DOI:
10.1039/C3LC50572C
(Paper)
Lab Chip, 2013,
13, 3707-3713
Real-time image processing for label-free enrichment of Actinobacteria cultivated in picolitre droplets†
Received
8th May 2013
, Accepted 25th June 2013
First published on 26th June 2013
Abstract
The majority of today's antimicrobial therapeutics is derived from secondary metabolites produced by Actinobacteria. While it is generally assumed that less than 1% of Actinobacteria species from soil habitats have been cultivated so far, classic screening approaches fail to supply new substances, often due to limited throughput and frequent rediscovery of already known strains. To overcome these restrictions, we implement high-throughput cultivation of soil-derived Actinobacteria in microfluidic pL-droplets by generating more than 600
000 pure cultures per hour from a spore suspension that can subsequently be incubated for days to weeks. Moreover, we introduce triggered imaging with real-time image-based droplet classification as a novel universal method for pL-droplet sorting. Growth-dependent droplet sorting at frequencies above 100 Hz is performed for label-free enrichment and extraction of microcultures. The combination of both cultivation of Actinobacteria in pL-droplets and real-time detection of growing Actinobacteria has great potential in screening for yet unknown species as well as their undiscovered natural products.
Introduction
Since the 1940s, antibiotics derived from natural products of Actinobacteria have not only saved billions of lives, but also have significantly improved quality of life by minimizing infection-related distress. However, the demand for novel classes of antimicrobial substances is becoming increasingly urgent, as the emergence of multiresistant pathogens develops into a serious threat for public health. Recent screening efforts failed to provide new substances, even though it is commonly accepted that the majority of natural metabolic diversity is still untapped. Leading experts agree that 99% of soil-derived Actinobacteria are hitherto not culturable, which excludes their natural products from activity assessment.1,2 For several reasons, classical agar-plate-based cultivation approaches appear to lose momentum in unveiling new species: Limited throughput impedes testing a broad range of culture conditions, e.g. medium composition, pH, aeration, etc., which is indispensable for promoting growth of less abundant Actinobacteria with unknown physiology.3 By employing nutrient-rich standard media, ubiquitous, fast-growing strains are favoured, frequently outcompeting less assertive slow-growers with putatively interesting but yet unknown secondary metabolites.4,5 Outdated culture techniques and the mycelial growth of Actinobacteria also hamper efficient screening for biological activity after the growth phase. In particular, automated sample-processing, which is a prerequisite for high-throughput screening, is difficult to implement.
Recently, pL-droplet-based, surfactant-stabilised microfluidics proved its extraordinary potential for high-throughput implementation of biotechnological applications such as directed enzyme evolution,6–8 polymerase chain reaction (PCR)9,10 and dose–response testing11 – to mention only a few. Despite the undoubted success of pL-droplet-based screening techniques for a wide range of applications, droplet interrogation is mostly restricted to a fluorescence-based read-out,12–22 since it is currently the only approach allowing for the desired rates of in-line sample analysis.23
Here, we demonstrate the applicability of pL-droplets as a high-throughput cultivation platform for Actinobacteria. Furthermore, to provide a promising alternative to fluorescence-based interrogation methods, we establish automated imaging of droplet-confined structures with real-time image processing for label-free droplet analysis and sorting. For this purpose, we implement brightness-dependent, triggered imaging of single droplets. The feasibility of image-based droplet sorting (IDS) is investigated by growth-dependent sorting of spore-loaded droplets after incubation – a promising approach for selective enrichment of slow-growing Actinobacteria with putatively new secondary metabolites.
Experimental
Preparation of spore suspensions
Mycelium suspensions of Streptomyces puniceus JA2640 were spread on solid oatmeal agar media (20 g L−1 oatmeal, 20 g L−1 agar, tap water) for spore formation and incubated at 28 °C for 10–14 days. Spores were scraped from the surface after adding 5–10 mL phosphate buffered saline (PBS) and collected in a microtube. To remove the mycelia, the suspensions were centrifuged 2 min at 100g. The spore density in the supernatant was determined using a Thoma counting chamber and diluted with PBS to 109 spores mL−1. For storage at −20 °C, glycerol preservation medium24 was added in equal volumes to the spore suspension.
Microfluidic setup and periphery
Microfluidic chips were designed in-house and manufactured by LioniX BV. Channels were deep reactive ion-etched in fused silica to a depth of 50 μm. Platinum electrodes were sputtered onto the cover plate and sealed with a layer of silicon-dioxynitride for levelling and electrical insulation. Microfluidic channels were hydrophobised with RepelSilanTM (GE Healthcare). Novec HFE7500 (3M) with 0.5% Pico-Surf (Dolomite) surfactant served as the continuous phase and modified malt medium (MMM) (2 g L−1 yeast extract, 2 g L−1 beef extract, 15 g L−1 malt extract, pH 7.2) with suspended spores as the dispersed phase (0.5–1 × 106 spores mL−1). All fluids were actuated with neMESYS syringe pumps (cetoni GmbH), connected via 1/16′′ OD-PTFE-tubings (0.5 and 0.25 mm ID) to the microfluidic chips. After generation, droplets (140 pL in volume) were transferred into a silanised clear-glass incubator which was handcrafted from a micro-insert for HPLC-vials by removal of the tip with a diamond cutter and then sealed with silicon-rubber plugs. Incubation was accomplished at room temperature. To generate a 20 kHz AC-electric field of 110 V for sorting, a device chain comprising a USB-1608FS analog/digital-converter (Measurement Computing), a 33210A function generator (Agilent) and a 623B high-voltage amplifier (TREK) was used. The estimated maximum field strength was 20 kV cm−1. Efflux velocities downstream of the sorting junction were regulated with an OB1 pressure controller (Elveflow) connected to both chip outlets with a droplet incubator in between. An Eosens 3CL high-speed camera (Mikrotron) was used to observe and validate the sorting.
Influence of electric field-assisted sorting on viability of Streptomyces puniceus
To determine whether there were any detrimental effects of electric field-assisted sorting, all droplets were subjected to field exposure irrespective of their content after 36 h incubation. 10 μl were extracted from the resulting emulsion and carefully suspended in 4 mL melted MMM soft-agar (0.6% agar, 50 °C) by gentle hand-rolling of the test tube. 2 ml were spread out on MMM agar plates (2% agar) and colony forming units (CFUs) were counted after three days incubation at 28 °C (ESI†).
Triggered imaging
Droplets were detected in transmissive mode with a photodiode attached to the front port of an inverted microscope (Zeiss Axio Observer Z1). To enable single-droplet detection at five-fold magnification, the sensor area of the photodiode was reduced by a 150 μm pinhole aperture in closest possible proximity to the image plane. The amplified signal of the photodiode was fed into an inverted Schmitt trigger circuit, which was connected to the trigger input of a Pike-F032B camera (Allied Vision Technologies) attached to the side port of the microscope. Frame grabbing and image analysis was implemented in a callback function that was executed upon notification by the camera after receiving a new frame (Fig. 1).
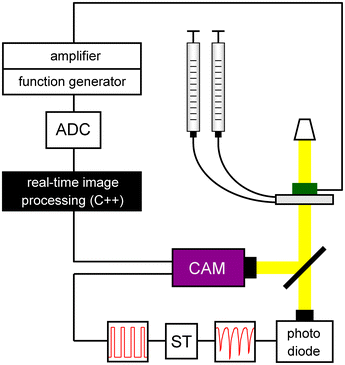 |
| Fig. 1 Experimental setup for triggered imaging, image analysis and sorting of single pL-droplets. Droplets are detected by the photodiode due to total light reflection at their interface with the carrier oil. The resulting signal is transformed into a TTL-signal by an inverted Schmitt trigger (ST) that actuates the camera. Frame grabbing, image processing, time buffering and pulse generation are executed within a callback function once a new frame is available. In case of detected hyphae, the function generator is triggered via the A/D-converter (ADC). The amplified square wave serves to sort the respective droplet. | |
Automated real-time image processing
Automated real-time image processing was implemented in C++ using the computer vision library opencv.25 Calculations were performed on a 64-bit Windows 7 system with Intel® CoreTM i7-2600 CPU @ 3.40 GHz x8 and 8GB RAM.
The main objective of image analysis is the detection of droplets, which are observed as circular objects with radial decreasing light scattering properties, and the detection of microbial hyphae growing inside the droplets (Fig. 2). Images received from the camera were saved as 8-bit gray level images with intensity values between 0 and 255. The detection of droplets and microbial hyphae was achieved by applying a Difference of Gaussians (DoG) filter,26 constructed by subtracting two Gaussian filters that can have different dimensions and/or variances. The Gaussian filters had dimensions 7 × 7 with variances σ1 = 1 and σ2 = 3. Image convolution by the DoG filter preserves the droplet boundaries and the fine structures inside the droplets. Histogram normalisation was performed to enhance contrast and the image was subsequently binarised using global intensity thresholding with threshold tb = 10. Noise was reduced by removing small foreground objects of size less than ts = 5 px. Finally, a DoG-filtered, normalised and binarised reference image Î, which was taken from an empty microfluidic channel, was subtracted to minimise background noise. After these pre-processing steps, images exhibited droplet boundaries and microbial hyphae as white foreground objects (Fig. 2).
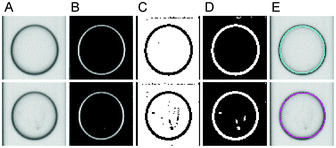 |
| Fig. 2 Image processing steps for droplet segmentation and growth classification. First and second rows show empty and colonised droplets, respectively. (A) Original images. (B) Applying the Difference of Gaussians and (C) binarisation by intensity thresholding. (D) Background subtraction and removal of small foreground objects. (E) Droplet classification using the number of detected hyphae thn and the hyphal sizes ths. The cyan and magenta circles indicate classification as empty and occupied droplets, respectively. | |
The pre-processed, binary image was partitioned into background and the region of interest (ROI). Of note, every contour pixel of the ROI either belongs to the boundary of a droplet or to that of the encapsulated mycelia. To ensure recognition of droplets, the contour of the ROI was subjected to a circularity criterion based on its signature. The signature is defined by the distance of all boundary pixels to the centre of the ROI as a function of the angle.26 The signature of a perfectly circular droplet boundary with radius r and no encapsulated mycelia is the constant function f(φ) = r, where φ denotes the polar angle. To determine the circularity of the contour pixels of the ROI, the variation of f(φ) from its mean value
was calculated. If the standard deviation
was lower than the empirically determined threshold
tc = 1 px, the ROI was classified as a circular droplet without encapsulated mycelia. However, hyphal structures of
Actinobacteria appeared as white foreground objects associated with contour pixels inside the ROI that give rise to values of
σs exceeding
tc. In this case, the droplet area was determined by estimating the minimum covering circle of the ROI followed by its adaptation to the boundary of the droplet.
Classification of droplets as empty or colonised by Actinobacteria was dependent on the number of detected hyphae thn and their areas ths. Threshold values were set to also ensure detection of faint hyphae. Thus, droplets are classified as colonised if at least one hypha was recognised (thn = 1) which exceeded a minimum size of ths = 5 px.
Results and discussion
pL-droplets as cultivation platform for Actinobacteria
Droplets of ∼140 pL were generated at 520 Hz. This translates into a maximum pure culture generation rate of ∼190 Hz, if spore concentrations in the aqueous phase are adjusted according to Poisson's prediction for highest single encapsulation probability. However, we used lower spore concentrations in all experiments to ensure sufficient representation of empty droplets for testing purposes. The small size of the spores used for seeding (<1 μm) did not influence droplet pinch-off at the flow focusing junction. Therefore, we achieved polydispersities of droplet diameters7,27 lower than 1.5% in each experiment. After transfer into the incubation vial, spore germination and formation of small mycelial pellets within the droplets were observed within less than 24 h. Interestingly, strong mycelial growth during prolonged incubation for several days did not disrupt the droplet interface. Droplet integrity and hence its function as an individual small-scale bioreactor was preserved (Fig. 3). Nonetheless, the polydispersity of reinjected droplets increased with incubation time, mainly due to a reduction in droplet volume associated with high mycelial density (data not shown). As already described by Boitard et al.,28 growing bacteria catabolise dissolved nutrients to volatile products (e.g. CO2). These diffuse out of the droplets and hence reduce the osmotic pressure causing water efflux towards empty droplets.
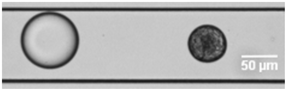 |
| Fig. 3 Droplet with microculture exhibiting strong mycelial growth after prolonged incubation period (18 days). | |
Single droplet detection and triggered imaging
After 24–36 h incubation, droplets were reinjected into the imaging and sorting chip by pushing with carrier oil. At a reinjection ratio of 1
:
20 (droplets to carrier oil), droplets were sufficiently spaced to allow individual detection using the photodiode in bright field illumination, exploiting total light reflection at the droplet phase boundary. After setting the light intensity for optimal illumination, the signal-to-noise ratio (SNR) calculated as
was ∼47 dB, indicating the high reliability of triggered imaging (Fig. 4).
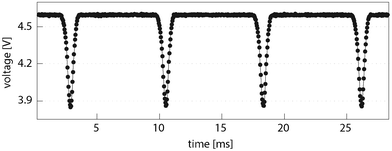 |
| Fig. 4 Photodiode signal for reinjected droplets detected in brightfield at 5-fold magnification. | |
By adjusting the reference voltage of the Schmitt trigger to the range of the photodiode signal, each inverse voltage peak generated by a droplet was reliably transformed into a TTL-conform signal for camera triggering. However, satellite droplets overstepping a critical minimal size or shading caused erroneous triggering, which was observed in less than 0.03% of the images.
Recognition of mycelia by real-time image processing
Each triggered image of a droplet is analysed on the fly to determine if spore germination and hyphal growth occurred in the droplet. For this purpose, the callback function implementing real-time image processing is invoked as soon as a new frame is available. To ensure simultaneous detection of faint mycelial structures and droplet boundaries, images require appropriate illumination as well as sufficient resolution and must be focused to the central plane of the droplet. Due to the fine structures of hyphae they typically appear as blurred edges, resulting in a low signal-to-noise ratio, which is a challenging condition for automated image analysis. Low resolution may ultimately lead to recognition failure and eventually droplet segmentation errors. In contrast, changes in illumination influence image processing only to a small extent. Within reasonable limits, adjustments in illumination shift gray level intensities without substantially altering their range. Recognition of mycelia is further dependent on their position within the droplet. Besides hyphae not being located in the focal plane, proximity to the droplet boundaries may impede recognition due to light refraction at the interface.
Several edge detection methods, including intensity thresholding, Sobel and Canny operators as well as morphological operators, are available for droplet segmentation.29 We applied a Difference of Gaussians (DoG) method that was tailored to simultaneously segment droplet boundaries and microbial hyphae. The selection of the appropriate Gaussian kernel dimensions and variances for the DoG is crucial for correct mycelia segmentation. The DoG algorithm applies a digital band pass filter that (i) removes high and low frequencies, i.e. noise and homogeneous regions, respectively, and (ii) preserves intermediate frequencies associated with hyphae and droplet boundaries. To allow for recognition of faint mycelia, we empirically set the Gaussian kernel dimensions according to the typical shapes of the bacterial hyphae.
Detected regions are classified as empty or colonised droplets according to the applied parameter configuration for minimum hyphae number thn and size ths. Fine-tuning these parameters changes the sensitivity of growth detection. Hence, the algorithm can be adapted to a particular application, e.g. sorting based on a user-defined minimum amount of biomass to discriminate Actinobacteria with different growth rates.
Computation time varies mainly dependent on the number of boundary pixels of the ROI. Images of small droplets are usually processed more rapidly than images of large droplets unless they contain a large number of hyphae. Droplets with high mycelia content need time-consuming image processing steps to determine the correct boundary of the droplet. Higher performance may be achieved by outsourcing the computations to the graphics processing units (GPU), which is also supported by the opencv library.25
Image-based droplet sorting (IDS)
Depending on the results from the real-time image processing, droplets were sorted using dielectrophoretic forces in a y-junction (Movie S1, ESI†). To gain insight into triggered imaging and IDS performance, all required operations for sorting of a single droplet were grouped into three phases (Fig. 5): detection and imaging (phase I), frame grabbing and image processing (phase II) and electric pulse generation (phase III). Phase I was dominated by the exposure time of the camera, which was set to the constant minimum value of 16 μs. In contrast, phase II lasted 0.48 ms (1.61 ms) for images of size 72 × 72 px (144 × 144 px) on average, exhibiting strong variations due to differences in the time required for processing of single images (discussed above). The time for frame grabbing was negligible. Since the droplet residence time in proximity to the sorting electrodes is a function of the droplet velocity, sorting at moderate frequencies of up to 100 Hz was not affected by variations in the image processing time. The difference in the minimal and maximal time required for analysis of a single droplet at 10-fold magnification was 0.89 ms (Fig. 6A), opposed to a time slot of ∼4.5 ms for the droplet being sufficiently close to the sorting electrode and displaced by an electric pulse. However, it may be desirable to realise higher sorting frequencies at concomitant reduction of inter-droplet spacing, which translates into sorting structures of reduced dimensions and a smaller time window for droplet displacement. To anticipate IDS under these conditions, we implemented a time buffer after the image analysis function. Applied to IDS with 144 × 144 px images, we could reduce the range of the overall duration of the callback function by 47% to 0.83 ms (Fig. 6B). Surprisingly, further reduction of this range was impeded by the process of pulse generation (phase III), which constitutes the last part of the callback function. The minimal and maximal time required for pulse generation was 0.44 ms and 1.34 ms, respectively, presumably depending on the current processor load and the internal priority of the thread managing the corresponding function. Therefore, applications with ultra high-throughput will also require optimised pulse generation control to enable precise timing.
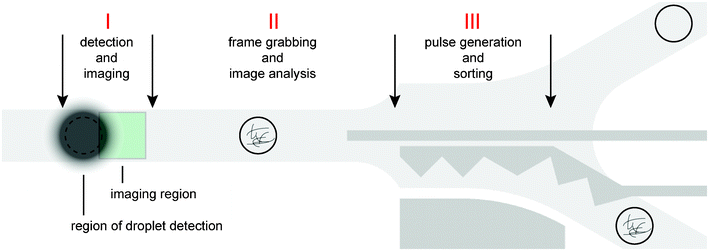 |
| Fig. 5 Spatio-temporal arrangement of the three phases for triggered imaging and sorting. Phase I: Droplet detection by the photodiode and subsequent triggered imaging. The distance between the regions of droplet detection and imaging, as well as their location, depends on configured droplet velocity and exposure time. Phase II: Image retrieval and analysis implemented in the callback function. Phase III: Generation of sorting pulse transmitted through the electrodes (dark grey) based on the outcome of the image analysis. | |
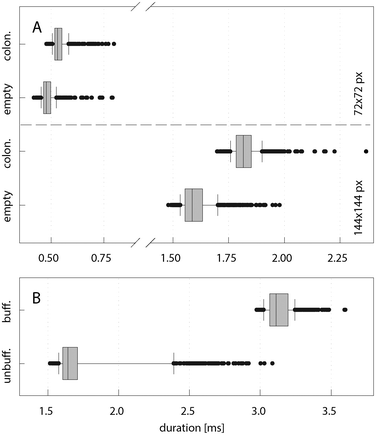 |
| Fig. 6 Computation time analysis of phase II (frame grabbing and image processing) and the callback function. (A) Duration of phase II for empty and colonised droplets. The average computation time was 0.48 ms (1.61 ms) for images of size 72 × 72 px (144 × 144 px). (B) Duration of the callback function for image size 144 × 144 px with and without implementation of a time buffer (set to 2.5 ms) after image analysis. Whisker caps indicate the 10th and 90th percentile. A sample set of 1000 images was used. All outliers are displayed. | |
Validation of image-based droplet sorting
We monitored droplet sorting by an additional high-speed camera enabling a complete view on the sorting structure and the afferent channel (Movie S1, ESI†). This allowed for tracking of single droplets, which eventually revealed hyphae that were hidden in a different focal plane upon triggered imaging. The statistical measures sensitivity (true positive rate) and specificity (true negative rate) were calculated to evaluate the performance of the sorting procedure. We observed a sensitivity of 70% and a specificity of over 99%, i.e. 70% of the colonised droplets and more than 99% of the empty droplets were sorted correctly. The results confirmed the hypothesis of potential missorting events due to the localisation of hyphae in a different focal plane. At the time point of image triggering they may not be recognised, leading to false sorting of the corresponding droplets. However, the sensitivity can be easily improved by employing chips with reduced channel height, e.g. in the range of 20 μm, decreasing the probability of not seeing hyphae.
Another potential source of sorting errors is the image processing algorithm itself. To verify its accuracy, we classified a set of 500 images with different DoG filters (Table 1). The number of false negative and false positive classifications was manually assessed by four experts in an independent fashion. Parameter setting 3 yields a true positive rate of 94% and a true negative rate of over 99%. Compared to other settings, fewer colonised droplets were misclassified while empty droplets were correctly classified with higher probability. The occurrence of false negative classifications is mainly caused by a low signal-to-noise ratio, i.e. faint hyphae that are not in the optimal focal plane cannot be clearly distinguished from noise. The overall classification into empty and colonised droplets exhibited an accuracy of 98%. This value is further increased to 99% by using a higher magnification (Table 2), revealing more details of the structures confined in the droplets. Different values for the specificity observed comparing Table 1 and Table 2 are due to different ratios of colonised to empty droplets.
Table 1 Validation of 500 images with different parameter settings. Setting 1: Gaussian kernel dimensions of size 5 × 5 with variances σ1 = 1.5 and σ2 = 1. Setting 2: Gaussian kernel dimensions of size 5 × 5 and 3 × 3 with variances σ1 = 3 and σ2 = 2. Setting 3: Gaussian kernel dimensions of size 7 × 7 with variances σ1 = 1 and σ2 = 3. Images were scanned for false negatives (FNs) and false positives (FPs) in four independent attempts and mean values are shown in the table. The true positive (TP) and true negative (TN) rate (sensitivity and specificity, respectively) describe the performance of the image processing algorithm
|

|

|

|

|
Sensitivity |
Specificity |
Accuracy |
Setting 1 |
13.5 |
40 |
269.5 |
177 |
92% |
87% |
89% |
Setting 2 |
41.5 |
0.25 |
330.5 |
127.75 |
75% |
>99% |
92% |
Setting 3 |
10.3 |
0.25 |
319.75 |
169.75 |
94% |
>99% |
98% |
Table 2 Droplet sorting validation at different magnifications (low = 72 × 72 px, high = 144 × 144 px). For each magnification three samples of sets of 500 images were generated within one droplet sorting experiment. The classified images were examined for false negative (FN), false positive (FP), true negative (TN) and true positive (TP) detection of microbial hyphae. The corresponding mean values are shown in the table
|

|

|

|

|
Sensitivity |
Specificity |
Accuracy |
Low resolution |
1.67 |
2.33 |
453.33 |
42.67 |
95% |
96% |
>99% |
High resolution |
2.67 |
0.67 |
442.67 |
54.0 |
99% |
95% |
>99% |
Advantages of pL-droplets and IDS over other high-throughput approaches
In their pioneering work, Martin et al.30 demonstrated cultivation of Actinobacteria within nL-droplets. Yet, to the best of our knowledge, the work presented here is the first report of mycelia forming bacteria being cultivated within surfactant-stabilised pL-droplets. By selective depletion of fast-growing species, which are likely to produce already known secondary metabolites, enrichment of slow-growing Actinobacteria with yet undiscovered natural products can be achieved.
Compared to state of the art microtiter plate (MTP)-based cultivation platforms,6,31 the rate of generation of pure cultures was increased by at least two orders of magnitude, supplying the throughput that is required to provide new momentum to natural product research.2,32,33 Ingham et al.34 reached a similar throughput with unicellular microorganisms cultivated in micro-Petri dishes etched into porous aluminium oxide (PAO) chips. However, all post-cultivation steps to recover strains were performed manually and the system was prone to cross-contamination. While approaches using gel microdroplets (GMDs)35 were shown to be capable of providing a throughput in the range of ∼106 cultures per hour, further handling of microcolonies after the initial growth phase required staining with non-lethal fluorogenic dyes and expensive FACS-equipment to enable detection and sorting. In contrast, IDS is label-free and independent of a FACS device, which opens up new opportunities for effective sample treatment. Droplets sorted on-chip may be separately deposited into wells of an MTP, which allows fully automated hit recovery and validation while avoiding FACS-inherent sample pooling. Moreover, pL-droplets lack a solid gel or alginate shell and therefore remain amenable to electric-field-supported picoinjection of aqueous solutions or suspensions,36 which was verified in preliminary experiments (Movie S2, ESI†). By adding reporter cells susceptible to antibiotics, our approach paves the way for ultra-high-throughput antimicrobial whole-cell-testing of soil sample-derived cultures.37–39 This concept is further enhanced by the possibility of exploring microbial interactions,40 which have been shown to favour growth of rare species and to stimulate production of secondary metabolites in Actinobacteria.41,42 The combination of assay flexibility and unsurpassed throughput makes pL-droplet-based screening a powerful technique for the discovery of novel natural products. Yet, to provide a fully functional screening system, a single-droplet depositing device as interface to MTPs is required to enable reliable strain recovery, but an adaption of related systems43 can be expected in the near future.
Conclusions
The combination of both pL-droplets as a ultra high-throughput screening platform and image-based droplet sorting (IDS) has great potential to accelerate generation of pure cultures of yet uncultivated Actinobacteria species – a rich source of novel natural products. Furthermore, this technique is a leap towards more complex, multistep screening for active secondary metabolites with fully automated recovery of their producers.
Triggered imaging with real-time IDS can be customised for the detection and evaluation of any droplet-confined structures. It is therefore a highly promising alternative to the widely applied fluorescence-based read-out and sorting methods. The performance of triggered imaging and IDS is mainly affected by computational power and the sorting structures employed, which may be easily improved to achieve even higher sorting rates. Hence, pL-droplet-based microfluidics – an uHTS platform with rapidly increasing acceptance – is enhanced by a powerful tool for label-free, real-time droplet discrimination.
Acknowledgements
The authors thank Thomas Weber, Lisa Mahler and Karin Perlet for their assistance to this work. We are grateful to J. Michael Köhler (Technical University Ilmenau) for his comments on the manuscript. This work has been supported by the "International Leibniz Research School for Microbial and Biomolecular Interactions", the excellence graduate school "Jena School for Microbial Communication" which is funded by the German excellence initiative, and the German Federal Ministry of Education and Research BMBF (Project DiNAMiD, grant no. 0315501B).
References
- M. Watve, V. Shejval, C. Sonawane, M. Rahalkar, A. Matapurkar, Y. Shouche, M. Patole, N. Phadnis, A. Champhenkar, K. Damle, S. Karandikar, V. Kshirsagar and M. Jog, Curr. Sci., 2000, 78, 1535–1542 Search PubMed.
- R. H. Baltz, Curr. Opin. Pharmacol., 2008, 8, 557–563 CrossRef CAS.
- K. Alain and J. Querellou, Extremophiles, 2009, 13, 583–594 CrossRef.
- P. H. Janssen, P. S. Yates, B. E. Grinton, P. M. Taylor and M. Sait, Appl. Environ. Microbiol., 2002, 68, 2391–2396 CrossRef CAS.
- K. Zengler, G. Toledo, M. Rappe, J. Elkins, E. J. Mathur, J. M. Short and M. Keller, Proc. Natl. Acad. Sci. U. S. A., 2002, 99, 15681–15686 CrossRef CAS.
- J. J. Agresti, E. Antipov, A. R. Abate, K. Ahn, A. C. Rowat, J.-C. Baret, M. Marquez, A. M. Klibanov, A. D. Griffiths and D. A. Weitz, Proc. Natl. Acad. Sci. U. S. A., 2010, 107, 4004–4009 CrossRef CAS.
- A. Fallah-Araghi, J.-C. Baret, M. Ryckelynck and A. D. Griffiths, Lab Chip, 2012, 12, 882–891 RSC.
- B. Kintses, C. Hein, M. F. Mohamed, M. Fischlechner, F. Courtois, C. Lainé and F. Hollfelder, Chem. Biol., 2012, 19, 1001–1009 CrossRef CAS.
- N. R. Beer, E. K. Wheeler, L. Lee-Houghton, N. Watkins, S. Nasarabadi, N. Hebert, P. Leung, D. W. Arnold, C. G. Bailey and B. W. Colston, Anal. Chem., 2008, 80, 1854–1858 CrossRef CAS.
- M. M. Kiss, L. Ortoleva-Donnelly, N. R. Beer, J. Warner, C. G. Bailey, B. W. Colston, J. M. Rothberg, D. R. Link and J. H. Leamon, Anal. Chem., 2008, 80, 8975–8981 CrossRef CAS.
- O. J. Miller, A. El Harrak, T. Mangeat, J.-C. Baret, L. Frenz, B. El Debs, E. Mayot, M. L. Samuels, E. K. Rooney, P. Dieu, M. Galvan, D. R. Link and A. D. Griffiths, Proc. Natl. Acad. Sci. U. S. A., 2012, 109, 378–383 CrossRef CAS.
- T. M. Tran, F. Lan, C. S. Thompson and A. R. Abate, J. Phys. D: Appl. Phys., 2013, 46, 114004–114021 CrossRef CAS.
- J. Q. Boedicker, L. Li, T. R. Kline and R. F. Ismagilov, Lab Chip, 2008, 8, 1265–1272 RSC.
- E. Brouzes, Single-Cell Analysis, 2012, 853, 105–139 CrossRef CAS.
- J. Clausell-Tormos, D. Lieber, J.-C. Baret, A. El-Harrak, O. J. Miller, L. Frenz, J. Blouwolff, K. J. Humphry, S. Koster, H. Duan, C. Holtze, D. A. Weitz, A. D. Griffiths and C. A. Merten, Chem. Biol., 2008, 15, 427–437 CrossRef CAS.
- F. Courtois, L. F. Olguin, G. Whyte, D. Bratton, W. T. Huck, C. Abell and F. Hollfelder, ChemBioChem, 2008, 9, 439–446 CrossRef CAS.
- L. Granieri, J.-C. Baret, A. D. Griffiths and C. A. Merten, Chem. Biol., 2010, 17, 229–235 CrossRef CAS.
- A. Huebner, M. Srisa-Art, D. Holt, C. Abell, F. Hollfelder, A. J. deMello and J. B. Edel, Chem. Commun., 2007, 1218–1220 RSC.
- L. Mazutis, A. F. Araghi, O. J. Miller, J.-C. Baret, L. Frenz, A. Janoshazi, V. Taly, B. J. Miller, J. B. Hutchison, D. Link, A. D. Griffiths and M. Ryckelynck, Anal. Chem., 2009, 81, 4813–4821 CrossRef CAS.
- B. El Debs, R. Utharala, I. V. Balyasnikova, A. D. Griffiths and C. A. Merten, Proc. Natl. Acad. Sci. U. S. A., 2012, 109, 11570–11575 CrossRef CAS.
- J.-C. Baret, O. J. Miller, V. Taly, M. Ryckelynck, A. El-Harrak, L. Frenz, C. Rick, M. L. Samuels, J. B. Hutchison, J. J. Agresti, D. R. Link, D. A. Weitz and A. D. Griffiths, Lab Chip, 2009, 9, 1850–1858 RSC.
- J. Lim, P. Gruner, M. Konrad and J.-C. Baret, Lab Chip, 2013, 13, 1472–1475 RSC.
- B. Kintses, L. D. van Vliet, S. R. Devenish and F. Hollfelder, Curr. Opin. Chem. Biol., 2010, 14, 548–555 CrossRef CAS.
-
W. Salser, in Genetic Engineering, CRC Press, West Palm Beach, Fla., 1978, pp. 53–71 Search PubMed.
- G. Bradski, Dr Dobbs J. Softw. Tools, 2000 Search PubMed.
-
R. C. Gonzalez and R. E. Woods, Digital image processing, Prentice Hall, Upper Saddle River, N.J., 2008 Search PubMed.
- C. Mabille, F. Leal-Calderon, J. Bibette and V. Schmitt, Europhys. Lett., 2003, 61, 708–714 CrossRef CAS.
- L. Boitard, D. Cottinet, C. Kleinschmitt, N. Bremond, J. Baudry, G. Yvert and J. Bibette, Proc. Natl. Acad. Sci. U. S. A., 2012, 109, 7181–7186 CrossRef CAS.
- A. S. Basu, Lab Chip, 2013, 13, 1892–1901 RSC.
- K. Martin, T. Henkel, V. Baier, A. Grodrian, T. Schön, M. Roth, J. M. Köhler and J. Metze, Lab Chip, 2003, 3, 202–207 RSC.
- O. Genilloud, I. González, O. Salazar, J. Martín, J. R. Tormo and F. Vicente, J. Ind. Microbiol. Biotechnol., 2011, 38, 375–389 CrossRef CAS.
- D. I. Kurtböke, Appl. Microbiol. Biotechnol., 2012, 93, 1843–1852 CrossRef.
- J. Bérdy, J. Antibiot., 2005, 58, 1–26 CrossRef.
- C. J. Ingham, A. Sprenkels, J. Bomer, D. Molenaar, A. van den Berg, J. E. van Hylckama Vlieg and W. M. de Vos, Proc. Natl. Acad. Sci. U. S. A., 2007, 104, 18217–18222 CrossRef CAS.
- Y. Akselband, C. Cabral, T. P. Castor, H. M. Chikarmane and P. McGrath, J. Exp. Mar. Biol. Ecol., 2006, 329, 196–205 CrossRef CAS.
- A. R. Abate, T. Hung, P. Mary, J. J. Agresti and D. A. Weitz, Proc. Natl. Acad. Sci. U. S. A., 2010, 107, 19163–19166 CrossRef CAS.
- M. A. Fischbach and C. T. Walsh, Science, 2009, 325, 1089–1093 CrossRef CAS.
- R. H. Baltz, J. Ind. Microbiol. Biotechnol., 2006, 33, 507–513 CrossRef CAS.
- J. A. DeVito, J. A. Mills, V. G. Liu, A. Agarwal, C. F. Sizemore, Z. Yao, D. M. Stoughton, M. G. Cappiello, M. D. Barbosa, L. A. Foster and D. L. Pompliano, Nat. Biotechnol., 2002, 20, 478–483 CrossRef CAS.
- J. Park, A. Kerner, M. A. Burns and X. N. Lin, PLoS One, 2011, 6, e17019 CAS.
- T. Kaeberlein, K. Lewis and S. S. Epstein, Science, 2002, 296, 1127–1129 CrossRef CAS.
- V. Schroeckh, K. Scherlach, H.-W. Nützmann, E. Shelest, W. Schmidt-Heck, J. Schuemann, K. Martin, C. Hertweck and A. A. Brakhage, Proc. Natl. Acad. Sci. U. S. A., 2009, 106, 14558–14563 CrossRef CAS.
- S. K. Küster, S. R. Fagerer, P. E. Verboket, K. Eyer, K. Jefimovs, R. Zenobi and P. S. Dittrich, Anal. Chem., 2013, 85, 1285–1289 CrossRef.
Footnotes |
† Electronic supplementary information (ESI) available. See DOI: 10.1039/c3lc50572c |
‡ Authors contributed equally to this work. |
|
This journal is © The Royal Society of Chemistry 2013 |
Click here to see how this site uses Cookies. View our privacy policy here.