DOI:
10.1039/C0MD00207K
(Concise Article)
Med. Chem. Commun., 2011,
2, 181-184
Scaffold-hopping from aminoglycosides to small synthetic inhibitors of bacterial protein biosynthesis using a pseudoreceptor model†
Received
7th November 2010
, Accepted 25th November 2010
First published on 10th January 2011
Abstract
Virtual screening was used to find replacements for aminoglycoside antibiotics that are able to block in vitrotranscription and translation. A pseudoreceptor model retrieved potent vanilloid derivatives as sugar-mimics. These bioactive antibiotic agents were retrieved by a two-step virtual screening process. ‘Fuzzy’ pharmacophore models allowed for finding alternative chemotypes with lower structural complexity and greater synthetic accessibility than the query.
Introduction
Increasing resistance of pathogenic bacteria to antibiotics urgently requires massive research effort to finding novel antibiotic agents.1 Computer-assisted ‘virtual screening’ can be used as a tool for identifying candidate compounds for activity screening.2 Here, we report the discovery of small synthetic inhibitors of bacterial protein biosynthesis, which were retrieved from a large compound pool using pseudoreceptor models of aminoglycosides targeting the ribosomal A-site. This study exemplifies that the scaffold-hopping concept enables the identification of simplistic mimetics of structurally more complex antibiotics by virtual screening with limited experimental effort.
Aminoglycosides like COMPOUND LINKS
Read more about this on ChemSpider
Download mol file of compoundstreptomycin inhibit protein biosynthesis by blocking the 16S RNA A-site of bacterial ribosomes. Several structurally resolved complexes between the inhibitors and the target site formed exclusively by ribosomal RNA are available from the Protein Data Bank (PDB),3 which served as a basis for our study (Fig. 1). Since automated ligand docking into an RNA environment is still unreliable with a high risk of retrieving false-positive hits,4 we decided to follow a different structure-based approach to finding small molecular aminoglycoside mimetics.
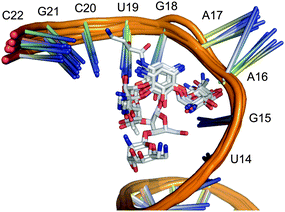 |
| Fig. 1 Alignment of 16S RNA structures with reference aminoglycosides bound to the ribosomal A-site. | |
Results and discussion
Crystal conformations of receptor-bound gentamicin C1A (PDB identifier: 2et35), COMPOUND LINKS
Read more about this on ChemSpider
Download mol file of compoundparomomycin (1j7t6), COMPOUND LINKS
Read more about this on ChemSpider
Download mol file of compoundtobramycin (1lc47), COMPOUND LINKS
Read more about this on ChemSpider
Download mol file of compoundneomycin (2et45), neamin (2f4s8), COMPOUND LINKS
Read more about this on ChemSpider
Download mol file of compoundkanamycin A (2esi5), geneticin (1mwl9), and COMPOUND LINKS
Read more about this on ChemSpider
Download mol file of compoundamikacin (2g5q10) served as reference structures. Assuming that 2-doxystreptamine is the common scaffold, their superposition was calculated by Kabsch alignment11 of atoms 2, 5, and 6 (Scheme 1). This ensemble of bioactive conformations implicitly mirrors binding site plasticity in the ligand-bound state. Then, we employed our software PRPS12 for pseudoreceptor model building. Such a model consists of potential receptor interaction sites at geometrically optimal positions around the ligand ensemble. This representation of an idealized receptor site reflects potential structural differences between the ligand-receptor complexes used for pseudoreceptor construction and might therefore be less confined to certain ligand chemotypes than models generated from single ligand conformations. Such ‘fuzzy’ pharmacophore points13 can be expected to allow for the retrieval of alternative ligand scaffolds (‘scaffold-hopping’)14 (Fig. 2). The pseudoreceptor model was used for virtually sieving through a large screening compound collection (201,007 compounds, Specs, Delft, The Netherlands). Compounds were sorted by decreasing similarity to the weighted pharmacophoric features of the pseudoreceptor. From the top-ranking candidates, ten compounds were selected, ordered and tested in an in vitrotranscription/translation assay (TTA) (Scheme 2, Table 1). Manual selection was done to ensure that i) representatives of different chemotypes were among the test set, and ii) apparent reactive groups were absent.
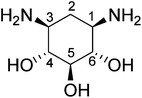 |
| Scheme 1 Common scaffold (2-doxystreptamine) of the reference aminoglycosides. | |
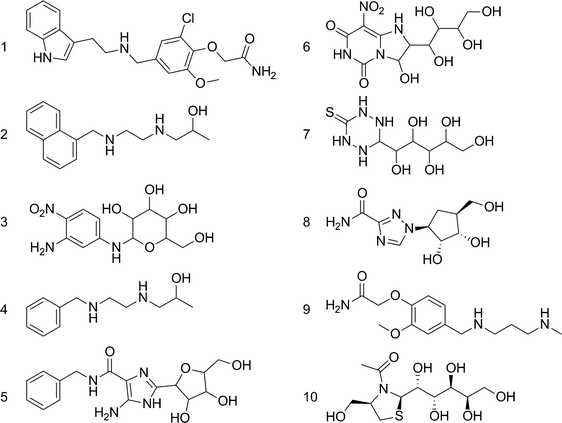 |
| Scheme 2 Compounds retrieved by pseudoreceptor screening that were selected for testing. | |
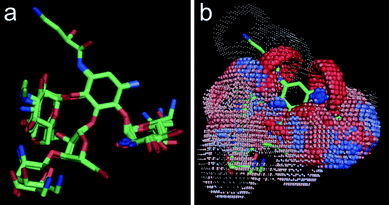 |
| Fig. 2 Alignment of A-site bound crystal conformations of aminoglycoside antibiotics (a), and the computed pseudoreceptor model of an idealized ‘A-site like receptor’ (b). Spheres indicate potential interaction sites (red: potential hydrogen-bridge acceptor; blue: potential hydrogen-bridge donor). Individual pharmacophoric features are weighted by their relevance for the underlying ligand ensemble. For actual virtual screening of a compound collection, we used a correlation-vector representation of the pseudoreceptor model. | |
Activity testing revealed several hits that reduced in vitrotranscription/translation of a reporter protein (green fluorescent protein, GFP), with compound 1 as the most potent candidate (relative assay inhibition rAI = 82%, Table 1). Surprisingly, this substance was inactive in the whole-cell assay testing minimal inhibitory concentration (MIC) against Bacillus subtilis. For preliminary exploration of the structure–activity relationship of the vanilloid derivative 1 we searched for analogs from the screening compound collection using fragments 1a and 1b as query structures (Scheme 3). A set of 17 compounds was manually retrieved by substructure searching and tested for activity in the TTA (Table S1†). We found five hits with rAI > 50%, among which compound 11 was most potent (rAI = 71%; MIC = 125 μM, 55 mg ml−1). Derivatives 11–14 were active in the Bacillus subtilis whole-cell assay with MICs between 63 and 250 μM (Table S1†). None of the vanilloid derivatives tested in the second screening round yielded greater inhibitory activity in vitro than the seed compound 1, but gained cellular antibiotic activity. Notably, among these candidates only compound 11 contains the 2,3-ether substitution, all other compounds possess the 3,4-substitution pattern present in 1. Apparently this variation does not dramatically affect inhibitory activity. Presence of a hydrogen-bond donor at the end of the hydrophobic ‘tail’ of the molecules seems to increase activity. We wish to point out that we cannot exclude the possibility that the antibiotic agents found in this study exert their activity by other mechanisms than ribosomal A-site blockade.
Aminoglycosides have been described as ligands of human vanilloid receptor 1 (TRPV1): COMPOUND LINKS
Read more about this on ChemSpider
Download mol file of compoundNeomycin blocks this Ca2+ channel with an IC50 around 400 nM.15 Here, we present evidence that vanilloid derivatives and aminoglycosides have common pharmacophoric features that could explain these observations. Moreover, the active component of red pepper (chilli, COMPOUND LINKS
Read more about this on ChemSpider
Download mol file of compoundcapsaicin 12), an alkamide, has striking resemblance to compounds 1 and 11, and is also known to exhibit antimicrobial effects and reduce Helicobacter pylori induced gastric ulcer.16 It might thus be worthwhile testing vanilloids for their potential in combating Helicobacter infection.
Conclusions
Our study demonstrates that drug-like antibiotic agents targeting the bacterial ribosome can be found by virtual screening, by starting from known antibiotic agents reference compounds. Fuzzy pharmacophore representations of molecular structure allow for retrieving alternative chemotypes with lower structural complexity and greater synthetic accessibility than the query. We were able to relate aminoglycosides to vanilloid-derivatives with respect to common targets and biological function. In this way, pharmacophore similarity searching can be used for off-target prediction.17
The pseudoreceptor approach complements the many existing tools for pharmacophore-based hit and lead structure identification.18 Although it is possible that different virtual screening tools actually retrieve similar or even identical compounds, the overlap between virtual hit lists produced by different techniques is target-dependent, and their intersection is usually small.19 This ambiguity certainly justifies and motivates to employ several such tools in parallel, and either fuse the resulting sets of high-ranking candidate compounds,20 or treat each result list separately. Here, we have shown how straightforward two-step virtual screening can be used to rapidly retrieve compounds with a desired activity from a large compound pool with limited experimental effort.
Experimental
Computational procedure
The initial alignment of aminoglycoside reference compounds was generated using the Kabsch algorithm11 in PyMol v0.99 (DeLano Scientific, San Francisco, USA). Pseudoreceptor modeling and similarity searching was perfomed using PRPS as described.12 Single 3D conformations of the screening compounds (Specs NP, Delft, The Netherlands) were computed using CORINA v.3.2 (Molecular Networks GmbH, Erlangen, Germany).
In vitro
transcription/translation assay
Assay components were obtained from RTS 100 Escherichia coli (E. coli) HY Kit (Roche, Germany). It contains E. coli lysate, reaction mix, amino acid mixture, COMPOUND LINKS
Read more about this on ChemSpider
Download mol file of compoundmethionine, reaction buffer and a template vector coding for the reporter protein GFP (green fluorescent protein). The translation system was prepared as recommended by the manufacturer (solvent A). Then, test substances were dissolved in COMPOUND LINKS
Read more about this on ChemSpider
Download mol file of compoundDMSO. The assay volume of 15 μl contained 14 μl solvent A and 1 μl test substance solution. Concentrations were adjusted to 5 mM for pre-screening and 500 μM for the final screening. Negative controls were prepared by adding 1 μl COMPOUND LINKS
Read more about this on ChemSpider
Download mol file of compoundDMSO to solvent A. As a positive control, 1 μl of the protein biosynthesis inhibitor COMPOUND LINKS
Read more about this on ChemSpider
Download mol file of compoundchloramphenicol was dissolved in COMPOUND LINKS
Read more about this on ChemSpider
Download mol file of compoundDMSO and mixed with 14 μl solvent A. The concentration of 600 μM in the assay volume inhibits the GFP synthesis completely. All experiments were performed in triplicates. Samples were incubated four hours at 30 °C. After a 40-fold dilution with COMPOUND LINKS
Read more about this on ChemSpider
Download mol file of compoundwater, GFP was quantified by measuring the fluorescence intensity (FI; excitation: 395 nm, emission: 509 nm) using a Hitachi F-4500 fluorescence spectrophotometer. Inhibition of GFP biosynthesis causes no or only weak fluorescence at 509 nm. Successful synthesis results in a strong signal. Relative assay inhibition rAI [%] was calculated by dividing the relative fluorescence intensity measured for a test substance by the relative fluorescence intensity of the positive control (600 mM COMPOUND LINKS
Read more about this on ChemSpider
Download mol file of compoundchloramphenicol). The relative fluorescence gives the ratio of the fluorescence intensities measured for a test substance and the negative control (COMPOUND LINKS
Read more about this on ChemSpider
Download mol file of compoundDMSO). Absolute error values were calculated from standard deviations.
Whole cell assay for MIC determination
MICs were determined by the microtiter broth dilution method21 using Bacillus subtilis 168. The bacterium was grown in sterile 96-well plates (Greiner Bio-One, Frickenhausen, Germany). The TY-medium contained 5 g l−1tryptone, 8 g l−1 yeast extract (Formedium Ltd., Hunstanton, England), and 5 g l−1sodium chloride (Sigma Aldrich, Germany). Each compound was tested at 11 concentrations between 500 μM and 0.5 μM. As a control, the same dilution steps were performed with pure solvent. After an incubation period of 20 h at 37 °C, cell growth was specified by measuring the optical density of each well. The data were acquired at 620 nm using a microplate reader (SpectraMax M5, Molecular Devices, MDS Analytical Technologies).
Acknowledgements
We are grateful to the Chemical Computing Group Inc. (Montreal, Canada) for generous software support. G.S. was supported by the DFG (FOR 1406, TP4). E.P. is grateful to LOEWE Lipid Signaling Foschungszentrum Frankfurt (LiFF) for financial support.
Notes and references
-
(a) A. Yonath, Annu. Rev. Biochem., 2005, 74, 649–679 CrossRef CAS;
(b) L. D. Högberg, A. Heddini and O. Cars, Trends Pharmacol. Sci., 2010 DOI:10.1016/j.tips.2010.08.002 , in press.
-
(a) M. M. Hann and T. I. Oprea, Curr. Opin. Chem. Biol., 2004, 8, 255–263 CrossRef CAS;
(b) R. E. Amaro and W. W. Li, Current Topics in Medicinal Chemistry, 2010, 10, 3–13 Search PubMed;
(c) G. Schneider, Nat. Rev. Drug Discovery, 2010, 9, 273–276 CrossRef CAS.
- H. M. Berman, J. Westbrook, Z. Feng, G. Gilliland, T. N. Bhat, H. Weissig, I. N. Shindyalov and P. E. Bourne, Nucleic Acids Res., 2000, 28, 235–242 CrossRef CAS.
-
(a) P. Pfeffer and H. Gohlke, J. Chem. Inf. Model., 2007, 47, 1868–1876 CrossRef CAS;
(b) P. T. Lang, S. R. Brozell, S. Mukherjee, E. F. Pettersen, E. C. Meng, V. Thomas, R. C. Rizzo, D. A. Case, T. L. James and I. D. Kuntz, RNA, 2009, 15, 1219–1230 CrossRef CAS.
- B. François, R. J. M. Russell, J. B. Murray, F. Aboul-ela, B. Masquida, Q. Vicens and E. Westhof, Nucleic Acids Res., 2005, 33, 5677–5690 CrossRef CAS.
- Q. Vicens and E. Westhof, Structure, 2001, 9, 647–658 CrossRef CAS.
- Q. Vicens and E. Westhof, Chem. Biol., 2002, 9, 747–755 CrossRef CAS.
- J. B. Murray, S. O. Meroueh, R. J. M. Russell, G. Lentzen, J. Haddad and S. Mobashery, Chem. Biol., 2006, 13, 129–138 CrossRef CAS.
- Q. Vicens and E. Westhof, J. Mol. Biol., 2003, 326, 1175–1188 CrossRef CAS.
- J. Kondo, B. François, R. J. M. Russell, J. B. Murray and E. Westhof, Biochimie, 2006, 88, 1027–1031 CrossRef CAS.
- W. Kabsch, Acta Crystallogr., Sect. A: Cryst. Phys., Diffr., Theor. Gen. Crystallogr., 1976, 32, 922–923 CrossRef.
-
(a) Y. Tanrikulu, E. Proschak, T. Werner, T. Geppert, N. Todoroff, A. Klenner, T. Kottke, K. Sander, E. Schneider, R. Seifert, H. Stark, T. Clark and G. Schneider, ChemMedChem, 2009, 16, 258–266;
(b) Y. Tanrikulu and G. Schneider, Nat. Rev. Drug Discovery, 2008, 7, 667–677 CrossRef CAS.
-
(a) S. Renner and G. Schneider, J. Med. Chem., 2004, 47, 4653–4664 CrossRef CAS;
(b) S. Renner, V. Ludwig, O. Boden, U. Scheffer, M. Göbel and G. Schneider, ChemBioChem, 2005, 6, 1119–1125 CrossRef CAS.
-
(a) G. Schneider, W. Neidhart, T. Giller and G. Schmid, Angew. Chem., Int. Ed., 1999, 38, 2894–2896 CrossRef CAS;
(b) R. D. Cramer, R. J. Jilek and K. M. Andrews, J. Mol. Graphics Modell., 2002, 20, 447–462 CrossRef CAS;
(c) S. R. Langdon, P. Ertl and N. Brown, Mol. Inf., 2010, 29, 366–385 Search PubMed.
- M. Raisinghani and L. S. Premkumar, Pain, 2005, 113, 123–133 CrossRef CAS.
-
(a) R. H. Cichewicz and P. A. Thorpe, J. Ethnopharmacol., 1996, 52, 61–70 CrossRef CAS;
(b) J. Molina-Torres, A. García-Chávez and E. Ramírez-Chávez, J. Ethnopharmacol., 1999, 64, 241–248 CrossRef CAS;
(c) M. N. Satyanarayana, Crit. Rev. Food Sci. Nutr., 2006, 46, 275–328 CrossRef CAS.
-
(a) D. Vidal, R. Garcia-Serna and J. Mestres, Methods Mol Biol., 2011, 672, 489–502 CrossRef CAS;
(b) R. Steri, P. Schneider, A. Klenner, M. Rupp, M. Schubert-Zsilavecz and G. Schneider, Mol. Inf., 2010, 29, 287–292 Search PubMed.
- T. Langer, Mol. Inf., 2010, 29, 470–475 Search PubMed.
-
(a) D. M. Krüger and A. Evers, ChemMedChem, 2010, 5, 148–158 CrossRef;
(b) P. Willett, Drug Discovery Today, 2006, 11, 1046–1053 CrossRef CAS.
- B. Chen, C. Mueller and P. Willett, Mol. Inf., 2010, 29, 533–541 Search PubMed.
-
D. Amsterdam, in: V. Lorian (ed.), Antibiotics in Laboratory Medicine, 4th ed. Williams & Wilkins, Baltimore, pp. 52–111 Search PubMed.
Footnote |
† Electronic supplementary information (ESI) available. See DOI: 10.1039/c0md00207k |
|
This journal is © The Royal Society of Chemistry 2011 |