DOI:
10.1039/C4MB00741G
(Paper)
Mol. BioSyst., 2015,
11, 1004-1011
An integrated analysis of differential miRNA and mRNA expressions in human gallstones†
Received
23rd December 2014
, Accepted 20th January 2015
First published on 21st January 2015
Abstract
Gallstone disease, including cholesterol precipitation in bile, increased bile salt hydrophobicity and gallbladder inflammation. Here, we investigated miRNA and mRNA involved in the formation of gallstones, and explored the molecular mechanisms in the development of gallstones. Differentially expressed 17 miRNAs and 525 mRNA were identified based on Illumina sequencing from gallbladder mucosa of patients with or without gallstones, and were validated by randomly selected 6 miRNAs and 8 genes using quantitative RT-PCR. 114 miRNA target genes were identified, whose functions and regulating pathways were related to gallstones. The differentially expressed genes were enriched upon lipoprotein binding and some metabolic pathways, and differentially expressed miRNAs enriched upon ABC transportation and cancer related pathways. A molecular regulatory network consisting of 17 differentially expressed miRNAs, inclusive of their target genes, was constructed. miR-210 and its potential target gene ATP11A were found to be differentially expressed in both miRNA and mRNA profiles. ATP11A was a direct target of miR-210, which was predicted to regulate the ABC-transporters pathway. The expression levels of ATP11A in the gallstone showed inverse correlation with miR-210 expression, and up-regulation of miR-210 could reduce ATP11A expression in HGBEC. This is the first report that indicates the existence of differences in miRNA and mRNA expression in patients with or without gallstones. Our data shed light on further investigating the mechanisms of gallstone formation.
Introduction
In modern society, gallstone disease is becoming increasingly common and is often a major affliction in all age groups.1,2 Furthermore, although the prevalence of gallstones varies geographically, the disease is a worldwide medical problem.3–6 There are three types of gallstones: (i) pure cholesterol stones; (ii) pigment stones; and (iii) mixed composition stones.7 More than 70% of gallstones are composed primarily of cholesterol, either purely or mixed with pigment, mucoglycoprotein, and calcium carbonate.8 Gallstones occur in the gallbladder when an imbalance in the chemical constituents of bile results in the precipitation of one or more of these components.9 In short, when the concentration of cholesterol in bile exceeds the ability of bile to hold it in solution, crystals form and grow into gallstones.10
However, the pathogenesis of gallstones is in fact complex and also includes genetic factors related to the hepatic hypersecretion of biliary cholesterol, such as the Niemann-Pick C1-like 1 (NPC1L1) pathway, LITH genes family, which lead to the unphysiological supersaturation of gallbladder bile with cholesterol, and result in gallstone formation. In recent years, microRNAs (miRNAs) have been found to be closely related to many diseases. miRNAs are a class of evolutionarily conserved, small (∼22 nucleotides) non-coding RNAs that regulate gene expression at the post-transcriptional level and participate in the regulation of various cellular processes, such as cell differentiation, cell cycle progression, metabolism and apoptosis.11 Present estimates suggest that nearly a third of all cellular transcripts may be regulated by the few hundred human miRNAs currently known to exist.12 With respect to gallstones, miRNAs may regulate the tightly controlled homeostatic mechanisms that affect bile acid synthesis and secretion;13 however, the role of miRNAs is still unclear. Lee et al. suggested that the FXR/SHP cascade pathway controls the expression of miR-34a and its target SIRT1, which are associated with gallstones.14 miRNAs 122a and 422a were reported to directly target and inhibit the translation of the human cholesterol 7a-hydroxylase (CYP7A1), which maintains the bile-acid pool.15 miR-33 was shown to target the ABCB11 and ATP8B1 mRNAs, thereby regulating FIC1, which is a canalicular phospholipid translocase required for normal function of the hepatocyte secretory machinery.16 In addition, ABCA1, which is a major determinant of plasma high-density lipoprotein (HDL)-cholesterol levels, has been shown to be related to the bile-acid sensor farnesoid X receptor (FXR) via miR-144. Activated FXR interacts with FXR response elements upstream of the miR-144 locus and increases hepatic levels of miR-144, which in turn lower hepatic ABCA1 and plasma HDL levels in mice.17
In the current study, we utilized high-throughput sequencing to perform miRNA and mRNA expression profiling in patients with or without gallstones, in order to identify significant differences in miRNA and mRNA expression patterns. We further performed an integrated analysis to identify miRNAs whose expression was inversely correlated with the expression of an mRNA target. To our knowledge, this is the first analysis of miRNA and mRNA expression in gallstone disease. Our analyses provide both an integrated and comprehensive search for possible candidate miRNAs and their mRNA targets for further studies on gallstone formation.
Results and discussion
Overview of mRNA and miRNA sequencing results
We sequenced mRNAs and microRNAs from samples using a HiSeq 2500 system, whose demographics is shown in Table S1 (ESI†). From the two mRNA libraries, an average of 13
470
672 single-end 50 bp reads were generated (Table S2, ESI†); from the miRNA libraries, 4
452
180 single-end 51 bp reads were also generated on average (Table S3, ESI†). After applying the quality filter, the mRNA sequence reads were aligned to the human genome (http://hgdownload.cse.ucsc.edu/goldenpath/hg19/bigZips/chromFa.tar.gz and http://hgdownload.cse.ucsc.edu/goldenpath/hg19/bigZips/ref Mrna.fa.gz), while small RNA sequence reads were aligned to the miRBase V20 and Rfam V11 databases. The data were filtered by removing reads without a 3′ adaptor sequence, 5′ adaptor contaminants, poly-A reads, and reads that did not map to the human genome; reads with sizes between 18 and 32 nt were kept. The length distribution and the composition of sRNAs are summarized in Fig. 1. The majority of the reads were in the range of 20 to 23 nt, with 22 nt being the most represented. Detailed sequencing data have been uploaded to the DNA Data Bank of Japan under DDBJ Series accession numbers DRA002324 and DRA002325.
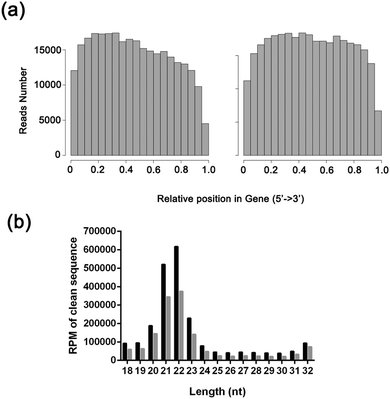 |
| Fig. 1 The characteristics of the data. (A) RNAseq random assess hist map. Left, gallbladder mucosal tissue from patients with gallbladder polyps; right, gallbladder mucosal tissue from patients with gallbladder stone. (B) The length distribution of miRNA. Black, gallbladder mucosal tissue from patients with gallbladder polyps; gray, gallbladder mucosal tissue from patients with gallbladder stone. | |
Differentially expressed mRNAs and miRNAs in gallbladder mucosal tissue from patients with or without gallstones
We first investigated miRNA and mRNA expression, and a total of 525 genes were differentially expressed based on two-sample t tests (P < 0.05) (Table S4, ESI†). We obtained 294 up-regulated and 231 down-regulated mRNAs in patients with gallstones versus those without gallstones. The results of high-throughput mRNA sequencing revealed that four differentially expressed genes are involved in lipoprotein particle binding and protein–lipid complex binding in gallstones, which is in agreement with several previous studies. Meanwhile, certain differentially expressed genes were found to be predominantly enriched in the immune system, obesity, and even directly in bile secretion, which is fairly consistent with the pathogenesis of gallstone disease.
In contrast, only 17 miRNAs showed differential expression with an FDR of 0.1% between the two groups at the nominal P-value 0.05 level (Table S5, ESI†), and 9 up-regulated genes and 8 down-regulated miRNAs were defined. These miRNAs have been confirmed to be associated with some biliary diseases, such as the miRNA-200 family (miR-200c, miR-141, miR-21), which is involved in human gall bladder cancer via epigenetic regulation,18 and the miR-200b/429 cluster, which was shown to be significantly increased in the sera of biliary atresia.19 Quantitative real time-PCR also confirmed that miR-146a expression was increased in PBMCs from primary biliary cirrhosis patients13, although the biological role remains unclear.
We used qRT-PCR to further validate the changes in 6 miRNAs and 8 mRNA identified in the sequencing analysis. The results showed that miR-133a and miR-891a expression was significantly decreased, whereas miR-210, miR-200c, miR-194 and miR-192 expression was significantly increased, which was consistent with the results of high-throughput miRNA sequencing (Fig. 2a). qRT-PCR also showed that ATP11A, TRDN, IFI27 and MYL3 were significantly decreased, whereas RPS4Y1, USP9Y, AMH and SLC28A2 were significantly increased. These results were consistent with the results of high-throughput mRNA sequencing (Fig. 2b).
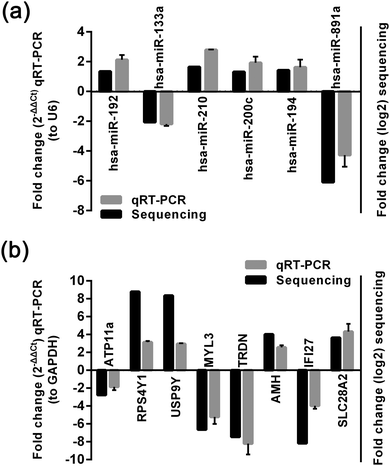 |
| Fig. 2 Validation results of quantitative RT-PCR for miRNA and mRNA expression. (a) qRT-PCR and HT-sequencing results of 6 miRNAs examined in patients with gallstone and without gallstone. (b) qRT-PCR and HT-sequencing results of 8 mRNAs examined in patients with gallstone and without gallstone. | |
miRNA target prediction
We performed target prediction for 17 miRNAs using scripts from Targetscan, PITA, and miRanda. The UTRs of the 2812 significantly expressed mRNAs were predicted from at least two out of three algorithms with high efficacy. The genome sequences and transcriptome sequences of human-relative species, including Pan paniscus, Pongo pygmaeus, Gorilla, Pan troglodytes, and Symphalangus syndactylus, were also used to predict potential novel miRNAs. A total of 32 novel miRNAs were obtained (Table S6, ESI†).
GO and KEGG analysis of mRNA targets of differentially expressed miRNAs
To investigate the pathway perturbations underlying gallstones, we performed gene ontology (GO) and KEGG pathway analyses on differentially expressed genes from mRNA sequencing and the target genes of differentially expressed miRNAs. The miRNA target genes were formulated into an XML-based input data set to query the GO database. The GO functions annotated are shown in Fig. 3.
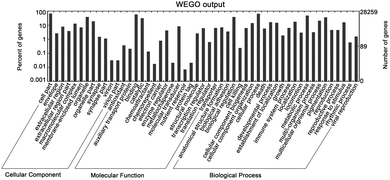 |
| Fig. 3 Gene ontology analysis of mRNA. Good hits were aligned to the GO database, and 28 259 transcripts were assigned to at least one GO term. All the unigenes were grouped into three major functional categories, including cellular component, molecular function and biological process. The right y-axis indicates the number of unigenes in a category. The left y-axis indicates the percentage of a specific category of unigenes in that main category. | |
For differentially expressed genes, only 1 GO term in the category of “biological process”, 2 GO terms in the category of “molecular function” and 4 GO terms in the category of “cellular component” were significantly enriched at an FDR threshold of P < 0.05 (Table S7, ESI†). The enriched GO terms of “molecular function” were related to “lipoprotein particle binding” and “protein–lipid complex binding”. Interestingly, KEGG pathway analyses revealed that several different pathways that were seemingly irrelevant to gallstones were significantly disturbed (FDR P < 0.05) (Table S7, ESI†). However, some metabolic pathways involved in gallstones were observed to be enriched in our study (FDR P < 0.1), such as bile secretion, etc. (Table S7, ESI†).
For the target genes of all differentially expressed miRNAs with an FDR threshold of P < 0.05, 26 GO terms were enriched from “biological process”, 11 GO terms form “molecular function” and 1 GO term form “cellular component” (Table S8, ESI†). The biological categories of “insulin receptor signaling pathway via phosphatidylinositol 3-kinase cascade”, “positive regulation of hormone biosynthetic process”, and “positive regulation of steroid hormone biosynthetic process” were most significantly enriched (Table S8, ESI†). KEGG analyses of the miRNA targets revealed pathways in “Proteoglycans in cancer”, “Wnt signaling pathway”, and “Hippo signaling pathway”, suggesting a role for gallstone in the development of gallbladder cancer (Table S8, ESI†).
GO comparisons of differentially expressed mRNAs between groups with and without gallstones demonstrated that these groups were quite different, as the former always contained more genes involved in lipoprotein particle binding and protein–lipid complex binding. Previous studies confirmed that the lipid-binding properties of gallbladder mucin may also be important in the pathophysiology of gallstones.20–22 In the GO analysis of differentially expressed miRNAs, in addition to lipoprotein particle binding, target genes were also found to be enriched in fibroblast growth factor-activated receptor (FGF) activity,23,24 metal endopeptidase activity, protein kinase activity25,26 and calcium channel activity, which show relation to gallstones. In particular, FGF activity has been shown to be important for gallbladder motility, such as FGF-19.27 Calcium channel activity also appears to maintain the electrical driving force for continued chloride efflux in gallbladder and bile duct epithelial cells,28 and could regulate the smooth muscle function. Lavoie et al. confirmed that disruption of gallbladder smooth muscle function is an early feature in the development of cholesterol gallstone disease.29 However, the role of metal endopeptidase activity in gallstones has never been reported. More comprehensive in-depth analysis is warranted.
In our KEGG analysis of differentially expressed genes, several genes were found to be enriched in the ABC transporters and bile secretion pathways, which are directly associated with the pathogenesis of gallstones. Other genes were enriched in several KEGG pathways indirectly related to gallstones, including glycolysis/gluconeogenesis, PPAR signaling pathway and steroid hormone biosynthesis. Dong et al. found that pravastatin can prevent gallstones by activating PPARs (especially PPAR gamma) in the liver and the gallbladder epithelium of hamsters.30 According to the reported mechanism, the failure to generate endogenous PPARγ agonists leads to cholesterol supersaturation in bile and thus gallstone formation,31 and another study suggested that decreased hepatic expression of PPARγ coactivator-1 is associated with the presence of cholesterol gallstones.32 Steroid hormone biosynthesis, as a mediator of cholesterol, may on the one hand control gallbladder shrinkage and on the other hand alter the expression of factors regulating cholesterol metabolism, which promotes lithogenic bile secretion and stone formation. In addition, the results of KEGG analysis show that differentially expressed mRNAs are related to inflammation, even if we strongly ruled out the samples with cholecystitis. It is not unexpected because presence of gallstones can lead to the inflammation of gallbladder.33,34 The result supported that the microscopic molecular levels reflect earlier than clinical pathological symptoms upon detection of the disease. Interestingly, with the exception of the ABC transporters pathway and calcium signaling pathway related to gallstones, the KEGG analysis revealed that most differentially expressed miRNAs were enriched in cancer-related pathways, such as proteoglycans in cancer, basal cell carcinoma, and the Wnt signaling pathway. These pathways are likely involved in the mechanism by which gallstones influence the development of gallbladder cancer.
The posttranscriptional regulatory network of miRNAs and target genes
The miRNA–target gene regulatory network of gallstones was constructed as described in Materials and methods. A total of 17 miRNAs and 94 genes formed 188 miRNA–target gene pairs with inversely correlated expression (Fig. 4).
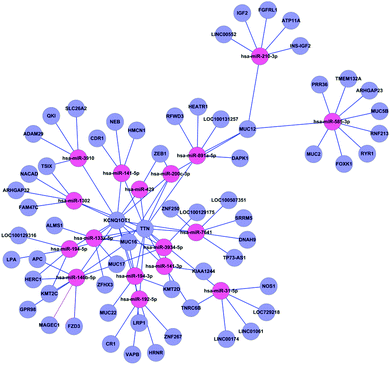 |
| Fig. 4 Regulatory network from integrated analysis of miRNA–mRNA data. Negatively correlated miRNA–mRNA interactions were visualised as a network using Cytoscape. Lines represent predicted interactions considering negatively correlated miRNA (red) and mRNA (purple) expression data. | |
Potential role of miRNAs in regulating mRNA expression
We combined the mRNA and miRNA data to perform GO ontology and KEGG pathway enrichment analyses (Table S9, ESI†) and identify intersection between target genes of differentially expressed miRNAs and the differentially expressed mRNAs.
Integrated analyses of miRNA and mRNA expressions identified has-miR-210-3p and its possible anti-correlate target gene, Homo sapiens ATPase, class VI, type 11A (ATP11A, NM_032189), which encodes a novel ABC transporter homolog, ATP11A.35 There have been no studies on the relationship between miR-210 or ATP11A and gallstone disease, though the correlation among them was further validated. Previous studies reported that miR-210 was associated with hypoxia, which influences cellular functions, including metabolism, survival, proliferation, migration and angiogenesis.36,37 miR-210 is highly expressed in hypoxic cells and tissues and is reportedly regulated by HIF-1α stabilization in response to hypoxia.38–40 In Balb/c mice with chronic cholestasis induced by DLD, miR-210 up-regulation accelerated the progression of cholangiocarcinoma via the HIF-2α-miR-210-Mnt pathways.41 As chronic cholestasis is an important pathogenic factor for gallstones, we predict that miR-210 may play a critical role in the formation of gallstones and may even be involved in gallbladder development.
ATP11A belongs to an extended family of P-type ATPases, which transport compounds across membranes, and are essential for generation and maintenance of phospholipid asymmetry in lipid bilayers.42,43 Recently, Takatsu et al.'s study found that ATP11A can flip aminophospholipids, phospholipids and phosphatidylethanolamine (PE). Hydrolysis of bile phospholipids (PS) into lysolecithin and free fatty acids has an important role in gallstone formation, and needs the participation of PS flippase. Although there are no other reports linking ATP11A to the pathogenesis of gallstones, genetic traits support effects of ATP11A. Based on the similarity of residue sequence, primary structure and homology to domains with the other members in this gene family, ATP11a is predicted to have 10 transmembrane domains and 1 ATP-binding site,35 which may mediate the biliary secretion of bile acids (ABCB11),44–49 phospholipids (ABCB4)50–53 and cholesterol (ABCG5/G8).54–58 This is consistent with the results of our KEGG pathway analysis. Because ATP11A is expressed widely in many different types of tissues,59 if as a transporter, it is likely to have a transport function more general than that in, for example, primary bile formation. In addition, ATP11A is probably phosphorylated in its intermediate state and likely drives the transport of ions such as calcium or other molecules across membranes, which may affect the mobility of gallbladder. However, the regulatory mechanism of calcium changes by ATP11A is still unclear and needs further exploration.
Validation of ATP11A as a target gene of miRNA-210
To test whether ATP11A is a direct target of miRNA-210 in 293T cells, a dual luciferase reporter with or without the 3′UTR of ATP11A was cotransfected with a miR-210 mimic or a control mimic into 293T cells. The result indicated a 53.2% reduction in the activity of a dual luciferase reporter containing the 3′UTR of ATP11A when transfected with the miR-210 mimic compared with the control. No significant change in luciferase activity was detected in the cells co-transfected with the dual luciferase reporter lacking the 3′UTR of ATP11A and either the miRNA-210 mimic or the control (Fig. 5a). Meanwhile, Western blotting revealed that transfecting miR-210 into HGBEC cells resulted in significant inhibition of ATP11A protein expression compared with cells transfected with the mimic control (Fig. 5b). In addition, we examined the expression of miR-210 and ATP11A in the gallbladder tissues by qRT-PCR and found that ATP11A expression levels were inversely correlated with those of miR-210 (Fig. 5c). Together, these results demonstrated that ATP11A is a target of miRNA-210 and that the up-regulation of miR-210 and down-regulation of ATP11A may be involved in the gallbladder cells with gallstones.
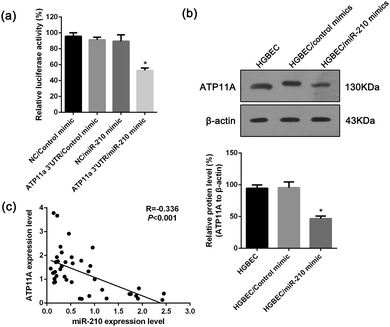 |
| Fig. 5 Validation of ATP11A as a target of miR-210. (a) miR-210 mimic significantly reduced the luciferase activity of a dual luciferase reporter with the 3′UTR of ATP11A compared to the controls. Values are the means ± SD of percent changes over controls after normalization to the renilla luciferase activity. (b) A representative result of Western blot shows the expression level of ATP11A in the HGBEC cells transfected with the miR-210 mimic or mimic control. β-actin was used as an internal control for loading. Three experiments were done: columns, mean; bars, SD *P, 0.05 differ from the controls. (c) Correlation analysis between ATP11A and miR-210. Pearson's correlation coefficient and P-value for individual analysis are shown in the insets. | |
Experimental
Cell lines and specimens
Human gallbladder epithelial cells (HGBEC) were previously established by our laboratory. The cells were cultured in the culture dishes coated with collagen type IV and bovine calf serum, and with DMEM/F12 medium supplemented with 10% FBS (Invitrogen, USA) and 1% streptomycin–penicillin (Invitrogen) in an incubator at 37 °C with humidified 5% CO2.
All samples of patients were obtained from the 1st Affiliated Hospital of Kunming Medical University (city of Kunming, Yunnan province, China) between June, 2013 and December, 2014, which assures written informed consent for the use of material to research purpose from all subjects. The study was approved by the ethics committee of Kunming Medical University. Patient eligibility policy: A, all patients were confirmed no obvious inflammation by pathology. B, all the patients were free of viral hepatitis, diabetes and metabolic diseases. C, no acute cholecystitis attacks and without the use of any antibiotics within three months. Samples of gallstone tissue and gallbladder polyps tissue obtained from fresh surgery specimens, frozen in liquid nitrogen, then stored at −80 °C.
Additional 20 samples were collected to be used for qRT-PCR validation. Informed consents were obtained from all the patients and the study was approved by the ethics committee of Kunming Medical University.
Isolation of total RNA
Total RNA was isolated using Trizol reagent (Invitrogen) according to the manufacture's protocol. The RNA pellet was finally resuspended in 30 μl RNase-free water. The concentration and integrity of RNA was assessed using the Agilent BioAnalyzer 2100 (Agilent Technology, USA).
Library preparation and sequencing
cDNA library preparation was performed using the Illumina DGE SmallRNA Sample and RNA-Seq Prep kit (Illumina, USA) following the manufacturer's instructions. Briefly, total RNA was poly-A-selected to deplete the ribosomal RNA fraction. cDNA was synthesized using random hexamers, end-repaired and ligated with appropriate adaptors for sequencing. The library was then subjected to size selection and PCR amplification, followed by PAGE purification before sequencing. Stranded small RNA libraries were prepared by ligating different 3′ and 5′ adaptors sequentially to the total RNA followed by reverse transcription and PCR amplification. Small RNAs with insert sizes of 20–70 bp were PAGE-purified for sequencing. The purified DNA was quantified and diluted to 10 nM for cluster generation and sequencing on an Illumina Genome Analyzer GAII. The data discussed in this publication have been deposited in the DNA Data Bank of Japan and are accessible through DDBJ Series accession numbers DRA002324 and DRA002325.60
Analysis of deep sequencing data
Primary data analysis: sequences were obtained using Illumina HiSeq. Reads were mapped against Illumina adaptor sequences using blat17 and the 3′ adaptors were subsequently clipped from the reads using NGS QC Toolkit (v2.3). The reads were then filtered for low complexity regions and the remaining reads were size selected for 16–32 nt, resulting in the inclusion of more than 70% of reads on average for each sample.
Analysis of mRNA expression: mRNA expression data were based on the counts of genes and were subsequently background corrected, normalized, and polished using robust multichip average (RMA) as previously described.61 mRNA signal intensities were log2 transformed, and analyzed for differentially expressed mRNAs using the EdgeR software, and the P-values of the t-test were calculated. Differentially detected mRNA signals with ≥1.0 fold-change and P < 0.05 were considered to be significant. Unsupervised hierarchical clustering was performed for the differentially expressed mRNAs with P < 0.01 using Cluster 3.0 and Java TreeView-1.1.6-win.
Analysis of miRNA expression: For differential miRNAs expression, the overlap between mapped reads and human mature microRNAs (based on miRBase v1962) was found using the findOverlaps function in the Bioconductor GenomicRanges package (http://bioconductor.org/packages/2.6/bioc/html/GenomicRanges.html). The normalization and differential expression analysis were performed using the Bioconductor edgeR package v2.3.5763 using both common and tagwise dispersion. The significant differentially expressed microRNAs were determined by an adjusted P < 0.05 based on the Benjamini and Hochberg multiple testing corrections.
miRNA target prediction
miRNA target prediction was performed using scripts from Targetscan,64 PITA,65 and miRanda.66 miRNA target sites are identified to a well-defined set of 3′UTRs, and a gene was considered to be a target of an miRNA if there was at least one conserved binding site in the 3′UTR of at least one transcript of the gene. For binding sites predicted by at least 2 of the 3 aforementioned algorithms, we replaced the reference sequence with the variant and repeated the prediction with all the reference and variant sequences.
Gene ontology
The predicted miRNA target genes and the differentially expressed genes were subjected to the gene ontology analysis using the database for annotations, visualization and integrated discovery (DAVID).66–68 The target genes were mapped to the GO annotation dataset, and the enriched biological processes were extracted using the hypergeometric test. Statistics related to the over-representation of functional categories was based upon a Fisher's exact statistic methodology similar to that described by Al-Shahrour et al.69 A P < 0.05 was considered to be significant.
Gene ontology and KEGG pathway analyses of miRNA target genes
GO and KEGG pathway analysis was performed using CytoscapeV2.7 (http://cytoscape.org/) with the ClueGO V1.3 plug-in.70 ClueGO determines the distribution of the target gene list across systematic analysis of methylation, mRNA and miRNA GO terms and pathways. The P-value was calculated using a right-side hypergeometric test, and a Benjamini–Hochberg adjustment was used to perform multiple test correction. An adjusted P < 0.05 indicates a statistically significant deviation from the expected distribution, and thus, the corresponding GO terms and pathways were enriched in target genes. We analyzed all of the differentially methylated loci, expressed genes, and target genes of deregulated miRNAs using GO and KEGG pathway analysis.
Construction of a miRNA–target gene regulatory network
The experimentally identified gallstone → gene and gallstone → miRNA interactions were combined with predicted miRNA → gene interactions to form an integrated gene regulatory network. In the network, we only included genes for which both differential expression data and miRNA target-site predictions were available, namely, those genes used as the input for gallstone differential expression identification and miRNA target prediction.
miRNA–mRNA co-expression
Analyses to identify negative correlations between miRNA and mRNA expression were performed using an in-house Rscript. Briefly, normalized miRNA and mRNA data were sample-matched for all samples with both miRNA and mRNA sequencing data. Then, for each miRNA, Pearson correlation coefficients were computed for the predicted target mRNAs, and a contingency table was created for all mRNAs and used to assess the enrichment level of the negative correlated mRNAs (correlation < 0 and P value of correlation ≤0.05) within predicted targets of the intended miRNA using Fisher's exact test.
Real-time quantification of miRNAs and mRNAs
To validate the reliability of the sequencing data, real-time quantification of random mature miRNAs and mRNAs was performed using a two-step RT-PCR according to the manufacturer's protocol. For miRNA expression, cDNA was reverse-transcribed from 2 μg of total RNA and detected by real-time PCR using the SYBR PrimeScript miRNA RT-PCR Kit (Takara, Japan) according to the manufacturer's instructions; U6 was used as the internal control.
To validate mRNA expression, 2 μg of total RNA was reverse-transcribed into cDNA using MMLV (Promega) and Oligo dT primers (Tiangen, Beijing) according to the manufacturers' protocols. The RT products were amplified by real-time PCR using the QuantiFast SYBR green PCR kit (Qiagen) according to the manufacturer's instructions, and GAPDH was used as the internal control.
All qRT-PCR reactions were typically run in triplicate on an ABI QuantStudio 12K Flex System (ABI, USA). The primers are shown in Table S10 (ESI†). The relative expression was quantified using the “2-ΔΔCt” method.71
Dual-Luciferase Reporter Assay
To test whether miRNA-210 can specifically target ATP11A, we constructed a dual-luciferase reporter containing the 3′UTR of ATP11A, which was cotransfected into 293T cells together with a miR-210 mimic or a control mimic (RiboBio) using Lipofectamine 2000 as previously described. A dual-luciferase reporter without the 3′UTR of ATP11A, pEZX-MT01 was used as a control. Cells were harvested 48 h after transfection, and both firefly luciferase and renilla luciferase activities were measured using the Dual-Luciferase Reporter Assay System (Promega) according to the manufacturer's protocol. The statistical significance of the differences in luciferase activity was determined using Student's t test.
Western blot
Cultured HGBEC cells or transfected cells were collected in lysis buffer (50 mM Tris-HCl, pH 7.5, 150 mM NaCl, 1% Triton X-100, and 0.25% sodium deoxycholate) and briefly sonicated to shear DNA and reduce sample viscosity. The protein concentration was measured using the BCA Reagent kit (TIANGEN, Beijing, China). Samples were run on a 12% PAGE gel and transferred onto PVDF membranes. After blocking in 5% nonfat dry milk in TBST (0.1% Tween 20 in PBS) for 1 h, membranes were incubated with monoclonal anti-human ATP11A Antibody (Santa Cruz Biotechnology, sc-83985, 1
:
500 dilution) overnight at 4 °C. After three washes in TBST for 10 min each, membranes were incubated in goat anti-rabbit IgG conjugated with horseradish peroxidase for 1 h followed by two washes in TBST and TBS for 5 min each, respectively. The signals were developed in the ECL Chemiluminescent kit (Amersham Pharmacia Biotech, Arlington Heights, IL).
Analysis of miRNA-210 and ATP11A expression in tissues
The expression levels of ATP11A and miR-210 in the same gallbladder tissues from 20 patients were detected by qRT-PCR as described above. Significant inverse correlations between miRNA-210 and ATP11A expression were determined using Pearson's correlation analysis.
Conclusions
To our knowledge, this is the first study reporting on the difference between patients with or without gallstones. In the present study, many differentially expressed miRNAs and mRNAs were identified in gallstone patients. A total of 9 miRNAs were found to be up-regulated, and 8 miRNAs were down-regulated. In addition, mRNAs for 294 genes were up-regulated and 231 were down-regulated. miR-210 and its target gene ATG11A were identified through an integrated analysis of differentially expressed miRNAs and mRNAs and may be involved in the ABC transporters pathway, which was confirmed by the KEGG pathway analysis. We also showed that ATP11A was a direct target of miR-210, and upregulated miR-210 play an important role in gallstones by targeting ATP11A. Our data are helpful for further studying the molecular mechanism of gallstone formation.
Acknowledgements
We gratefully acknowledge the assistance of Bo Yang in processing the data and drawing the graphs.
Notes and references
- T. A. Bouchier, Proc. R. Soc. Med., 1977, 70, 597–599 CAS.
- E. A. Shaffer, Best Pract. Res., Clin. Gastroenterol., 2006, 20, 981–996 CrossRef PubMed.
- S. Hadidy, J. Turki and H. T. Misri, Am. J. Surg., 1987, 153, 392–393 CrossRef CAS.
- R. E. Sampliner, P. H. Bennett, L. J. Comess, F. A. Rose and T. A. Burch, N. Engl. J. Med., 1970, 283, 1358–1364 CrossRef CAS PubMed.
- M. Acalovschi, Postgrad. Med. J., 2001, 77, 221–229 CrossRef CAS.
- I. Halldestam, E. L. Enell, E. Kullman and K. Borch, Br. J. Surg., 2004, 91, 734–738 CrossRef CAS PubMed.
- I. J. Beckingham, BMJ, 2001, 322, 91–94 CrossRef CAS.
- J. T. LaMont, B. F. Smith and J. R. Moore, Hepatology, 1984, 4, 51S–56S CrossRef CAS.
- N. A. Channa, F. D. Khand, T. U. Khand, M. H. Leghari and A. N. Memon, Pak. J. Med. Sci., 2007, 23, 546 Search PubMed.
- D. E. Johnston and M. M. Kaplan, N. Engl. J. Med., 1993, 328, 412–421 CrossRef CAS PubMed.
- S. Sassen, E. A. Miska and C. Caldas, Virchows Arch., 2008, 452, 1–10 CrossRef CAS PubMed.
- D. P. Bartel, Cell, 2004, 116, 281–297 CrossRef CAS.
- J. J. Marin, L. Bujanda and J. M. Banales, Curr. Opin. Gastroenterol., 2014, 30, 303–309 CrossRef CAS PubMed.
- J. Lee and J. K. Kemper, Aging, 2010, 2, 527–534 CAS.
- K.-H. Song, T. Li, E. Owsley and J. Y. Chiang, J. Lipid Res., 2010, 51, 2223–2233 CrossRef CAS PubMed.
- R. M. Allen, T. J. Marquart, C. J. Albert, F. J. Suchy, D. Q. H. Wang, M. Ananthanarayanan, D. A. Ford and Á. Baldán, EMBO Mol. Med., 2012, 4, 882–895 CrossRef CAS PubMed.
- T. Q. de Aguiar Vallim, E. J. Tarling, T. Kim, M. Civelek, A. Baldan, C. Esau and P. A. Edwards, Circ. Res., 2013, 112, 1602–1612 CrossRef CAS PubMed.
- Y. Han, H. L. Francis, S. Glaser, J. McCarra, G. Alpini, J. Sharma, G. Dusio, C.-G. Liu, C. Johnson and F. Meng, Gastroenterology, 142, S-926–S-927 Search PubMed.
- A. M. Zahm, N. J. Hand, L. A. Boateng and J. R. Friedman, J. Pediatr. Gastroenterol. Nutr., 2012, 55, 366–369 CrossRef CAS PubMed.
- B. F. Smith and J. T. LaMont, J. Biol. Chem., 1984, 259, 12170–12177 CAS.
- C. W. Ko, C. Murakami, J. H. Sekijima, M. H. Kim, G. B. McDonald and S. P. Lee, Am. J. Gastroenterol., 1996, 91, 1207–1210 CAS.
- A. Dikkers and U. Tietge, World J. Gastroenterol., 2010, 16, 5936–5945 CAS.
- T. Inagaki, M. Choi, A. Moschetta, L. Peng, C. L. Cummins, J. G. McDonald, G. Luo, S. A. Jones, B. Goodwin, J. A. Richardson, R. D. Gerard, J. J. Repa, D. J. Mangelsdorf and S. A. Kliewer, Cell Metab., 2005, 2, 217–225 CrossRef CAS PubMed.
- M. Choi, A. Moschetta, A. L. Bookout, L. Peng, M. Umetani, S. R. Holmstrom, K. Suino-Powell, H. E. Xu, J. A. Richardson, R. D. Gerard, D. J. Mangelsdorf and S. A. Kliewer, Nat. Med., 2006, 12, 1253–1255 CrossRef CAS PubMed.
- W. Huang, R. R. Bansode, Y. Xie, L. Rowland, M. Mehta, N. O. Davidson and K. D. Mehta, J. Biol. Chem., 2011, 286, 22795–22805 CrossRef CAS PubMed.
- T. Claudel, G. Zollner, M. Wagner and M. Trauner, Biochim. Biophys. Acta, 2011, 1812, 867–878 CrossRef CAS PubMed.
- A. H. Smelt, Clin. Chim. Acta, 2010, 411, 1625–1631 CrossRef CAS PubMed.
- M. J. Amaya and M. H. Nathanson, Cell Calcium, 2014, 55, 317–324 CrossRef CAS PubMed.
- B. Lavoie, B. Nausch, E. A. Zane, M. R. Leonard, O. B. Balemba, A. C. Bartoo, R. Wilcox, M. T. Nelson, M. C. Carey and G. M. Mawe, Neurogastroenterol. Motil., 2012, 24, e313–e324 CrossRef CAS PubMed.
- S. H. Dong, J. Lee, D. H. Koh, M. H. Choi, H. J. Jang and S. H. Kae, Hepatobiliary Pancreatic Dis. Int., 2011, 10, 185–190 CrossRef CAS.
- J. H. Miyake, S.-L. Wang and R. A. Davis, J. Biol. Chem., 2000, 275, 21805–21808 CrossRef CAS PubMed.
- M. Bertolotti, C. Gabbi, C. Anzivino, N. Mitro, C. Godio, E. Fabiani, M. Crestani, M. Puppo, M. Ricchi and L. Carulli, Eur. J. Clin. Invest., 2006, 36, 170–175 CrossRef CAS PubMed.
- B. D. Schirmer, K. L. Winters and R. F. Edlich, J. Long-Term Eff. Med. Implants, 2005, 15, 329–338 CrossRef.
- A. Abou-Saif and F. H. Al-Kawas, Am. J. Gastroenterol., 2002, 97, 249–254 CrossRef PubMed.
- B. Zhang, J. Groffen and N. Heisterkamp, Blood, 2005, 106, 1355–1361 CrossRef CAS PubMed.
- G. L. Semenza, Curr. Opin. Cell Biol., 2001, 13, 167–171 CrossRef CAS.
- G. L. Semenza, J. Appl. Physiol., 2000, 88, 1474–1480 CAS.
- H. Wang, S. Bian and C. S. Yang, Carcinogenesis, 2011, 32, 1881–1889 CrossRef CAS PubMed.
- P. G. Corn, Cancer Biol. Ther., 2008, 7, 265–267 CrossRef CAS.
- C. Nakada, Y. Tsukamoto, K. Matsuura, T. L. Nguyen, N. Hijiya, T. Uchida, F. Sato, H. Mimata, M. Seto and M. Moriyama, J. Pathol., 2011, 224, 280–288 CrossRef CAS PubMed.
- H. Yang, T. W. Li, J. Peng, X. Tang, K. S. Ko, M. Xia and M. A. Aller, Gastroenterology, 2011, 141, 378–388 CrossRef CAS PubMed , 388 e371–e374.
- T. R. Graham, Trends Cell Biol., 2004, 14, 670–677 CrossRef CAS PubMed.
- T. Pomorski, J. C. Holthuis, A. Herrmann and G. van Meer, J. Cell Sci., 2004, 117, 805–813 CrossRef CAS PubMed.
- B. Stieger, Curr. Opin. Lipidol., 2009, 20, 176–181 CrossRef CAS PubMed.
- M. Trauner and J. L. Boyer, Physiol. Rev., 2003, 83, 633–671 CAS.
- H. H. Wang, F. Lammert, A. Schmitz and D. Q. Wang, Eur. J. Clin. Invest., 2010, 40, 541–551 CrossRef CAS PubMed.
- R. Wang, P. Lam, L. Liu, D. Forrest, I. M. Yousef, D. Mignault, M. J. Phillips and V. Ling, Hepatology, 2003, 38, 1489–1499 CrossRef CAS PubMed.
- R. Wang, H. L. Chen, L. Liu, J. A. Sheps, M. J. Phillips and V. Ling, Hepatology, 2009, 50, 948–956 CrossRef CAS PubMed.
-
R. P. Elferink and C. C. Paulusma, The Liver: Biology and Pathobiology, 5th edn, 2009, pp. 339–348 Search PubMed.
- J. J. Smit, A. H. Schinkel, R. P. Oude Elferink, A. K. Groen, E. Wagenaar, L. van Deemter, C. A. Mol, R. Ottenhoff, N. M. van der Lugt and M. A. van Roon,
et al.
, Cell, 1993, 75, 451–462 CrossRef CAS.
- R. P. Oude Elferink, R. Ottenhoff, M. van Wijland, J. J. Smit, A. H. Schinkel and A. K. Groen, J. Clin. Invest., 1995, 95, 31–38 CrossRef CAS PubMed.
- P. Fickert, A. Fuchsbichler, M. Wagner, G. Zollner, A. Kaser, H. Tilg, R. Krause, F. Lammert, C. Langner, K. Zatloukal, H. U. Marschall, H. Denk and M. Trauner, Gastroenterology, 2004, 127, 261–274 CrossRef CAS PubMed.
- T. H. Mauad, C. M. van Nieuwkerk, K. P. Dingemans, J. J. Smit, A. H. Schinkel, R. G. Notenboom, M. A. van den Bergh Weerman, R. P. Verkruisen, A. K. Groen and R. P. Oude Elferink,
et al.
, Am. J. Pathol., 1994, 145, 1237–1245 CAS.
- L. Yu, J. Li-Hawkins, R. E. Hammer, K. E. Berge, J. D. Horton, J. C. Cohen and H. H. Hobbs, J. Clin. Invest., 2002, 110, 671–680 CrossRef CAS.
- L. Yu, R. E. Hammer, J. Li-Hawkins, K. von Bergmann, D. Lutjohann, J. C. Cohen and H. H. Hobbs, Proc. Natl. Acad. Sci. U. S. A., 2002, 99, 16237–16242 CrossRef CAS PubMed.
- E. L. Klett, K. Lu, A. Kosters, E. Vink, M.-H. Lee, M. Altenburg, S. Shefer, A. K. Batta, H. Yu and J. Chen, BMC Med., 2004, 2, 5 CrossRef PubMed.
- T. Plösch, V. W. Bloks, Y. Terasawa, S. Berdy, K. Siegler, F. van der Sluijs, I. P. Kema, A. K. Groen, B. Shan and F. Kuipers, Gastroenterology, 2004, 126, 290–300 CrossRef PubMed.
- L. Yu, S. Gupta, F. Xu, A. D. Liverman, A. Moschetta, D. J. Mangelsdorf, J. J. Repa, H. H. Hobbs and J. C. Cohen, J. Biol. Chem., 2005, 280, 8742–8747 CrossRef CAS PubMed.
- M. S. Halleck, J. J. Lawler, S. Blackshaw, L. Gao, P. Nagarajan, C. Hacker, S. Pyle, J. T. Newman, Y. Nakanishi, H. Ando, D. Weinstock, P. Williamson and R. A. Schlegel, Physiol. Genomics, 1999, 1, 139–150 CAS.
- R. Edgar, M. Domrachev and A. E. Lash, Nucleic Acids Res., 2002, 30, 207–210 CrossRef CAS PubMed.
- R. A. Irizarry, B. Hobbs, F. Collin, Y. D. Beazer-Barclay, K. J. Antonellis, U. Scherf and T. P. Speed, Biostatistics, 2003, 4, 249–264 CrossRef PubMed.
- M. R. Willmann and R. S. Poethig, Curr. Opin. Plant Biol., 2007, 10, 503–511 CrossRef CAS PubMed.
- M. D. Robinson, D. J. McCarthy and G. K. Smyth, Bioinformatics, 2010, 26, 139–140 CrossRef CAS PubMed.
- A. Grimson, K. K. Farh, W. K. Johnston, P. Garrett-Engele, L. P. Lim and D. P. Bartel, Mol. Cell, 2007, 27, 91–105 CrossRef CAS PubMed.
- M. Kertesz, N. Iovino, U. Unnerstall, U. Gaul and E. Segal, Nat. Genet., 2007, 39, 1278–1284 CrossRef CAS PubMed.
- D. Betel, M. Wilson, A. Gabow, D. S. Marks and C. Sander, Nucleic Acids Res., 2008, 36, D149–D153 CrossRef CAS PubMed.
- W. Huang da, B. T. Sherman and R. A. Lempicki, Nat. Protoc., 2009, 4, 44–57 CrossRef PubMed.
- D. Huang, T. R. Chang, A. Aggarwal, R. C. Lee and H. P. Ehrlich, Ann. Biomed. Eng., 1993, 21, 289–305 CrossRef CAS.
- F. Al-Shahrour, R. Diaz-Uriarte and J. Dopazo, Bioinformatics, 2004, 20, 578–580 CrossRef CAS PubMed.
- G. Bindea, B. Mlecnik, H. Hackl, P. Charoentong, M. Tosolini, A. Kirilovsky, W. H. Fridman, F. Pages, Z. Trajanoski and J. Galon, Bioinformatics, 2009, 25, 1091–1093 CrossRef CAS PubMed.
- T. D. Schmittgen and K. J. Livak, Nat. Protoc., 2008, 3, 1101–1108 CrossRef CAS.
Footnote |
† Electronic supplementary information (ESI) available: Tables S1–S10. See DOI: 10.1039/c4mb00741g |
|
This journal is © The Royal Society of Chemistry 2015 |