DOI:
10.1039/C3LC51281A
(Paper)
Lab Chip, 2014,
14, 1336-1347
Spatiotemporal control of gene expression using microfluidics†
Received
15th November 2013
, Accepted 16th January 2014
First published on 16th January 2014
Abstract
Accurate spatiotemporal regulation of genetic expression and cell microenvironment are both essential to epithelial morphogenesis during development, wound healing and cancer. In vivo, this is achieved through the interplay between intrinsic cellular properties and extrinsic signals. Amongst these, morphogen gradients induce specific concentration- and time-dependent gene expression changes that influence a target cell's fate. As systems biology attempts to understand the complex mechanisms underlying morphogenesis, the lack of experimental setup to recapitulate morphogen-induced patterning in vitro has become limiting. For this reason, we developed a versatile microfluidic-based platform to control the spatiotemporal delivery of chemical gradients to tissues grown in Petri dishes. Using this setup combined with a synthetic inducible gene expression system, we were able to restrict a target gene's expression within a confluent epithelium to bands of cells as narrow as four cell diameters with a one cell diameter accuracy. Applied to the targeted delivery of growth factor gradients to a confluent epithelium, this method further enabled the localized induction of epithelial–mesenchymal transitions and associated morphogenetic changes. Our approach paves the way for replicating in vitro the morphogen gradients observed in vivo to determine the relative contributions of known intrinsic and extrinsic factors in differential tissue patterning, during development and cancer. It could also be readily used to spatiotemporally control cell differentiation in ES/iPS cell cultures for re-engineering of complex tissues. Finally, the reversibility of the microfluidic chip assembly allows for pre- and post-treatment sample manipulations and extends the range of patternable samples to animal explants.
Introduction
Tissue patterning is key to morphogenesis during development and cancer. Understanding both its genetic and biophysical determinants holds promise to enable the re-engineering of complex tissues from embryonic (ESC) or induced-pluripotent (iPSC) stem cells, whether it is for scientific or medical purposes. To deal with the multi-parametric and multi-scale nature of developmental patterning and morphogenesis, systems biology approaches have been employed.1–3 However, progress has been hampered by the lack of suitable methods to experimentally test theoretical models of developmental and cancer morphogenesis.
During animal embryonic development and tumorigenesis, epithelia undergo complex morphogenetic transformations resulting from timed and spatially localized differences in gene expression. The well-studied vertebrate neural tube formation, whose failure is the second most common cause of human birth defects,4 provides many such examples. Localized expression of shroom family proteins allows neural plate invagination.5 Localized changes in cadherin expression promote neural tube closure and neural crest cell emigration.6 Along the rostral–caudal axis, spatially-restricted cyclic expression of clock genes drives somitogenesis,7 while neuron progenitors are patterned along the dorsal–ventral axis.8 Patterning is also essential post-developmentally to maintain tissue organization/morphostasis in rapidly turning-over epithelia such as the colon9 thereby preventing metaplasia.9,10 Upon injury, tissue healing processes reengage developmental morphogenetic pathways (e.g. amphibian limb regeneration11).
Tissue patterning, morphogenesis and morphostasis all rely on a complex interplay between extrinsic chemical or mechanical signals and intrinsic cellular properties. The best characterized extrinsic signals are morphogens, signalling entities that form gradients and induce distinct cellular responses depending on the dose and duration of target cell exposure.12 How far morphogens diffuse from their source depends on their initial concentration, the nature of their carrier and its binding kinetics to target cells. This versatility is well illustrated by morphogens of the Hedgehog family (Hh): Hh production levels vary over time; Hh can be carried by Hh multimers, lipoproteins, or vesicles; and binding to various receptors shapes Hh gradients over the field of exposed cells.8,13,14 Target cells can respond to various dose–time thresholds, each resulting in a different temporal gene expression profile and a distinct cell fate.8 Other diffusive developmental signals and morphostats are expected to act similarly.10,12 This complex regulation of extracellular signal propagation and intracellular signal integration gives rise to precise and robust gene expression patterns but renders morphogen gradient signalling difficult to dissect.
In vivo models have been instrumental in identifying essential processes that orchestrate morphogenesis and morphostasis. However, in vivo observations are clouded by underlying pre-patterns established during earlier developmental stages, and tuning in vivo patterning signals is often restricted to gross perturbations with poor spatiotemporal resolution. To gain a true systems understanding of morphogenetic and morphostatic mechanisms, in vitro experimental setups allowing for the exposure of naive tissues to precisely controlled spatiotemporal concentrations of morphogens are necessary.15 Micropatterning, optogenetics or LightON can afford various degrees of spatial patterning of cell subpopulations or timed gene expression induction,16–18 while microfluidic gradient generators can deliver defined chemical concentrations in a spatially and/or temporally controlled manner over short periods of time.19–23 However, the ability of microfluidic methods to control gene expression through the delivery of chemical gradients in living tissues has not been demonstrated. Such a setup would not only allow an in depth dissection of morphogenetic signalling networks15,24 but also enable re-engineering of developmental patterns, recapitulation of carcinogenesis, and the production of complex patterned tissues from ES or iPSC cells.15,24
Here, we present a versatile microfluidic approach relying on timed hydrodynamic flow focusing to precisely deliver gene expression modifier gradients to cells cultured in Petri dishes, with a one-cell diameter (1 cd) precision over durations of several hours. As proof of principle, we show how to pattern gene expression in a monolayer using the broadly used Tet-On system25 and how to locally induce changes in gene expression, epithelial polarity and morphology in monolayers using spatially restricted perfusion of the diffusive Hepatocyte Growth Factor (HGF) and Transforming Growth Factor beta 1 (TGFβ1).
Methods
Cell line generation and handling
Tet-On Madin-Darby Canine Kidney II cells (MDCK II cells26) were grown at 37 °C under a 5% CO2 atmosphere in Dulbecco's modified Eagle medium (DMEM) (high glucose (4.5 g L−1) + Glutamax without phenol red (Invitrogen, Paisley, UK)), supplemented with penicillin/streptomycin, 10% tetracycline-free foetal bovine serum (tet-free FBS, Clontech), and blasticidin (5 μg mL−1, Merck Biosciences, Nottingham, UK). The MLC-GFP construct was obtained from Prof Hiroshi Hosoya27 and cloned into pcDNA4/T0 (Invitrogen). Tet-On MDCK cells were then transfected with Lipofectamine 2000 (Invitrogen) following manufacturer's recommendations. Cells were further amplified and subcloned in zeocin (400 μg mL−1, Merck Biosciences) supplemented medium, in order to obtain the cell line used in this study, which exhibits very low levels of MLC-GFP expression in the absence of doxycycline and very high levels upon doxycycline addition. E-Cadherin was excised from E-Cadherin pBAT, cloned into EGFP-N1 (Takara-Clontech, CA, U.S.A), and inserted into the retroviral vector pRetroQAcGFPN1 (Takara-Clontech). Keratin18-GFP pLNCX was a kind gift from Prof Rudolf Leube (University of Aachen, Germany). α-Tubulin was excised from EGFP-C1 and inserted into the retroviral vector pRetroQAcGFPC1 (Takara-Clontech). Retroviruses were then generated by transfecting the plasmids into 293-GPG cells for packaging (a kind gift from Prof Daniel Ory, Washington University28). Retroviral supernatants were then used to infect wild type MDCK II cells. Cells were selected in the presence of 1 mg ml−1 G418 (Merck Biosciences, Nottingham, UK) (for pLNCX plasmids) or 500 ng ml−1 puromycin (for pRetroQ plasmids) for 2 weeks and subcloned to obtain a monoclonal cell line with an epithelial phenotype.
Microfabrication
Computer-aided design of microfluidic chip geometries was done using the AutoCAD 2009 software (Autodesk, Inc.). Emulsion masks were obtained from JD photo-tools (Oldham, Lancaster, UK). 3 inch silicon wafers were ordered from Virginia Semiconductor (Fredericksburg, VA, USA). Moulds were made from SU-8-2050 photoresist (MicroChem Corp./MCC, MA. USA) using standard microfabrication techniques.29 They were then plasma exposed (using a PLASMA clean 4, ILMVAC, UK), plunged in 1% tridecafluoro-1,1,2,2-tetrahydrooctyl-1-dimethylchlorosilane (13F, MCC) in toluene for 30 min, washed, dried and baked at 55 °C for 15 min. Poly-dimethyl, methylhydrogen siloxane (PDMS) casts were prepared by mixing 1
:
10 parts of the curing agent to the base Sylgard 184 elastomer (Dow Corning Corp. Midland, MI, USA). The PDMS mix was degassed and poured onto the 13F-coated patterned wafer and cured at 55 °C overnight. Then, inputs/outputs were created using a biopsy punch. For experiments in sealed chips, PDMS casts were exposed to plasma for 30 s and irreversibly bonded to glass coverslips. Finally, the assembled chips were baked for 15 min at 75 °C. For experiments in clamped chips, PDMS casts were sterilized by 30 s plasma exposure before use.
In silico simulations
Two-dimensional CAD microchip designs were imported using the MEMS module of Comsol Multiphysics 4.0a (Comsol, Stockholm, Sweden). Quasi-3D models were generated using the shallow-channel approximation, considering a channel height of 80 μm (corresponding to the typical height of our microfluidic chambers). We used the Navier–Stokes equations and the model for the transport of diffusive species to calculate flow dynamics and species concentrations in the chip under perfusion. Given the microscopic scale and the aqueous nature of the cell culture medium, the perfused fluid was considered Newtonian incompressible and in a laminar regime. Non-slip boundary conditions were applied to all microchannel walls. We applied laminar flow conditions at the inlets and outlet. The initial conditions for inlet/outlet pressures and tubing length corresponded to the values measured in a typical experiment at steady-state. For each chip design, flow dynamics and species concentrations were first determined at steady-state. The treatment width at each position along the channel was determined choosing a threshold of 10% of the initial input concentration. In further simulations, chip performances were compared by measuring the variation in the treatment width normalized to the treatment width at the entry of the microchamber after reaching steady state. The perturbations introduced in the simulation of chip perfusion were the following:
1) stepped variations in pressure (Fig. 1D): PinputN(t) = P0inputN + PvarN for 40 s < t < 100 s and PinputN(t) = P0inputN − PvarN for 100 s < t < 160 s, where P0inputN is the initial baseline pressure at inlet N and PvarN is the maximum amplitude of variation allowed at inlet N;
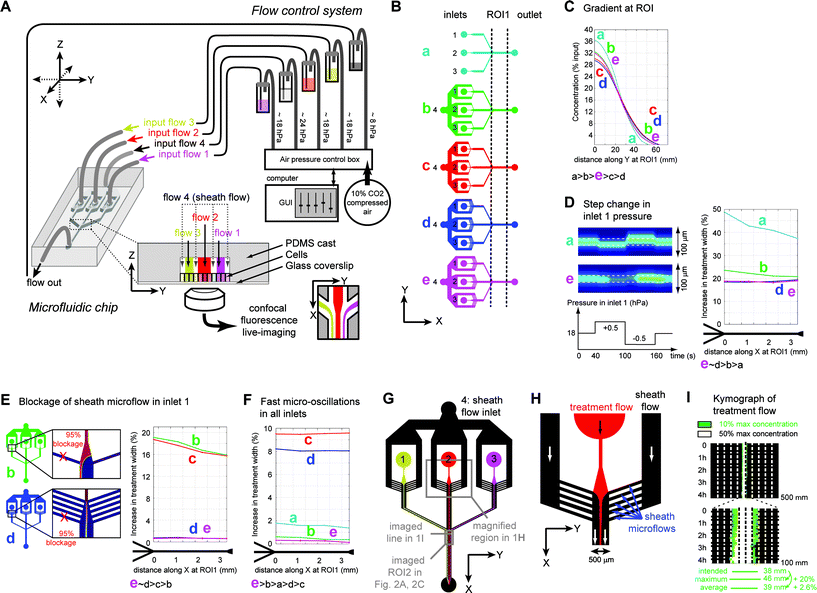 |
| Fig. 1 Setup and optimization of the microfluidic-imaging platform for hydrodynamic cell patterning. (A) Schematic illustration of the experimental setup. Cells were grown to confluence in-chip. Reservoirs containing perfusion media were independently pressurized, using a pressure-driven flow control system. Live-imaging was performed simultaneously to enable the visual assessment of the position and width of the treatment flow. (B) Chip designs tested in silico. (C) Effect of the chip design on the concentration gradient shape under steady-state conditions. Half of the concentration gradient across the channel width is plotted. Design “a” leads to the steepest gradient while design “d” leads to the shallowest. (D) Impact of step-changes in pressure on treatment flow positioning. Design “a” leads to the largest deviation while designs “d/e” lead to the smallest. (E) Effect of sheath flow blockage on treatment flow positioning. Design “b” leads to the largest deviation while designs “d/e” lead to the smallest. (F) Impact of pressure micro-oscillations on treatment flow positioning. Design “c” leads to the largest deviation while designs “e” leads to the smallest. (G) Optimized chip design. (H) Magnification of the treatment-flow stabilization zone. (I) Kymograph of treatment flow reveals that the maximum amplitude of experimentally observed oscillations is 20%, which leads to a less than 3% increase in the average treatment width. ROI: region of interest. | |
2) periodic low amplitude and short period variations in all inlets simultaneously (Fig. 1F): PinputN(t) = P0inputN + PvarN × sin(2πt/ΔtN), where ΔtN is the perturbation period for inlet N, 1/ΔtN = 1.0 Hz for N = 1, 1.2 Hz for N = 2, 1.1 Hz for N = 3, 1.5 Hz for N = 4, and PvarN = 0.8 × P0inputN/100;
3) a 3 s-long 95% blockage of a microchannel (Fig. 1E) applied by locally defining a channel depth of 5% the original depth (i.e. 4 μm for a 80 μm-deep channel) in the shallow channel approximation formula. For all simulations, we chose P0inputN = 18.7 hPa for N = 1 and N = 3, = 18.6 hPa for N = 2, = 24.2 hPa for N = 4, corresponding to empirically determined values.
In-chip cell culture
MDCK cells from a 95–100% confluent culture in a T75 flask were resuspended in trypsin/EDTA (TE), washed twice with culture medium, diluted in culture medium at a concentration of 50–100 million cells mL−1 and injected into the microfluidic chip through the output. Inputs and outputs were then blocked and the chips were kept in humid chambers in a 37 °C incubator under a 5% CO2 atmosphere. Cells were allowed to settle for 4–10 h. Then, 100–200 μL of fresh medium was perfused through the device. Afterwards, 100–200 μL of fresh medium was perfused every 24 h until confluence.
Perfusion media
All fluorescent dyes were obtained from Life technologies. Bodipy-TR Ceramide (excited at 633 nm), TRITC (excited at 543 nm), Hoechst 33342 (excited at 405 nm), 10 kDa dextran-Alexa647 (excited at 633 nm), 3 kDa dextran-cascade blue (excited at 405 nm) and doxycycline (Sigma-Aldrich) were dissolved in cell culture medium. All perfusion media were filtered at 0.22 μm and degassed under vacuum before each experiment.
Perfusion of irreversibly sealed microfluidic chips
We performed preliminary experiments using gravity flow, a syringe pump (Harvard Apparatus) or a micro-injector (Narishige IM-300, Narishige, Tokyo, Japan) to regulate inlet pressures; all failed to provide a stable solution for the parallel perfusion of 3 or more microflows (ESI Fig. S1†). To regulate independently the pressure in each inlet and outlet of the chip, we utilized an 8-channel pressure-driven flow control system (MFCS-8, Fluigent, Paris, France). To maintain physiological pH and oxygen levels of the cell culture medium during perfusion, we used 10% CO2 compressed air as our pressure source. A custom-made 8-channel holder hosted the reservoirs containing the perfusion media for each inlet and outlet. One day after cell confluence was reached in-chip, each inlet/outlet of the chip was connected to the appropriate reservoir using sterile tubing extensions of 30 cm primed with medium. Suitable inlet pressure values were established empirically, and the inlet delivering the treatment was connected last, ensuring a treatment-free perfusion setup. Once the closed-loop system was established, the flow was directly visualized by epifluorescence microscopy and pressures were adjusted (using the MFCS-8 software) to obtain the desired flow conditions. Treatment flow was established by gradually increasing the central input pressure until the desired treatment width was obtained. Typically, flow stability was obtained for pressures of ~18 mbar in inlets 1, 2, 3, ~24 mbar in inlet 4 and ~8 mbar in the outlet. In all of the experiments presented here, we used inlet 2 to deliver the treatment and inlets 1 and 3 to adjust the position of the treatment flow (flow focusing). In the Z-plane of the maximal signal intensity, confocal flow-imaging was calibrated using the inlet fluorescence to define saturation levels and the fluorescence outside the microchannels to determine the background levels and adjust the offset. Using these imaging settings for the ROI, treatment width was controlled visually by varying the pressure applied to inlet 2 (typically within ±1.5 mbar from pressures in inlets 1 and 3). Intended treatment width was defined by superimposing a scale bar (using the Olympus acquisition software F10-ASW viewer) on acquired images. Post-treatment analysis of treatment flow imaging allowed precise calculation of the input treatment width (see below).
Preparation of cell monolayers for perfusion with reversibly sealed chips
5 cm-diameter glass-bottom dishes were spin-coated with a ~100 μm thick PDMS layer (1
:
15 parts of the curing agent to the elastomer base), and baked overnight at 55 °C. After plasma cleaning, the dishes were coated with 10% aminopropyl-triethoxy-silane (APTES)/ethanol, followed by a 3%-glutaraldehyde/PBS treatment, and finally coated with 10 μg mL−1 type I collagen (R&D Systems) in PBS, according to the published procedures for covalent binding of collagen to PDMS.30 Following extensive washes with the cell culture medium, cells were seeded on the dishes and further handled using standard procedures.
Perfusion of reversibly sealed microfluidic chips. To achieve reversible tight seals between microfluidic chips and cells growing on Petri dishes, we designed a clamp adapted from Tkachenko et al.31 (Fig. 4A). Glass-bottom dishes containing 100% confluent cell monolayers were fitted into the clamp holder interfaced to the confocal imaging platform stage. The microfluidic chip was affixed to the upper half of the clamp and connected to the reservoirs and the flow-control system. The tubing and the chip were primed with the medium, and pressures were adjusted to keep inlets/outlet wetted. The two halves of the clamp were then assembled carefully to avoid trapping air bubbles between the chip and the cell layer. Using the thumb screws, the chip was slowly brought into contact with the cell layer until a sufficiently tight seal was obtained. A very slight leakage was allowed to take place to minimise the mechanical stress applied by the PDMS chip to the cells outside of the microchannels while still maintaining a sufficiently tight seal for flow focusing. Leakage was visually confirmed by the presence of very faint fluorescence outside the microchannels. Then, pressures were adjusted for each inlet/outlet to allow the desired treatment to start. Pressure values necessary for flow focusing were in the same range as for the closed-chip experiments, further confirming correct sealing of the chip.
Cell treatment and imaging protocol
The treatment protocols included three phases. During the equilibration phase (10–30 min), the flow was monitored directly by epifluorescence imaging to ensure flow stability. During the treatment phase (lasting from 1 h 30 min to 7 h depending on the experiment), confocal images were acquired automatically to monitor the treatment flow width and position over time. For the washout phase (30–60 min), pressures in inlets 1–3 were reduced and pressure in inlet 4 increased so that the flow from inlets 1–3 disappeared and the doxycycline-free medium could remove residual doxycycline from the channel. The absence of fluorescence in confocal images taken during that time proved the efficiency of the washout phase. To allow post-treatment analysis of the flow and cell staining dynamics, we used a time-lapse protocol in which time-series of XY flow imaging (at the plane of maximal flow fluorescence intensity) alternated with high-resolution Z-stack acquisitions of cell staining by the fluorescent vital dyes (ceramide and Hoechst 33342). Imaging was performed on an inverted microscope IX81 (Olympus Corp., Tokyo, Japan) equipped with the FV1000 scanning confocal system (Olympus) housing 405, 488, 543, and 633 nm lasers. Brightfield and fluorescence confocal live-imaging of ~1600 × 600 μm2 portions of the microfluidic device was achieved through a 10× dry UPlanSApo (0.40 NA, Olympus), acquiring images every 30 s for time-lapse movies at 1024 × 384 pixel2, and at 2048 × 768 pixel2 every 3 μm for Z-stack acquisitions, allowing for individual cell membranes to be resolved. Higher magnification images were acquired through a 40× dry UPlanSApo (0.90 NA, Olympus). Red (543 nm) and far red (633 nm) fluorescent dyes were favored for time-lapse flow monitoring to limit phototoxicity during cell treatments.
Acute doxycycline treatment optimization and analysis
Doxycycline (Sigma-Aldrich) was diluted in sterile distilled water, aliquoted at 1 mg mL−1, and stored frozen, protected from light. Cells seeded in 24-well plates (BD Biosciences) were grown to confluence. 24 h after confluence, wells were exposed to 0, 5, 10, 20, 40, 80 ng mL−1 of doxycycline in culture medium for durations of 1 h, 3 h, 4 h or 24 h. After doxycycline exposure, cells were washed three times for 5 min with doxycycline-free culture medium. 24 h after exposure, cells were washed once with a doxycycline-free medium to remove debris, and MLC-GFP expression was imaged at 3 different positions (each covering ~1.5 × 1.5 mm2), for each condition. MLC-GFP intensity was measured at 6 different positions per image (each covering 200 × 200 μm2). Dose–response curves were plotted from those measurements and fitted with sigmoid functions constrained between 0% and 100% using GraphPad Prism (GraphPad Software, CA, USA), allowing us to determine the minimal concentration of doxycycline at which 50% of the maximal GFP intensity signal is reached at 24 h post-exposure for acute treatments of 1 h 30 min, 3 h 15 min, 4 h 30 min and for a continuous treatment of 24 h.
Determination of effective input and expected output widths
To determine the concentration profile of unlabelled doxycycline (MW = 444 g mol−1) at the ROI during treatment, we imaged TRITC (MW = 443 g mol−1) fluorescence intensity under identical flow conditions, or simultaneously with doxycycline treatment. Averaged TRITC fluorescence intensity values at the ROI were normalized based on fluorescence intensities at the inlet. Knowing that the inlet doxycycline concentration was fixed at 1 μg mL−1, we converted fluorescence intensities to equivalent doxycycline concentrations (see Fig. 3F, ESI S4, S7†), giving us an expected doxycycline concentration profile at the ROI. Threshold doxycycline concentrations were determined from doxycycline treatments performed on Petri-dish-grown monolayers (see previous paragraph). The effective input width was then determined by measuring the distance between the two abscissa coordinates for which the corresponding doxycycline concentration was equal to the threshold dose. The output width was determined as illustrated in Fig. 3 and ESI Fig. S7,† by considering the average width of the monolayer that expresses >50% of the maximal MLC-GFP intensity within the treated region. A Matlab (Mathworks, Natick, MA, USA) routine was used to automatically measure the effective input and output widths at each x coordinate along the ROI. Average cell sizes (ESI Fig. S7D†) were determined by measuring at least 12 rows of 15–20 cells (i.e. >180 cells) per experiment, using the 24 h post-treatment images. Using all those data, we evaluated the correlation between effective input and output widths expressed in microns or in cell diameters using linear regression, as a measure of the predictability of our technique (Fig. 4C and ESI S7E–F†). Those results were plotted using the GraphPad Prism software.
TGFβ1 and HGF treatments
Growth factors solutions were diluted in FBS-free culture medium at 100 ng mL−1, warmed-up to 37 °C and degassed for 30 min before perfusion.
Immunostaining
In-chip staining was performed by carefully perfusing media through the chip output. Cells in-chip or in Petri dishes were rinsed with fresh FBS-free DMEM medium, fixed at room-temperature (RT) for 20 min in PBS
:
DMEM containing 1.75% paraformaldehyde + 1% sucrose + 0.5% Triton-X, followed by a PBS wash and 10 min incubation at 4 °C in PBS–BSA 1%. Primary antibodies or rhodamine–phalloidin were prepared in PBS containing 10 mg ml−1 BSA and applied for >4 h at 4 °C, followed by three 5 min washes with PBS–BSA 1% at 4°C, and 1 h-secondary antibody incubation at RT. Then, a 5 min PBS–BSA 1% with 0.1 μg mL−1 Hoechst 33342 incubation step was followed by three additional PBS–BSA 1% washes before PBS–BSA 1% was substituted with an anti-photobleaching solution (Fluorsave, Calbiochem) for 1 h at 37 °C. Polyclonal rabbit anti-ZO1 (Life technologies) was used at 1
:
50; monoclonal mouse anti-α-tubulin (Life technologies) was used at 1/300; rhodamine–phalloidin (Life technologies) was diluted at 1
:
200. Secondary antibodies were used as follows: Alexa647 goat anti-rabbit 1
:
200, and TexasRed donkey anti-mouse 1
:
50.
Results and discussion
In designing our approach, we were guided by the physiological characteristics of morphogen gradients and practical considerations for in vitro use. In vivo, growth factor and morphogen gradients span distances from one to tens of cell diameters (twenty to hundreds of microns32), persist for tens of minutes to days, and have a precision down to 1 cd.32 Therefore, our approach needed to enable the delivery of a chemical signal to cells with an accuracy better than one cell diameter over durations of several hours. A second constraint was to render the technique compatible with samples that cannot be readily introduced into or grown in sealed microfluidic channels, but have been successfully grown on Petri-dishes, such as ESCs, iPSCs and animal tissue explants.
Computational optimization of microfluidic chip design
Microfluidic devices represent an attractive technological solution because they allow for the creation of chemical gradients across large areas with high accuracy, even enabling the exposure of different parts of the same cell to different chemical environments.33 To date, microfluidic devices have primarily been used with high spatial accuracy for durations <35 min33 or with low spatial accuracy for several hours.34 Positioning accuracy and stability of microfluidic gradients essentially depend on the flow control system and the design of the microfluidic chip. To assess the robustness of gradient positioning, we utilized microfluidic devices that generate three main streams, and observed stream boundaries over time under test conditions. The simplest device consists of three inlets converging into one channel (ESI Fig. S1A†), in which streams flow side-by-side without intermixing due to the short length-scales and low flow-rates involved. Diffusion of solutes can however occur at the interfaces between streams, a phenomenon known as lateral diffusion. In our experiments, we perfused fluorescent solutions through the central stream and imaged their position in the region of interest (ROI) over durations up to 8 h by confocal fluorescence microscopy. After testing several flow-control systems and rejecting those generating too large oscillations in the position of the stream (ESI Fig. S1B†), we settled for a closed-loop pressure-controlled device that independently pressurizes each inlet and outlet (Fig. 1A, ESI S1C†). Nevertheless, experimentally, flow disturbances occasionally occurred due to debris, air bubbles, and pressure oscillations. Therefore, we sought to optimize the chip design using computational fluid dynamics modeling of diffusive chemical species within the channel. For each design (Fig. 1B), we evaluated gradient steepness (Fig. 1C) and robustness versus local blockage and pressure changes in one or all inlets (Fig. 1D, E, F). To compare the performance of all designs, we plotted the maximum change in the width of the treated area (“treatment width”, i.e. the area exposed to a concentration >10% of the maximum concentration) in response to perturbations that diverted the treatment flow (Fig. 1G–H), and made it transiently span a larger area than intended. Overall, treatment width variations in response to experimental perturbations were minimized in designs in which the sheath flow was split into many parallel microflows (Fig. 1H, ESI Fig. S2†) and in which the treatment flow widened shortly prior to convergence with the sheath flow (Fig. 1D–F). Hence, in our simulations, the chip with the optimal design (Fig. 1G–H) reduced maximum treatment width variations to less than 20% upon any experimental perturbation (Fig. 1D–F). Four hour long experimental perfusion of a soluble dye through this chip confirmed that transient maximum variations in the treatment width did not exceed 20% (Fig. 1I), leading to an average treatment width increase of less than 3% over the total duration of the experiment.
Optimized devices allow stable, accurate, long term delivery of chemical gradients to cells
Having optimized chip design, we experimentally tested the ability of our setup to accurately deliver chemicals through the apex of epithelial cells grown to confluence in-chip (Madine-Darby Canine Kidney cells, MDCK, ESI Fig. S1D†).
We used two cell-permeant vital dyes simultaneously: the blue-fluorescent DNA intercalant Hoechst 33342 and a far-red fluorescent ceramide probe, for live imaging of cell nuclei and membranes, respectively. During experiments, we continuously imaged the treatment flow by confocal microscopy using the fluorescence from the ceramide dye (imaged ROI shown in Fig. 1G). In a representative experiment, following 4 h 30 min exposure to a 23 μm-wide treatment stream (or 1.1 cd wide, Fig. 2A, ESI S3A–C†) and subsequent washout of the unbound vital dyes, ceramide stained a 57 μm-wide (or 2.5 cd wide) strip, a width larger than the treatment flow due to marker uptake by partially exposed cells (Fig. 2A5, B) but significantly narrower than the DNA labeling, which extended up to 92 μm (Fig. 2A6, B). This difference in the treatment width between two dyes of a similar molecular weight (Hoechst: 615 g mol−1, Ceramide: 706 g mol−1) resulted from the combination of two phenomena: 1) the greater lateral diffusion of Hoechst 33342 across stream boundaries, and 2) the diffusion across cell walls within the cell monolayer, as the Hoechst stain kept spreading during the washout (ESI Fig. S3C†). Observation of the cells at 24 h post-treatment further confirmed the good retention of ceramide in treated cells and the spreading of the Hoechst stain through the whole monolayer (Fig. 2C, D). It highlighted the importance of carefully selecting dyes for flow and cell-tracking purposes in our setup. To select the best dye for flow position tracking, we next observed lateral diffusion along the chip for various fluorescent markers with molecular weights ranging from 0.3 to 2000 kDa (ESI Fig. S4A–C†). A good agreement was found between the molecular weight and the treatment flow width, with the exception of ceramide, whose hydrophobicity likely accounted for its very low lateral diffusion, making it an excellent reporter for the treatment flow position (ESI Fig. S4C†). Therefore, in subsequent experiments, we utilized fluorescent ceramide to follow the position of the treatment flow over time and to highlight treated cells for hours post-treatment.
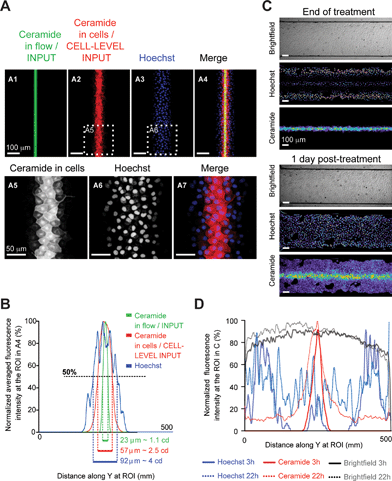 |
| Fig. 2 Visualization of the treatment width using fluorescent dyes. (A) Correspondence between the input flow width and the cell labeling width. The effectively treated region (revealed by the fluorescence of ceramide in cells, A2) is wider than the width of the treatment flow (visualized by the fluorescence of ceramide in flow, A1), due to ceramide diffusion within the membranes of cells partially exposed to the treatment (A5). The cell permeant dye Hoechst 33342 displays intercellular diffusion outside the treated region (A3, A6). (B) Line scan across A4 showing the width of the treatment flow (green), the width of monolayer labeling with ceramide (red), and the width of nuclear labeling (blue). (C) Comparison of the diffusion of ceramide and Hoechst within the cell monolayer up to 22 h after treatment. The ceramide stain was well retained by treated cells while the Hoechst stain spreads to the whole monolayer. (D) Line scans across C immediately after cessation of treatment and 22 h after. | |
Conditional gene expression can be predictably and locally induced within a sealed chip
Next, we evaluated the ability of our platform to apically deliver a chemical gradient to predictably induce gene expression in a target region of a cultured epithelium. In vivo, morphogen-induced gene expression changes are not a simple readout of the input morphogenetic gradient, but result from the integration of the gradient by complex transduction pathways over time (ESI Fig. S5A–B†). Hence, to determine the relative contributions of the gradient dynamic shape (“input signal”) and the intracellular transduction pathway (“transfer function”) to the target cell response (“output”), one has to be able to separate both components. As we saw earlier (Fig. 2), for any biochemical treatment, in-flow treatment (“input”) differs from the effective treatment (“cell-level input”), which depends on the treatment chemical binding and diffusion within the cell layer. To test our chemical gradient delivery method's reliability, we needed to be able to predict the effective treatment width from in-flow treatment imaging.
We therefore needed an input signal that translates into a cellular output through a simple transfer function. To answer these needs, we used the Tet-On system, where addition of the cell-permeant doxycycline above a concentration/time threshold activates a genetically encoded transactivator that specifically induces the transcription of transgenes driven by tet-responsive promoters (ESI Fig. S5C†).25 Hence, the Tet-On mechanism functions as a “simplified morphogen signaling pathway” without any intracellular transduction or feedback steps (ESI Fig. S5D†). We thus generated a Tet-On MDCK cell line that expresses myosin light chain (MLC) fused to a green-fluorescent protein (GFP) upon doxycycline treatment, and grew it to confluence in-chip. A 3 h 15 min-long local perfusion of ceramide and doxycycline yielded a narrow band of MLC-GFP expressing cells that persisted for over 48 h (Fig. 3A–E, ESI S3D–F†), proving that local induction of gene expression can be achieved with our setup.
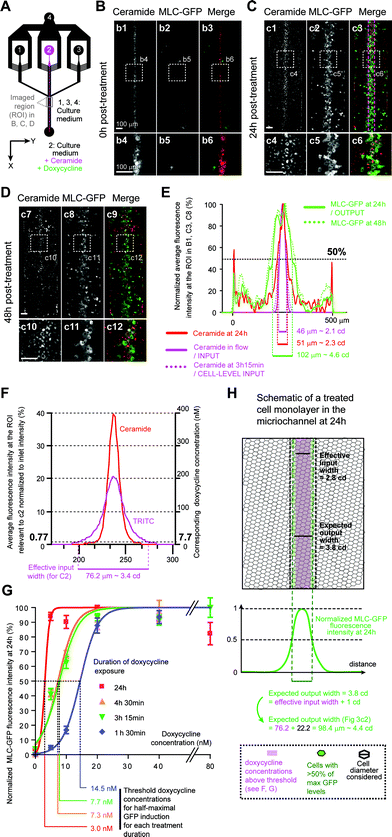 |
| Fig. 3 Microfluidic delivery of chemical inducer gradients allows spatiotemporal control of gene expression in sealed chips. (A) Chip setup and regions of interest (ROI). (B–C–D) Extent of MLC–GFP and ceramide fluorescence, immediately after (B), 24 h after (C) and 48 h after (D) acute local doxycycline treatment, in a sealed chip. (E) Comparison between input and output normalized fluorescence intensity profiles at the ROI at 3 h, 24 h and 48 h post treatment. (F–I) Determination of the effective input width and the expected output width. (F) The TRITC fluorescence gradient across the channel averaged over the duration of the treatment used to estimate the doxycycline gradient. (G) Doxycycline/GFP dose–response curves used to determine the threshold doxycycline concentration for half maximal MLC–GFP fluorescence 24 h post-treatment for each treatment duration. (H) Prediction of the output width (MLC–GFP expression) from the effective input width (inferred from the TRITC fluorescence gradient). | |
We then determined if the width of the band of cells expressing GFP above half-maximal levels (output), could be predicted from the width of the treatment flow (input). GFP induction extended beyond the region exposed to ceramide (Fig. 3E), as expected given that doxycycline is a 444 g mol−1 hydrosoluble chemical and the amphiphilic 706 g mol−1 ceramide probe was shown to display the least lateral diffusion (ESI Fig. S4C†). We thus experimentally estimated the concentration profile of doxycycline from the concentration profile of tetramethyl-rhodamine iso-thiocyanate (TRITC, MW = 443 g mol−1, ESI Fig. S4†) in the ROI under perfusion conditions identical to our experiment and normalized fluorescence to that at the inlet (Fig. 3F, ESI S4D–G†). We next determined, under our experimental conditions, the doxycycline concentration above which GFP-induction is half-maximal (threshold concentration). As both duration and dose of exposure influence induction thresholds, we exposed confluent monolayers to several doxycycline concentrations for various durations yielding dose–response curves (Fig. 3G, ESI S6†). Using these curves, we determined that for a 3 h 15 min treatment the threshold concentration was 7.7 nM. Using this value and the estimated doxycycline concentration profile at the ROI, we estimated the effective input width to be 76.2 μm (Fig. 3F). Taking into consideration cells partially exposed to above threshold doxycycline concentrations (accounting, on average, for an additional 0.5 cd on each side of the treated region with 1 cd = 22.2 μm, Fig. 3H), we then predicted an output width of 98.4 μm. Imaging of the ROI at 24 h (Fig. 3C) and 48 h (Fig. 3D) post-treatment revealed the steady induction of MLC-GFP expression in a 102 μm-wide band (Fig. 3E), comparable to our estimated output width. This result showed that the output width can be predicted from the input width by using a fluorescent probe with similar hydrodynamic properties to the chemical inducer.
Reversible chip clamping increases versatility without affecting treatment accuracy
However, many cells are not readily grown in microfluidic chips because of their growth requirements in terms of cell density, medium homeostasis and substrate properties. Moreover, hard coated glass surfaces are not natural substrates for cell cultures, inducing the formation of long ventral actin stress fibers, structures rarely present in vivo.35 Biological 3D-gels are more suitable substrates for morphogenetic studies, because they recapitulate the chemical and mechanical properties of natural substrates,36 but they are difficult to set in a chip. To circumvent the limitations of our setup, we therefore adapted a previously described clamping device31 to allow enclosure into microfluidic channels of animal tissue explants or cells cultured on their favorite substrate in glass-bottom Petri dishes (Fig. 4A).
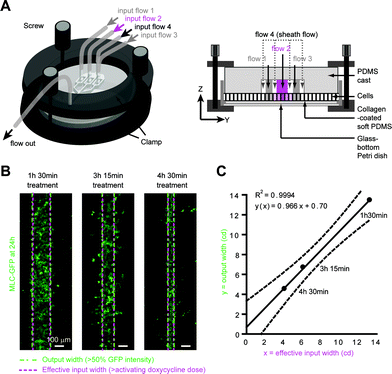 |
| Fig. 4 Reusable clamped chips allow versatile control of gene expression. (A) Schematic illustration of the clamped chip for localized treatment of cells grown on Petri dishes. (B) Localized MLC–GFP expression after 24 h under three treatment conditions in clamped devices. Pink and green dotted lines delineate effective input and output widths, respectively, inferred from the in-flow TRITC imaging and MLC–GFP fluorescence intensity. (C) Correlation between effective input and output widths expressed in cell diameters (cd). Dotted lines denote the 95% confidence interval. | |
To maintain a tightly sealed chip without breaking the glass coverslip, we added a transparent PDMS cushion layer below the cell layer. To “mask” the PDMS surface to the cells, we used a protocol that improves substrate surface coating.30 We thus grew MDCK cells on thin collagen 3D gels set onto the PDMS cushion (Fig. 4A). The combination of the soft PDMS cushion and the collagen gel also prevented cells outside of the microchannels from being crushed during clamping, thereby avoiding release of apoptotic or necrotic factors. Under those conditions, cells were well polarized, as shown by E-cadherin–GFP and ZO-1 apical junction staining. Using this setup on MLC–GFP expressing cells under a range of treatment conditions, we showed that the output width correlated linearly with the effective input width (Fig. 4B, C, ESI S7†). Knowing the input width, we could predict the width of gene expression with a <1 cd accuracy (Fig. 4C). Together, these data indicated that reversible clamping maintains patterning precision while allowing users to retain established culture protocols and post hoc characterization methods.
Morphogenetic changes can be locally triggered by targeted growth factor treatments
Having established a method to induce spatially restricted gene expression through the controlled delivery of chemical gradients to confluent cell cultures, we sought to locally induce an endogenous genetic program leading to morphogenetic changes by exposure to growth factor gradients.
One widely studied morphogenetic process in vitro is Epithelial to Mesenchymal Transition (EMT), which occurs during development, wound healing, and epithelial cancers.37,38 EMT is triggered by growth factors that modify the cytoskeleton and polarity gene expression dynamics, resulting in cell polarity, cell shape and cell motility changes which are revealed by the mislocalisation of apical junction markers, loss of primary cilia, and cell emigration outside the epithelial layer. Depending on the cell type and conditions of induction, EMT leads to an array of phenotypes ranging from rounded “somewhat-polarized” cells to flattened highly motile mesenchymal-like cells.37–40 In MDCK cells, long-term exposure (>24 h) to TGFβ1 leads to loss of epithelial polarity and cell spreading,41 while exposure to HGF leads to tubulogenesis which maintains some cell polarity.42,43
First, we verified that such phenotypes were observed in confluent polarized MDCK cells cultures grown on 3D collagen-coated Petri dishes. A 24 h-exposure to 10 ng mL−1 TGFβ1 led to cell scattering and crawling on the monolayer, while a 24 h-exposure to 10 ng mL−1 HGF resulted in globular protuberances characteristic of the tubulogenesis onset (ESI Fig. S8A†). Though in vitro EMT has traditionally been studied using global stimuli on subconfluent cell monolayers, in vivo, growth factor gradients ensure that only a subset of cells undergoes EMT within a fully confluent polarized epithelium. We therefore aimed to induce localized EMT events within a mature epithelium by acutely applying growth factor gradients generated with our setup. To ensure local maximal concentrations above induction threshold at the ROI under transient treatment conditions (<8 h), input growth factor concentrations were fixed at 100 ng mL−1 (i.e. 10 times the induction threshold for a 24 h exposure). Upon localized HGF treatment, MDCK monolayers cultured on glass (Fig. 5A–B) or on collagen gels (Fig. 5C–G), formed protuberances in the treated area only (Fig. 5B, E–G, ESI S9†). E-cadherin–GFP fluorescence levels significantly and specifically increased in HGF treated cells within 18 h (Fig. 5C–D, ESI S9†), leading to an accumulation of cytoplasmic E-cadherin–GFP without detectable loss of junctional E-cadherin–GFP. In protuberances formed on ciliated confluent monolayers, apical ZO1 staining was often maintained (Fig. 5F–G), whereas primary cilia were locally missing, as revealed by the lack of acetylated-α-tubulin antibody staining (Fig. 5G). Hence, apical polarity was partially maintained in HGF treated cells, consistent with previous studies.42,43 TGFβ1 treatment led to cell scattering on glass substrates, with treated cells leaving the monolayer or the treated area (Fig. 5H), eventually forming small protuberances (Fig. 5I). On ciliated confluent monolayers grown on collagen gels, TGFβ1 treatment led to the formation of protuberances similar to those induced by HGF, but with a clearly deficient ZO1 staining and fully devoid of primary cilia44 (Fig. 5J), revealing a more severe loss of apical polarity similar to what is observed in pancreatic tumors in situ.45 TGFβ1 also led to a clear relocalization of junctional E-cadherin–GFP to the cytoplasm (ESI Fig. S10†), as previously reported.46,47 Hence, local acute treatment of MDCK cells with either HGF or TGFβ1 recapitulated each type of EMT in the target area only. These results validate our technique for studying local morphogenetic changes induced by spatiotemporally controlled growth factor gradients.
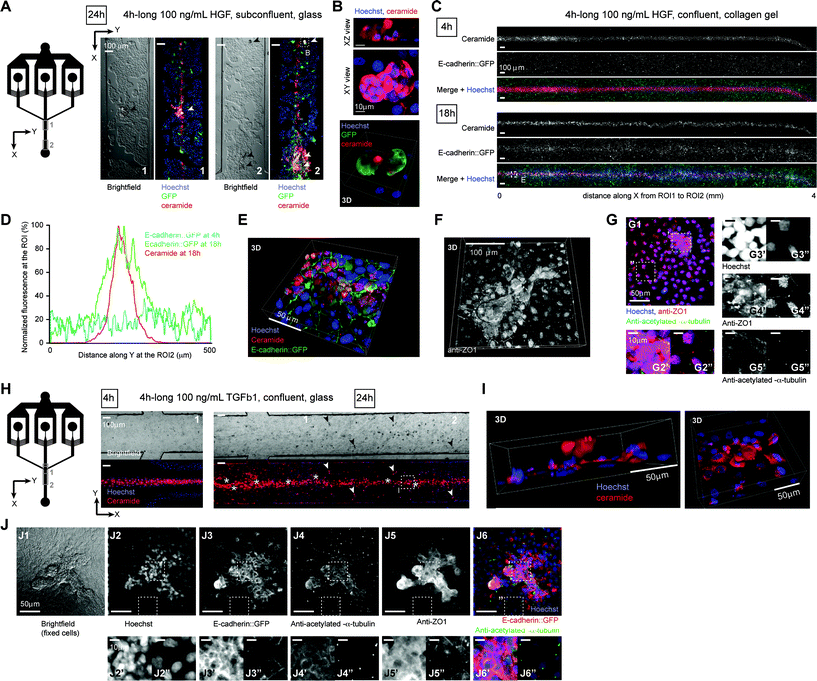 |
| Fig. 5 Local morphogenetic changes are induced by localized exposure to growth factor gradients. (A) Schematic illustration of the chip and live-imaging at 24 h of a subconfluent monolayer grown on glass in a sealed-chip and locally exposed for 4 h to a 100 ng mL−1 HGF treatment. Arrowheads indicate atypical protuberances only observed in the treated area stained with ceramide. (B) Maximum projections and 3D-rendering of a protruding group of treated cells. (C) Live-imaging at 4 h and 18 h of a confluent monolayer grown on a collagen gel in a clamped-chip and locally exposed for 4 h to a 100 ng mL−1 HGF treatment. Cytoplasmic E-cadherin–GFP levels are increased in HGF-treated cells 18 h post-treatment. (D) Line scan across C at 4 h and 18 h post treatment. (E) Treated cells form a protrusion at 2 days post-treatment in the region imaged in C–D. E-cadherin–GFP remained at the apical intercellular junctions. (F) 3D-rendering of a multicellular protrusion induced by HGF exposure stained for ZO-1 (24 h after treatment). ZO-1 remained localized to apical intercellular junctions. (G) Maximal projection of a protrusion induced by exposure to HGF (24 h after treatment of a confluent ciliated MDCK monolayer). Anti-acetylated α-tubulin staining reveals a lack of primary cilia on the protruding cells. Panels G2′ to G5′, and G2′′ to G5′′ are magnifications of the boxed areas, respectively, marked with ′ and ′′ in G1. (H) Schematic illustration of the chip and live-imaging at 4 h and 24 h of a subconfluent monolayer grown on glass in a sealed-chip and locally exposed for 4 h to a 100 ng mL−1 TGFβ1 treatment. Arrowheads indicate cells and group of cells strongly labeled with ceramide that have emigrated from the treated area. Asterisks identify areas in which treated cells detached, leaving gaps in the monolayer. (I) 3D-rendering of a portion of the treated area where cells have protruded above the monolayer. (J) Protrusion induced by exposure to TGFβ1 24 h after treatment of a confluent ciliated MDCK monolayer. The protruding cells lost their primary cilia as revealed by anti-acetylated-α-tubulin antibody and ZO1 staining, while retaining some E-cadherin–GFP at apical intercellular junctions. Panels J2′ to J6′, and J2′′ to J6′′ are magnifications of boxed areas ′ and ′′ in J2–6, respectively. | |
Conclusions
By combining long-term hydrodynamic flow-focusing and conditional gene-expression in a clamped microfluidic chip, we predictably induced gene-expression in strips of cells down to ~4 cd-wide with high precision (<1 cd) within epithelia cultured in Petri-dishes. This approach enables spatial control of gene expression with a one-cell diameter accuracy in virtually any existing chemically inducible adherent cell line. The successful local induction of EMT by HGF and TGFβ1 treatments further demonstrated that the delivery of spatiotemporally defined growth factor gradients to cultured tissues can be used to recapitulate in vitro natural morphogenetic patterns relevant to developmental organogenesis and cancer. The reversible assembly of the microfluidic chip confers several key advantages. First, it makes this patterning technique compatible with any adherent tissue that can be grown in a Petri dish, and does not require any further optimization of culture conditions, allowing application to primary cultures, iPS and ES cells, or animal explants. Second, it can be combined with substrate micropatterning and optogenetics to achieve very complex patterning. Third, it allows pre- and post-patterning treatments such as substrate engineering, successive localized treatments, long-term imaging, tissue differentiation, immunostaining, “omics” analysis of patterned tissue sections, mechanical characterization by atomic-force microscopy, etc. Finally, the stability of the treatment flow over several hours enables quantitative approaches that seek to relate a complex spatiotemporal treatment with a tissue response, a key step in the understanding of intracellular transduction pathways. This is particularly relevant to the modelling and recapitulation of morphogenetic signalling, necessary to attain a systems understanding of cell fate determination mechanisms during developmental organogenesis or engineering of tissues for regenerative medicine.
Acknowledgements
We thank Simon Townsend and Duncan Farquharson at the UCL Biosciences Mechanical Workshop for the fabrication of the clamp, Drs Andrew Harris, Catherine Hogan and Kerry Wilson for their technical advice, and Drs Miia Bovellan, Marco Fritzsche and Catherine Au for their comments and proof-reading of the manuscript. The authors wish to acknowledge the UCL Comprehensive Biomedical Research Centre for the generous funding of microscopy equipment. This work was supported by the BBSRC (BB/F019769/1) and a Royal Society University Fellowship to GC. YF is supported by Next Generation World-Leading Researchers (NEXT Program), the Takeda Science Foundation, the Uehara Memorial Foundation, Daiichi-Sankyo Foundation of Life Science, and Naito Foundation.
Notes and references
- P. Krupinski, V. Chickarmane and C. Peterson, Curr. Opin. Genet. Dev., 2012, 22, 613–618 CrossRef CAS PubMed.
- L. G. Morelli, K. Uriu, S. Ares and A. C. Oates, Science, 2012, 336, 187–191 CrossRef CAS PubMed.
- A. Kicheva, M. Cohen and J. Briscoe, Science, 2012, 338, 210–212 CrossRef CAS PubMed.
- Z. Kibar, V. Capra and P. Gros, Clin. Genet., 2007, 71, 295–310 CrossRef CAS PubMed.
- S. L. Haigo, J. D. Hildebrand, R. M. Harland and J. B. Wallingford, Curr. Biol., 2003, 13, 2125–2137 CrossRef CAS PubMed.
- L. A. Taneyhill, Cell Adhes. Migr., 2008, 2, 223–230 CrossRef.
- O. Pourquie, Cell, 2011, 145, 650–663 CrossRef CAS PubMed.
- V. Ribes and J. Briscoe, Cold Spring Harbor Perspect. Biol., 2009, 1, a002014 Search PubMed.
- G. R. van den Brink and G. J. Offerhaus, Cancer Cell, 2007, 11, 109–117 CrossRef CAS PubMed.
- J. D. Potter, Nat. Rev. Cancer, 2007, 7, 464–474 CrossRef CAS PubMed.
- J. P. Brockes and A. Kumar, Annu. Rev. Cell Dev. Biol., 2008, 24, 525–549 CrossRef CAS PubMed.
- K. W. Rogers and A. F. Schier, Annu. Rev. Cell Dev. Biol., 2011, 27, 377–407 CrossRef CAS PubMed.
- S. Eaton, Curr. Opin. Genet. Dev., 2006, 16, 17–22 CrossRef CAS PubMed.
- D. Yan and X. Lin, Cold Spring Harbor Perspect. Biol., 2009, 1, a002493 Search PubMed.
- G. T. Reeves, C. B. Muratov, T. Schupbach and S. Y. Shvartsman, Dev. Cell, 2006, 11, 289–300 CrossRef CAS PubMed.
- M. J. Kennedy, R. M. Hughes, L. A. Peteya, J. W. Schwartz, M. D. Ehlers and C. L. Tucker, Nat. Methods, 2010, 7, 973–975 CrossRef CAS PubMed.
- M. Thery, J. Cell Sci., 2010, 123, 4201–4213 CrossRef CAS PubMed.
- X. Wang, X. Chen and Y. Yang, Nat. Methods, 2012, 9, 266–269 CrossRef CAS PubMed.
- D. M. Cate, C. G. Sip and A. Folch, Biomicrofluidics, 2010, 4, 44105 CrossRef CAS PubMed.
- D. Irimia, D. A. Geba and M. Toner, Anal. Chem., 2006, 78, 3472–3477 CrossRef CAS PubMed.
- T. M. Keenan, C. H. Hsu and A. Folch, Appl. Phys. Lett., 2006, 89, 114103 CrossRef PubMed.
- M. A. Qasaimeh, T. Gervais and D. Juncker, Nat. Commun., 2011, 2, 464 CrossRef PubMed.
- Y. Zhou, Y. Wang, T. Mukherjee and Q. Lin, Lab Chip, 2009, 9, 1439–1448 RSC.
- C. J. Tomlin and J. D. Axelrod, Nat. Rev. Genet., 2007, 8, 331–340 CrossRef CAS PubMed.
- S. Urlinger, U. Baron, M. Thellmann, M. T. Hasan, H. Bujard and W. Hillen, Proc. Natl. Acad. Sci. U. S. A., 2000, 97, 7963–7968 CrossRef CAS PubMed.
- C. Hogan, S. Dupre-Crochet, M. Norman, M. Kajita, C. Zimmermann, A. E. Pelling, E. Piddini, L. A. Baena-Lopez, J. P. Vincent, Y. Itoh, H. Hosoya, F. Pichaud and Y. Fujita, Nat. Cell Biol., 2009, 11, 460–467 CrossRef CAS PubMed.
- W. Chan, G. Calderon, A. L. Swift, J. Moseley, S. Li, H. Hosoya, I. M. Arias and D. F. Ortiz, J. Biol. Chem., 2005, 280, 23741–23747 CrossRef CAS PubMed.
- D. S. Ory, B. A. Neugeboren and R. C. Mulligan, Proc. Natl. Acad. Sci. U. S. A., 1996, 93, 11400–11406 CrossRef CAS.
- C. D. Garcia and C. S. Henry, Methods Mol. Biol., 2006, 339, 27–36 CAS.
- P. J. Wipff, H. Majd, C. Acharya, L. Buscemi, J. J. Meister and B. Hinz, Biomaterials, 2009, 30, 1781–1789 CrossRef CAS PubMed.
- E. Tkachenko, E. Gutierrez, M. H. Ginsberg and A. Groisman, Lab Chip, 2009, 9, 1085–1095 RSC.
- J. B. Gurdon and P. Y. Bourillot, Nature, 2001, 413, 797–803 CrossRef CAS PubMed.
- S. Takayama, E. Ostuni, P. LeDuc, K. Naruse, D. E. Ingber and G. M. Whitesides, Chem. Biol., 2003, 10, 123–130 CrossRef CAS.
- E. M. Lucchetta, J. H. Lee, L. A. Fu, N. H. Patel and R. F. Ismagilov, Nature, 2005, 434, 1134–1138 CrossRef CAS PubMed.
- T. Kobayashi and M. Sokabe, Curr. Opin. Cell Biol., 2010, 22, 669–676 CrossRef CAS PubMed.
- F. Brandl, F. Sommer and A. Goepferich, Biomaterials, 2007, 28, 134–146 CrossRef CAS PubMed.
- M. A. Nieto, Annu. Rev. Cell Dev. Biol., 2011, 27, 347–376 CrossRef CAS PubMed.
- K. Polyak and R. A. Weinberg, Nat. Rev. Cancer, 2009, 9, 265–273 CrossRef CAS PubMed.
- T. Geiger, H. Sabanay, N. Kravchenko-Balasha, B. Geiger and A. Levitzki, PLoS One, 2008, 3, e1574 Search PubMed.
- M. A. Futterman, A. J. Garcia and E. A. Zamir, Dev. Dyn., 2011, 240, 1502–1511 CrossRef CAS PubMed.
- J. Xu, S. Lamouille and R. Derynck, Cell Res., 2009, 19, 156–172 CrossRef CAS PubMed.
- D. F. Balkovetz, A. L. Pollack and K. E. Mostov, J. Biol. Chem., 1997, 272, 3471–3477 CrossRef CAS PubMed.
- A. L. Pollack, G. Apodaca and K. E. Mostov, Am. J. Physiol., 2004, 286, C482–494 CrossRef CAS PubMed.
- I. R. Veland, A. Awan, L. B. Pedersen, B. K. Yoder and S. T. Christensen, Nephron Physiol., 2009, 111, 39–53 CrossRef PubMed.
- E. S. Seeley and M. V. Nachury, Methods Cell Biol., 2009, 94, 299–313 CAS.
- M. Saitoh, T. Shirakihara and K. Miyazono, Biochem. Biophys. Res. Commun., 2009, 381, 560–565 CrossRef CAS PubMed.
- E. Janda, M. Nevolo, K. Lehmann, J. Downward, H. Beug and M. Grieco, Oncogene, 2006, 25, 7117–7130 CrossRef CAS PubMed.
Footnotes |
† Electronic supplementary information (ESI) available: Supplementary figures and supplementary methods accompany this submission. See DOI: 10.1039/c3lc51281a |
‡ Current address: Institute of Healthy Ageing, University College London, London, UK. |
|
This journal is © The Royal Society of Chemistry 2014 |
Click here to see how this site uses Cookies. View our privacy policy here.