Modelling phosphorus loading and algal blooms in a Nordic agricultural catchment-lake system under changing land-use and climate
Received
22nd November 2013
, Accepted 5th February 2014
First published on 5th February 2014
Abstract
A model network comprising climate models, a hydrological model, a catchment-scale model for phosphorus biogeochemistry, and a lake thermodynamics and plankton dynamics model was used to simulate phosphorus loadings, total phosphorus and chlorophyll concentrations in Lake Vansjø, Southern Norway. The model network was automatically calibrated against time series of hydrological, chemical and biological observations in the inflowing river and in the lake itself using a Markov Chain Monte-Carlo (MCMC) algorithm. Climate projections from three global climate models (GCM: HadRM3, ECHAM5r3 and BCM) were used. The GCM model HadRM3 predicted the highest increase in temperature and precipitation and yielded the highest increase in total phosphorus and chlorophyll concentrations in the lake basin over the scenario period of 2031–2060. Despite the significant impact of climate change on these aspects of water quality, it is minimal when compared to the much larger effect of changes in land-use. The results suggest that implementing realistic abatement measures will remain a viable approach to improving water quality in the context of climate change.
Environmental impact
Computer-based environmental modelling offers an essential aid to understand current catchment dynamics and to investigate the potential effectiveness of remedial actions aimed at improving water quality. Here, we present a novel network of process-based, mass-balance models linking climate, hydrology, catchment-scale P dynamics and lake processes. This study exemplifies how an objectively calibrated model network allows disentangling the effects of climate change from those of land-use change on lake water quality and phytoplankton growth. The model network can thus support decision-making to achieve good water quality and ecological status.
|
1. Introduction
The use of the nutrient phosphorus (P), an essential fertilizer element enhancing plant growth, has underpinned global agriculture and food production since the beginning of the 20th century. Global P-based food production, which has doubled over the past 45 years,1 has been hypothesized to be responsible for the estimated three-fold increase in the river borne flux of P to the oceans since pre-industrial times (e.g., Haygarth2). When P is delivered to water bodies, negative influences on water quality are likely, and the eutrophication of freshwater and coastal marine ecosystems resulting from increased anthropogenic P loadings is a global problem.3 In lake basins specifically, excess nutrients from both point and nonpoint sources throughout the catchment can give rise to harmful algal blooms, degrade water quality, and create extensive oxygen depletion.
The discharge of P to surface water is subject to comprehensive regulations worldwide, such as the Clean Water Act (CWA) in the USA, Water Pollution Prevention and Control (WPPC) Law in China and the Water Framework Directive (WFD) in the European Union. In Europe, the WFD 2000/60/EC has been designed to achieve good biological and chemical status for water bodies by 2015,4 promoting an approach to water and land management through river basin planning explicitly aimed at reducing the impacts of eutrophication caused by excess nutrient inputs.
Climatic conditions − in addition to land use, agricultural practices, urban and sewage nutrient inputs − are key drivers of eutrophication in lakes.5–8 For instance, in a given catchment, air temperature, precipitation, and the morphometry of a lake will determine the extent to which wind-mixing will influence the vertical transfer of P and influence the effect of light on P uptake by phytoplanktons. In the context of climate change, it is becoming increasingly difficult to disentangle the complex climatic effects influencing water quality from the effects of specific measures implemented to improve it.9 A better understanding of the response of specific catchments to both climate and land-use change is needed for a scientifically guided management design to mitigate the impact of these changes on water quality.
Computer-based environmental modelling offers an aid to understanding current catchment dynamics and investigating the potential effectiveness of remedial actions in the context of climate change. Building on previous catchment modelling efforts aiming at predicting P delivery to lakes in agricultural catchments,10–13 we constructed a novel network of chained model to integrate climate, hydrologic, catchment, and in-lake processes. At the top of the model chain is a global climate model (GCM) whose output for daily temperature, precipitation and other variables was downscaled to the region. These are used as inputs to a hydrologic rainfall-runoff model (PERSiST14) to produce daily discharge values for rivers, which, in turn, are used as inputs for INCA-P15 to simulate daily fluxes of suspended sediments and P to the lake. At the end of the model chain is the lake model MyLake.16 Here, we take advantage of these models' matching state variables, spatial scales and temporal resolutions,17 couple them into a network consisting of river stretches and lake basins, and perform automated calibration and uncertainty analysis across the network. The seamless connection between model components allows for the propagation of changes in boundary conditions – such as climatic or land-use changes – within the model network (e.g., Voinov18). The model network is applied to the Vansjø–Hobøl catchment (Norway), whose water quality, nutrient loading,19 as well as past and recent land-uses have been thoroughly documented due to the basin's pivotal importance for water supply and its sensitivity to eutrophication in lake Vansjø.20
The main anthropogenic pressure on the Vansjø–Hobøl catchment is a surplus of P, which has resulted in eutrophication and severe blooms of cyanobacteria, including the potentially toxic Microcystis.7,19–23 Although it is generally recognized that the abundance of the essential nutrients nitrogen (N) and silicon (Si) is also a key factor controlling algal growth and thus water quality,24,25 our work has focussed on P based on evidence that phytoplankton growth in this system is P-limited.19 As agricultural practices continue to expand in the basin, and with the observed increase in temperature and precipitation in northern Europe,26 the occurrence of algal blooms is expected to increase. We thus aimed to model the response of biological (i.e., chlorophyll) and chemical (i.e., phosphorus) indicators of water quality, as defined by the WFD, to climate and land-use changes in the Vansjø–Hobøl catchment and to assess the influence of climate change on the feasibility of achieving existing water quality targets.
2. Material and methods
2.1. Site description
The Vansjø–Hobøl catchment (area = 690 km2), also referred to as the Morsa catchment, is located in south-eastern Norway (59°24′N 10°42′E). The Hobøl River, with a mean discharge of 4.5 m3 s−1, drains a sub-catchment of 301 km2 into Lake Vansjø, the catchment's main lake. Lake Vansjø has a surface area of 36 km2 and consists of several sub-basins, the two largest being Storefjorden (eastern basin, L1 in Fig. 1) and Vanemfjorden (western basin, L2 in Fig. 1), whose characteristics are described in Table 1. The water-column of both basins remains oxygenated throughout the year. In addition, there are six smaller lakes which together represent less than 15% of the lake surface area. The Storefjorden basin drains into the Vanemfjorden basin through a shallow channel. The outlet of Vanemfjorden discharges into the Oslo Fjord (Fig. 1).
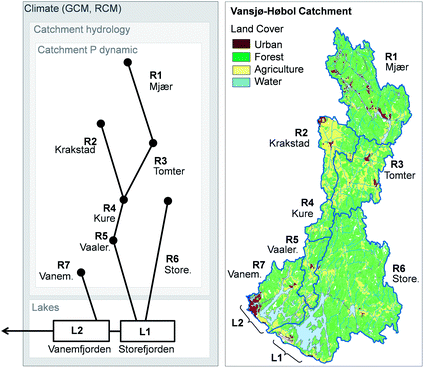 |
| Fig. 1 Land-use distribution of the Vansjø–Hobøl catchment (right panel) and the corresponding schematic representation of the catchment-lake model network (left panel) indicating river reaches (R) modelled with INCA-P and lake basins (L) modelled with MyLake. The hydrological model PERSiST provides input for the catchment model, and the climate models provide forcing for all models. | |
Table 1 Location and characteristics of the lake basins
Basin name |
Storefjorden |
Vanemfjorden |
Location (latitude and longitude) |
59°23′24′′ N, 10°49′52′′E |
59°24′53′′ N, 10°42′46′′ E |
Mean depth (m) |
8.7 |
3.8 |
Maximum depth (m) |
41.0 |
19.0 |
Area (km2) |
23.8 |
12 |
Volume (m3) |
206.1 × 106 |
46.1 × 106 |
Residence time (years) |
0.85 |
0.21 |
2.2. The model network
The model network consists of four separate models: a climate model, a hydrological model, a catchment model for P, and a lake model. The model network is first calibrated to present-day observed data, then run with four storylines to simulate conditions in the future. The model network is shown in Fig. 1 and described in detail below.
Climate models.
For a given greenhouse gas emission scenario (see Section 2.4), projections of future climate change differ depending on the GCM used.27 Consequently, we tested the following three GCMs independently as inputs: (1) HadCM3,28 (2) ECHAM5,29 and (3) Bergen Climate Model (BCM).30,31 The outputs from the GCMs were the basis for RCMs, yielding dynamically downscaled daily weather projections. Details on the GCM-RCM pairs are given in Table 2. This approach has been shown to be an effective way to couple climate with hydrology.32
Table 2 Change in the yearly mean temperature (ΔT) and precipitation (Δp) predicted by climate models for the Vansjø–Hobøl catchment during the scenario period 2030–2052 relative to the reference period 1990–2012
Scenario |
GCM |
RCM |
ΔT (°C) |
Δp (mm) |
Configuration |
Hadley Centre, UK.
Max Planck Institute for Meteorology, Germany.
Nansen Centre, Norway.
|
C1 |
HadRm3a |
HADRM3 |
+1.6 |
+78.8 |
Q0 with normal sensitivity |
C2 |
ECHAM5b |
RACMO |
+0.7 |
+43.4 |
−r3 set of initial conditions |
C3 |
BCMc |
RCA |
+0.9 |
−10.5 |
|
Catchment models.
The outputs of the RCMs, together with basin characteristics, were used as inputs for the hydrological PERSiST model to produce daily estimates of runoff, hydrologically effective rainfall and soil moisture deficit. Previously, external time series of runoff, hydrologically effective rainfall and soil moisture deficits were obtained from rainfall-runoff models such as HBV.33 Here, we use instead the new model PERSiST v. 1.0.17,14 a daily time step, semi-distributed rainfall-runoff model designed specifically for use with INCA models. Although PERSiST shares many conceptual characteristics with the HBV model, such as the temperature index representation of snow dynamics and evapotranspiration, it differs in its description of water storage.14 PERSiST uses the same conceptual representation of water storage as the INCA models. Coupling PERSiST with INCA allows a consistent conceptual model of the runoff generation process for both hydrological estimations and water chemistry simulations.
Water chemistry models.
Daily hydrological outputs from PERSiST, and weather forcing from the RCMs, were used as inputs for INCA-P. The catchment P-dynamic model INCA-P,15 one of the iterations of the INCA-suite of models, is a process-based, mass balance model that simulates temporal variation in P export from different land-use types within a river system. It has been used extensively in Europe and North America to simulate P dynamics in soils and surface waters and to assess the potential effects of climate and land management on surface water quality.7,11–13,15,34,35 We use a recent fully branched version of INCA-P11 (branched-INCA-P v. 0.1.31), in which reaches are defined as stretches of a river between two arbitrarily defined points, such as a gauging station, a topographic feature or a lake basin. INCA-P is so-called semi-distributed, that is, soil properties are spatially averaged within user-defined sub-catchment branches. It produces daily estimates of discharge (Q, m3 d−1), concentration of suspended solids (SS, mg L−1), soluble reactive P (SRP; μg L−1) and total phosphorus (TP; μg L−1). The application here (Fig. 1) simulates the 7 catchment reaches: five reaches of the Hobøl River catchment, each with defined land-use and hydrology (R1–R5); the local Storefjorden sub-catchment (R6); and the Vanemfjorden sub-catchment (R7). The multi-branch reach structure was established using GIS and land-use maps for the area (Section 2.3) and the location of monitoring stations and discharge point into lake basins.11
MyLake model.
The lake model used, MyLake v. 1.2.1, is a one-dimensional process-based model designed for the simulation of seasonal ice-formation and snow-cover in lakes, as well as for simulating the daily distribution of heat, light, P species, and phytoplankton abundance in the water column.16 MyLake has been successfully applied to several lakes in Norway, Finland and Canada16,36,37 to simulate lake stratification and ice formation.16,36,37 It uses daily meteorological input data such as global radiation (MJ m−2), cloud cover, air temperature (°C), relative humidity (%), air pressure (kPa), wind speed (m s−1) and precipitation (mm), as well as inflow volumes and P fluxes to produce daily temperature (T, °C) profiles in the water column, concentration profiles and outflow concentrations of SS, dissolved inorganic P (PO4–P, μg L−1), particulate inorganic P (PIP, μg L−1), dissolved organic P (DOP, μg L−1), chlorophyll-α (Chl, μg L−1) and TP. The biogeochemical processes linking these state variables in the water-column are the mineralisation of DOP and of Chl to PO4, and the removal of PO4 through phytoplankton growth (yielding Chl) or through sorption onto SS (yielding PIP). In the sediments, mineralisation of organic-P and equilibrium partitioning of PIP to the pore water govern the fluxes of PO4 to the water-column, while resuspension allows Chl and PIP to return to the bottom water. Details on the equations governing these processes are given by Saloranta and Andersen.16 In the MyLake model, phytoplanktons have a constant C
:
P ratio of 106
:
1 and a organic-P
:
Chl ratio of 1
:
1, such that particulate organic-P is a proxy for Chl. Similar stoichiometries and constant P
:
Chl ratios can be found in other models for lake plankton dynamics, such as PROTECH.25 Finally, total particulate P (PP = TP − PO4; μg L−1) was calculated offline and compared to field observations (see Section 2.3) to calculate performance metrics.
MyLake was set-up for 2 lake basins (Fig. 1), Storefjorden (L1) and Vanemfjorden (L2). The outputs of the R1 to R6 simulations from INCA-P are combined and used as inputs for L1. L1 and R7 are then combined and used as inputs for L2. The MyLake setups L1 and L2 are at the end of the model chain, because the lake Vanemfjorden (L2) discharges into the Oslo fjord.
2.3. Model input
The observed climate, precipitation, temperature and wind data at Lake Vansjø were obtained from daily weather data at the Norwegian Meteorological Institute stations (1715 Rygge; 1750 Fløter; 378 Igsi) located between the Vanemfjorden and Storefjorden basins (59°38′N, 10°79′E). These data were used as the common atmospheric forcing throughout the study; either as is for present-day climate or scaled using the RCM predictions for climate change scenarios (see Section 2.4). Catchment hydrology was constrained using daily flows measured at the gauging station at Høgfoss (station #3.22.0.1000.1; Norwegian Water Resources and Energy Directorate, NVE).
The land cover structure for the Vansjø–Hobøl catchment was constructed from GIS digital terrain elevation maps provided by the Norwegian Forest and Landscape Research Institute and complemented by a recent report on the fertilization regimes of agricultural fields.20 Historical nutrient outputs from waste-water treatment plants (WWTPs) were obtained from the online database KOSTRA, maintained by Statistics Norway (http://www.ssb.no/offentlig-sektor/kostra). TP and SS data were analysed downstream of Høgfoss, at Kure.38 P loadings from scattered dwellings are provided by the online GIS information system GISavløp maintained by the Norwegian Institute for Agricultural and Environmental Research (Bioforsk; http://www.bioforsk.no/webgis). The land cover of the Vansjø–Hobøl catchment is dominated by forestry (78%), agriculture (15%) and water bodies (7%). The agricultural land-use is dominated by cereal production (89%), with a smaller production of grass (9.8%), vegetables (0.6%) and potatoes (<0.1%). Together, agricultural practices contribute an estimated 48% of the total P input to the river basin, followed by natural runoff (39%), WWTPs (5%) and scattered dwellings (8%). It is estimated that these external sources of P contribute to the majority of the P loads to Lake Vansjø.20
For the Vanemfjorden and Storefjorden basins, water chemistry and temperature data were provided by the Vansjø–Hobøl monitoring program, conducted by Bioforsk and by the Norwegian Institute for Water Research (NIVA). Water-column sampling was conducted weekly from 1990 to 2004, and bi-weekly from 2004 on, at the deepest-site of both basins whose coordinates are given in Table 1, using a depth-integrating pipe water-column sampler positioned at a 0–4 m depth. Values of TP, PP, Chl and PO4 water-column concentrations for both basins are accessible through NIVA's online database (http://www.aquamonitor.no).
2.4. Scenarios and storylines
Scenarios are valuable to evaluate alternative directions for development and policy implementation. Here, we have defined scenarios representing possible futures in global and regional climates and in catchment management. We combine these climate predictions and management scenarios into storylines, which help convey the output of the simulations into quantitative expectations for future P loadings in the Vansjø catchment (Fig. 2). The assumptions made in defining these scenarios, and the choice made to combine them into storylines, are detailed below.
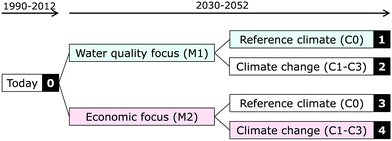 |
| Fig. 2 Management and climate scenarios defining the storylines. Storyline 0 represents the reference management focus and reference climate that were compared to observations in calibrating the river-lake model network and deriving model performance metrics. | |
Climate.
Three GCMs were used to obtain predictions according to the A1B greenhouse gas emission scenario (2030–2052) of the Intergovernmental Panel on Climate Change (IPCC).27 The A1 scenario family describes a future world of rapid economic and population growth and the introduction of new and more efficient technologies. It is subdivided into groups that describe alternative directions of technological change in the energy system. The A1B sub-scenario, which describes a balance between a growing reliance on fossil energies and an emergence of new technology, assuming that similar improvement rates apply to all energy supply and end-use technologies. This scenario projects that anthropogenic emission of greenhouse gases (CO2, CH4 and N2O) peaks and begins to decline past the year 2050. GCM runs, prepared from the results of the ENSEMBLES EU FP6 project,39,40 provided boundary conditions for the RCMs. The outputs of these model pairs, all based on the A1B scenario of climate change, are hereafter referred to as future climates C1–C3 (Table 2 and Fig. 3), whereas the climate condition during the reference period 1990–2012 is referred to as climate C0.
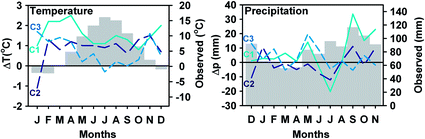 |
| Fig. 3 Monthly means of the changes in temperature and precipitation imposed by the climate models HadCM3/HadRM3 (solid line, C1), ECHAM5/RACMO (long dashed line, C2) and BCM/RCA (short dashed line, C3) for the period of 2030–2052 relative to the present-day conditions (C0) over the period of 1990–2012, along with monthly means of the observed temperature and precipitation over the same period (grey vertical bars). | |
Because the RCMs were based on spatial domains much larger than the catchment, they may contain seasonal biases. Consequently, RCM outputs for the Vansjø–Hobøl catchment were bias corrected on a monthly basis. Daily resolution scenario data for surface air temperature and precipitation were derived from a sub-set of these regional climate model simulations41 and implemented by scaling the observed weather (1990–2012). The observed temperatures were changed to reflect the increase in both median and variance predicted by the climate models. Precipitation was scaled using a ratio of change approach, multiplying observation by the ratio of observed (1990–2012) over predicted (2030–2052) precipitation. Averaged, monthly local changes in temperature and precipitation predicted by the three RCMs under the A1B scenario for the 2030–2052 period are shown in Fig. 3. Overall, HadRm3 predicts average yearly changes in both temperature and precipitation that are greater than those predicted by ECHAM5 or BCM (Table 2).
Management.
Three management scenarios were developed together with stakeholders involved in the catchment's land-use and water management. As a result, the following scenarios represent realistic actions that the stakeholders have the capacity to implement. The reference scenario (M0) represents historical riverine nutrient concentrations and current loadings from land-use, fertilization and WWTPs. The sustainable management scenario (M1), referred to as the “water-quality focus”, represents the implementation of measures to further mitigate the risk of eutrophication in the catchment. These measures impose (1) a 10% reduction in the agricultural land, which is then converted to a forest, (2) a 25% decrease in vegetable production, which is then converted to grass production, (3) a 25% decrease in P-based fertilizer applications, and (4) a 90% improvement in the P-removing performance of WWTPs. Finally, a less sustainable management scenario (M2), referred to as the “economic focus”, reflects a projected increase in anthropogenic pressure throughout the catchment due to population growth and an intensification of food production. Further growth of agricultural and urban activities in the catchment in scenario M2 is imposed as follows: (1) a 10% reduction of forest cover, which is then converted into agricultural lands, (2) a shift of 25% of the grass production to vegetable production, (3) an increase of fertilizer applications by 25%, and (4) a 25% increase in the P load of effluents from scattered dwellings and WWTPs throughout the catchment.
Storylines.
The management scenarios M1 and M2 were either considered with the reference climate (C0) or with future climate change, thus defining 4 storylines which represent the possible combined effects of climate change and management practices in the Vansjø–Hobøl catchment (Fig. 2). Storylines 1 and 2 encompass the water-quality focus scenario with and without climate change, respectively, while storylines 3 and 4 encompass the economic focus scenario with and without climate change, respectively. The reference storyline represents the present climate conditions combined with the historical management of the catchment.
2.5. Calibration and uncertainty analysis
PERSiST was manually calibrated against the measured stream flow in the Hobøl river at the end of reach R4 for the observation period of 1 January 1996 to 3 December 2000. The INCA-P and MyLake models were calibrated using a Markov Chain Monte Carlo (MCMC) approach. Given the large number of parameters involved in the simulation of 7 river reaches and 2 lake basins using INCA-P and MyLake, probably many alternative sets of parameters could achieve the same degree of fit with the observed data. Manual calibration identifies only one possible set, and perhaps not the best fit, while locally scoped and uniquely defined auto-calibration software, such as PEST, would fail to adequately address the multimodality and equifinality.42 To capture the envelope of acceptable parameter sets systematically throughout the parameter combination space, a probabilistic calibration was performed using a Bayesian inference scheme, where each parameter was given a prior distribution and a posterior distribution using a recent MCMC approach, within the framework of a self-adaptive differential evolution learning scheme (DREAM)42 implemented in MATLAB (Starrfelt et al., this issue).68 The calibration was performed by choosing site-specific parameters, which are not known with certainty beforehand, and allowing those values to vary within the parameter space.
INCA-P (28 parameters varied) was calibrated by using the MCMC-DREAM algorithm described by Starrfelt et al. (this issue)68 against log10-transformed time series acquired at R4 (Fig. 1) for the observation period of 1 December 1992 to 31 January 1995. After calibration, parameter sets from the last iterations were sampled and the model was run for the scenario period and over the whole catchment. Median simulated values from ∼600 runs per scenarios were then passed to MyLake. MyLake (10 parameters varied) was calibrated against a time series of measurements in the surface waters of the Vanemfjorden and the Storefjorden basins for the observation period of 1 April 2005 to 1 September 2012. Technical details on the sensitivity and uncertainty analysis of such a model network are given elsewhere.43
The goodness of fit between observations in the catchment and the model predictions from PERSiST and INCA-P, as well as between observations in the lake water columns and the model predictions from MyLake, was evaluated using the coefficient of determination (R2), the root-mean-square error (RMSE) and the Nash–Sutcliffe coefficient (NS) statistics. The latter was calculated both on normal and on log-transformed values. These metrics were chosen because they represent the following three major categories of model performance metrics:44 (1) standard regression statistics to determine the strength of the linear relationship between simulated and measured data (i.e., R2), (2) error indices to quantify the deviation in the units of the data of interest (i.e., RMSE) and (3) dimensionless techniques to provide a relative model evaluation assessment (i.e., NS). R2 values range from 0 to 1, with higher values indicating less error variance, and typically values greater than 0.5 are considered acceptable. RMSE values retain the same units as the constituent being evaluated and can be directly compared with the data (as in Fig. 4 and 5). A RMSE value of 0 indicates a perfect fit. NS ranges between −∞ and 1, with a value of 1 being optimal and values between 0.5 and 1 being generally viewed as good. Negative NS values indicate that the mean observed value is a better predictor than the simulated value, pointing to poor model performance. We refer the reader to a study by Moriasi44 for extensive discussion on the procedures used to qualify the calculated values of these statistics.
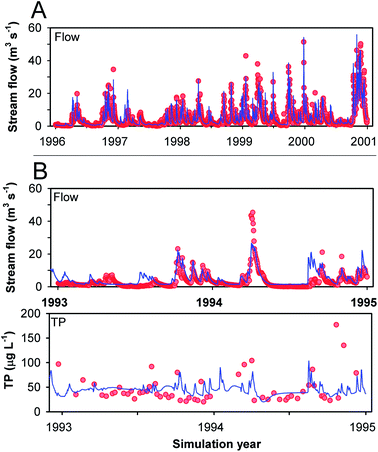 |
| Fig. 4 Observed (symbols) and simulated (solid line) stream flows at the end of R4 using the model PERSiST (panel A), as well as observed and simulated stream flows and TP at the end of R4 using INCA-P (panel B). | |
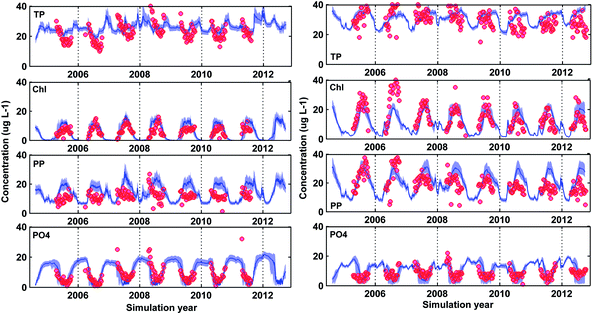 |
| Fig. 5 Calibration performance of MyLake at Storefjorden (L1, left panels) and Vanemfjorden (L2, right panels) for total phosphorus (TP), chlorophyll (Chl), particulate phosphorus (PP) and phosphate (PO4) over the calibration period of 2005–2012. The results are reported as the median (solid line), daily quartile statistics sampled from the parameter sets of equal likelihood (continuous area) together with the observations (circles). | |
In addition to the performance metrics described above, “target diagrams”45,46 were used to compare the model's performance with respect to Q, TP, Chl and PO4. Target diagrams conveniently represent aggregated performance metrics by plotting the normalized bias (B*, where * denotes normalization) against the normalized unbiased root mean square difference (RMSD'*).43,44B* is defined as:
| 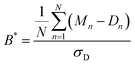 | (1) |
where
N is the total number of observations and model output pairs,
Dn is the observation at each site,
Mn is the corresponding model output, and
σD is the annual standard deviation of the observed data. RMSD'* is calculated as follows:
|  | (2) |
where sgn represents the sign of the standard deviation difference and
σM is the annual standard deviation of the modelled data. If the model standard deviation is greater than the observation standard deviation, RMSD'* is positive.
3. Results and discussion
3.1. Model performance
The hydrology of the catchment was well simulated with PERSiST and yielded satisfactory fits to the observed discharge (Fig. 4), as reflected by the high NS coefficient (>0.85; Table 3). The hydrological model HBV,33 previously used in conjunction with INCA-P, yielded similarly satisfactory simulations of flows.17 Although the use of log-transformed values yielded satisfactory fits with respect to NSlog for both Q and TP, the INCA-P calibration against TP measurements is characterized by relatively poor performance metrics (Table 3 and Fig. 4). Here, we aimed for a compromise between the performance in some components of the individual models and a realistic propagation of the changes in boundary conditions through the integrated system across the model components, as discussed by Voinov.18
Table 3 Summary of models' performance statistics. The coefficient of determination (R2), root-mean-square error (RMSE), and Nash–Sutcliffe coefficient on normal (NS) and log-transformed data (NSlog) for reach R4 (Hobøl at Kure), stations L1 (Storefjorden) and L2 (Vanemfjorden) of the model network
Parameter |
Model (station) |
R
2
|
RMSE |
NS |
NSlog |
Q |
PERSiST (R4) |
0.85 |
52.58 m3 s−1 |
0.85 |
0.99 |
Q |
INCA-P (R4) |
0.59 |
3.34 m3 s−1 |
0.48 |
0.99 |
TP |
INCA-P (R4) |
0.04 |
0.09 μg L−1 |
−0.51 |
0.16 |
TP |
MyLake (L1) |
0.93 |
6.37 μg L−1 |
0.19 |
0.99 |
TP |
MyLake (L2) |
0.94 |
7.76 μg L−1 |
−0.23 |
0.99 |
PO4 |
MyLake (L1) |
0.92 |
6.70 μg L−1 |
0.39 |
0.84 |
PO4 |
MyLake (L2) |
0.72 |
2.54 μg L−1 |
−0.96 |
0.90 |
Chl |
MyLake (L1) |
0.74 |
4.48 μg L−1 |
−0.68 |
0.89 |
Chl |
MyLake (L2) |
0.82 |
8.11 μg L−1 |
0.21 |
0.96 |
PP |
MyLake (L1) |
0.47 |
11.36 μg L−1 |
−0.52 |
0.92 |
PP |
MyLake (L2) |
0.85 |
8.16 μg L−1 |
−0.50 |
0.98 |
The water quality simulated by MyLake during the calibration period for the surface waters of Storefjorden (L1) and Vanemfjorden (L2) is shown in Fig. 5, and the corresponding model performance statistics are summarized in Table 3. The observed P dynamics in both basins display strong seasonal features, with TP, Chl, and PP all reaching maximum values during the summer, when the lake productivities are at their highest. Conversely, PO4 is at a minimum during the summer, consistent with its uptake by phytoplanktons. The observed TP values show a high degree of variability from week-to-week, likely due to the integrating nature of the TP parameter. A visual inspection of Fig. 5 shows that MyLake simulations for both basins well captured the seasonal minima in PO4 and maxima in both PP and Chl. The seasonal trends in Chl, a measure of the abundance of phytoplanktons, are also well captured by the model, with the exception of an algal bloom in the summer of 2006, whose magnitude was not fully captured (Fig. 5). The algal bloom in the summer of 2008 is reproduced by the model, although also underestimated, despite the high magnitude rain events that occurred throughout the catchment during that year. In particular, a single bank erosion event in the winter of 2008 resulted in high SS in the river.22 The NS metric is high for simulated Q with PERSiST, but low for simulations of TP with both INCA-P and MyLake (Table 3). This metric is unforgiving, in that it is strongly affected by simulations that do not match observed peak concentrations.
The target diagrams (Fig. 6) allow for the comparison of the model performance among parameters and stations in a normalized manner, independent of the magnitudes of the simulated values. The RMSD'* calculation involves the multiplication of a term in eqn (2) by the sign of difference between the standard deviation (σ) of simulations and observations. As a result, the RMSD'* provides information whether the σ of simulated values is larger or smaller than the σ of the observations. An increase in RMSD'* reflects an increase in the discrepancy between simulations and observations,46 pointing to incommensurability between what is modelled and the available observations, while lower values indicate less residual variance between them. B* represents systematic over- or under-estimation of the simulated vs. observed values. Fig. 6 reveals that the simulations are generally unbiased, and that the residual variances increase as we move from INCA-P to MyLake, that is, further along the model network. When compared to the observations, INCA-P simulations are less biased and, on an absolute scale, have a smaller RMSD'* than the simulations generated by MyLake. This information was not revealed solely by calculating the metrics reported in Table 2.
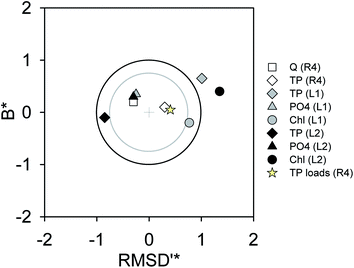 |
| Fig. 6 Target diagram presenting the normalized bias (B*) against the normalized unbiased root mean square difference (RMSD'*) of simulated Q, TP and TP loads for INCA-P at R4 and of simulated TP, PO4, and Chl for MyLake at Vanemfjorden and Storefjorden over the calibration periods. The median simulated values were used for TP, PO4 and Chl. The inner and outer circles indicate ±0.75 and ±1 standard deviations (σ) on the X-axis and 75% and 100% B* on the Y-axis, respectively. | |
Despite the low NS metrics reported for INCA-P, three lines of evidence suggest that the model delivers representative TP loads to the lake model: (1) a linear regression of cumulative TP loads estimated from the observed Q and TP vs. those predicted by INCA-P yields a R2 of 0.90 (n = 124, p < 0.05), (2) the B* and RMSD'* values obtained when comparing the estimated and predicted TP loads are low (Fig. 6), and (3) the performance of the lake models is acceptable. Previous – although simpler – INCA-P setups calibrated on data from other Norwegian catchments35,47 were also deemed satisfactory when evaluated against fortnightly or monthly TP loads rather than daily TP values. Thus, during the scenario period, the response of INCA-P to the climate and land-use changes is expected to be reasonable both in magnitude and in direction.
MCMC-DREAM analysis provides information on the sensitivity of the simulations to INCA-P and MyLake parameters. For INCA-P, of the 28 parameters tested, TP concentrations were the most sensitive to parameters controlling hydrology and erosion across the different land-uses, in particular the soil reactive zone time constant (d−1), which in INCA refers to the amount of water present in the soil and its residence time, the soil erodibility (kg m−2 d−1), the direct runoff time constant, and the base flow index. Downstream, P speciation predicted by MyLake was the most sensitive to 5 out of the 10 parameters tested: the re-suspension rate of sediments (m d−1), the sinking rate of suspended inorganic particles (m d−1), the algae growth rate (d−1), the heat vertical diffusion coefficient, and the wind sheltering coefficient. P speciation was moderately sensitive to the sinking rate (m d−1), the sorption coefficient of P onto inorganic particles (mg P m−3), and to the algae mortality rate (d−1), while insensitive to PAR saturation (mol quanta m−2 s−1) and snow albedo. The co-variance structure in the parameter space gathered by applying MCMC-DREAM analysis is described elsewhere for INCA-P (Starrfelt et al., this issue)68 and MyLake.43
3.2. Impact of climate and land-use change on the water quality
Several P-mitigation measures have been implemented in the Vansjø–Hobøl catchment over recent decades. These measures consist of reduced tillage to control erosion, reduced fertilizer application rate, implementation of vegetated buffer strips along most of the streams in cultivated areas, construction of artificial wetlands, and incremental improvement of WWTP performance.20,21 As a result, TP loads and Chl concentrations steadily decreased throughout the reference period (Fig. 7). Imposing the storylines described in Section 2.4 under these historical reference conditions reveals (i) what the water quality status in the Vansjø–Hobøl catchment would have been should additional management decisions have been made and (ii) the effect of different climate change scenarios on the water quality.
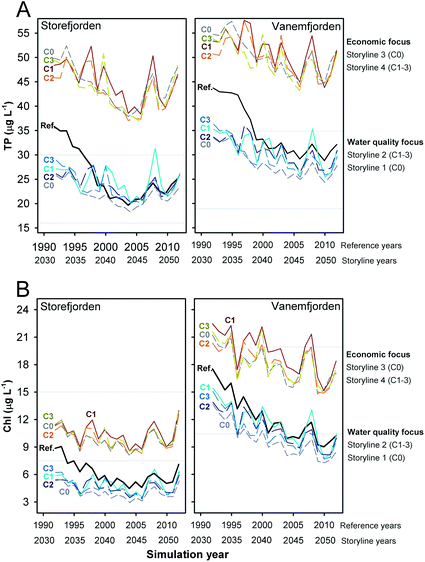 |
| Fig. 7 Predicted yearly median total P (panel A) and chlorophyll (panel B) at Storefjorden (L1) and Vanemfjorden (L2) by the MyLake model without (C0; storylines 1 and 3) or with climate change predictions made using the HadRm3 (C1), the ECHAM5 (C2) or the BCM models (C3) as climate forcing (storylines 2 and 4) for the river-lake model network. The thick solid lines represent the reference conditions and the thin horizontal solid lines indicate the WFD thresholds specific to each basin (see Table 4). | |
PERSiST and INCA-P predict that the hydrological response to climate change causes a significant increase in runoff and in the fluxes of TP to the lake basins. This result is consistent with observations in Danish lakes5 where higher TP loads were ascribed to climate-induced increases in rainfall. The MyLake output indicated no significant differences between the thermocline depths predicted under climate change and those predicted under present-day climate conditions (t-test, n = 523, p > 0.05). This suggests that changes in air temperature and precipitation in storylines 2 and 4 do not induce significant variations in the water-column structures at the scale modelled by MyLake (i.e., vertical resolution of 1 m). On the other hand, the ice cover duration was predicted by MyLake to decrease significantly (p < 0.05) under climate change; indeed, MyLake projected a shorter duration of ice cover for lakes in the entire Nordic region.37 For a given management scenario, TP and Chl values predicted under climate change were significantly higher (t-test, n = 523, p < 0.05) than those predicted using present-day climate conditions (96% of the times for Chl and 76% of the times for TP). Amongst the three climate models tested, HadRm3 (C1) projected the largest climate change39 and yielded the highest TP and Chl values. Most likely this was done to the higher amount of precipitation projected by HadRm3, which resulted in higher P loads and runoff from the catchment in INCA-P.
The increase in Chl production predicted by MyLake was higher in the summer months (Fig. 8). The model's handling of phytoplankton growth, which is temperature-driven when neither light nor PO4 is limiting,16 explains this result. Recent studies have further highlighted that temperature-mediated P release from lake sediments can increase under a warmer climate,5,6,48 thus promoting algal growth. However, the influence of higher temperatures on internal P loadings in Lake Vansjø cannot be ascertained here, because the relevant sediment–water processes are only partly implemented in the MyLake model (see Section 3.4). In addition, the climate scenario used here, A1B, predicted that greenhouse gas emissions will be curbed by the mid-21st century. Other scenarios, such as those in the A2 and B2 families of scenarios, assume larger increases of greenhouse gas emissions as well as higher increases in temperature and precipitation in Nordic catchments. The outcome of our simulations indicates that these climatic conditions would further increase the risk of eutrophication in Nordic lakes, as previously suggested.6,12,49,50 Thus, projected increases of Chl concentrations are likely conservative.
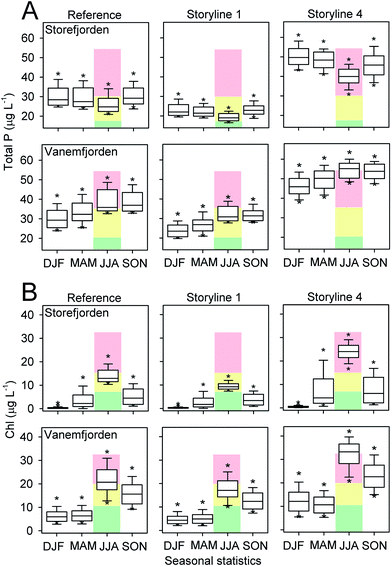 |
| Fig. 8 Seasonal range of MyLake-predicted daily TP (panel A) and chlorophyll (panel B) concentrations in the top 4 m of the Storefjorden (L1) and Vanemfjorden (L2) water columns. The green, yellow and red shaded zones indicate the basin-specific WFD water quality targets for good, moderate and bad water quality status, respectively (see Table 4), while the asterisks indicate the 5th and 95th percentile outliers. | |
In general, any given management scenario resulted in higher TP and Chl concentrations when the climate change was included. This is seen for the Storefjorden basin in the years following 2040, for which the detrimental effect of climate change overrides the beneficial effects of the water-quality focus storylines. Both TP and Chl reach values above those of the reference storylines, for which no additional P-load reduction was imposed. Nonetheless, and although the effects of climate change are significant, variations in water quality brought about by different management scenarios are always greater than those brought about by climate change (Fig. 7). Land-use and management regimes had a profound impact on the water quality, more so than the projected climate change under the A1B scenario. Relative to the reference storyline, imposing a water-quality focus (storyline 1) improved the water quality overall by decreasing TP and Chl by 24% and 33%, respectively, in Storefjorden, and by 18% and 23%, respectively, in Vanemfjorden. Conversely, an economic focus (storyline 3) adversely affected the water quality by increasing TP and Chl by 58% and 59%, respectively, in Storefjorden, and by 44% and 42%, respectively, in Vanemfjorden. It thus follows that storyline 1 represents the best case, while storyline 4 represents the worst case (Fig. 2).
3.3. Implications of climate and land-use change for water management
The seasonal distributions of the daily predicted TP and Chl concentrations (Fig. 8) show that the water quality is much worse during the summer months under all storylines. Using the lake-specific water quality thresholds of the WFD,51 we calculated the proportion of simulated days for which the regulatory thresholds for good/moderate and moderate/bad water quality were exceeded. These thresholds are specific to each lake type, so that the TP and Chl concentrations below which the water quality meets the guidelines are different for Storefjorden and Vanemfjorden (Table 4).
Table 4 Proportion (%) of days above the good/moderate or the moderate/bad thresholds set by the WFD for TP and Chl for basins of classes L-N3 (Storefjorden) and L-N8 (Vanemfjorden) in the months of June, July and August. Lower numbers indicate better water quality
Threshold name |
Good/moderate |
Moderate/bad |
Basin |
Storefj. |
Vanemfj. |
Storefj. |
Vanemfj. |
Parameter |
TP |
Chl |
TP |
Chl |
TP |
Chl |
TP |
Chl |
Threshold values (μg L−1) |
16 |
7.5 |
19 |
10.5 |
30 |
35 |
15 |
20 |
Reference (%) |
99 |
99 |
99 |
95 |
21 |
32 |
58 |
58 |
Storyline 1 (%) |
92 |
99 |
88 |
90 |
0 |
0 |
30 |
29 |
Storyline 4 (%) |
98 |
99 |
99 |
99 |
94 |
95 |
99 |
93 |
The water-quality focus scenario without climate change (storyline 1) increases the number of days for which the concentrations of TP and Chl are deemed “good” and has a greater influence on Chl than for TP (Table 4). Nevertheless, the “good/moderate” water quality threshold will still be exceeded 98–99% of the time for TP and 88–90% of the time for Chl. Under an economic focus scenario with climate change (storyline 4), the water quality degrades such that the concentrations of both TP and Chl exceed the moderate/bad threshold values 99% of the time in the summer. Together, these results suggest that it will be difficult to achieve the environmental targets set for TP and Chl in Lake Vansjø under the European WFD, even under the best-case scenario represented by storyline 1. More stringent water-quality focused measures are, therefore, likely needed. Arguably, a full assessment of the compliance of water quality indicators to the WFD directive requires greater details regarding algal species assemblages, in particular observations and predictions regarding the abundance of potentially harmful algae such as cyanobacteria, which in addition to higher TP levels are expected to be stimulated by the increased temperature.52
3.4. Sources of uncertainty
Assessing the level of uncertainty in the outcome of an environmental model provides a forthright basis for decision-making and regulatory formulation. The sources of uncertainty in water quality modelling at the river-basin scale range from uncertainty linked to the choice of processes represented, the uncertainty in the model parameters and the data themselves. Here, the uncertainty was assessed by performing auto-calibration (see Section 2.4) and accepting as usable those parameter sets yielding simulations of equal likelihood. This uncertainty is represented by the interquartile space shown in Fig. 5. Overall, the uncertainty in Chl predictions is the greatest around the time where its level peaks during spring and summer months (Fig. 5). Conversely, the model generally agreed with the observation on the timing of the clear water period occurring between the spring and summer blooms, as the uncertainty band visibly narrows around the simulated median (Fig. 5). For the scenario simulations, the uncertainty was the largest for scenarios where climate change and increased external nutrient loads were combined, relative to the scenarios with climate change alone. MyLake's predictions of phytoplankton abundance thus bear a greater uncertainty at higher biomass levels.
In addition to estimating uncertainty statistically, we identified shortcomings in the models that likely introduce further uncertainty in the predictions. As mentioned above, INCA-P predictions are sensitive to soil erosion parameters. INCA-P is somewhat limited in its handling of erosion processes and of particle transport, resulting in an increased uncertainty surrounding its predictions. Erosion events generating pulses of particles, such as landslides, were observed in the Vansjø–Hobøl catchment, for instance in 2008,20 when river bank erosion occurred following a flood and temporarily increased the particle load in the Storefjorden basin. The effect of bank collapse on runoff and particle transport is not spatially represented in INCA and particle retention measures, such as sedimentation ponds and buffer strips, cannot be explicitly represented in the model. Although such structures are better modelled using fully distributed codes,53 their effect on P migration in the catchment and on erosion control remains problematic to model because landscapes are not at steady-state and are subject to tipping points under increasing climatic pressures54 and extreme hydrologic events. Finally, INCA-P is a rather heavily parameterized model, and the lack of data on some of the processes represented in the model introduces uncertainty. Using INCA-P within the framework of an automated parameter estimation procedure, as has been done here, is likely a reasonable approach to estimate this uncertainty.34
MyLake's underlying conceptual model is purposely simple, in order to allow fast use of the model in automated auto-calibration schemes, as has been done here or in global sensitivity analysis. The drawback is that MyLake lacks the representation of some key processes, the most relevant of which are identified below. First, MyLake does not represent the phytoplankton community dynamics, thus not capturing possible community shifts due to climate change.55 Second, MyLake does not capture the thermodynamic decrease of oxygen availability at higher temperatures which, combined with the higher metabolism of respiring heterotrophic organisms, enhances the risk of oxygen depletion and ultimately of anoxia, in the hypolimnion.5 Given that the hypolimnetic oxygen concentration may control P sequestration and release by sediments, neglecting it introduces a source of uncertainty in the model's predictions, especially for lakes with high internal P loads. As suggested by Mooij et al.56 and others,5,48,57–60 describing the exchange of phosphorus between the sediments and the overlying water column beyond the daily timescale, as it is currently done in MyLake, is an important step in predicting eutrophication. Although recent lake models do represent internal P loading processes61,62 we elected to use the simpler MyLake model based on the available information on internal P loading in lake Vansjø (see Section 2.3). Third, MyLake, as with most lake system models used to study eutrophication, does not consider the coupled biogeochemical cycles of key macronutrients such as sulphur (S), calcium (Ca) and iron (Fe). It has long been recognized that these elements play a key role in controlling P cycling in the water column and in the sediments.63,64 In oligotrophic lakes a decrease in Ca concentrations, correlated with acid deposition, has been reported in Nordic lakes over the past decade and may have induced changes in plankton assemblages.63 Finally, a recent increase in dissolved organic carbon (DOC) loadings to Nordic lakes65 may have an effect on the lake photon budget and thus on phytoplankton growth. Although photon absorption by DOC is included in MyLake, it was not systematically investigated here due to the lack of DOC data in the river. These phenomena, acting in conjunction with climate and land-use change, may be changing the lake productivity in directions that, to our knowledge, current models do not predict.
4. Conclusion
This study demonstrates the usefulness and potential limitations of a novel network of process-based, mass-balance models linking climate, hydrology, catchment-scale P dynamics, and lake processes to support the decision-making needed to improve the surface water quality. The management scenarios tested here are projected to have a profound effect on the water quality. The model results suggest that achievement of the water quality target of good ecological status in eutrophic Nordic lakes such as Lake Vansjø represents a challenge, given the current land use and the expected changes in climatic conditions. In order to achieve good water quality status, managerial choices consistent with a water-quality focus scenario are needed. Such measures are deemed “climate-proof” because they will not only improve the water quality but also counteract the detrimental impact of projected climate change. Nevertheless, consistent with previous catchment-scale studies conducted in northern,35 central,66 and southern Europe,67 climate changes will probably worsen the water quality. Should the future Nordic climate (2030–2060) be wetter and warmer than that projected by the A1B scenario, additional stringent management measures must be implemented in order to achieve water quality. The conclusions presented here on the changes of water quality as a result of management and climate change are likely to hold even if different calibration periods, parameter sets, or even different catchment and lake models were used.
Acknowledgements
This study was funded by REFRESH (EU FP7 no. 244121), by the Strategic Institute Initiative “Climate effects from Mountains to Fjords” (Research Council of Norway no. 208279) at NIVA and by Eutropia (Research Council of Norway no. 190028/S30) at the University of Oslo. Monitoring data for this study came from NIVA, NVE, Bioforsk and Met. no. Monitoring programs were financed in part by MORSA. We thank stakeholders and water managers at MORSA for their work with the storylines. We acknowledge J. R. Selvik, A. B. Christiansen, S. Haande, and A. L. Solheim (NIVA) for their assistance with GIS, hydrological modelling, retrieval of observation data and for discussions on the scenarios, respectively.
References
- D. Tilman, J. Fargione, B. Wolff, C. D'Antonio, A. Dobson, R. Howarth, D. Schindler, W. H. Schlesinger, D. Simberloff and D. Swackhamer, Science, 2001, 292, 281–284 CrossRef CAS PubMed.
- P. M. Haygarth, A. Delgado, W. J. Chardon, M. I. Litaor, F. Gil-Sotres and J. Torrent, Soil Use Manage., 2013, 29, 1–5 CrossRef.
- V. H. Smith and D. W. Schindler, Trends Ecol. Evol., 2009, 24, 201–207 CrossRef PubMed.
-
WFD, CIS Guidance Document No. 3: Analysis of Pressures and Impacts, 92-894-5123-8, Directorate General Environment of the European Commission, Brussels, 2002 Search PubMed.
- E. Jeppesen, B. Kronvang, M. Meerhoff, M. Sondergaard, K. M. Hansen, H. E. Andersen, T. L. Lauridsen, L. Liboriussen, M. Beklioglu, A. Ozen and J. E. Olesen, J. Environ. Qual., 2009, 38, 1930–1941 CrossRef CAS PubMed.
- S. Kosten, V. L. M. Huszar, E. Becares, L. S. Costa, E. van Donk, L. A. Hansson, E. Jeppesenk, C. Kruk, G. Lacerot, N. Mazzeo, L. De Meester, B. Moss, M. Lurling, T. Noges, S. Romo and M. Scheffer, Global Change Biol., 2012, 18, 118–126 CrossRef.
- J. Crossman, M. N. Futter, S. K. Oni, P. G. Whitehead, L. Jin, D. Butterfield, H. M. Baulch and P. J. Dillon, J. Great Lake Res., 2013, 39, 19–32 CrossRef CAS PubMed.
- A. M. Michalak, E. J. Anderson, D. Beletsky, S. Boland, N. S. Bosch, T. B. Bridgeman, J. D. Chaffin, K. Cho, R. Confesor, I. Daloglu, J. V. DePinto, M. A. Evans, G. L. Fahnenstiel, L. L. He, J. C. Ho, L. Jenkins, T. H. Johengen, K. C. Kuo, E. LaPorte, X. J. Liu, M. R. McWilliams, M. R. Moore, D. J. Posselt, R. P. Richards, D. Scavia, A. L. Steiner, E. Verhamme, D. M. Wright and M. A. Zagorski, Proc. Natl. Acad. Sci. U. S. A., 2013, 110, 6448–6452 CrossRef CAS PubMed.
- B. Bajželj, J. M. Allwood and J. M. Cullen, Environ. Sci. Technol., 2013, 14, 8062–8069 CrossRef PubMed.
- L. Norton, J. A. Elliott, S. C. Maberly and L. May, Environ. Model. Software, 2012, 36, 64–75 CrossRef PubMed.
- P. G. Whitehead, L. Jin, H. M. Baulch, D. A. Butterfield, S. K. Oni, P. J. Dillon, M. Futter, A. J. Wade, R. North, E. M. O'Connor and H. P. Jarvie, Sci. Total Environ., 2011, 412, 315–323 CrossRef PubMed.
- H. M. Baulch, M. N. Futter, L. Jin, P. G. Whitehead, D. T. Woods, P. J. Dillon, D. A. Butterfield, S. K. Oni, L. P. Aspden, E. M. O'Connor and J. Crossman, Inland Waters, 2013, 3, 187–206 Search PubMed.
- L. Jin, P. G. Whitehead, H. M. Baulch, P. J. Dillon, D. Butterfield, S. K. Oni, M. N. Futter, J. Crossman and E. M. O'Connor, Inland Waters, 2013, 3, 207–220 Search PubMed.
- M. N. Futter, M. A. Erlandsson, D. Butterfield, P. G. Whitehead, S. K. Oni and A. J. Wade, Hydrol. Earth Syst. Sci., 2014, 18, 855–873 CrossRef.
- A. J. Wade, P. G. Whitehead and D. Butterfield, Hydrol. Earth Syst. Sci., 2002, 6, 583–606 CrossRef.
- T. M. Saloranta and T. Andersen, Ecol. Modell., 2007, 207, 45–60 CrossRef PubMed.
- Ø. Kaste, R. F. Wright, L. J. Barkved, B. Bjerkeng, T. Engen-Skaugen, J. Magnusson and N. R. Sælthun, Sci. Total Environ., 2006, 365, 200–222 CrossRef PubMed.
- A. Voinov and H. H. Shugart, Environ. Model. Software, 2013, 39, 149–158 CrossRef PubMed.
- F. Bouraoui, B. Grizzetti, G. Adelskold, H. Behrendt, I. de Miguel, M. Silgram, S. Gomez, K. Granlund, L. Hoffmann, B. Kronvang, S. Kvaerno, A. Lazar, M. Mimikou, G. Passarella, P. Panagos, H. Reisser, B. Schwarzl, C. Siderius, A. S. Sileika, A. A. M. F. R. Smit, R. Sugrue, M. VanLiedekerke and J. Zaloudik, J. Environ. Monit., 2009, 11, 515–525 RSC.
-
E. Skarbøvik and M. E. Bechmann, Some Characteristics of the Vansjø–Hobøl (Morsa) Catchment, Bioforsk Soil and Environment, Ås, 2010 Search PubMed.
-
A. Lyche Solheim, N. Vagstad, P. Kraft, Ø. Løvstad, S. Skoglund, S. Turtumøygard and J. R. Selvik, Tiltaksanalyse for Morsa (Vansjø–Hobøl-vassdraget) – Sluttrapport, OR-4377, Norsk institutt for vannforskning (NIVA), 2001.
-
E. Skarbøvik, M. Bechmann, T. Rohrlak and S. Haande, Overvåking Vansjø/Morsa 2008. Resultater fra overvåkingen i perioden oktober 2007 til oktober 2008, Bioforsk, vol. 4, Nr 13, Bioforsk, Ås, 2009.
-
S. Haande, A. Lyche Solheim, J. Moe and R. Brænden, Klassifisering av økølogist tilstant i elver og innsjøer i Vannområde Morsa iht, Vanndirectivet 6166–2011, Norsk institutt for vannforskning (NIVA), 2011.
- A. M. Dolman, J. Rücker, F. R. Pick, J. Fastner, T. Rohrlack, U. Mischke and C. Wiedner, PLoS One, 2012, 7, e38757 CAS.
- C. S. Reynolds, A. E. Irish and J. A. Elliott, Ecol. Modell., 2001, 140, 271–291 CrossRef CAS.
- E. J. Forland, T. E. Skaugen, R. E. Benestad, I. Hanssen-Bauer and O. E. Tveito, Arctic Antarct. Alpine Res., 2004, 36, 347–356 CrossRef.
-
N. Nakicenovic, J. Alcamo, G. Davis, B. de Vries, J. Fenhann, S. Gaffin, K. Gregory, A. Grubler, T. Y. Jung, T. Kram, E. L. La Rovere, L. Michaelis, S. Mori, T. Morita, W. Pepper, H. Pitcher, L. Price, K. Riahi, A. Roehrl, H.-H. Rogner, A. Sankovski, M. Schlesinger, P. Shukla, S. Smith, R. Swart, S. van Rooijen, N. Victor and Z. Dadi, IPCC Special Report on Emissions Scenarios, Cambridge, United Kingdom and New York, NY, USA, 2000 Search PubMed.
- C. Gordon, C. Cooper, C. A. Senior, H. Banks, J. M. Gregory, T. C. Johns, J. F. B. Mitchell and R. A. Wood, Climate Dynamics, 2000, 16, 147–168 CrossRef.
- J. H. Jungclaus, N. Keenlyside, M. Botzet, H. Haak, J. J. Luo, M. Latif, J. Marotzke, U. Mikolajewicz and E. Roeckner, J. Clim., 2006, 19, 3952–3972 CrossRef.
- T. Furevik, M. Bentsen, H. Drange, I. K. T. Kindem, N. G. Kvamstø and A. Sorteberg, Climate Dynamics, 2003, 21, 27–51 CrossRef PubMed.
- O. H. Otterå, M. Bentsen, I. Bethke and N. G. Kvamstø, Geosci. Model Dev., 2009, 2, 197–212 CrossRef.
- Z. Yu, E. J. Barron and F. W. Schwartz, Geophys. Res. Lett., 2000, 27, 2561–2564 CrossRef.
-
N. R. Sælthun, The“Nordic” HBV Model. Description and Documentation of the Model Version Developed for the Project Climate Change and Energy Production, Norwegian Water Resources and Energy Administration, Oslo, 1996 Search PubMed.
- S. Dean, J. Freer, K. Beven, A. J. Wade and D. Butterfield, Stoch. Environ. Res. Risk Assess., 2009, 23, 991–1010 CrossRef.
- C. Farkas, S. Beldring, M. Bechmann and J. Deelstra, Soil Use Manage., 2013, 29, 124–137 CrossRef.
- Y. Dibike, T. Prowse, B. Bonsal, L. d. Rham and T. Saloranta, Int. J. Climatol., 2012, 32, 695–709 CrossRef.
- S. Gebre, T. Boissy and K. Alfredsen, The Cryosphere Discuss., 2013, 7, 743–788 CrossRef.
-
E. Skarbøvik, S. Haande and M. Bechmann, Overvåking Vansjø/Morsa 2011–2012. Resultater fra overvåkingen i perioden oktober 2011 til oktober 2012, Bioforsk vol. 8, Nr 71, Bioforsk, Ås, 2013.
-
J. H. Christensen, M. Rummukainen and G. Lenderink, in ENSEMBLES: Climate Change and its Impacts, ed. P. van der Linden and J. F. B. Mitchell, Met Office Hadley Centre, FitzRoy Road, Exeter EX1 3PB, UK, 2009, p. 160 Search PubMed.
- M. R. Haylock, N. Hofstra, A. M. G. Klein Tank, E. J. Klok, P. D. Jones and M. New, J. Geophys. Res.: Atmos., 2008, 113, D20119 CrossRef.
-
M. Shahgedanova, Down-scaled Climate Projections for Eight Demonstration Catchments under Different SRES Scenarios, REFRESH Deliverable 1.6, University of Reading, United Kingdom, 2011 Search PubMed.
- J. A. Vrugt, C. J. F. ter Braak, C. G. H. Diks, B. A. Robinson, J. M. Hyman and D. Higdon, Int. J. Nonlinear Sci. Numer. Simul., 2009, 10, 273–290 Search PubMed.
-
K. Tominaga, Lake modelling: an interdisciplinary context, Ph.D. Dissertation no. 1368, University of Oslo, 2013 Search PubMed.
- D. N. Moriasi, J. G. Arnold, M. W. Van Liew, R. L. Bingner, R. D. Harmel and T. L. Veith, Trans. ASABE, 2007, 50, 885–900 CrossRef.
- F. J. Los and M. Blaas, J. Marine Syst., 2010, 81, 44–74 CrossRef PubMed.
- J. K. Jolliff, J. C. Kindle, I. Shulman, B. Penta, M. A. M. Friedrichs, R. Helber and R. A. Arnone, J. Mar. Syst., 2009, 76, 64–82 CrossRef PubMed.
- I. Panagopoulos, M. Mimikou and M. Kapetanaki, J. Soils Sediments, 2007, 7, 223–231 CrossRef CAS.
- M. Sondergaard, R. Bjerring and E. Jeppesen, Hydrobiologia, 2013, 710, 95–107 CrossRef CAS PubMed.
-
The Impact of Climate Change on European Lakes, ed. G. George, Springer, Dordrecht, 2010, 507 pp Search PubMed.
- M. Vetter and A. Sousa, Fundam. Appl. Limnol., 2012, 180, 41–57 CAS.
-
A. Iversen, Klassifisering Av Miljøtilstand I Vann Økologisk Og Kjemisk Klassifiseringssystem for Kystvann, Innsjøer Og Elver I Henhold Til Vannforskriften, Direktoratsgruppa for gjennomføringen av vanndirektivet, Trondheim, Norway, 2009 Search PubMed.
-
S. J. Moe, in Environmental Risk Assessment and Management from a Landscape Perspective, ed. L. Kapustka, W. G. Landis and A. Johnson, Wiley's, Hoboken, New-Jersey, 2010, p. 396 Search PubMed.
- D. Karssenberg, O. Schmitz, P. Salamon, K. de Jong and M. F. P. Bierkens, Environ. Model. Software, 2010, 25, 489–502 CrossRef PubMed.
- T. J. Coulthard, M. J. Kirkby and M. G. Macklin, Hydrol. Processes, 2000, 14, 2031–2045 CrossRef.
- C. C. Carey, B. W. Ibelings, E. P. Hoffmann, D. P. Hamilton and J. D. Brookes, Water Res., 2012, 46, 1394–1407 CrossRef CAS PubMed.
- W. Mooij, D. Trolle, E. Jeppesen, G. Arhonditsis, P. Belolipetsky, D. Chitamwebwa, A. Degermendzhy, D. DeAngelis, L. De Senerpont Domis, A. Downing, J. Elliott, C. Fragoso, U. Gaedke, S. Genova, R. Gulati, L. Håkanson, D. Hamilton, M. Hipsey, J. 't Hoen, S. Hülsmann, F. Los, V. Makler-Pick, T. Petzoldt, I. Prokopkin, K. Rinke, S. Schep, K. Tominaga, A. Van Dam, E. Van Nes, S. Wells and J. Janse, Aquat. Ecol., 2010, 44, 633–667 CrossRef.
- M. Sondergaard, J. P. Jensen and E. Jeppesen, Freshwater Biol., 2005, 50, 1605–1615 CrossRef.
- S. Katsev and M. Dittrich, Ecol. Modell., 2013, 251, 246–259 CrossRef CAS PubMed.
- G. K. Nürnberg, L. A. Molot, E. O'Connor, H. Jarjanazi, J. Winter and J. Young, J. Great Lake Res., 2013, 39, 259–270 CrossRef PubMed.
- J. McCulloch, A. Gudimov, G. Arhonditsis, A. Chesnyuk and M. Dittrich, Chem. Geol., 2013, 354, 216–232 CrossRef CAS PubMed.
- D. F. Burger, D. P. Hamilton and C. A. Pilditch, Ecol. Modell., 2008, 211, 411–423 CrossRef PubMed.
- J. G. C. Smits and J. K. L. van Beek, PLoS One, 2013, 8, e68104 CAS.
- K. Hadley, A. Paterson, R. Hall and J. Smol, Aquat. Sci., 2013, 75, 349–360 CrossRef CAS.
- J. Lehtoranta, P. Ekholm and H. Pitkanen, Ambio, 2009, 38, 303–308 CrossRef CAS PubMed.
- G. B. Lawrence, J. E. Dukett, N. Houck, P. Snyder and S. Capone, Environ. Sci. Technol., 2013, 47, 7095–7100 CAS.
- J. Kopáček, J. Hejzlar and M. Posch, Biogeochemistry, 2013, 115, 1–17 CrossRef.
- F. Caille, J. L. Riera and A. Rosell-Melé, Hydrol. Earth Syst. Sci., 2012, 16, 2417–2435 CrossRef CAS.
- J. Starrfelt and Ø. Kaste, Environ. Sci.: Processes Impacts, 2014 10.1039/c3em00619k.
|
This journal is © The Royal Society of Chemistry 2014 |