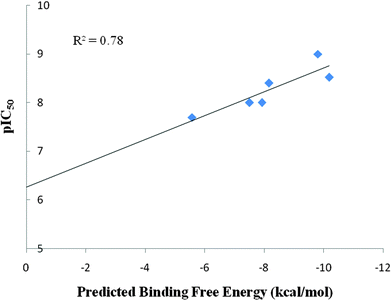 |
| Fig. 3 Correlation between experimental pIC50 and predicted binding free energies (in kcal mol−1) for the binding of Batimastat with MMP-1, MMP-2, MMP-3, MMP-8, MMP-9, MMP-13. | |
For Batimastat the inhibitor was considerably less specific against any MMP. One of the major reasons for the lack of efficacy of MMP inhibitors is due to the reason that they are broad spectrum. Design of specific MMP inhibitors is thus interesting and challenging. In MMPs, in addition to the catalytic site, there are other sites designated as S1, S2, S3 and S1′, S2′ S3′, shown1 in Fig. 4, along with the catalytic region. The main site for substrate recognition is the specificity pocket S1′ as the site differs in shape and size among different MMPs. Moreover, due to flexibility of the MMP binding pockets74–78 predicting the binding free energies in silico has been difficult. Though structural information from the X-ray structures can be used to design inhibitors with high affinity and selectivity towards a given MMP, the flexibility of the MMP binding pockets limits an accurate estimation of binding free energies against a given MMP.
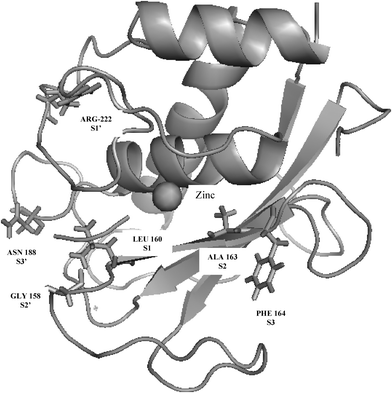 |
| Fig. 4 S1, S2, S3 and the S1′, S2′, S3′ sites in matrix metalloproteinases (pdb id: 2OY2). | |
With the above concerns in focus, we further extended our study to the design of specific inhibitors against diverse MMPs dealt with above. A sequence alignment was performed on the MMPs using Clustal W program.79 The specificity loop regions of different MMPs are shown in Fig. 5. While designing inhibitor molecules, amino acids showing a variation in the specificity loop region need to be considered to take care of the specificity issue.
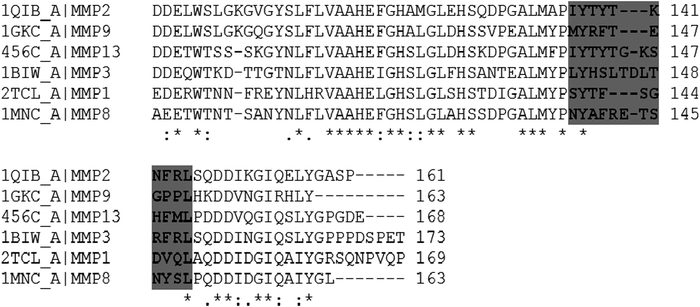 |
| Fig. 5 Sequence alignment of MMPs. The highlighted region shows the amino acids lying in the specificity loop region. | |
Some design principles for MMP inhibitors
We designed a few specific molecules against different MMPs with a special focus on MMP-2, MMP-3, MMP-8, MMP-9 and MMP-13 whose over expression is associated with various diseases. The molecules were designed with the main goal to fit in the S1′ groove adjacent to the catalytic site of the MMPs to come up with specific hit molecules. The docked structures were subjected to molecular dynamics simulations using the protocol detailed in the methodology section for 10 ns to consider the flexibility/dynamics of the ligand and the active site residues of the target along with the explicit solvent, salt effects and also to account for the induced fit effects. After running the simulations, the docked structures obtained during the last four ns simulation runs were subjected to BapplZ calculation43 and an average binding free energy was calculated. To check on the affinity versus specificity of the molecules designed against each class of MMP, a cross docking study was also performed. Table 1 shows the potential ligands obtained against different MMPs and an affinity versus specificity matrix for the molecules designed.
Table 1 Affinity versus specificity matrix of the designed molecules (the binding energies predicted by Sanjeevini web server are reported in kcal mol−1)
|
Molecule |
MMP |
Molecule 2 |
Molecule 3 |
Molecule 8 |
Molecule 9 |
Molecule 13 |
MMP1 |
−2.05 |
−10.93 |
−3.44 |
−9.52 |
−10.92 |
MMP2 |
−10.67
|
−7.09 |
−0.68 |
−8.18 |
−9.748 |
MMP3 |
−7.09 |
−16.64
|
−9.5 |
−12.94 |
−9.84 |
MMP8 |
−6.25 |
0.82 |
−10.45
|
−12.09 |
−10.42 |
MMP9 |
−7.89 |
−9.64 |
−1.15 |
−15.55
|
−8.71 |
MMP13 |
−1.21 |
−11.26 |
−1.07 |
−8.12 |
−15.09
|
The scaffolds of the molecules designed are shown in Fig. 6–10 against MMP-2, MMP-3, MMP-8, MMP9 and MMP-13 respectively. The nomenclature to the designed molecules was given as per the MMP target for which it was designed. Molecule 2, molecule 3, molecule 8, molecule 9 and molecule 13 correspond to MMP-2, MMP-3, MMP-8, MMP-9 and MMP-13, respectively.
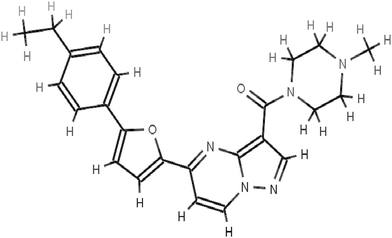 |
| Fig. 6 Scaffold of molecule 2 designed against MMP-2. | |
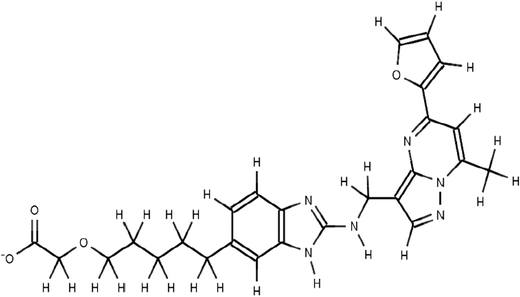 |
| Fig. 7 Scaffold of molecule 3 designed against MMP-3. | |
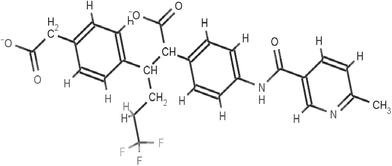 |
| Fig. 8 Scaffold of molecule 8 designed against MMP-8. | |
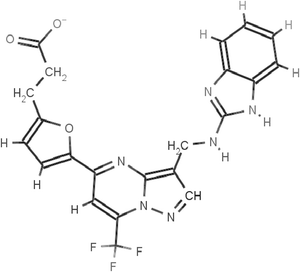 |
| Fig. 9 Scaffold of molecule 9 designed against MMP-9. | |
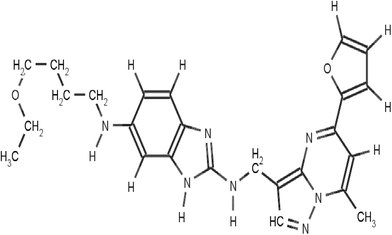 |
| Fig. 10 Scaffold of molecule 13 designed against MMP-13. | |
There is considerable variation in the amino acids defining the shape and size of the S1′ site. The MMP-1 has a shallow S1′ pocket, MMP-2, MMP-8 and MMP-9 have intermediate depths and the MMP-3 and MMP-13 have deep S1′ pockets as can be seen in Fig. S13–S17 (ESI†). In MMP-1, Arg 114 at the start of the catalytic helix makes the S1′ site very shallow, providing scope for designing molecules specific for other MMPs and not against MMP-1, as they are reported to be involved in musculo-skeletal pains on getting inhibited by broad spectrum MMP inhibitors.43 The RASPD module of Sanjeevini54 was used to obtain the initial hit molecules against the target MMPs from a million molecule database of small organic molecules (public version of zinc database80). The module sets up a QSAR type equation to find complementarity between the physico-chemical properties of the binding site of the protein and the candidate ligand molecule to estimate a binding energy between them. The molecule with ZINCID 20601870 (IUPAC name: 5-(2-furyl)-N-[1-[2-(1-piperidyl)ethyl]benzimidazol-2-yl]-7-(trifluoromethyl)pyrazolo[1,5-a]pyrimidi) was obtained as the top hit for all the MMPs as the binding site of the MMPs are very homologous to each other. Our attention was engaged by the presence of the carbonyl group which can show potential interaction with the zinc and the aromatic groups with suitable curvatures to show interactions with the S1′ site. However, since the S1′ site varies in the amino acid sequence and overall architecture, the starting scaffold of the molecule was modified through a structure based design approach targeting the S1′ site along with the catalytic/binding site to bring in affinity as well as specificity of the molecules designed for each class of MMPs.
Thus, the main focus while designing the inhibitors for the respective MMPs was to optimize the length of the molecule designed according to the differences in the depth of the S1′ pocket for different MMP subtypes. The fragment of the molecule penetrating the S1′ site is referred to as the P1′ fragment.1 An inhibitor molecule that can show a chelation with the catalytic zinc ion and protrude into the S1′ site of MMP-2, which is lined by the hydrophobic side chains81 of Leu-83, Phe-115, Leu-116, Val-117, Ile-141 and Phe-148, was designed. We focused on designing an expected fragment targeting the S1′ site, which was elongated and had a hydrophobic group along with a zinc chelating group. The inhibitor molecule 2, designed for MMP-2 fitted well in the S1′ hydrophobic pocket facilitated by the long hydrophobic chains and showed electrostatic interaction with the zinc ion facilitated by the oxygen atom as shown in Fig. S13 (ESI†). During the molecular dynamics run on the MMP-2 inhibitor docked complex, conformational changes occurred suggesting the aromatic ring of Phe-148 moving towards the inhibitor molecule to make interactions and the Arg-149 rotating to accommodate changes in Phe-148 (Fig. S13, ESI†). The above study suggests that molecules with a longer alkyl chain protruding towards the cleft formed by the movement of Phe-148 show better affinity against MMP-2. Moreover, while designing molecule 2 the structure and amino acid sequence of the other MMPs were critically examined to come up with a molecule showing high affinity as well as specificity against MMP-2. The S1′ loop of MMP-2 is two amino acids shorter than MMP-8 due to which there is an extra hump at the bottom of the loop before converging with MMP-2. The non-conservative substitution Thr-143 in MMP-2 or Ala-240 in MMP-8 and Thr-145 in MMP-2 or Arg-243 in MMP-8 throw in differences in the inhibitory pattern of MMP-2 and MMP-8. In the uninhibited MMP-882 residue Arg-243 obstructs the S1′ groove due to which molecule 2 with a longer alkyl chain failed to fit in the S1′ groove of MMP-8. Similarly, inhibitors with long substituents protruding into the S1′ inhibit MMP-2 (Fig. S13, ESI†) but fail to inhibit MMP-9 due to occlusion by1,83 Arg 424 (Fig. S16, ESI†). A structural alignment of MMP-2 and MMP-9 shows an equivalent Pro-429 and Pro-430 in MMP-9 corresponding to Phe-148 and Arg-149 in MMP-2 which does not offer enough conformational flexibility to accommodate molecules with longer alkyl chains in MMP-9. Molecule 2 showed lesser affinity against MMP-3 and MMP-13 due to a longer S1′ groove of the same as evident from Table 1 making the molecule specific to MMP-2.
The S1′ channel of MMP-3 includes Leu-197, Val-198, His-201, Leu-218, Tyr-220, along with S1′ wall residues as highlighted in Fig. 5. While designing molecule 3 the length of the P1′ group was increased in comparison to molecule 2 to make it more specific towards MMPs with a deeper S1′ site as in MMP-3 in comparison with MMP-1, MMP-2, MMP-8 and MMP-9. A carboxylate group was introduced at the terminal towards the floor of the S1′ site at the end of the hydrophobic chain which could show hydrogen bonding interaction with Arg 233 in MMP-3. A possible alternative would have been an electropositive group showing interaction with Asp-228. However, a carboxylate group interacting with arginine can be considered to have more conformational flexibility than Asp-228. This interaction would maintain a tight fitting of molecule 3 in the S1′ site along with the electrostatic interaction of the N1 atom of molecule 3 with the zinc ion (Fig. S14, ESI†). The docked complex was subjected to molecular dynamics simulations. The Arg-233 (Fig. S14, ESI†) during simulation contributed to an induced fit interaction towards the carboxylate group of molecule 3 along with a good hydrophobic fit in the S1′ groove and an electrostatic interaction with the zinc ion. A structural superimposition of MMP-3 and MMP-13 shows an equivalent Met-232 to Arg-233 in MMP-13 providing a scope for designing specific inhibitors against MMP-3 (Table 1).
Molecule 8 was designed to show high affinity against MMP-8. The key residues in MMP-8 to bring in affinity as well as specificity can be accredited to Arg-243 and Asn-239 (Fig. S15, ESI†). The orientation of the carboxylate group in molecule 8, which can interact with Arg-243 and an electronegative fluorine atom to show hydrogen bonding with the Asn-239 (Fig. S15, ESI†), was designed along with an N1 atom of molecule 8 chelating with the zinc ion. These residues vary sequentially as well as structurally in other MMPs (Fig. 5) in the S1′ region. The unique conformation of molecule 8 resulting due to the carboxylate groups and the trifluoromethyl group at the terminal of molecule 8 made the above residues acquiescent to interact favorably with MMP-8 (Fig. S15, ESI†) and poorly with other MMPs due to steric clashes.
The S1′ pocket of MMP-9 consists of Asp-185–Leu-188 and Pro-421–Tyr-423. The S1′ wall comprises1,84,85 Leu-188, Leu-397, Val-398, His-401, Leu-418, Met-422 and Tyr-423. The catalytic sites of MMP-2 and MMP-9 show high degree of similarity but with variations in the S1′ loop region. The orientation of the equivalent residues Arg424 and Thr 426 in the S1′ pocket of MMP-9 and MMP-2 respectively causes difference in the overall shape and size of the S1′ pocket. Inhibitors with long substituents protruding into the S1′ inhibit MMP-2 (Fig. S13, ESI†) but fail to inhibit MMP-9 due to occlusion by Arg-424 and vice versa (Fig. S16, ESI†). Molecule 9 designed specifically to bind MMP-9 showed lesser binding to MMP-2, MMP-3, MMP-8 and MMP-13 as the molecule did not make a way inside the groove due to steric clashes and failed to show favorable interaction with the equivalent residues in the S1′ region. Molecule 9 comprised of three sub-fragments. The fragment with a zinc chelating N atom (Fig. S16, ESI†), and a P1′ fragment with a carboxylate group at the terminal showing hydrogen bonding with Arg 424 with an aromatic linker carrying a trifluoromethyl group maintains a good fit of molecule 9 at the catalytic and S1′ sub site of MMP-9. Molecule 2, molecule 9 and molecule 8 showed lesser affinity against MMP-3 and MMP-13 due to a longer S1′ groove of the same as evident from Table 1.
The specificity loop of MMP-13 shows flexibility86 when it is in contact with inhibitors which is critical for binding. The meticulous conformation of S1′ loop defines its shape and size. The conformational restriction appears obvious for the other MMPs with shorter specificity loop regions in MMP-1, MMP-2, MMP-8 and MMP-9. However, for MMPs with similar or longer specificity loops as in MMP-3, the nature of the residues in the specificity loop region (Fig. 5) in MMP-13 and their conformations are the structural determinants for high MMP-13 selectivity. Molecule 13 (Fig. S17, ESI†) was designed to show specificity against MMP-13 by fitting well in the S1′ groove with a hydrogen bond interaction with Thr-245 and Thr-247 in comparison to MMP-3 which has an equivalent Leu-226 which restricts conformational flexibility to the equivalent residues. Gly-248 in MMP-13 provides conformational flexibility in this position allowing the neighboring residues Thr-245, Tyr-246, Thr-247 to interact with the inhibitor molecules by providing room for induced fit interaction with the ligand molecule.
The fragments added or modified in the starting hit molecule obtained from the RASPD module, was not inspired from any molecule already in use for the MMPs but was designed to come up with important pharmocophoric suggestions for each MMPs studied. The functional groups/fragments added were varied according to the length of the S1′ site and the key residues discussed in different MMPs in order to account for the specificity and affinity of the designed molecules. The quality of chemical collection increases if the compounds have drug like properties. A well known rule of five developed by Lipinski has been a milestone of filtering techniques. The rule identifies compounds that are likely to exhibit good bioavailability. The molecular weight, log
P, number of hydrogen bond donor and number of hydrogen bond acceptor atoms were calculated using the Lipinski module of Sanjeevini web server. The values are reported in Table S3 (ESI†) signifying the drug like properties of the molecules designed. Additionally, synthesizability of the proposed molecules albeit with a large number of synthetic steps has been confirmed. Further synthesis of these molecules or similar molecules satisfying the phramacophoric/geometric criteria to interact with the key residues highlighted in different MMPs and further in vitro experimental test is conceivable in near future.
Free energy analyses
The semi empirical methodology (BapplZ/MD) adopted has been reported to accurately predict the electrostatics, van der Waals, hydrophobicity and loss in conformational entropy of protein side chains upon ligand binding and an approach to model the interactions of zinc with the other atoms. The MMPs belong to a highly flexible class of proteins especially the S1′ site which is considered to bring in specificity for the hit molecules while binding against different MMPs. To fully understand the role of structural adaptation in cases where flexibility could be an important issue i.e. when significant structural changes in the target and the ligand are expected upon binding, one must perform an additional initial state (before binding) versus the final state (after binding) analysis. Thus a post facto MMGBSA (modified generalized Born solvent accessibility) analysis of the trajectories of the bound protein–inhibitor complex and the unbound protein and ligand was performed to further understand the energetic consequences of flexibility and structural adaptation.74,75 Here, the net binding free energy is considered to be a sum of the free energy changes due to rotational translational entropy losses, deformation or adaptation expense, van der Waals interactions between the protein and ligand, and net electrostatics and cavitation term. The net electrostatics includes the Coulomb interaction between protein and the ligand and the electrostatic component of solvation. The thermodynamic cycle to obtain the standard free energy of complex formation comprising the above energy components has been discussed in Fig. 2 of Jayaram et al. The modified MMGBSA method was applied to the docked structures collected over the last 4 ns trajectories for the molecules designed and an averaging was done to study the various components contributing towards binding free energy. The average binding free energy and the various components contributing towards the binding free energy for the docked molecule 2, molecule 3, molecule 8, molecule 9 and molecule 13 against their respective MMPs have been shown in Fig. S18 (ESI†). The direct electrostatics, van der Waals interactions and the hydrophobic interactions favour the binding of protein and the designed molecules. The electrostatic and the van der Waals component of desolvation, the deformation and the rotational translational entropy disfavour complexation. Overall, the MMGBSA analyses indicate that despite the flexibility of the target proteins, the designed molecules retain their binding affinity. Different terms or descriptors are generally employed in any scoring function to obtain a binding free energy estimate of the docked protein–ligand complexes. Both empirical (BapplZ) and the MMSGSA methodologies have been adopted in the current work for addressing different components of binding generating a hit molecule with high affinity and specificity against the target MMPs. While the overall picture remains similar to empirical energy function analyses, the overall binding free energies being negative suggesting a hit against the corresponding MMPs, the MD trajectory analyses take into account structural adaptation of the interacting molecules and role of solvation/desolvation effects in binding more explicitly. The magnitudes of the components provide a good indicator to the order of importance of the energy components favourable to binding and prove valuable in design efforts.
The docking and scoring methodology adopted in the current study including a QM/MM based method to derive charges on the docked protein–ligand complex was compared with some of the previously reported work in the literature where a binding free energy calculation was done using Autodock software on 16 known inhibitors87 of MMPs. The predicted binding free energies of the top ranked docked structure reported by Autodock and the Sanjeevini software are shown in Table S2 (ESI†). A correlation coefficient r of 0.59 and 0.87 were obtained by Autodock and Sanjeevini software respectively between the experimental and the predicted binding free energies. Molecules showing activity against some of these MMPs like MMP-9 have been reported using pharmacophore based approaches with a focus on the S1 subsite.88 In the present study, a protein structure based approach is used to design inhibitors against the five classes of MMPs. Selectivity being the major issue, a protein structure based approach using a robust and high accuracy docking and scoring methodology followed by a molecular dynamics simulation study has an edge in comparison to the pharmacophore based design of MMP inhibitors. Moreover a comparison between the S1′ site followed by a cross docking and scoring approach (Table 1) between different MMPs as carried out in the present study versus a consideration of the S1 site alone of a particular MMP as done previously (Kalva, Azhagiya et al. 201488) can help in the design of more specific inhibitors against each class of the MMPs.
Conclusions
Rational design of specific MMP inhibitors has been challenging due to various isomorphs belonging to the above family of enzymes having very flexible binding sites. The docking and scoring module of Sanjeevini used in the current work was successful in giving a high correlation between the experimental and predicted binding energy for MMP inhibitors with known crystal structures. A high accuracy docking and scoring methodology, an accurate QM/MM approach to calculate charge on the docked protein ligand complex followed by molecular dynamics simulations, gave a high correlation between the experimental and predicted binding energy for a known MMP inhibitor (Batimastat) with an unknown crystal structure. An MMGBSA analysis accounts for various computational challenges like receptor flexibility and induced fit effects. Further, a comparison between the S1′ site followed by a cross docking and scoring approach (Table 1) between different MMPs as carried out in the present study versus a consideration of the S1 site alone of a particular MMP can help in the design of more specific inhibitors against each class of MMPs. Certain key residues are identified in S1′ sites of different MMPs to design molecules with suitable fragments to hold a stable coordination with the zinc ion and a proper fit in the S1′ site to address the affinity and specificity issues.
Acknowledgements
This work is supported by grants from the Department of Biotechnology, Govt. of India. Tanya Singh is a recipient of Senior Research Fellowship from Council of Scientific & Industrial Research, Govt. of India. Adekoya Olayiwola acknowledges the support from Norwegian research council. The authors thank Prof. N. G. Ramesh for commenting on the synthesizability of the molecules designed.
References
- J. W. Skiles, N. C. Gonnella and A. Y. Jeng, Curr. Med. Chem., 2004, 11, 2911–2977 CrossRef CAS.
- T. Cawston, Mol. Med. Today, 1998, 4, 130–137 CrossRef CAS.
- L. Blavier, P. Henriet, S. Imren and Y. A. Declerck, Ann. N. Y. Acad. Sci., 1999, 878, 108–119 CrossRef CAS PubMed.
- C. Chang and Z. Werb, Trends Cell Biol., 2001, 11, S37–S43 CrossRef CAS.
- J. Hu, P. E. Van den Steen, Q. X. Sang and G. Opdenakker, Nat. Rev. Drug Discovery, 2007, 6, 480 CrossRef CAS PubMed.
- V. W. Yong, Nat. Rev. Neurosci., 2005, 6, 931–944 CrossRef CAS PubMed.
- D. L. Weinstat-Saslow, V. S. Zabrenetzky, K. vanHoutte, W. A. Frazier, D. D. Roberts and P. S. Steeg, Cancer Res., 1994, 54, 6504–6511 CAS.
- M. Whittaker, C. D. Floyd, P. Bowen and A. J. H. Gearing, Chem. Rev., 1999, 99, 2735–2776 CrossRef CAS PubMed.
- K. Kessenbrock, V. Plaks and Z. Werb, Cell, 2010, 141, 52–67 CrossRef CAS PubMed.
- V. C. Ardi, T. A. Kupriyanova, E. I. Deryugina and J. P. Quigley, Proc. Natl. Acad. Sci. U. S. A., 2007, 104, 20262–20267 CrossRef CAS PubMed.
- M. Egeblad and Z. Werb, Nat. Rev. Cancer, 2002, 2, 161–174 CrossRef CAS PubMed.
- J. Hu, P. E. V. Steen, Q. A. Sang and G. Opdenakker, Nat. Rev. Drug Discovery, 2007, 6, 480–498 CrossRef CAS PubMed.
- P. Elkington, T. Shiomi, R. Breen, R. K. Nuttall, C. A. Ugarte-Gil, N. F. Walker, L. Saraiva, B. Pedersen, F. Mauri, M. Lipman, D. R. Edwards, B. D. Robertson, J. D'Armiento and J. S. Friedland, J. Clin. Invest., 2011, 121, 1827–1833 CAS.
- G. Murphy, V. Knäuper, S. Atkinson, G. Butler, W. English, M. Hutton, J. Stracke and I. Clark, Arthritis Res., 2002, 4(suppl 3), S39–S49 CrossRef.
- B. Fingleton, Curr. Pharm. Des., 2007, 13, 333–346 CrossRef CAS.
- H. Liu, Y. Kato, S. A. Erzinger, G. M. Kiriakova, Y. Qian, D. Palmieri, P. S. Steeg and J. E. Price, BMC Cancer, 2012, 12, 583 CrossRef CAS PubMed.
- Q. W. Cai, J. Li, X. Li, J. Wang and Y. Huang, Mol. Med. Rep., 2012, 5(6), 1438–1442 CAS.
- Y. Kawasaki, Z. Z. Xu, X. Wang, J. Y. Park, Z. Y. Zhuang, P. H. Tan, Y. J. Gao, K. Roy, G. Corfas, E. H. Lo and R. R. Ji, Nat. Med., 2008, 14, 331–336 CrossRef CAS PubMed.
- L. Y. Liu, H. Zheng, H. L. Xiao, Z. Y. She, S. M. Zhao, Z. L. Chen and G. M. Zhou, Neurosci. Lett., 2008, 434, 155–159 CrossRef CAS PubMed.
- V. I. Shubayev and R. R. Myers, Brain Res., 2008, 855, 83–89 CrossRef.
- R. Ramer and B. Hinz, J. Natl. Cancer Inst., 2008, 2, 59–69 CrossRef PubMed.
- D. Amantea, M. T. Corasaniti, N. B. Mercuri, G. Bernardi and G. Bagetta, Neuroscience, 2008, 152, 8–17 CrossRef CAS PubMed.
- C. Sommer, C. Schmidt, A. George and K. V. Toyka, Neurosci. Lett., 1997, 237, 45–48 CrossRef CAS.
- L. J. Noble, F. Donovan, T. Igarash, S. Goussev and Z. Werb, J. Neurosci., 2002, 1, 7526–7535 Search PubMed.
- R. Pannu, D. K. Christie, E. Barbosa, I. Singh and A. K. Singh, J. Neurochem., 2007, 101, 182–200 CrossRef CAS PubMed.
- J. C. Fleming, M. D. Norenberg, D. A. Ramsay, G. A. Dekaban, A. E. Marcillo, A. D. Saenz, M. Pasqale-Styles, W. D. Dietrich and L. C. Weaver, Brain, 2008, 129, 3249–3269 CrossRef PubMed.
- M. Fujimoto, Y. Takagi, T. Aoki, M. Hayase, T. Marumo, M. Gomi, M. Nishimura, H. Kataoka, N. Hashimoto and K. Nozaki, J. Cereb. Blood Flow Metab., 2008, 28, 1674–1685 CrossRef CAS PubMed.
- A. Vilalta, J. Sahuquillo, A. Rosell, M. A. Poca, M. Riveiro and J. Montaner, Intensive Care Med., 2008, 34, 1384–1392 CrossRef CAS PubMed.
- J. S. Truettner, O. F. Alonso and D. W. Dalton, J. Cereb. Blood Flow Metab., 2005, 11, 1505–1516 CrossRef PubMed.
- E. Sunami, N. Tsuno, T. Osada, S. Saito, J. Kitayama, S. Tomozawa, T. Tsuruo, Y. Shibata, T. Muto and H. Nagawa, Oncologist, 2000, 5, 108–114 CrossRef CAS PubMed.
- W. F. Rodrigues, M. F. Madeira, T. A. da Silva, J. T. Clemente-Napimoga, C. B. Miguel, V. J. Dias-da-Silva, O. Barbosa-Neto, A. H. Lopes and M. H. Napimoga, Br. J. Pharmacol., 2012, 165, 2140–2151 CrossRef CAS PubMed.
- A. B. Wysocki, A. O. Kusakabe, S. Chang and T. L. Tuan, Wound Rep. Reg., 1997, 7, 154–165 CrossRef.
- R. L. Warner, N. Bhagavathula, K. C. Nerusu, H. Lateef, E. Younkin, K. J. Johnson and J. Varani, Exp. Mol. Pathol., 2004, 76, 189–195 CrossRef CAS PubMed.
- S. D. Sagel, R. K. Kapsner and I. Osberg, Pediatr. Pulmonol., 2005, 39, 224–232 CrossRef PubMed.
- H. Yang, Y. Dai, H. Dong, D. Zang, Q. Liu, H. Duan, Y. Niu, P. Bin and Y. Zheng, Toxicol. In Vitro, 2011, 25, 1638–1643 CrossRef CAS PubMed.
- N. Shibataa, T. Ohnumaa, S. Higashia, C. Usuia, T. Ohkuboa, A. Kitajimaa, A. Uekib, M. Nagaoc and H. Araia, Neurobiol. Aging, 2005, 26, 1011–1014 CrossRef PubMed.
- A. Kofla-Dlubacz, M. Matusiewicz, M. Krzystek-Korpacka and B. Iwanczak, Dig. Dis. Sci., 2012, 57, 706–712 CrossRef CAS PubMed.
- M. S. Kumar, G. Vamsi, R. Sripriya and P. K. Sehgal, J. Periodontol., 2006, 77, 1803–1808 CrossRef CAS PubMed.
- H. M. Berman, J. Westbrook, Z. Feng, G. Gilliland, T. N. Bhat, H. Weissig, I. N. Shindyalov and P.
E. Bourne, Nucleic Acids Res., 2000, 28, 235–242 CrossRef CAS PubMed.
- G. Tu, W. Xu, H. Huang and S. Li, Curr. Med. Chem., 2008, 15, 1388–1395 CrossRef CAS.
- J. F. Fisher and S. Mobashery, Cancer Metastasis Rev., 2006, 25, 115–136 CrossRef CAS PubMed.
- B. Fingleton, Curr. Pharm. Des., 2007, 13, 333–346 CrossRef CAS.
- T. Jain and B. Jayaram, Proteins: Struct., Funct., Bioinf., 2007, 67, 1167–1178 CrossRef CAS PubMed.
- T. Dudev and C. Lim, J. Phys. Chem. B, 2009, 113, 11754–11764 CrossRef CAS PubMed.
- D. R. Langley, A. W. Walsh, C. J. Baldick, B. J. Eggers, R. E. Rose, S. M. Levine, A. J. Kapur, R. J. Colonno and D. J. Tenney, J. Virol., 2007, 81, 3992–4001 CrossRef CAS PubMed.
- M. Rouffet, C. Denhez, E. Bourget, F. Bohr and D. Guillaume, Org. Biomol. Chem., 2009, 7(18), 3817–38125 CAS.
- O. Kleifeld, L. P. Kotra, D. C. Gervasi, S. Brown, M. M. Bernardo, R. Fridman, S. Mobashery and I. Sagi, J. Biol. Chem., 2001, 276(20), 17125–17131 CrossRef CAS PubMed.
- X. Hu and W. H. Shelver, J. Mol. Graphics Modell, 2003, 22(2), 115–126 CrossRef CAS.
- D. A. Pearlman, D. A. Case, J. W. Caldwell, W. S. Ross, J. E. Cheathem III, S. DeBolt, D. Ferguson, G. Seibe and P. Kollman, Comput. Phys. Commun., 1995, 91, 1–41 CrossRef CAS.
- I. Giangreco, G. Lattanzi, O. Nicolotti, M. Catto, A. Laghezza, F. Leonetti, A. Stefanachi and A. Carotti, PLoS One, 2011, 6, 1 Search PubMed.
- A. Jakalian, B. L. Bush, D. B. Jack and C. I. Bayly, J. Comput. Chem., 2004, 21, 132–146 CrossRef.
- J. Wang, R. M. Wolf, J. W. Caldwell, P. A. Kollman and D. A. Case, J. Comput. Chem., 2004, 25, 1157–1174 CrossRef CAS PubMed.
- W. D. Cornell, P. Cieplak, C. I. Bayly, I. R. Gould and K. M. Merz, J. Am. Chem. Soc., 1995, 117, 5179–5197 CrossRef CAS.
- B. Jayaram, T. Singh, G. Mukherjee, A. Mathur, S. Shekhar and V. Shekhar, BMC Bioinf., 2012, 13, S7 CrossRef PubMed.
- T. Singh, D. Biswas and B. Jayaram, J. Chem. Inf. Model., 2011, 51, 2515–2527 CrossRef CAS PubMed.
- A. Gupta, A. Gandhimathi, P. Sharma and B. Jayaram, Protein Pept. Lett., 2007, 14, 632–646 CrossRef CAS.
- T. Jain and B. Jayaram, FEBS Lett., 2005, 579, 6659–6666 CrossRef CAS PubMed.
- A. Soni, K. M. Pandey, P. Ray and B. Jayaram, Curr. Pharm. Des., 2013, 19, 4687–4700 CrossRef CAS.
- S. A. Shaikh, T. Jain, G. Sandhu, N. Latha and B. Jayaram, Curr. Pharm. Des., 2007, 13, 3454–3470 CrossRef CAS.
- A. Warshel and M. Levitt, J. Mol. Biol., 1976, 103, 227–249 CrossRef CAS.
-
M. J. Frisch, G. W. Trucks, H. B. Schlegel, G. E. Scuseria, M. A. Robb, J. R. Cheeseman, G. Scalmani, V. Barone, B. Mennucci, G. A. Petersson, H. Nakatsuji, M. Caricato, X. Li, H. P. Hratchian, A. F. Izmaylov, J. Bloino, G. Zheng, J. L. Sonnenberg, M. Hada, M. Ehara, K. Toyota, R. Fukuda, J. Hasegawa, M. Ishida, T. Nakajima, Y. Honda, O. Kitao, H. Nakai, T. Vreven, J. A. Montgomery Jr., J. E. Peralta, F. Ogliaro, M. Bearpark, J. J. Heyd, E. Brothers, K. N. Kudin, V. N. Staroverov, R. Kobayashi, J. Normand, K. Raghavachari, A. Rendell, J. C. Burant, S. S. Iyengar, J. Tomasi, M. Cossi, N. Rega, N. J. Millam, M. Klene, J. E. Knox, J. B. Cross, V. Bakken, C. Adamo, J. Jaramillo, R. Gomperts, R. E. Stratmann, O. Yazyev, A. J. Austin, R. Cammi, C. Pomelli, J. W. Ochterski, R. L. Martin, K. Morokuma, V. G. Zakrzewski, G. A. Voth, P. Salvador, J. J. Dannenberg, S. Dapprich, A. D. Daniels, O. Farkas, J. B. Foresman, J. V. Ortiz, J. Cioslowski and D. J. Fox, Gaussian, Inc., Wallingford, CT, 2009.
- R. H. Stote and M. Karplus, Proteins: Struct., Funct., Genet., 1995, 23, 12–31 CrossRef CAS PubMed.
- P. Kalra, T. V. Reddy and B. Jayaram, J. Med. Chem., 2001, 44, 4325–4338 CrossRef CAS PubMed.
- H. W. Horn, W. C. Swope, J. W. Pitera, J. D. Madura, T. J. Dick, G. L. Hura and T. Head-Gordon, J. Chem. Phys., 2004, 120, 9665–9678 CrossRef CAS PubMed.
- H. J. C. Berendsen, J. P. M. Postma, W. F. van Gunsteren, A. DiNola and J. R. Haak, J. Chem. Phys., 1984, 81, 3684–3690 CrossRef CAS PubMed.
- R. Lavery, K. Zakrzewska, D. L. Beveridge, T. C. Bishop, D. A. Case, T. Cheatham III, S. Dixit, B. Jayaram, F. Lankas, C. Laughton, J. H. Maddocks, A. Michon, R. Osman, M. Orozco, A. Perez, T. Singh, N. Spackova and J. Sponer, Nucleic Acids Res., 2009, 38, 299–313 CrossRef PubMed.
- S. A. Shaikh, S. R. Ahmed and B. Jayaram, Arch. Biochem. Biophys., 2004, 429, 81–99 CrossRef CAS PubMed.
- B. Jayaram, K. Mcconnell, S. B. Dixit and D. L. Beveridge, J. Comput. Chem., 2002, 23, 1–14 CrossRef CAS PubMed.
- B. Jayaram, K. J. McConnell, S. B. Dixit and D. L. Beveridge, J. Comput. Phys., 1999, 151, 333–357 CrossRef CAS.
- P. A. Greenidge, C. Kramer, J. Mozziconacci and R. M. Wolf, J. Chem. Inf. Model., 2013, 53, 201–209 CrossRef CAS PubMed.
- S. Wong, R. E. Amaro and J. A. McCammon, J. Chem. Theory Comput., 2009, 5, 422–449 CrossRef CAS PubMed.
- S. A. Shaikh and B. Jayaram, J. Med. Chem., 2007, 50, 2240–2244 CrossRef CAS PubMed.
- G. Mukherjee and B. Jayaram, Phys. Chem. Chem. Phys., 2013, 15, 9107–9116 RSC.
- J. D. Durrant, C. F. de Oliveira and J. A. McCammon, J. Mol. Recognit., 2010, 23, 173–182 CAS.
- R. E. Amaro, R. Baron and J. A. McCammon, J. Comput.-Aided Mol. Des., 2008, 22, 693–705 CrossRef CAS PubMed.
- S. A. Adcock and J. A. McCammon, Chem. Rev., 2006, 106, 1589–1615 CrossRef CAS PubMed.
- M. Berynskyy and R. C. Wade, Curr. Phys. Chem., 2013, 3, 27–35 CrossRef CAS.
- D. B. Kokh, R. C. Wade and W. Wolfgang, Wiley Interdiscip. Rev.: Comput. Mol. Sci., 2011, 1, 298–314 CrossRef CAS.
-
http://www.ebi.ac.uk/Tools/msa/clustalw2/
.
-
http://zinc.docking.org/
.
- Y. Feng, J. J. Likos, L. Zhu, H. Woodward, G. Munie, J. J. McDonald, A. M. Stevens, C. P. Howard, G. A. De Crescenzo, D. Welsch, H. S. Shieh and W. C. Stallings, Biochim. Biophys. Acta, 2002, 1598, 10–23 CrossRef CAS.
- I. Bertini, V. Calderone, M. Fragai, C. Luchinat, M. Maletta and K. J. Yeo, Angew. Chem., Int. Ed., 2006, 45, 7952–7955 CrossRef CAS PubMed.
- J. W. Skiles, N. C. Gonnella and A. Y. Jeng, Curr. Med. Chem., 2004, 11, 2911–2977 CrossRef CAS.
- S. Rowsell, P. Hawtin, C. A. Minshull, H. Jepson, S. M. V. Brockbank, D. G. Barratt, A. M. Slater, W. L. McPheat, D. Waterson, A. M. Henney and R. A. Pauptit, J. Mol. Biol., 2002, 319, 173–181 CrossRef CAS.
- P. A. Elkins, Y. S. Ho, W. W. Smith, C. A. Janson, K. J. D'Alessio, M. S. McQueney, M. D. Cummings and A. M. Romanic, Acta Crystallogr., Sect. D: Biol. Crystallogr., 2002, 58, 1182–1192 CrossRef PubMed.
- B. Lovejoy, A. R. Welch, S. Carr, C. Luong, C. Broka, R. T. Hendricks, J. A. Campbell, K. A. Walker, R. Martin, H. Van Wart and M. F. Browner, Nat. Struct. Biol., 1999, 6, 217–221 CrossRef CAS PubMed.
- X. Hu and W. H. Shelver, J. Mol. Graphics Modell, 2003, 22(2), 115–126 CrossRef CAS.
- S. Kalva, E. R. Azhagiya Singam, V. Rajapandian, L. M. Saleena and V. Subramanian, J. Mol. Graphics Modell, 2014, 49C, 25–37 CrossRef PubMed.
Footnote |
† Electronic supplementary information (ESI) available. See DOI: 10.1039/c5mb00003c |
|
This journal is © The Royal Society of Chemistry 2015 |