DOI:
10.1039/C4NJ00304G
(Perspective)
New J. Chem., 2014,
38, 3336-3349
Experimental and theoretical methods for the analyses of dynamic combinatorial libraries
Received
(in Montpellier, France)
28th February 2014
, Accepted 28th April 2014
First published on 28th April 2014
Abstract
Because of their recent interest towards dynamic combinatorial systems, chemists have to challenge their capacity to analyse complex mixtures of components exchanging their molecular fragments over time. Here we present, by a selection of practical examples from the literature, how chromatographic, spectroscopic, and microscopic techniques, as well as theoretical modelling and statistical data treatment, are instrumental in this new research area which is of significance at the interfaces with molecular biology and materials science.
Introduction
The history of chemical synthesis has always been strongly coupled to the advances of physical characterization and simulation methods.1 The development of analytical tools to probe molecular structures and their associated electronic properties is instrumental to understand the laws of chemical reactivity. Although originally based on the synthesis, purification, and analysis of single molecular components, new paradigms have progressively emerged in chemistry with the study of supramolecular complexes or assemblies and, very recently, with the interest for mixtures of reversibly interacting molecules in dynamic combinatorial libraries (DCLs).2 These studies are partly motivated by the understanding of complex matter which can produce emergent properties from the collective behaviour of individual components. They have also found a number of interesting implementations at the interfaces with molecular biology and materials science. The analyses of such systems are challenging for several reasons. First of all, the signature of individual components needs to be resolved from one another in libraries of various molecules. Second, the thermodynamic parameters of such mixtures can be easily perturbed by the analytical tool itself. Third, the rate of constitutional reorganization often requires a short analysis timescale to probe kinetic aspects. Last, in complex mixtures, the topology of the constitutional network can produce by itself a number of supplementary effects leading to false positives. This is why theoretical simulations should complement the analytical toolbox. Here we illustrate the advantages of a number of experimental and theoretical methods by a non-exhaustive review of concrete examples found in the recent literature.
Characterisation by chromatography techniques
Liquid chromatography
High pressure liquid chromatography (HPLC) is routinely used for the isolation and purification of small molecules thanks to the improved separation efficiency and wide range of affinity of HPLC columns. For DCLs, comparison of HPLC chromatograms of a same mixture, but under different thermodynamic conditions, can lead to the identification of enhanced and depleted species. However, one of the main problems associated with DCLs concerns the intrinsic instability of the components that might dissociate while interacting with the mobile or the stationary phase during the separation process. Therefore, the exchange reactions in DCLs are often frozen prior to HPLC analysis by using either chemical reactions such as reductive amination for imine bonds, pH modulation for thiol–disulphide exchange, or oxidation for dynamic covalent peptide bonds.3 In an interesting example, and in order to circumvent any potential bias caused by such a prior chemical step, Beau et al. took advantage of the pH drop upon injection of a sample of the DCL into the acidic mobile phase containing TFA (0.1%) during HPLC analysis, which effectively freezes the equilibrating mixture.4 Using this methodology, two inhibitors (0.12 and 0.15 mM) of the hen egg white lysozyme were identified among a small DCL of six glycosylated imines. Despite the apparent simplicity of a DCC experiment, identifying hits is not always straightforward as thermodynamics do not always favour the formation of the fittest, even in the presence of the molecular target.5 Indeed, due to the random statistical distribution, the best binders might only be present at low concentration even after amplification, requiring us to vary the amount of target in order to get an optimal amplification.5b To solve this problem, Case et al. have proposed to use HPLC in a so-called recursively enriched dynamic combinatorial libraries (REDCLs) methodology for the deconvolution of complex DCLs containing up to 8436 members (Fig. 1a).6 The DCLs were obtained by mixing peptides covalently modified with an N-terminal 2,2′-bipyridyl moiety and a substoichiometric amount of a metal template (Fe(II)). The use of substoichiometric template concentrations removes the bias toward heterotrimers that would exist in the presence of excess template. These DCLs were subjected to a recursive enrichment process consisting in the separation, after equilibration, of the peptides metallotrimers bound to the metal target from the rest of the DCL (monomeric peptides) by size-exclusion chromatography, followed by removal of the metal by EDTA. Subsequent HPLC analysis allows for the identification of the peptides leading to the most stable metallotrimers. This process can be repeated on the peptide mixture obtained after the first round and then reequilibrated in the presence of the metal template to further amplify the peptides of interest until the DCL converges, that is until no further evolution in the peptide distribution can be observed by HPLC analysis (Fig. 1a(ii)). From an initial set of 36 peptides, the authors were able to identify three peptide sequences of interest using the REDCLs methodology and an optimally stable heterotrimer among the 8436 possible metallotrimers from this subset of peptides.
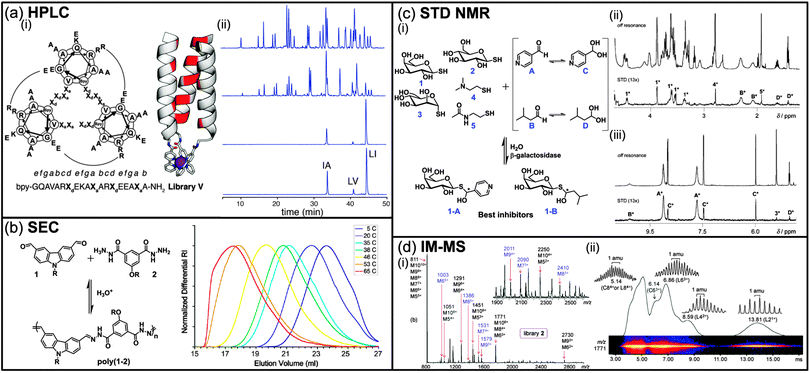 |
| Fig. 1 (a) (i) Amino acid sequence of peptide library V superimposed on a helical wheel diagram. Sequences are viewed from the N (metalbinding) termini and potential R–E salt bridges are shown by arcs. Bpy is 2,20-bipyridyl-5-carboxamide. Model of a metal-assembled parallel triple helix with: hydrophobic positions shown in red, metal ion shown as a purple sphere and Bpy residues shown as sticks; (ii) analytical HPLC chromatograms showing evolution of the peptide distribution for library V over 4 cycles of recursive enrichment (for clarity, only three are represented). The initial peptide distribution before selection (top) shows approximately equimolar amounts of 36 peptides. After cycle 4 (bottom) the library has converged to 3 sequences. Ordinate intensities are absorbance at 290 nm. (b) Acid catalyzed dynamic polymerization of di(aldehyde) 1 and di(acylhydrazine) 2 to give the polyacylhydrazone poly(1–2) (R = (CH2CH2O)6Me) and size exclusion chromatography traces of aqueous solutions of poly(1–2) ([1]0 = [2]0 = 550 mM, pH = 1.8) equilibrated for 3 days at different temperatures. (c) (i) Dynamic hemithioacetal library and enzymatic selection of the best inhibitors, 1H STD NMR studies: enlarged areas of (ii) the aliphatic region and (iii) the aromatic region. *Signals from corresponding constituents. (d) (i) ESI mass spectra of a library of bis(terpyridine)–ZnII complexes; the labels on top of the peaks give the m/z ratio of the most abundant isotope and the possible ion compositions. Charge states with unique m/z ratios (no overlapping compositions) are labeled in blue, (ii) two-dimensional IM MS plot (relative intensity vs. drift time) of bis(terpyridine)–ZnII complexes from the library: the ions appearing at m/z 1771 gave signals at 13.81, 8.59, 6.86, 6.14, and 5.14 ms, revealing the presence of superimposed L21+, L42+, L63+, C63+, and L84+/C84+ stoichiometries, respectively (L: linear, C: cyclic). (Reproduced/adapted with permission from (a) ref. 6, Copyright 2011, American Chemical Society; (b) ref. 7b, Copyright 2011, American Chemical Society; (c) ref. 21, Copyright 2010, Wiley-VCH; (d) ref. 31b, Copyright 2011, American Chemical Society.) | |
Size exclusion chromatography
In the former example, high molecular weight complexes of peptides with a metal ion target have been separated by size-exclusion chromatography (SEC) before subsequent HPLC analysis. However, SEC or gel permeation chromatography (GPC) can also be used to monitor the evolution of DCLs. As these techniques are based on the separation of molecules according to their hydrodynamic radius, they turned out to be more suitable for the analysis of DCLs based on macromolecules. For instance, Lehn and co-workers studied the evolution of DCLs made of reversible polyacylhydrazone polymers obtained from the polycondensation of pegylated aromatic diamines and dialdehydes in water (Fig. 1b).7 These DCLs were shown to be thermoresponsive with a shift of the average molecular weight toward higher molecular masses when the temperature increases and under acidic conditions, i.e. when the acylhydrazone bond is reversible.7b As aggregation and increase in the molecular weight of the polymer inevitably resulted in an increase in the hydrodynamic radius of the polymer, SEC was perfectly suitable to probe the response of this DCL upon exposure to an external stimulus. Importantly, these dynamic polymers made of acylhydrazone were stable enough under dilution and for the timescale of the SEC experiment, avoiding the drawback of dissociation into monomers during the analysis. Size exclusion chromatography was also used, in combination with 1H NMR, to monitor and quantify the selective incorporation of a hydrophobic bis hydrazide monomer, at the expense of a less hydrophobic one, upon modification of the solvent composition (water–acetonitrile mixture) in more complex DCLs of polymers generated by the polycondensation of more than 2 monomers.7a In another example, Fulton et al. proposed a DCL based on a polyvinylbenzaldehyde scaffold with aromatic aldehyde side chains being reversibly decorated through acylhydrazone formation with hydrazide-functionalized small molecules.8 This polymer scaffold can incorporate side groups with different molecular weights, resulting in polymers having different elution times when analysed by gel permeation chromatography. Two reversibly grafted polymers with different molecular weights and side chains were then mixed under conditions which lower the acylhydrazone exchange and further injected on a GPC column resulting in two separated peaks. Upon addition of an acid catalyst, the two well-separated peaks merged into a single one with an intermediate retention time suggesting the reversible scrambling of the side chains of two polymers, and the possibility to apply this methodology to create polymer-scaffolded DCLs. Recently, the group of Sumerlin demonstrated the reversible redox association of block copolymers through disulfide bonds to yield disulfide-crosslinked star polymers.9 The authors successfully cross-linked well-defined block copolymers units made of poly(styrene-alt-maleic anhydride) with a diamine that contains a disulfide bridge. The resulting star-shaped copolymers showed increased elution volume in SEC compared to the starting block copolymer units, reflecting the increase in molecular weight during the formation of the star-polymer from 71 to 92 units. SEC was also used to demonstrate the reversibility of the system with dissociation of the star-shaped polymers upon reduction of the disulfide bonds with tributylphosphine and aggregation upon oxidation with oxygen. In this example, dynamic light scattering was combined with size exclusion chromatography to demonstrate the reversibility of the system (see section ‘Characterisation by soft matter techniques’).
Affinity chromatography
Due to the large number of components in equilibrium in a DCL, another interesting approach is to selectively remove the compounds of interest from the whole library. This could in principle be achieved by immobilizing the target (enzyme, receptor, guest…) onto a solid support and taking advantage of interactions between the target and DCL components in order to separate them from the bulk library by affinity chromatography. Library members interacting preferentially with the target could therefore be selectively retained on the solid support and further identified after elution. Besenius et al. demonstrated the practical advantages of polymer-supported targets in a study involving a DCL containing over 140 potential macrocyclic hosts for a solid supported adamantane guest.10 Importantly, the author carefully selected a poly(N,N-dimethylacrylamide) support in order to prevent unwanted non-specific ion-exchange effects between the resin and the DCL members. Although several macrocycles could be amplified in the presence of the solid supported adamantane target, the use of a polymer-bound template was crucial to discriminate the best host from a false positive that was concomitantly amplified in the identical solution-based DCL. Interestingly, the selective retention of this macrocycle on the solid support allows its easy identification from other species remaining in solution after elution from the polymer support.
Headspace measurements
DCLs have recently been used to control the release of bioactive volatiles over time.11 For such applications, both 1H NMR and dynamic headspace analyses12 are recorded, the latter technique allowing a precise quantification of the released fragrances. Indeed, all the libraries analysed so far using this methodology concern the evaporation of perfumery aldehydes and ketones from aminals13 or imine derivatives.14 For example, the group of Herrmann has demonstrated that the structure of the diamine used to form aminals in dynamic mixtures is important to control the kinetics of release of the 18 volatile carbonyl compounds present in a medium.13a Up to 23 diamines have been evaluated using dynamic headspace sampling under conditions that mimic a fabric softening process. Concretely, a cotton sheet is poured into a fabric softener formulation containing the various volatile molecules and one diamine, wringed out and line-dried. This sample is then placed in a headspace sampling cell under a constant flow of humid air and the released volatiles are then analyzed and quantified by gas chromatography using external standard calibrations. In collaboration with the group of Lehn, the same authors demonstrated that an electric field can modulate the composition of a dynamic mixture of imines and aldehydes containing an imine having liquid-crystal (LC) properties.14b In that case, using a homemade set-up that incorporates a LC cell into the sampling cell, dynamic headspace analysis was the only technique used to show that the released concentration of volatiles was much higher in the presence of the electric field than in its absence. All these examples clearly established headspace measurements as a crucial technique for the implementation of DCC in the field of fragrance delivery.
Characterisation by spectroscopy techniques
NMR spectroscopy
NMR spectroscopy is a non-destructive and quantitative analysis technique that can be performed directly in solution and that provides a multitude of structural and physico-chemical information on the solutes. Classical 1H NMR experiments are an invaluable tool for the quantitative analysis of DCLs, dynamic polymers, and for the optimization of reversible reactions for DCL applications.3a13C NMR has also been used for the analysis of DCLs15a–c and in particular to probe the formation of novel amides by transamidation catalyzed by Candida rugosa lipase.15a While this method can be useful to demonstrate the formation of individual exchange products owing to the high resolution and large spectral range, it must be coupled with other quantitative measurements such as HPLC in order to extract concentration and amplification information. More recently, Prins et al. used the selective 13C-isotope labelling of imines for the quantitative analysis of a 26 member dynamic library of imines and acylhydrazones.15b They took advantage of the wider range of Larmor frequencies accessible in 13C NMR with respect to 1H NMR and were able to assign resonance signals with a sensitivity close to that of 1H NMR thanks to the 13C-isotope labelling of carbonyl carbons. Furthermore, as the number of species in a DCLs increases, it is likely that 1H or 13C NMR signals of individual compounds are going to overlap and therefore preclude detection of all species in the mixture and quantitative integration of their signals. This phenomenon was observed by Bode et al. when they analyzed the evolution of a dynamic mixture of shapeshifting 13C-labelled bisporphyrin-bullvalene derivatives in response to the introduction of analytes.15c Such a mixture can contain up to 840 different potential constitutional isomers and as many 13C NMR chemical shifts. While they were not able to resolve individual 13C NMR peaks, they managed to observe different population patterns after the introduction of analytes with very similar structures such as C60 and C70 fullerenes. Therefore, even with the wider range of Larmor frequencies accessible in 13C NMR, overlap of signals can become an issue when increasing the complexity of DCLs. In some cases, an elegant solution to this problem is the use of Diffusion Ordered SpectroscopY (DOSY) experiment which performs on 1H or 13C nuclei. This technique has gained popularity over the last 10 years for the analysis of complex mixtures due to its resolving power and the availability of efficient gradient probes and softwares.16 DOSY is often called NMR chromatography simply because it allows for a virtual separation of the NMR spectrum of the solutes based on their individual diffusion coefficient in a magnetic field gradient.17 Therefore, NMR signals of molecules having different diffusion coefficients can still be resolved even if they overlap in a standard 1D experiment. In addition, as the diffusion coefficient is related to the hydrodynamic radius and therefore the shape of the molecules, DOSY experiments can also provide structural information on the system. As a proof of concept, Lehn et al. used DOSY NMR to analyze a DCL of at least 6 helical strands and its evolution in the presence of a ZnII metal template under Sc(OTf)3 catalysis.18 Interestingly, up to five levels of diffusion coefficient could be identified, demonstrating the usefulness of this experiment to distinguish between compounds with very similar structures where 1H NMR signals overlap in a standard 1H NMR experiment. Indeed, helical strands differing by only 1 Å in size were clearly separated by their diffusion coefficients (172 and 143 μm2 s−1). The selective evolution of this complex DCL of helical strands into a grid like array was also clearly demonstrated by DOSY. Interestingly, the diffusion coefficient of the individual helical strands was found to be strongly dependent on the temperature, revealing conformational changes such as contraction and extension as a function of temperature, and demonstrating the usefulness of DOSY NMR to investigate the dynamic of motional processes. Shortly after, Giuseppone and coworkers demonstrated the asset of DOSY for the study of self-replicating supramolecular assemblies obtained from a DCL of imine-based dynamic amphiphilic block copolymers.19 Using a combination of 1H and DOSY NMR, the authors demonstrated the self-assembly of some block copolymers into micelles and further determined their diffusion coefficient and size. DOSY was also instrumental to elucidate the localization of the exchanging unimers between the inside and the outside of the micelles, and to characterize their autopoietic behavior. Indeed, after having determined the diffusion coefficient of the individual DCL components, the same diffusion rate was observed for the free aldehyde and the micellar imine at 250 μm2 s−1, demonstrating the presence of the free aldehyde in the core of the micelles.
Since its origin, DCC has been applied to the discovery of ligands for biological macromolecules. Therefore, all NMR methods that have been optimized for the combinatorial screening of protein/ligand interactions in drug discovery can potentially be applied to DCC.20 In particular, NMR techniques that take advantage of chemical-shift changes, changes in relaxation times, changes of nuclear Overhauser effects (NOEs) in exchange of saturation of ligands interacting with large macromolecules could possibly be applied to DCLs. For example, the groups of Ramström and Barbero have applied a well-established saturation transfer difference (STD) NMR experiment for the identification of β-galactosidase inhibitors from a virtual dynamic hemithioacetal library (Fig. 1c).21 This NMR experiment is designed to quickly identify interactions between a macromolecule (protein) and a small molecule by taking advantage of the magnetization transfer between the macromolecule and its ligands. Saturation of a portion of the signals of the macromolecule will transfer to the bound ligands by spin diffusion through a negative NOE, and ultimately lead to a decrease in the intensity of the hydrogen signals of the ligand close to the protein. Comparison of the spectra before and after saturation only shows the NMR signals of the best binders (Fig. 1c(ii)). Importantly, as STD experiments are based on difference spectra, even trace amounts of potential inhibitors could in principle be detected, allowing the study of DCL containing a large number of components or even virtual DCLs. In their work, Ramström et al. used a DCL made of four aromatic and aliphatic aldehydes and five different aliphatic and carbohydrate thiols under neutral aqueous conditions. The virtual nature of the library was confirmed by the absence of 1H NMR signals for the expected hemithioacetal products. Careful analysis of the STD NMR spectrum allowed the assignment of the NMR signals corresponding to the components interacting with the target protein. Further evaluation of the rate hydrolysis of o-nitrophenyl-β-galactopyranoside in the presence of the STD NMR-identified dynamic inhibitors confirmed their activity as enzyme inhibitors. This powerful technique has been extensively used for high throughput screening in drug discovery22 and is therefore potentially able to quickly test for the presence of compounds of interest in a large number of samples if sets of smaller DCLs would be preferable to analyze.
NMR analysis can also provide much more information on the dynamics of covalent exchange processes than just a quantitative analysis of single 1H peaks. For instance, it can be used to extract temporal (kinetic) as well as spatial information on dynamic covalent systems. In a recent study that extend dynamic covalent chemistry to molecular motions processes, Lehn and Kovaříček studied constitutional and motional dynamic exchanges of imine-based dynamic combinatorial libraries by a combination of variable temperature, line shape, NOESY and EXSY NMR experiments.23 By studying simple mixtures of monoaldehydes and di- or polyamines, the authors were able to determine the rate of intramolecular transimination of the aldehyde residue between two terminal amines. In addition, using variable temperature experiments, the molecular design of the aldehyde was found to affect the rates of the intramolecular group transfer process, and EXSY experiments demonstrated the reversible intramolecular linear displacement of the aldehyde residue along linear polyamines.
Mass spectrometry
Among the various techniques used to identify organic molecules, mass spectrometry has become very popular as it can quickly and unambiguously confirm the presence/absence of a particular molecule. In dynamic combinatorial libraries, electrospray ionization mass spectrometry (ESI-MS) has very often been combined with liquid chromatography. For instance, a DCL of helical strands made of a complex mixture of 28 different helices of hydrazinopyrimidine oligomers was resolved by analytical HPLC associated with an electrospray mass detector that allowed peak assignment.24 Upon addition of a metal template, the dramatic amplification of a grid species was clearly observed after submitting the metal containing DCL to HPLC/ESI-MS analysis. More recently, Otto and Ludlow reported the use of LC-MS-MS experiments to identify ephedrine receptors out of at least 9000 theoretical compounds.25 The great asset of the tandem MS experiment, which consists in fragmenting a precursor ion into product ions, was the unambiguous identification of the amplified compound out of potentially four different molecules exhibiting the same mass spectrum. This approach was also successfully exploited by Sanders and coworkers to determine the structure of various donor–acceptor catenanes produced in aqueous DCLs.26 Owing to the great progress made on mass spectrometers in terms of sensitivity, high resolution and non-denaturing mild ionization techniques, mass spectrometry has become as itself a powerful tool for deciphering amplified products in DCLs. The groups of Poulsen and Schofield have developed the field of dynamic combinatorial mass spectrometry (DCMS) to directly analyze the mass of protein–ligand complexes in order to identify protein inhibitors.27 For instance, using a library of disulfides and an ESI-Q-TOF spectrometer, selective 2OG oxygenase inhibitors with improved potency have been discovered.28 Although this approach might not be suitable for all proteins and is highly dependent on the type of interactions involved in binding, the binding results observed by ESI-MS present a good correlation with IC50 values. ESI-MS has also been recently used to qualitatively predict the most-active catalyst among a library of small ligands.29 In a palladium-catalyzed allylic substitution, the least abundant Pd–allyl complex in the ESI-MS spectra was shown to display a higher turnover frequency, an observation which confirmed high-level DFT predictions on these intermediates. Recently, the group of Fletcher showed that ESI-MS is the best technique to monitor the evolution of a dynamic library of metal complexes upon addition of an anion.30 Although UV-Vis and 1H NMR spectroscopies could be used to determine the best receptor, both techniques provided complex data sets whereas ESI-MS experiments clearly evidence the best ligands. Finally, the group of Newkome has pioneered the use of ion mobility mass spectrometry (IM MS) to determine the composition of metallosupramolecular librairies (Fig. 1d).31 With this technique, ions with different masses, charges and/or shapes are separated based on their drift under the influence of an electric field and subsequently identified by their mass spectra and, potentially, their fragmentation patterns in tandem MS. Although this technique has been successfully applied to the deconvolution of terpyridine-metal libraries, IM MS has not yet been applied to DCLs but, the solvent-free environment and the absence of a stationary phase make it ideally suitable for their analysis.
Optical spectroscopies
UV-Vis and fluorescence spectroscopies have also been widely applied to dynamic systems in order to understand their functional evolution. In particular, they have found wide applications in sensing devices made out of DCL.32 For example, Fujii and Lehn demonstrated that the optical evolution of their constitutional donor–acceptor dynamic polymer library could be correlated with the evolution of a charge-transfer band at 517 nm upon addition of alkali metal ions.33 By monitoring the evolution of this band with the concentration of sodium ions, they proved the self-sensing behavior of their system. In a previous example, Lehn and Giuseppone showed the evolution of a DCL made out of fluorene moieties by monitoring the absorbance changes by UV-Vis.34 They additionally took advantage of the fluorescent nature of their dynamic polymers (so called “dynamers”) to demonstrate the “constitutional dynamic self-sensing” behaviour of the system, i.e. a chemical stimulus drives the constitutional rearrangement of the DCL towards the amplification and the selection of the polymer that generates an optical signal characteristic of the stimulus that has led to its production. Lehn and co-workers also monitored UV-Vis absorption spectra to determine the constitutional evolution of a library of two polyhydrazone dynamers upon heating.35 The evolution of these dynamic polymers was further confirmed both in solution and on films by recording the fluorescence spectra of the newly formed dynamers. This work demonstrates that exchange processes can occur at the interface between two different polymer films, resulting in chemical interconnection of the films. The same group also reported the use of fluorescence spectroscopy to follow the exchange reaction between an acylhydrazone dynamer bearing saccharide side-chain residues and a bis-hydrazide monomer.36 Interestingly, upon excitation at two different wavelengths, the authors could easily monitor the extent of the exchange process, which was further confirmed by imaging the solutions before and after chemical exchange. Finally, fluorescence has been recently used by the group of Winssinger in combination with DNA arrays to discover new ligands for carbonic anhydrase following several selection/amplification cycles.37 In this example, PNA-encoded drug fragments were combined on complementary DNA templates and then selected against a biological target. Amplification by PCR of the template corresponding to the active drug fragment combination and further iteration of the selection process during three rounds allowed the selection of a pair of non-covalent fragments, whose corresponding covalent adduct displays a nanomolar affinity for the biological target. In this strategy, fluorescence was used to identify the sequences of DNA fragments which correspond to the best binders. Recently, Shinoda and co-workers took advantage of circular dichroism (CD) spectroscopy to discover selective amino acid sensors from a DCL of lanthanide complexes (Fig. 2a).38 First, a library of lanthanide complexes made of a lanthanide cation, an N-aromatic molecule, and a chiral amino acid in a 1
:
2
:
3 or a 1
:
1
:
2 ratio was evaluated as new induced-CD chirality probes, leading to the discovery of four complexes for the selective sensing of either alanine or glutamine. Interestingly, using the same analytical technique, complexes that display selectivity for alanine were also shown to be good discriminating sensors for various alanine derivatives such as lactate or 2-methyl butyrate. Recently, CD spectroscopy was also used by Nalluri and Ulijn to demonstrate the structural rearrangement of their dipeptide-based dynamic system upon addition of a chemical stimulus.39 However, the analysis was not performed on the entire DCL system, and HPLC and fluorescence spectroscopy were the key techniques to analyze its composition. Nevertheless, the evolution of dynamic combinatorial libraries towards functional systems has promoted the use of optical spectroscopies to understand their structural rearrangements.
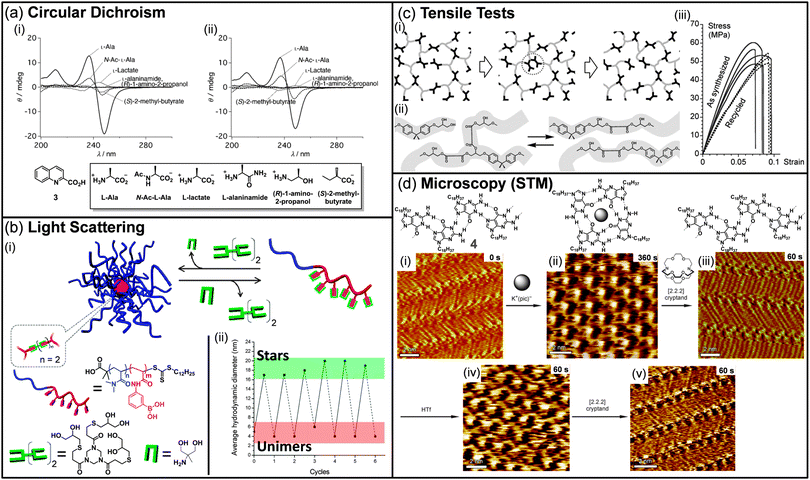 |
| Fig. 2 (a) Induced-CD spectra of (i) 3-Eu3+ and (ii) 3-Tb3+ with various alanine derivatives. Conditions: [Ln(CF3SO3)3] = 2.0 × 10−5 mol L−1, [3] = 2.0 × 10−5 mol L−1, [guest] = 4.0 × 10−5 mol L−1. (b) (i) Formation and degradation of dynamic-covalent macromolecular stars via boronic ester formation and transesterification. (ii) The graph shows the solution size measured by dynamic light scattering during investigations of the reversibility of dynamic-covalent star formation and dissociation in methanol. Solid lines indicate the addition of the (tris-diol) cross-linker, and dashed lines indicate the addition of the monodiol cross-link cleaving agent. (c) (i) Schematic view of a network with exchange processes that preserve the total number of links and average functionality of cross-links. The middle image illustrates that the exchange does not require depolymerisation in the intermediate step. (ii) Exchange process via transesterification in hydroxy-ester networks. (iii) Tensile test for samples as synthesized (solid lines) and after grinding into a powder and remolding (dashed lines). (d) Consecutive STM images showing the structural evolution of a monolayer of 4 over a 9 min time scale (time range displays in the upper right part of the images correspond to the time that was needed to reach the equilibrium after addition of reacting agents). (i), (iii), and (v) show ribbon-like structure, whereas (ii) and (iv) exhibit G4-based architectures. (Reproduced/adapted with permission from (a) ref. 38, Copyright 2012, Wiley-VCH; (b) ref. 41, Copyright 2011, American Chemical Society; (c) ref. 50, Copyright 2011, AAAS; (d) ref. 55, Copyright 2010, Wiley-VCH.) | |
Characterisation by soft matter techniques
Recently, dynamic combinatorial chemistry has been extended to the field of materials science.2b Both small molecules and polymers have been used to reach mesophases, self-healable materials, and dynamic functional nanostructures. This evolution has been made possible by the use of techniques related to the analysis of soft matter, such as scattering techniques, microscopies, or even rheology.1c
Scattering and diffraction techniques
Dynamic libraries have been recently characterized by scattering techniques, such as light, neutron, or X-ray scattering, following the extension of DCC to soft matter science. These techniques provide information on both the size and shape of the objects produced by the DCL and can be used to monitor in situ its evolution when exposed to chemical or physical stimuli. In 2011, the group of van Esch reported responsive dynamic vesicles made of double-tailed surfactants sensitive to pH modulation.40 The dynamic nature of the vesicles was first demonstrated by fluorescence spectroscopy, which results in a red shift upon lowering the pH, as the encapsulated fluorescent probe is released in the aqueous medium. The vesicle dissociation and regeneration were then confirmed by monitoring the hydrodynamic diameter of the vesicles by dynamic light scattering (DLS) upon decreasing and increasing the pH, respectively. The same year, Sumerlin and co-workers reported the formation of nanoscopic multiarm stars made of block copolymers incorporating boronic acid pending moieties and multifunctional diol cross-linkers (Fig. 2b).41 The reversibility of the boronic ester bond was then used to probe the degradation of these macromolecular stars. Upon addition of monofunctional diols to the polymeric stars, the cross-linked core was disrupted, resulting in a decrease of the average hydrodynamic diameter to a size similar to the one of the native block copolymer. By adding sequentially a diol cross-linker or a monodiol cross-link cleaving agent, dynamic light scattering was a very powerful technique to demonstrate the reversibility of the system from stars to unimers over several cycles. In 2009, Giuseppone et al. showed that small angle neutron scattering (SANS) can be used to follow the structural evolution of a library of amphiphilic dynamic block copolymers (dynablocks) upon pH modulation.42 Fitting the experimental data with an appropriate model revealed the presence of cylindrical micellar self-assemblies at any pH. Interestingly, SANS allowed the determination of the micellar structure, i.e. the length, mass, aggregation number, all highly dependent on the pH while their general shape remains unchanged. Recently, the same group took advantage of the complementarities of light scattering techniques, small angle neutron and X-ray scattering to determine quantitative structuring information on complex dynamic mixtures composed of various supramolecular self-assemblies.43 For instance, SANS was shown as a powerful technique to demonstrate the structural evolution of a library of dynablocks from pure cylindrical micelles to a mixture with vesicles upon lowering the pH of the DCL. Interestingly, the proportion of each shape could even be calculated when a coexistence was occurring. All these examples clearly demonstrate the power of scattering techniques to analyze and quantify DCL, particularly when various supramolecular self-assemblies coexist in the medium.
X-ray crystallography, which provides information on atomic scale arrangements within a crystal, was also applied to DCL to detect potent inhibitors of proteins.44 In particular, a dynamic library of up to 30 oxindole compounds made out of six hydrazines and five isatins was equilibrated in the presence of the cyclin-dependent kinase 2 protein. By monitoring the electron density within the ATP binding pocket, Congreve and co-workers could identify in situ one potent ligand, which was shown to display nanomolar activity. In a more fundamental work, the group of Barboiu has demonstrated that crystallization can serve as a trigger to sort metallosupramolecular DCLs.45 In most cases, mixing in acetonitrile terpyridine-type ligands with zinc or lead ions induces the crystallization of a unique compound, whose structure was revealed by X-ray structural analysis. However, analyses of DCLs by X-ray crystallography remains scarce as extensive data treatments are required and all dynamic systems are not suitable for such analyses.
Rheology
The recent extension of dynamic covalent chemistry to covalent polymers has opened a new route towards responsive materials.46 In particular, self-healing polymers have emerged as promising materials due to their capacity to repair themselves.47 Rheological measurements such as tensile tests, strain/recovery or stress relaxation experiments have been extensively used to demonstrate the self-healing behavior of such systems. In 2002, the group of Wudl reported the use of the thermoreversible Diels–Alder reaction to produce a thermosetting material which was characterized using fracture toughness tests to quantify the healing efficiency.48 However, although the polymer was successfully healed upon heating, the mechanical behavior could not be fully recovered. Some years later, following a similar strategy, Broekhuis and co-workers reported a thermally responsive cross-linked polymer whose self-healing ability was demonstrated using dynamic mechanical analyses and three-point bending tests.49 These analyses revealed that the nature and the amount of the cross-linking agents are highly important for reaching self-repairing polymers which display almost full recovery after thermal healing. Recently, the group of Leibler reported the topological rearrangement of polymeric networks without depolymerization using metal-catalyzed transesterification as the exchange process (Fig. 2c).50 Although the as-synthesized material presents all the properties of a classical hard epoxy resin at room temperature, samples that have been grinded and remolded at high temperatures were shown to display identical mechanical properties to the original material, as demonstrated by tensile tests. This new class of organic materials, so-called vitrimers, holds important promises as they display a reversible topological transition between a viscoelastic liquid state and an elastic solid. Further investigations demonstrated that these materials present welding properties over a broad range of temperature.51 As the presence of hydroxyl groups is crucial for the exchange reaction to occur, and considering that no consumption of chemical moieties occurs during the exchange process, this welding process can be repeated several times on the same samples without altering their robustness. The group of Guan in collaboration with Leibler and Tournilhac lately extended this work to olefin-containing polymer networks.52 By introducing small amounts of metathesis catalyst into a cross-linked polybutadiene, they demonstrated the adaptability of their network upon applying external forces to their samples. Cyclic strain recovery and stress relaxation experiments confirmed the malleability of the catalyst-loaded networks, without altering their mechanical properties. These rheological techniques clearly demonstrate their utility when reaching dynamic materials with potential applications.
Microscopies
Since DCC has been extended to large self-assemblies and materials, dynamic systems have been extensively imaged, mostly by using transmission electron microscopy (TEM) or atomic force microscopy (AFM), in order to understand the correlation between the evolution of the physical properties and the changes at the mesoscopic scale.53 However, the dynamic evolution of the library is difficult to follow in situ and, in most cases, only imaging of both the original state and the final state is provided. For example, the group of Ulijn used both AFM and TEM to demonstrate that the evolution of their DCL upon addition of an enzyme is associated with a morphological change from fibres to sheet-like structures.53a Interestingly, this structural evolution was well correlated with significant changes in fluorescence emission. In 2009, the group of Giuseppone developed the first example of dynamic combinatorial chemistry on surfaces.54 In that particular case, confocal microscopy was used to determine the distribution of the grafted molecules at different points on the surface. Using an aldehyde-coated quartz surface, it was demonstrated that avidin and streptavidin, which differ mainly by their arginine/lysine content, can be selectively anchored on the surface at different positions when varying the pH from 4 to 12. Recently, the groups of Spada and Samori demonstrated the successive interconversion between G ribbon and G quartet in situ using scanning tunneling microscopy (STM) (Fig. 2d).55 Upon addition of potassium picrate to the G ribbon, the quartet motif is formed. Further addition of [2.2.2] cryptand to sequester the potassium ions induces the recovery of G ribbon structures. Interestingly, potassium ions could be released from the cryptate to induce the regeneration of the G quartet assembly. This work represents the first example of in situ monitoring of dynamers at the surface and clearly opens new ways to follow the structural evolution of DCLs at the solid–liquid interface.
Theoretical modelling and statistical data treatment
Theoretical modelling
The application of a DCC approach to a large variety of research areas implies a significant evolution of the analytical techniques used to determine the composition of the library. Nevertheless, a better understanding of the fundamentals ruling such a dynamic process should be as essential for the design of the DCL as the analytical techniques used to identify the experimental library members.
The selection and amplification of specific species among all the possible virtual ones depend on the system itself and on its intrinsic molecular recognition pattern.56 However, the dynamic nature of the constitutive reversible interactions makes such supramolecular structures responsive to external factors, with the product distribution reflecting, in principle, the best answer of the mixture to that specific stimulus. One of the main features to consider for the design of a library is its size. From a practical point of view, small libraries are experimentally more accessible than larger ones, mainly due to the increasing complexity of the resulting mixture.57 If the number of building blocks is large, the resulting number of virtual combination is even larger and the detection of all possible products becomes problematic. In these conditions, the accuracy of the analysis relies mainly on the sensitivity of the available techniques, which might induce low resolution of members' amplification and false positive results.
One of the most classical application of the DCC concept deals with the generation of virtual dynamic libraries of receptors for a given substrate or, in contrast, virtual libraries of substrates for a given receptor. The final task of such an approach is, thus, the identification of the best binder and its amplification within the library. However, the composition of a DCL is thermodynamically driven, thus the amplification process of the library members tends to occur in such a way that the free energy of the overall system will be minimized, and this is not always in agreement with the selection of the fittest component.58 By means of numerical simulations on minimal model systems, Severin has demonstrated that the initial concentration of the template is a critical parameter to avoid the occurrence of such a false positive.5,59 When the library originates from only one type of monomer, thus leading to homo-oligomers, the size distribution of the resulting macrocycles, i.e. receptors, depends on their relative affinity for the template. However, in the presence of an excess of template, the system tends to maximize the global binding energy and smaller macrocycles are preferred to larger ones, regardless of the individual relative binding constants. On the other hand, when the library originates from two different types of monomer, either homo- or hetero-oligomers can be formed. In this case, when an excess of the template is present, the formation of hetero-oligomers tends to be favoured. Whereas Severin described a poor correlation between amplification and binding stability in the case of small DCLs, Corbett, Otto, and Sanders explored the same phenomenon for larger DCLs. To this end, they developed a computational methodology by which the experimental settings can be systematically varied, thus the best conditions to optimize the amplification–affinity correlation can be identified.60 Simulations were carried out using a purpose-written software, i.e. DCLSim, and virtual libraries of cyclic dimers to tetramers were generated starting from seven building blocks, both in the presence and absence of a template. Binding constant values for each receptor–template complex were randomly assigned from a normal distribution, setting a mean value for log
K at 2 and a standard deviation of 1. Different DCL scenarios were observed when the experimental conditions, i.e. building blocks and template concentrations, or the random distribution of the binding affinities over the library members were applied to simulations (Fig. 3a). The competition for building blocks between different receptors resulted to be a fundamental issue for the interpretation of the amplification output. In the absence of a template, smaller oligomers are preferred over larger ones, mainly due to entropic reasons. However, upon addition of a template, the amplification of the library members depends on both their initial concentration (i.e. without template) and the difference in affinity of each receptor for the template. If both a large and small receptor compete for the same building block, and the binding affinities to the template favour the larger one only to a small extent, the addition of a template does not lead to significant variation in the concentration of the species. Hence, small amplification factors are observed, i.e. the larger receptor which is a better binder is not amplified. On the other hand, if the difference in binding affinities to the template is more remarkable and the larger receptor is favoured, the higher binding energy compensates for the competition and the concentration of the larger oligomer increases. Under these conditions, the corresponding amplification factor correlates with the best binder. To reduce this effect and optimize the correlation between host–guest binding and amplification factors, a general template to building blocks ratio of 1
:
10 has been proposed which forces the individual receptors to compete for the template rather than for building blocks. Similarly, Otto et al. used the DCLSim software to analyse the effect of increasing the number of building blocks in a DCL. Computer simulations suggested that, even if the detection efficiency falls with increasing library size, larger DCLs enhance the probability of forming strong binders.61 An opposite approach has been later described by Sanders and Otto et al. where the affinities of a library of receptors for various templates became the output of the computer simulations performed with a second purpose-written software, i.e. DCLFit.62 By using the changes in the concentration of the library constituents as input, the proposed method allows for the determination of the binding energies without isolating the individual species (Fig. 3b).
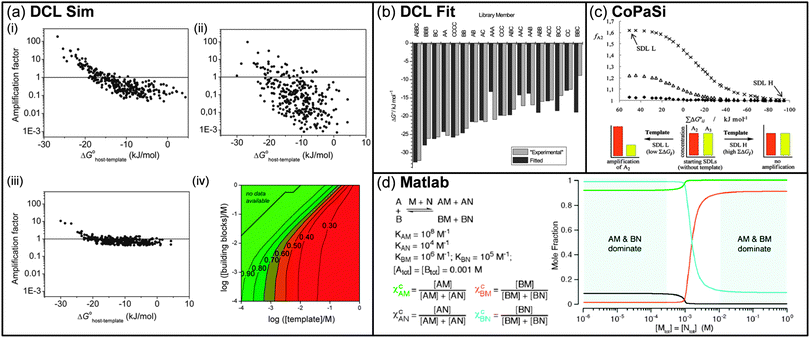 |
| Fig. 3 (a) Correlation between binding affinity and amplification factor in randomly generated dynamic combinatorial libraries using the software DCLSim. Panels (i) and (ii) have been obtained by varying the distribution of the binding constants over the library members (template concentration = 10 mM). Panel (iii) shows a simulation of the DCL in panel (ii) at a reduced template concentration (1.0 mM). Panel (iv) shows the correlation (described by the corresponding R2) between binding affinity and amplification factor as a function of template and total building block concentration. (b) Comparison between known binding affinities (light grey) and predicted values (dark grey) using the library distribution as input for the software DCLFit. (c) Amplification factor of the dimer A2 (fA2) upon addition of templates T1 (◆), T2 (△) and T3 (×) (binding constants: K2T3 > K2T2 > K2T1) as a function of the global free energy associated with all possible intermolecular interactions between library members (dimers and trimers). (d) Distribution of the four members of a library composed of two receptors (A and B) and two ligands (M and N) showing different relative affinities. The plot shows how the distribution of the four complexes can vary with increasing ligand concentration due to an induced-partner displacement mechanism. (Reproduced/adapted with permission from (a) ref. 60c, Copyright 2012, American Chemical Society; (b) ref. 2c, Copyright 2013, American Chemical Society; (c) ref. 63, Copyright 2010, American Chemical Society; (d) ref. 64, Copyright 2004, American Chemical Society.) | |
Recently, Furlan et al. investigated the effect of intermolecular interactions between library members on the amplification factor (Fig. 3c).63 In particular, several libraries of homo-dimers and -trimers were simulated assuming that each member of the library can interact with the other (i.e. dimer–dimer, trimer–trimer and dimer–trimer). The global free energy associated with this network of intermolecular interactions was varied for each simulation and further used as input to determine the amplification factor. If only the dimer is considered as a possible receptor for the template, the amplification factor decreases with increasing global free energy. In other words, the more competitive the receptor–receptor interactions are, the smaller the receptor–template population will be. Similarly, if more than one receptor with increasing affinity for the template is considered, the amplification of the best binder is achieved only when the intermolecular interactions between receptors are poorly competitive. Moving to negative values of the global free energy, i.e. strong receptor–receptor competition leads to no amplification or amplification of poor binders.
DCLs can also be referred as dynamic networks of compounds which are interconnected to each other. Isaacs et al. simulated the behaviour of a library composed of two receptors and two ligands able to form four host–guest combinations which distribution depends on the relative affinity of each receptor for each ligand (Fig. 3d).64 If both hosts can bind both guests, the more stable combination will be favoured, inducing the interaction between the remaining host and the remaining guest. However, in the presence of an excess of the two ligands, the more stable complex will form but the second host can still choose between the two guests, hence it will show independency and will form mainly its more stable host–guest complex. In a more general fashion, this kind of relationship was described by Lehn in terms of agonism and antagonism.65 The amplification of a specific member can be associated to the induced amplification of the corresponding agonist member and, at the same time, to the induced decrease of the corresponding antagonists. This scheme can be represented as a square network where the library components are placed at the vertices, diagonals connecting the corresponding agonists and edges connecting the corresponding antagonists. Three-dimensional networks are less common than two-dimensional ones. However, they are useful to describe systems which are subject to phase separation processes. In this case, a two-phase system can be described by a cube where the two opposite faces correspond to each of the phases of the system.66
Statistical data analysis
Analytical techniques such as UV-Vis and fluorescence spectroscopies can provide a large amount of data in a single measurement. Although each single molecule can be associated to a specific spectrum, discrimination between structure-related compounds is not always possible by mere comparison of the corresponding spectra. Similarly, resolution of mixtures into their individual components is all but a straightforward task for the experimentalist by a simple observation of the spectral profiles. Under these premises, multivariate statistical methods represent a valuable tool for the treatment of complex chemical databases and the interpretation of multivariate spectroscopic data. The goal of such an approach, otherwise known as chemometrics, is mainly discrimination and classification. The key idea is that in order to discriminate and classify diverse objects, distinctive patterns in the raw data need to be recognized. Multivariate analysis allows us to explore these patterns, and a new ambit in the field of analytical chemistry emerges such as the pattern recognition analysis. This is usually accomplished using different techniques: unsupervised methods, such as Principal Component Analysis (PCA) and Cluster Analysis (CA), and supervised methods, such as Linear Discriminant Analysis (LDA) and Artificial Neural Network (ANN), to name a few.67
The aim of PCA is mainly to disclose underlying information from multivariate raw data, reducing the original dataset to a smaller and more accessible one, revealing in this way the main trends. Note that the correlation between variables is a condicio sine qua non and this technique cannot be usefully applied if such a correlation is missing. Therefore, for linear combinations of the original variables, a new set of latent variables can be created, i.e. Principal Components (PCs). Among them, those which account for the largest variations are chosen, so that the number of original variables is reduced to a smaller number of PCs. In other words, an n-dimensional space is reduced when the selected PCs correspond to the new coordinates. CA includes a variety of methods to separate the objects of a mixture into classes, so that similar objects are grouped in the same class or cluster. The relationship between different objects in the n-dimensional space can be expressed in terms of Euclidian distance, and objects which are close in space can join to form a cluster. Among all the techniques, the simplest way to classify objects starts from clusters of size-one, i.e. each object forms a cluster, and such a process can proceed in a stepwise manner. Two size-one clusters can be combined to form a size-two cluster, which in turn can be combined to a third cluster to form a new bigger one, and so on up to one cluster containing all objects. The results can be represented by a dendrogram, a tree structure representing the complete hierarchy of partitions. Similar to CA, the aim of the k-means method is to classify the objects. However, whereas CA is a hierarchical method, i.e. the assignment of each object to a cluster is not reversible, the k-means is a non-hierarchical method, and a continuous rearrangement of the objects is carried out to find the best distribution. Here, k non-specific clusters, each associated to a centroid, are identified, and the objects are then assigned to the cluster whose centroid is the nearest. PCA and clustering are techniques for unsupervised pattern recognition, meaning that there is no prior definition of groups. On the other hand, LDA is a supervised pattern recognition method, meaning that a certain number of groups are generated using a training (or learning) set of known objects, and these predefined groups are then used in order to allocate unknown objects. The aim of LDA is to find a linear combination of the original variables, i.e. linear discriminant function (LDF). Different classes of objects will show very different LDF whereas objects belonging to the same group will have similar LDF. The most common approach is the so-called cross-validation, by means of which the LDF is found leaving one of the objects out of the training set, hence testing the correct allocation of the omitted object. The structure of an ANN reminds the organization of a biological neural network, where neurons receive an input signal via the dendrites, and transmit an output signal through the axons. Similarly, ANNs are formed by an input and an output layer connected by a number of intermediate or hidden layers. The input data, i.e. our known information, are processed in the intermediate layers to produce output data, i.e. the desired unknown information.
As already stated, multivariate analysis is the required approach to discriminate and classify different objects in complex sets. Therefore, many research groups applied multivariate analysis to the development of chemo-sensing systems which aim at the detection and identification of the diverse analytes in a multi-component solution, both in a qualitative and a quantitative manner. In this context, a very interesting application of DCLs as sensors has been firstly reported by Anslyn et al.68 Using Indicator Displacement Assays (IDAs) based on two cationic receptors and two different dyes, various sensors have been constructed for the discrimination of different analytes. Both dyes can bind to both receptors, thus a multicomponent sensor is formed by the four resulting dye–receptor complexes. The addition of analytes which are able to compete with the dyes for the binding to the receptors causes a change in the UV-Vis spectrum of the solution (note that free and bound dyes have divergent colours, hence different UV-Vis spectra). The information about the analytes is, therefore, distributed over the whole spectrum. The absorptions associated with a range of wavelengths are used as input data for the multivariate analysis which will emphasize the most indicative variations for the discrimination process.
Similarly, Severin conceived sensing systems based on IDAs where the involved dyes make complexes with metals (Fig. 4a). A large variety of analytes were used to prove the validity of this approach, e.g. dipeptides,69a,f tripeptides,69b,f nucleotides and phosphates,69c peptide hormones,69d and sulphated glycosaminoglycans.69e
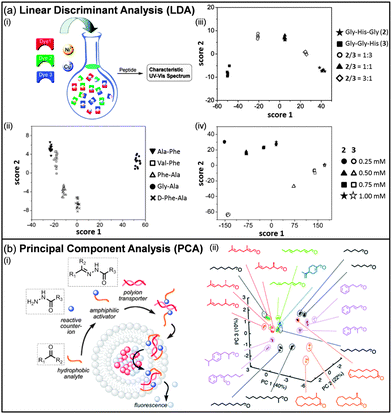 |
| Fig. 4 (a) Two-dimensional LDA score plots for the analysis of peptides using a sensor based on a dynamic combinatorial library of metal–dye complexes (i). Discrimination of dipeptides (ii) and tripeptides at different ratios (iii) and concentrations (iv) can be achieved. (b) PCA score plot for the analysis of odorants using a sensor based on a synthetic transport system. (i) Hydrophilic hydrazides are coupled with hydrophobic aldehydes/ketones (odorants) to obtain an amphiphilic activator for polyionic transporters in fluorogenic vesicles. (ii) The different activation promoted by the amphiphilic activators generates the pattern. (Reproduced/adapted with permission from (a) ref. 69a and b, Copyright 2005, Wiley-VCH and Copyright 2006, American Chemical Society; (b) ref. 70d, Copyright 2013, Royal Society of Chemistry.) | |
Matile et al. described the use of counterion-activated polyion transporters in fluorogenic vesicles for the differential sensing of hydrophobic analytes (Fig. 4b).70 The in situ condensation between charged hydrophilic hydrazides and hydrophobic aldehydes/ketones leads to the formation of charged amphiphilic acylhydrazones, which can interact with oppositely charged polyions and activate them as transporters in lipid bilayer membranes. The mediated transport through the membrane of a quencher will be detected as an increased emission of the internal fluorophores. Such a response varies depending on the hydrophobic analyte, e.g. odorants and perfumes, used to form the amphiphilic activator and multivariate methods are used to generate patterns.
Finally, Otto has recently reported the application of multivariate methods to a dataset obtained by LC-MS from the adaptation responses of a DCL.71 Dithiol building blocks were used to construct a library of cyclic receptors for the binding of amines and ammonium ions. The amplification factors of the various receptors upon addition of different ligands were used as input data for a clustering analysis. As a result, two classes of molecules were identified; allowing the assessment of molecular similarity in the set of proposed ligands.
Concluding remarks and perspectives
As illustrated by this short survey of the literature, the understanding of dynamic combinatorial libraries is highly dependent on progresses in the analytical techniques available, and in the opportunity to combine them. One can clearly see that these tools can act independently or in association at different levels of the libraries. The key factors to consider for selecting the appropriate techniques are: the size of the library (number of constituents), the length scale of the observation (molecular composition, mesoscale structuration, or macroscopic properties), and the rate of the recombination (time scale resolution). The pointed difficulties to enlarge the size of the libraries were among the strongest arguments to state on the limitations of these systems. Indeed, individual analysis of the constituents of the libraries is a very important challenge which has been discussed in numerous examples here. In addition, entropy is of detrimental effect in leading and precisely identifying amplification phenomena, and it was earlier seen as an important drawback in this type of approach. However, and interestingly, recent progresses tell us that the number of constituents involved in dynamic combinatorial libraries can be clearly overcome by various adapted experimental techniques (such as mass spectroscopy), experimental conditions (amplification from virtual libraries), and also by theoretical modelling. Even when the signals are fully convoluted in complex mixtures, such as is the case in some optical measurements, statistical analyses have been proved to be of high efficiency to extract quantitative data on single components. The length scale of observation has also been enlarged recently, going from small molecules (and necessitating local probes to extract binding constants for instance) to larger self-assemblies. This was in part motivated by the implementation of dynamic combinatorial systems in materials science.2b Thus, scattering techniques and various microscopies have shown their potential, although not yet fully exploited. Going further, macroscopic properties of materials have also been instrumental to understand the dynamics of adaptive and healable materials (by using rheology for instance). Importantly, the connections between the length scales can also provide a number of information by linking the molecular composition to higher levels of hierarchical organization. DOSY NMR is for instance very useful and more techniques would be needed to progress in this direction. Finally, time resolution is the third crucial aspect in the analysis of these mixtures. It is of high importance to have such information in order to understand the mechanistic pathways of reorganisations in networks of reactions and of supramolecular assemblies. This is true for understanding the mechanisms in the network itself, leading to the amplification of bioactive compounds for instance, but also to take control over relaxation times in such systems and to make use of them in materials science. We believe that this resolution in time is still lacking in a number of analytical techniques at all scales, and that progresses in this direction would be of great benefit to the field of dynamic combinatorial chemistry.
Overall, as experimental and theoretical tools progress, they offer new possibilities to study ever more complex mixtures and to extract crucial structural and dynamic information from the different components interacting in mixtures. One can predict that chemists will need more performing techniques in the future to implement the emerging field of systems chemistry, possibly far from thermodynamic equilibrium, and where an increase in spatial and temporal resolutions will be mandatory.72
Acknowledgements
We thank the European Research Council under the European Community's Seventh Framework Program (FP7/2007-2013)/ERC Starting Grant agreement no. 257099. We also thank a Marie Curie Career Integration Grant for a postdoctoral fellowship (Y.R.). We acknowledge the University of Strasbourg (UdS), the Centre National de la Recherche Scientifique (CNRS), the international center for Frontier Research in Chemistry (icFRC), the Laboratory of Excellence for Complex Systems Chemistry (LabEx CSC) and the Institut Universitaire de France (IUF).
Notes and references
-
(a) C. Seger, S. Sturma and H. Stuppner, Nat. Prod. Rep., 2013, 30, 970 RSC;
(b) B. K. Lavine and J. Workman Jr., Anal. Chem., 2013, 85, 705 CrossRef CAS PubMed;
(c)
Techniques, in Supramolecular Chemistry: From molecules to nanomaterials, ed. P. A. Gale and J. W. Steed, John Wiley & Sons, Chichester, UK, 2012, vol. 2 Search PubMed;
(d)
C. A. Schalley, Analytical Methods in Supramolecular Chemistry, 2nd edn, Completely Revised and Enlarged Edition, John Wiley & Sons, Ltd., 2012 Search PubMed.
-
(a) J.-M. Lehn, Top. Curr. Chem., 2012, 322, 1 CrossRef CAS;
(b) E. Moulin, G. Cormos and N. Giuseppone, Chem. Soc. Rev., 2012, 41, 1031 RSC;
(c) J. Li, P. Nowak and S. Otto, J. Am. Chem. Soc., 2013, 135, 9222 CrossRef CAS PubMed;
(d) N. Giuseppone, Acc. Chem. Res., 2012, 45, 2178 CrossRef CAS PubMed;
(e) M. Barboiu, Chem. Commun., 2010, 46, 7466 RSC.
-
(a) Y. Ruff, V. Garavini and N. Giuseppone, J. Am. Chem. Soc., 2014, 136, 6333–6339 CrossRef CAS PubMed;
(b) A. Herrmann, Chem. Soc. Rev., 2014, 43, 1899 RSC;
(c) I. Huc and J.-M. Lehn, Proc. Natl. Acad. Sci. U. S. A., 1997, 94, 2106 CrossRef CAS.
- S. Zameo, B. Vauzeilles and J.-M. Beau, Eur. J. Org. Chem., 2006, 5441 CrossRef CAS.
-
(a) Z. Grote, R. Scopelliti and K. Severin, Angew. Chem., Int. Ed., 2003, 42, 3821 CrossRef PubMed;
(b) I. Saur and K. Severin, Chem. Commun., 2005, 1471 RSC.
- L. Roy and M. A. Case, J. Phys. Chem. B, 2011, 115, 2454 CrossRef CAS PubMed.
-
(a) J. F. Folmer-Andersen and J.-M. Lehn, Angew. Chem., Int. Ed., 2009, 48, 7664 CrossRef CAS PubMed;
(b) J. F. Folmer-Andersen and J.-M. Lehn, J. Am. Chem. Soc., 2011, 133, 10966 CrossRef CAS PubMed.
- D. A. Fulton, Org. Lett., 2008, 10, 3291 CrossRef CAS PubMed.
- A. P. Bapat, J. G. Ray, D. A. Savin and B. S. Sumerlin, Macromolecules, 2013, 46, 2188 CrossRef CAS.
- P. Besenius, P. A. G. Cormack, R. F. Ludlow, S. Otto and D. C. Sherrington, Org. Biomol. Chem., 2010, 8, 2414 CAS.
- A. Herrmann, Chem. – Eur. J., 2012, 18, 8568 CrossRef CAS PubMed.
-
C. F. Ross, 2.02 - Headspace Analysis, in Comprehensive Sampling and Sample Preparation, ed. J. Pawliszyn, Academic Press, Oxford, 2012, p. 27 Search PubMed.
-
(a) A. Trachsel, C. Chapuis and A. Herrmann, Flavour Fragrance J., 2013, 28, 280 CrossRef CAS;
(b) B. Buchs, G. Godin, A. Trachsel, J.-Y. de Saint Laumer, J.-M. Lehn and A. Herrmann, Eur. J. Org. Chem., 2011, 681 CrossRef CAS.
-
(a) B. Levrand, W. Fieber, J.-M. Lehn and A. Herrmann, Helv. Chim. Acta, 2007, 90, 2281 CrossRef CAS;
(b) A. Herrmann, N. Giuseppone and J.-M. Lehn, Chem. – Eur. J., 2009, 15, 117 CrossRef CAS PubMed.
-
(a) A. Pałka, R. Musioł, M. Matlengiewicz, M. Sajewicz, K. Serafin and J. Polański, Acta Chromatogr., 2006, 17, 314 Search PubMed;
(b) M. Dal Molin, G. Gasparini, P. Scrimin, F. Rastrelli and L. J. Prins, Chem. Commun., 2011, 47, 12476–12478 RSC;
(c) K. K. Larson, M. He, J. F. Teichert, A. Naganawa and J. W. Bode, Chem. Sci., 2012, 3, 1825 RSC.
- Y. Cohen, L. Avram and L. Frish, Angew. Chem., Int. Ed., 2005, 44, 520 CrossRef CAS PubMed.
- K. A. Heisel, J. J. Goto and V. V. Krishnan, Am. J. Anal. Chem., 2012, 3, 401 CrossRef CAS.
- N. Giuseppone, J.-L. Schmitt, L. Allouche and J.-M. Lehn, Angew. Chem., Int. Ed., 2008, 47, 2235 CrossRef CAS PubMed.
- R. Nguyen, L. Allouche, E. Buhler and N. Giuseppone, Angew. Chem., Int. Ed., 2009, 48, 1093 CrossRef CAS PubMed.
- B. Meyer and T. Peters, Angew. Chem., Int. Ed., 2003, 42, 864 CrossRef CAS PubMed.
- R. Caraballo, H. Dong, J. P. Ribeiro, J. Jiménez-Barbero and O. Ramström, Angew. Chem., Int. Ed., 2010, 49, 589 CrossRef CAS PubMed.
- B. J. Stockman and C. Dalvit, Prog. Nucl. Magn. Reson. Spectrosc., 2002, 41, 187 CrossRef CAS.
- P. Kovaříček and J.-M. Lehn, J. Am. Chem. Soc., 2012, 134, 9446 CrossRef PubMed.
- N. Giuseppone, J.-L. Schmitt and J.-M. Lehn, J. Am. Chem. Soc., 2006, 128, 16748 CrossRef CAS PubMed.
- R. F. Ludlow and S. Otto, J. Am. Chem. Soc., 2008, 130, 12218 CrossRef CAS PubMed.
- F. B. L. Cougnon, H. Y. Au-Yeung, G. D. Pantos and J. K. M. Sanders, J. Am. Chem. Soc., 2011, 133, 3198 CrossRef CAS PubMed.
-
(a) S. A. Poulsen, J. Am. Soc. Mass Spectrom., 2006, 17, 1074 CrossRef CAS PubMed;
(b) B. M. R. Liénard, N. Selevsek, N. J. Oldham and C. J. Schofield, ChemMedChem, 2007, 2, 175 CrossRef PubMed.
- E. C. Y. Woon, M. Demetriades, E. A. L. Bagg, W. S. Aik, S. M. Krylova, J. H. Y. Ma, M. C. Chan, L. J. Walport, D. Wegman, K. N. Dack, M. A. McDonough, S. N. Krylov and C. J. Schofield, J. Med. Chem., 2012, 55, 2173 CrossRef CAS PubMed.
- J. Wassenaar, E. Jansen, W.-J. van Zeist, F. M. Bickelhaupt, M. A. Siegler, A. L. Spek and J. N. H. Reek, Nat. Chem., 2010, 2, 417 CrossRef CAS PubMed.
- H. I. A. Phillips, A. V. Chernikov, N. C. Fletcher, A. E. Ashcroft, J. R. Ault, M. H. Fiby and A. J. Wilson, Chem. – Eur. J., 2012, 18, 13733 CrossRef CAS PubMed.
-
(a) Y.-T. Chan, X. Li, M. Soler, J.-L. Wang, C. Wesdemiotis and G. R. Newkome, J. Am. Chem. Soc., 2009, 131, 16395 CrossRef CAS PubMed;
(b) X. Li, Y.-T. Chan, M. Casiano-Maldonado, J. Yu, G. A. Carri, G. R. Newkome and C. Wesdemiotis, Anal. Chem., 2011, 83, 6667 CrossRef CAS PubMed.
-
K. Severin, in Dynamic Combinatorial Chemistry, ed. J. N. H. Reek and S. Otto, Wiley-VCH Verlag GmbH & Co. KGaA, Weinheim, Germany, 2010, p. 169 Search PubMed.
- S. Fujii and J.-M. Lehn, Angew. Chem., Int. Ed., 2009, 48, 7635 CrossRef CAS PubMed.
-
(a) N. Giuseppone and J.-M. Lehn, J. Am. Chem. Soc., 2004, 126, 11448 CrossRef CAS PubMed;
(b) N. Giuseppone, G. Fuks and J.-M. Lehn, Chem. – Eur. J., 2006, 12, 1723 CrossRef CAS PubMed.
- T. Ono, S. Fujii, T. Nabori and J.-M. Lehn, Chem. Commun., 2007, 4360 RSC.
- Y. Ruff and J.-M. Lehn, Angew. Chem., Int. Ed., 2008, 47, 3556 CrossRef CAS PubMed.
- J. P. Daguer, M. Ciobanu, S. Alvarez, S. Barluenga and N. Winssinger, Chem. Sci., 2011, 2, 625 RSC.
- S. Shinoda, K. Terada and H. Tsukube, Chem. – Eur. J., 2012, 7, 400 CAS.
- S. K. M. Nalluri and R. V. Ulijn, Chem. Sci., 2013, 4, 3699 RSC.
- C. B. Minkenberg, F. Li, P. van Rijn, L. Florusse, J. Boekhoven, M. C. A. Stuart, G. J. M. Koper, R. Eelkema and J. H. van Esch, Angew. Chem., Int. Ed., 2011, 50, 3421 CrossRef CAS PubMed.
- A. P. Bapat, D. Roy, J. G. Ray, D. A. Savin and B. S. Sumerlin, J. Am. Chem. Soc., 2011, 133, 19832 CrossRef CAS PubMed.
- R. Nguyen, E. Buhler and N. Giuseppone, Macromolecules, 2009, 42, 5913 CrossRef CAS.
- N. Jouault, R. Nguyen, M. Rawiso, E. Buhler and N. Giuseppone, Soft Matter, 2011, 7, 4787 RSC.
- M. S. Congreve, D. J. Davis, L. Devine, C. Granata, M. O'Reilly, P. G. Wyatt and H. Jhoti, Angew. Chem., Int. Ed., 2003, 42, 4479 CrossRef CAS PubMed.
- M. Barboiu, F. Dumitru, Y.-M. Legrand, E. Petit and A. van der Lee, Chem. Commun., 2009, 2192 RSC.
- R. J. Wojtecki, M. A. Meador and S. J. Rowan, Nat. Mater., 2011, 10, 14 CrossRef CAS PubMed.
- Y. Yang and M. W. Urban, Chem. Soc. Rev., 2013, 42, 7446 RSC.
- X. Chen, A. D. Matheus, O. Kanji, M. Ajit, S. Hongbin, S. R. Nutt, K. Sheran and F. Wudl, Science, 2002, 295, 1698 CrossRef CAS PubMed.
- Y. Zhang, A. A. Broekhuis and F. Picchioni, Macromolecules, 2009, 42, 1906 CrossRef CAS.
- D. Montarnal, M. Capelot, F. Tournilhac and L. Leibler, Science, 2011, 334, 965 CrossRef CAS PubMed.
- M. Capelot, D. Montarnal, F. Tournilhac and L. Leibler, J. Am. Chem. Soc., 2012, 134, 7664 CrossRef CAS PubMed.
- Y.-X. Lu, F. Tournilhac, L. Leibler and Z. Guan, J. Am. Chem. Soc., 2012, 134, 8424 CrossRef CAS PubMed.
-
(a) R. J. Williams, A. M. Smith, R. Collins, N. Hodson, A. K. Das and R. V. Ulijn, Nat. Nanotechnol., 2009, 4, 19 CrossRef CAS PubMed;
(b) J. Li, J. M. A. Carnall, M. C. A. Stuart and S. Otto, Angew. Chem., Int. Ed., 2011, 50, 8384 CrossRef CAS PubMed;
(c) A. K. H Hirsch, E. Buhler and J.-M. Lehn, J. Am. Chem. Soc., 2012, 134, 4177 CrossRef PubMed.
- L. Tauk, A. Schröder, G. Decher and N. Giuseppone, Nat. Chem., 2009, 1, 649 CrossRef CAS PubMed.
- A. Ciesielski, S. Lena, S. Masiero, G. P. Spada and P. Samori, Angew. Chem., Int. Ed., 2010, 49, 1963 CrossRef CAS PubMed.
- M. Hochgürtel, H. Kroth, D. Piecha, M. W. Hofmann, C. Nicolau, S. Krause, O. Schaaf, G. Sonnenmoser and A. V. Eliseev, Proc. Natl. Acad. Sci. U. S. A., 2002, 99, 3382 CrossRef PubMed.
- J. S. Moore and N. W. Zimmerman, Org. Lett., 2000, 2, 915 CrossRef CAS.
-
(a) P. T. Corbett, J. K. M. Sanders and S. Otto, J. Am. Chem. Soc., 2005, 127, 9390 CrossRef CAS PubMed;
(b) P. T. Corbett, J. K. M. Sanders and S. Otto, Chem. – Eur. J., 2008, 14, 2153 CrossRef CAS PubMed.
- K. Severin, Chem. – Eur. J., 2004, 10, 2565 CrossRef CAS PubMed.
-
(a) P. T. Corbett, J. K. M. Sanders and S. Otto, Org. Lett., 2004, 6, 1825 CrossRef CAS PubMed;
(b) P. T. Corbett, J. K. M. Sanders and S. Otto, Chem. – Eur. J., 2004, 10, 3139 CrossRef CAS PubMed;
(c) S. Otto, Acc. Chem. Res., 2012, 45, 2200 CrossRef CAS PubMed.
- R. F. Ludlow and S. Otto, J. Am. Chem. Soc., 2010, 132, 5984 CrossRef CAS PubMed.
- R. F. Ludlow, J. Liu, H. Li, S. L. Roberts, J. K. M. Sanders and S. Otto, Angew. Chem., Int. Ed., 2007, 46, 5762 CrossRef CAS PubMed.
- A. G. Orrillo and R. L. E. Furlan, J. Org. Chem., 2010, 75, 211 CrossRef CAS PubMed.
- P. Mukhopadhyay, A. Wu and L. Isaacs, J. Org. Chem., 2004, 69, 6157 CrossRef CAS PubMed.
-
(a) N. Giuseppone and J.-M. Lehn, Chem. – Eur. J., 2006, 12, 1715 CrossRef CAS PubMed;
(b) S. Xu and N. Giuseppone, J. Am. Chem. Soc., 2008, 130, 1826 CrossRef CAS PubMed;
(c) J.-M. Lehn, Angew. Chem., Int. Ed., 2013, 52, 2836 CrossRef CAS PubMed.
- N. Hafezi and J.-M. Lehn, J. Am. Chem. Soc., 2012, 134, 12861 CrossRef CAS PubMed.
-
(a)
R. G. Brereton, Applied Chemometrics for Scientists, John Wiley & Sons, Ltd., 2007 Search PubMed;
(b)
J. N. Miller and J. C. Miller, Statistics and Chemometrics for Analytical Chemistry-Sixth Edition, Pearson Education Ltd., 2010 Search PubMed.
-
(a) S. L. Wiskur, P. N. Floriano, E. V. Anslyn and J. T. McDevitt, Angew. Chem., Int. Ed., 2003, 42, 2070 CrossRef CAS PubMed;
(b) S. C. McCleskey, P. N. Floriano, S. L. Wiskur, E. V. Anslyn and J. T. McDevitt, Tetrahedron, 2003, 59, 10089 CrossRef CAS PubMed.
-
(a) A. Buryak and K. Severin, Angew. Chem., Int. Ed., 2005, 44, 7935 CrossRef CAS PubMed;
(b) A. Buryak and K. Severin, J. Comb. Chem., 2006, 8, 540 CrossRef CAS PubMed;
(c) A. Buryak, A. Pozdnoukhov and K. Severin, Chem. Commun., 2007, 2366 RSC;
(d) F. Zaubitzer, T. Riis-Johannessen and K. Severin, Org. Biomol. Chem., 2009, 7, 4598 RSC;
(e) P.-K. Müller-Graff, H. Szelke, K. Severin and R. Krämer, Org. Biomol. Chem., 2010, 8, 2327 RSC;
(f) S. Rochat and K. Severin, J. Comb. Chem., 2010, 12, 595 CrossRef CAS PubMed.
-
(a) J. Montenegro, P. Bonvin, T. Takeuchi and S. Matile, Chem. – Eur. J., 2010, 16, 14159 CrossRef CAS PubMed;
(b) J. Montenegro and S. Matile, Chem. – Asian J., 2011, 6, 681 CrossRef CAS PubMed;
(c) T. Takeuchi, J. Montenegro, A. Hennig and S. Matile, Chem. Sci., 2011, 2, 303 RSC;
(d) T. Takeuchi and S. Matile, Chem. Commun., 2013, 49, 19 RSC.
- V. Saggiomo, Y. R. Hristova, R. F. Ludlow and S. Otto, J. Syst. Chem., 2013, 4, 2 CrossRef CAS.
- R. A. R. Hunt and S. Otto, Chem. Commun., 2011, 47, 847 RSC.
|
This journal is © The Royal Society of Chemistry and the Centre National de la Recherche Scientifique 2014 |